Edge computing is a crucial aspect of computer vision that allows for data processing to occur at the edge of a network, rather than in the cloud or a data center. This technology is becoming increasingly important as the demand for real-time processing and low-latency applications grows.
In this article, we explore the benefits and applications of edge computing in the field of artificial intelligence (AI) and computer vision. Particularly, we will cover:
- What edge computing is
- Benefits of edge computing for machine learning and computer vision
- How edge computing works with the cloud
About us: Viso.ai provides the leading end-to-end Computer Vision Platform Viso Suite. Our technology automates edge computing infrastructure to build and scale computer vision applications. Get a demo for your company.

Distributed Computing
Edge computing is essential to computer vision processes for bandwidth, speed, and security purposes. Edge computing tends to be a more efficient, more secure option for data processing related to computer vision.
Although most data processing can happen at the edge, we send data to the cloud for further analysis (partial data offloading). In addition, data sent back to the cloud can be aggregated and used for business analytics tasks.
What Is Edge Computing?
Edge computing describes computing that occurs near the source of data, rather than depending on cloud networks far from data access points to do all necessary computing. End devices refer to micro-servers, Edge-servers, computing devices, phones, laptops, and TVs, and include any device that shows the front end of data processing.
Meanwhile, computer vision is used to fulfill any tasks that would usually require the human eye. Edge computing is essential to computer vision operations because it improves response time, thus reducing bandwidth, and is more secure than most cloud computing platforms.
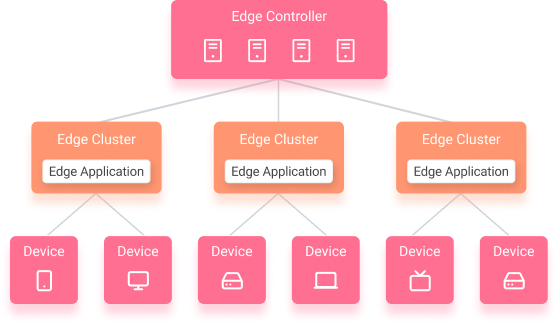
Why Is Edge Computing Useful?
- Real-time and reduced latency. Since edge computing allows data to be closer to the endpoints of networks, data can be accessed in real-time with little to no buffer. This allows computer vision operations in remote and urban places to have faster response times. Locations without efficient network infrastructure can also be added quickly. For example, a hospital in a semi-rural location using robotic surgery would need access to its computer vision data in real-time. Having the 6-9 second buffer that occasionally comes with a cloud connection would be detrimental to time-sensitive use cases such as this one.
- Bandwidth. Edge computing also has no dependency on a central data center or network bandwidth. Processing data through edge computing allows for reduced data transmission costs since you wouldn’t need to access the cloud nearly as much.
- Data privacy. Computer vision and video analytics are based on video data. Video data is sensitive because it involves people’s personal identification information and other secure images. Sending video data to the cloud and storing it there increases data security risks. Edge computing processes the video material, and no video data needs to be sent to the cloud or stored in the cloud. The risk of exposure to security threats is reduced since edge computing distributes the risk of exposure across multiple devices. Edge computing can perform all processing disconnected from a central server. Therefore, its private architecture allows it to be more secure. Autonomous vehicles utilize computer vision data regularly. Having on-vehicle edge computing rather than cloud-stored computing reduces the chances of an automated vehicle getting hijacked. Additionally, data isn’t shared with multiple developers. This element of privacy makes it much more difficult for hackers to attack edge computed systems.
What Are the Disadvantages of Edge Computing for AI Vision?
Involving all edge endpoints requires complex, distributed computing architectures. The need for integrated edge device management, monitoring, and configuration makes edge computing complex to implement and maintain.
Unlike cloud architecture, which is centralized around one common cloud, edge computing is distributed. The integration of multiple computing nodes requires networking and communication. The increased complexity requires fine balancing and optimization.
Compared to cloud-only architectures, edge computing enables large-scale systems with increased complexity. As a result, edge computing applications are more difficult and costly to build and maintain.
However, managed and automated infrastructure platforms help overcome the challenges of Edge Computing. Edge computing platforms enable organizations to utilize the benefits of both cloud and edge and to compute workloads in the most cost-efficient and scalable way.
What’s Next?
Due to the security and speed of edge computing regarding computer vision, it is an ever-growing industry and one of the major trends in IT. Therefore, more and more companies are implementing edge computing in combination with cloud services with their data because of its streamlined approach.
- Read more about Edge Intelligence and moving Deep Learning tasks to the edge.
- Explore the Most Popular Computer Vision Applications.
- A complete Guide about Edge AI.