The strategic importance of AI has led to an increased need for video analytics. Given the enormous potential of AI vision, attention is shifting from traditional video surveillance to process automation with computer vision.
About Video Analytics
Video analytics, or video analysis, is the process of extracting useful information from video footage. This can be anything from counting the number of people in a video to identifying specific objects or individuals. Modern video analytics applies computer vision, which is a field of artificial intelligence that deals with the analysis of digital images and videos.
There are several different applications for video analytics across different industries. In many use cases, video content analysis makes it possible to automate tasks that would otherwise be done manually, such as counting the number of people in a video or identifying specific objects across multiple live stream cameras.
Video Analysis With Deep Learning
Deep learning is a subset of machine learning that uses neural networks to learn patterns in data. Neural networks are composed of layers of interconnected processing nodes with multiple layers.
The rapid advances in deep learning have shown great success in applying AI for video analysis. In particular, deep learning algorithms are used to detect and track objects in videos, as well as to recognize specific actions.
Object Detection in Video Analytics
One of the most common applications of deep learning for video analysis is object detection and tracking. This involves detecting and tracking specific objects in a video recognition sequence. Popular techniques include the use of a convolutional neural network (CNN) to learn complex patterns from data.
Real-time Object Detection in video streams has been one of the most important computer vision tasks. The most popular algorithms to detect objects in video data include Mask R-CNN, YOLOv3, YOLOR, and YOLOv7. They can be custom-trained with collected video data or pre-trained on large image datasets such as MS COCO.
Such deep learning models are used in software for analyzing video and detecting and tracking objects for trained classes, such as vehicles, people, traffic lights, etc., in real-time. More advanced video analytics software provides functionality for object counting and rule-based analysis, for example, to perform people-counting in areas with large crowds.
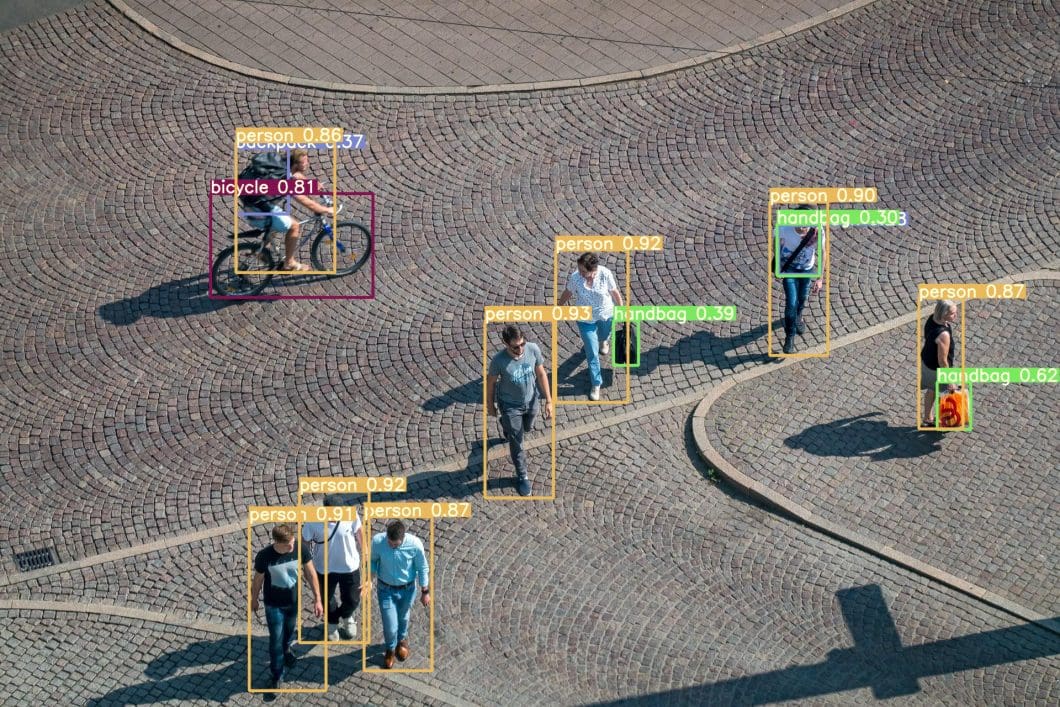
Action Recognition in Video Analysis
Another typical application of deep learning for video analysis is action recognition. This involves recognizing specific actions in a video sequence or real-time video streams. Deep learning models can be trained to classify actions performed in different contexts or environments.
Video recognition or motion detection analysis is very popular for detecting activities in a scene by analyzing a series of video frames. Techniques for video motion detection or progress analysis include frame referencing or pixel matching to detect horizontal and vertical changes between a set of images or video frames.
More advanced methods apply video recognition/understanding, pose estimation, emotion analysis, or face recognition to analyze and understand the context of video data. However, those advanced AI tasks require significant computing resources and sophisticated software infrastructure.
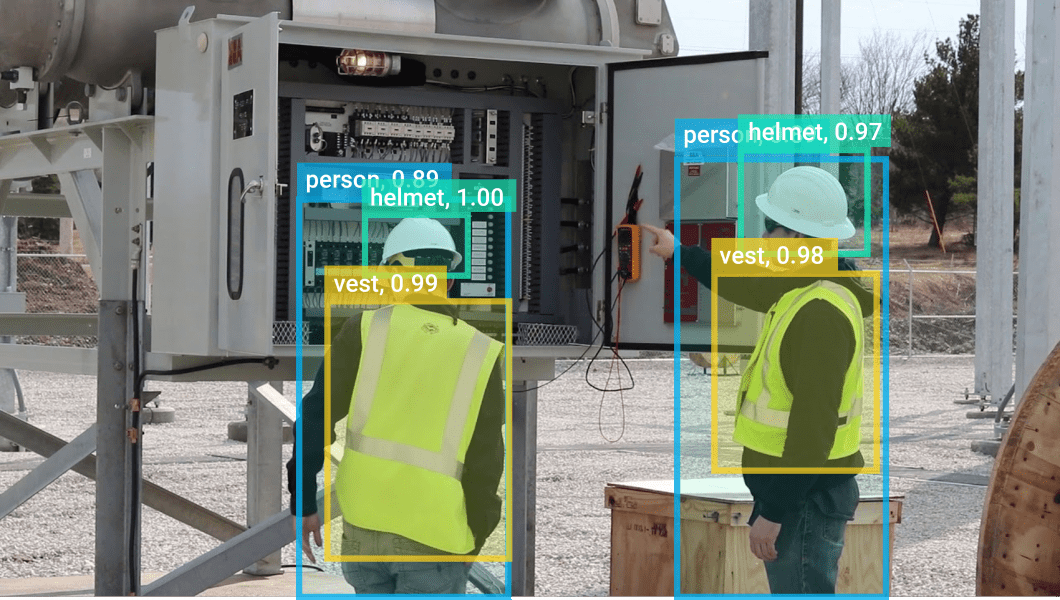
Video Management System (VMS) and Artificial Intelligence
Novel AI-powered video analytics are rapidly gaining popularity. Key adopters include those who have been operating traditional video surveillance systems even before the emergence of Artificial Intelligence (AI), and those looking to adopt disruptive technologies for automation.
It is important to distinguish between video management systems that mainly manage camera streams and computer vision systems that focus on video recognition and the application of advanced real-time video analysis to solve business tasks.
Video Management System (VMS)
A VMS is a software application that manages and records video from security cameras. A VMS can provide a single interface for viewing live and recorded video from many cameras, as well as search, playback, and export capabilities. Most VMS applications are designed to work with a specific type of camera, such as IP cameras or CCTV cameras. Popular VMS providers include Milestone, Avigilon, Axis, Bosch, Dahua, Hikvision, Honeywell, and Pelco.
Most VMS providers are not cloud or AI-native companies and have provided products for video surveillance before the emergence of artificial intelligence. However, more and more video management systems face the need to add video analytics capabilities to support manual operators who have to monitor video streams. Such features include face and person detection, and automated tagging or alerts.
Computer Vision Systems
A computer vision system uses image processing algorithms in multi-step computer vision pipelines to analyze images in order to extract information from video data. Computer vision systems can solve complex and business-specific tasks that involve person or object detection, facial recognition, activity recognition, quality inspection, and so on. Computer vision systems can acquire video input from cameras or VMS. Check our list of popular computer vision companies.
With the rise of AI technology, computer vision is becoming a strategic issue across industries. As a result, companies have started to implement a portfolio of computer vision applications to automate tasks using AI video analytics. While there are point solutions, companies tend to develop custom computer vision systems to meet business requirements for system integration, flexibility, cost-efficiency, data privacy, performance, and cost-efficiency.
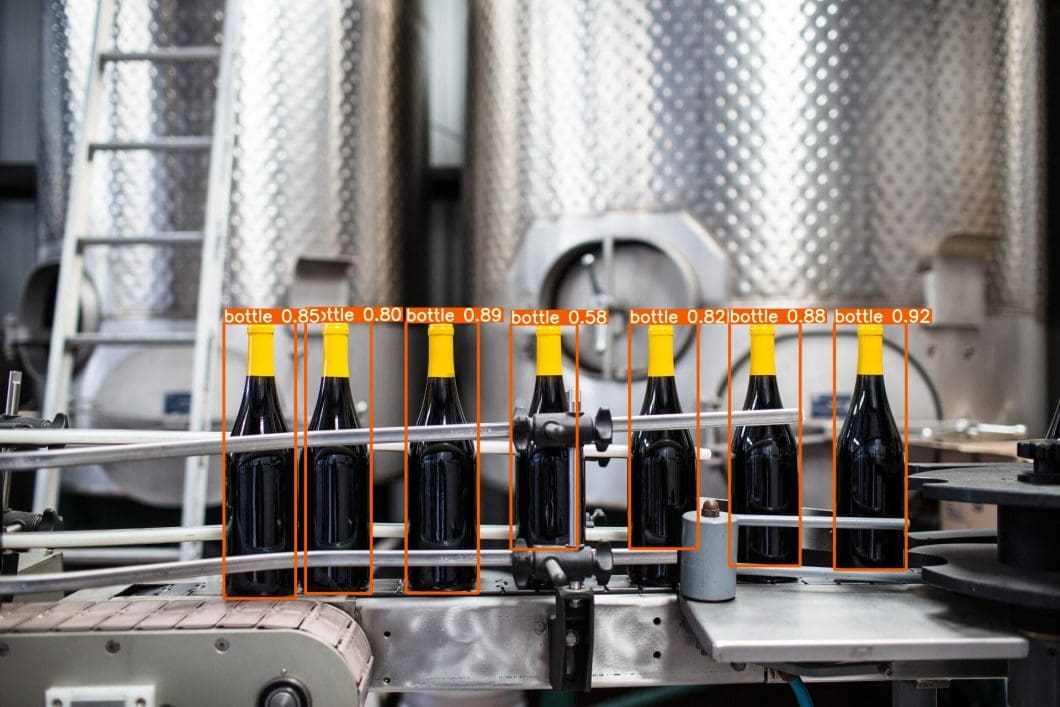
Platforms for AI Video Analytics
The high complexity of computer vision with machine learning drives the need for new infrastructure and computing methods such as Edge AI. Such distributed Edge Computing concepts improve the robustness, scalability, and efficiency of analyzing video with machine learning. Hence, computer vision platforms have been introduced that allow businesses to develop and deliver custom video analytics applications that integrate with existing cameras and VMS.
At viso.ai, we power the leading AI enterprise video analytics platform, Viso Suit,e to rapidly develop, deploy, and manage real-world solutions. The model-driven architecture abstracts cameras and AI models of all types to develop high-performing applications with building blocks. The vision applications can be deployed at scale to edge devices and process a large number of camera streams in real-time.
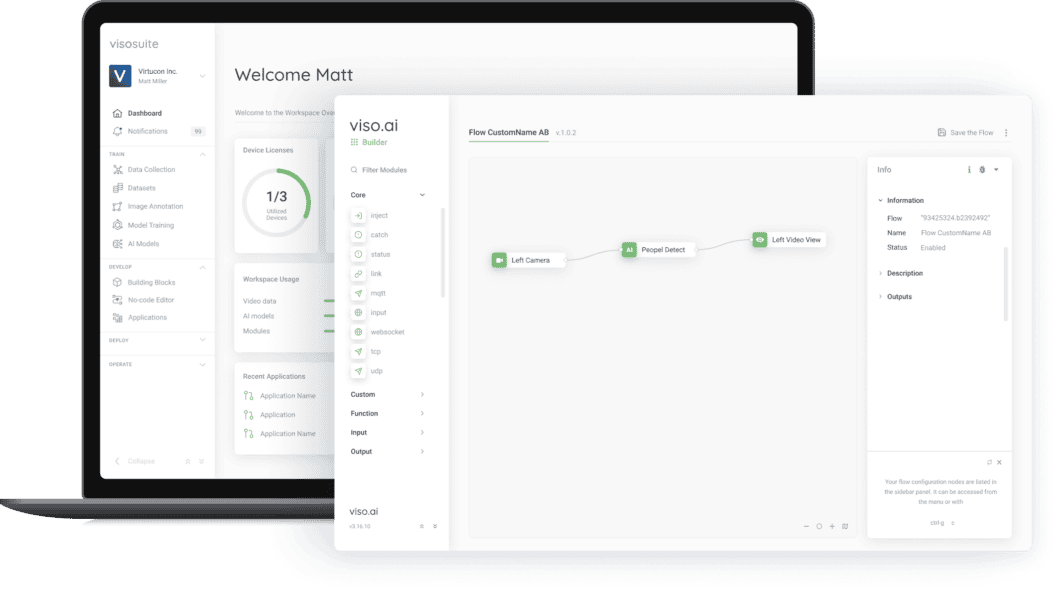
The largest applications in the video analytics market involve security: incident detection, intrusion management, people counting, traffic monitoring, Automatic Number Plate Recognition (ANPR), facial recognition, AR, and ego-motion estimation. In addition, video analytics has been useful for industries such as manufacturing, security, retail, healthcare and hospitality, and more.
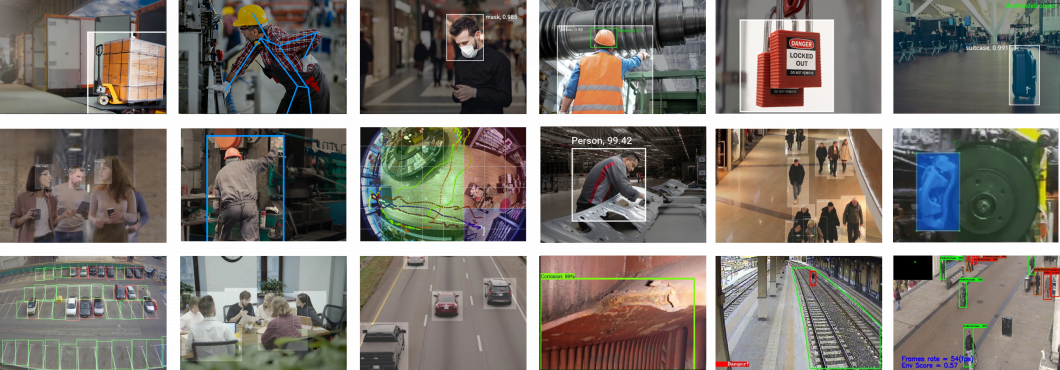
Security Applications
Video analytics has been working to provide solutions for surveillance and security by creating a general means for identifying and detecting different objects in video streams. Such technology is useful for tracking people or objects of interest in videos or identifying and detecting intruders. Using AI video analytics for these purposes allows certain objects to be flagged and alarms to be raised on suspicious behavior.
Vertical Motion Detection
A specific instance of video analytics for security could be a fence-climbing detection system. Security staff are usually trained to know that people walking outside a fence is considered regular, but climbing on top of or struggling with the fence is irregular.
Video analytics software trained to recognize the subtle differences in motion direction between the regular and irregular behavior involving the fence can be linked to the real-time video feed from security cameras.
If someone were to begin climbing the fence, the software would recognize the vertical motion as an abnormal occurrence and create an alarm of some sort. Comparatively, if someone were to walk next to the fence, they would be creating horizontal motion, which is not classified as suspicious activity by the detection system.
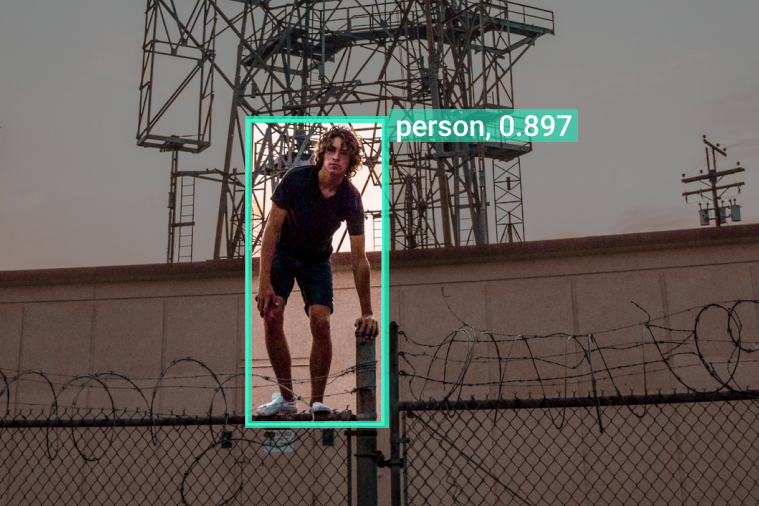
There exist multiple video analytics applications in different variations. For example, person detection with object tracking can be used to detect a person climbing a fence in an area of view. In this application, the video analytics capabilities are based on integrated object detection algorithms that run directly on-device instead of an external server to perform detection in real-time (Edge Computing).
A variety of rules can be run simultaneously and can send alerts directly from the camera via text message, email, or to a video management system.
Object Classification
Video feed object classification involves detecting dangerous objects in a live camera feed or a given video. Small differences between objects that are sometimes even hard to see by security guards in front of a camera can be detected by video analytics programs trained to find minuscule differences that could make the difference between a hazardous and safe object.
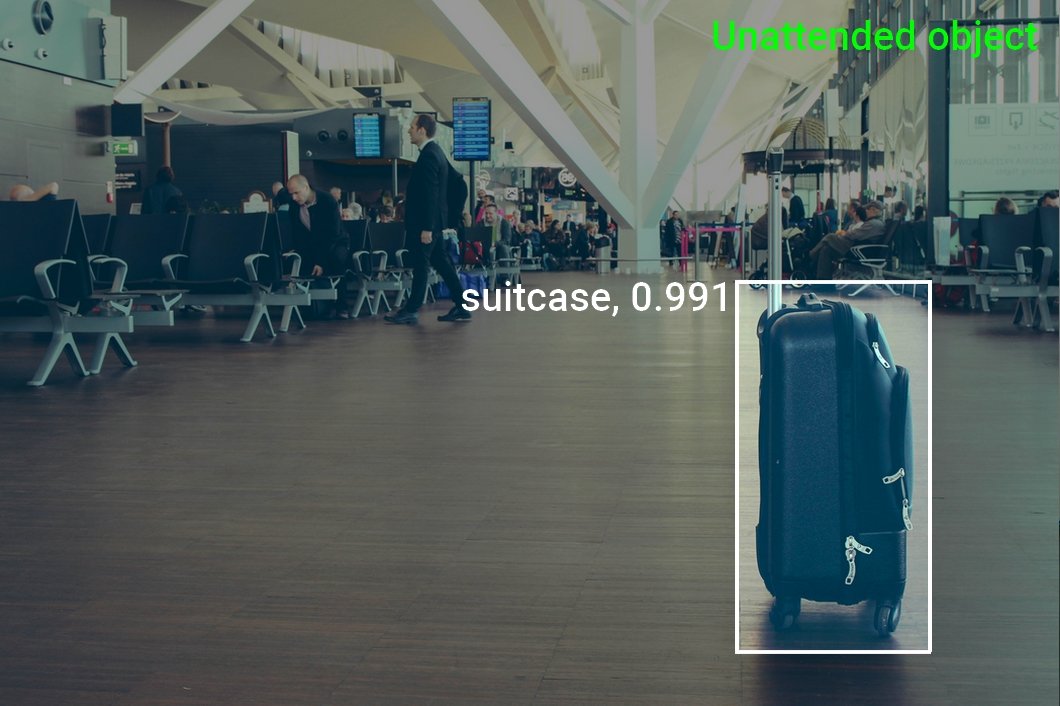
X-ray security screening, for example, can use video analytics programs trained to do object classification on real-time feeds of baggage at security check-ins to identify specific objects of interest, such as sharp tools or weapons. Such technology has already been implemented worldwide as its accuracy increases. The Transportation Security Administration (TSA) introduced computed tomography scanners (CT) with state-of-the-art 3-D technology at U.S. airport checkpoints (check more video analytics applications in Aviation).
AI video technology is currently being enhanced to increase the accuracy of detecting objects in video frames in a wide range of real-world computer vision applications.
Behavior Tracking
Similar to the motion detection discussed in the fence example, other kinds of behavior are also relevant grounds for video analytics to be able to classify. For example, behavior tracking involves human behavior concerning both themselves and larger objects, such as vehicles, and what it entails for the safety of a general area. The following are two smaller-scale examples of behavior tracking implemented in video analytics.
- Loitering Detection: In Smart Cities, video analytics are trained to notice when people or vehicles remain in a defined zone longer than the user-defined time allows. For the safety of the area, an alarm could be activated depending on the preferences of the program implementer. This behavior is effective in the real-time notification of suspicious behavior around pharmacy departments, ATMs, narcotic dispensaries, and other locations.
- Stopped Vehicle Detection: This portion of video analytics is useful for preventing vehicles from idling or stopping in an unauthorized location for prolonged periods. Vehicles stopped near a sensitive area longer than the user-defined time allow are detected. This behavior is ideal for stopping vehicles from obstructing loading and receiving docks, enforcing parking rules, and decreasing vehicle wait time at valet services or parking gates. Stopped vehicles in moving roadways can also indicate unreported accidents or vehicle issues, and such technology can alert the proper authorities to the instances.
- Camera Sabotage: Advanced video loss detection can recognize when a live video stream has been compromised or tampered with. For example, if a vandal paints or covers a lens or reaches to move a fixed camera away from an intended scene, an alarm is triggered.
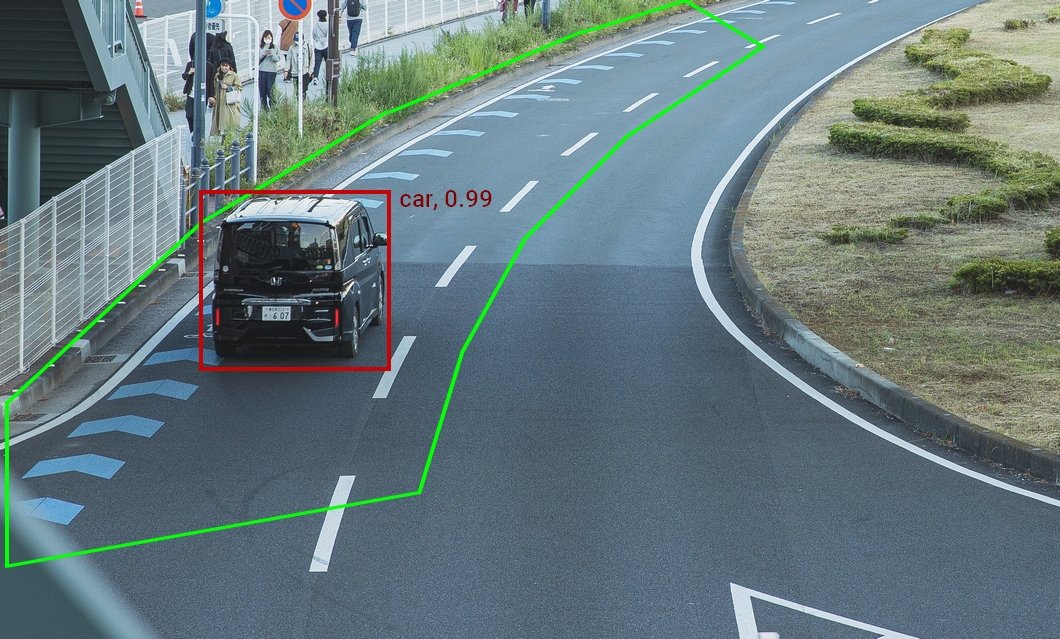
Retail Applications
The retail industry can implement AI analytics for video streams in multiple situations. These components of retail management help streamline operations and create better customer experiences without increasing human responsibility or adding other operational costs relating to expensive equipment. Explore our extensive article about visual AI in retail.
Intelligent Queue Management
Video analytics provides information on better policies for checkouts and can even set stores up for checkout-free capabilities. It allows stores to conduct self-checkout and honor-code activities without the fear of shoplifting or other nefarious infringements. Queue management can also provide insights into what is and isn’t working to manage the size of queues throughout stores. During the pandemic, for example, queue management could be essential for preventing the spread.
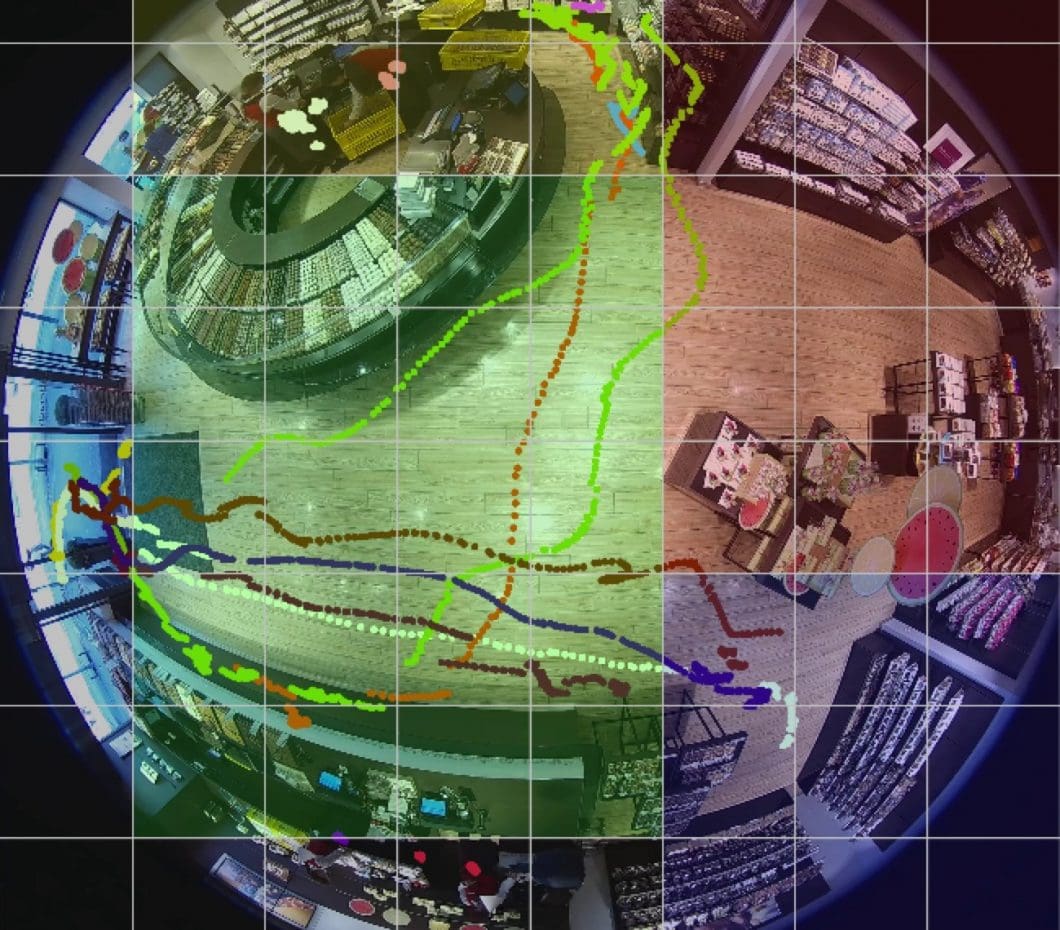
People Counting
People counting can be conducted using video analytics. Retail involves a lot of experimenting with displays and marketing strategies. Observing or having access to how many customers come in and when is helpful for stores to know what is working in terms of marketing and product overview.
In addition, noticing how many customers spend prolonged periods near displays is useful for the store because it improves the customer experience and business for the store. In terms of people counting, video analytics provides operational insights and branding insights and reveals a host of other aspects of customer relationships.
Healthcare Applications
Healthcare institutions have always prioritized having up-to-date technology to streamline costs and ensure the safety of their practices because healthcare, as an industry, is regulated by strict government and corporate legislation. AI video analytics implementations can be useful in healthcare for mental health, the accuracy of diagnosis, and monitoring elderly or young patients in hospitals. Explore more healthcare AI video applications.
At-home Patient Monitoring
Surveillance technology makes monitoring elderly patients in care homes feasible and convenient for caretakers. Falls are a major cause of injury and death in older persons, which is why at-home monitoring is useful for detecting unusual positions or periods in which a person is on the floor or incapacitated.
Personal medical devices can detect falls efficiently but require being worn at all times for effectiveness. Video analytics for AI fall detection provide a more hands-free solution and can be modified to do more than just detect falls. For example, such a system could also determine if an elderly took a given medication when they were supposed to.
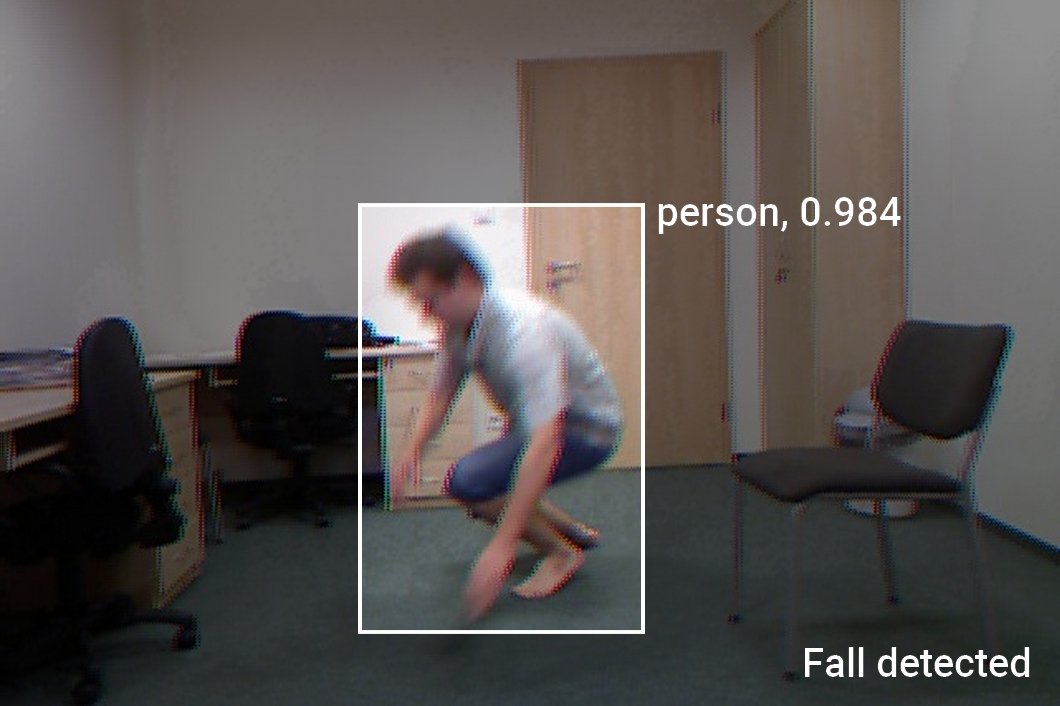
Mental Health Analysis
Combining advanced video analytics and machine learning with facial analysis and the expertise of human clinicians could enhance a healthcare provider’s ability to get to the right conclusion about a patient’s state of mental health. A prominent approach includes facial emotion analysis with AI. Video analytics can be trained to pinpoint differences in normal and abnormal facial or physical behavior.
Healthcare professionals often record these nonverbal communications as part of their prognosis, but in a fairly subjective manner and only if they notice them. Video analytics in mental health applications ensure that subtle hints in a patient’s behavior do not go unnoticed.
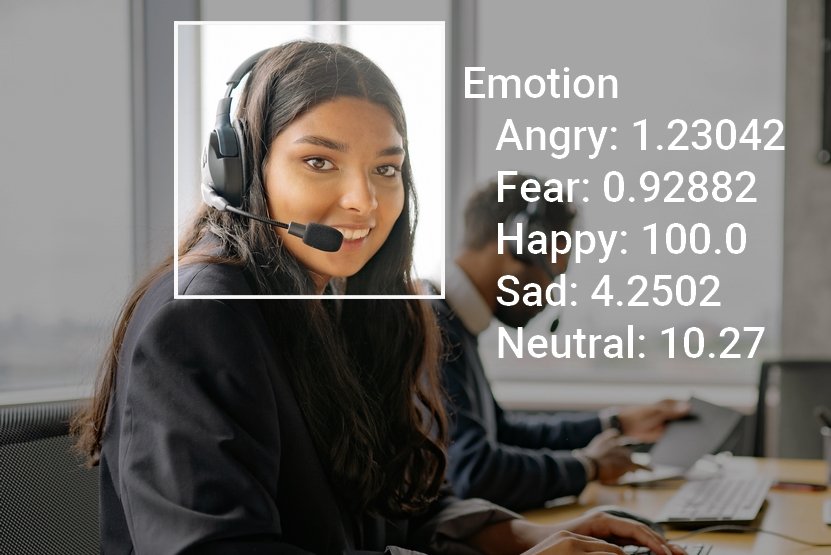
Biotechnology
Early screening of foodborne pathogens is key to ensuring food safety. Biosensors that aim to detect Salmonella through smartphone video processing and fluorescence labeling are currently being researched. Video analytics can also analyze live feeds of bacteria and identify certain bacteria from others, making it useful for identifying differences in bacterial composition.
Smart City Applications
Real-time video analysis with deep learning algorithms has prominent use cases in smart cities. Read our article about a state-of-the-art list of the best and most valuable applications of computer vision in smart cities.
Multiple companies involved in video analytics are trying to develop more integrated solutions that have to do with cities. At viso.ai, provide vision technology to cities and public service providers across the world, from Greenland to Switzerland, the United States, and Australia. Various distributed cameras of various types can be integrated to provide city operators with constant feedback to make informed decisions.
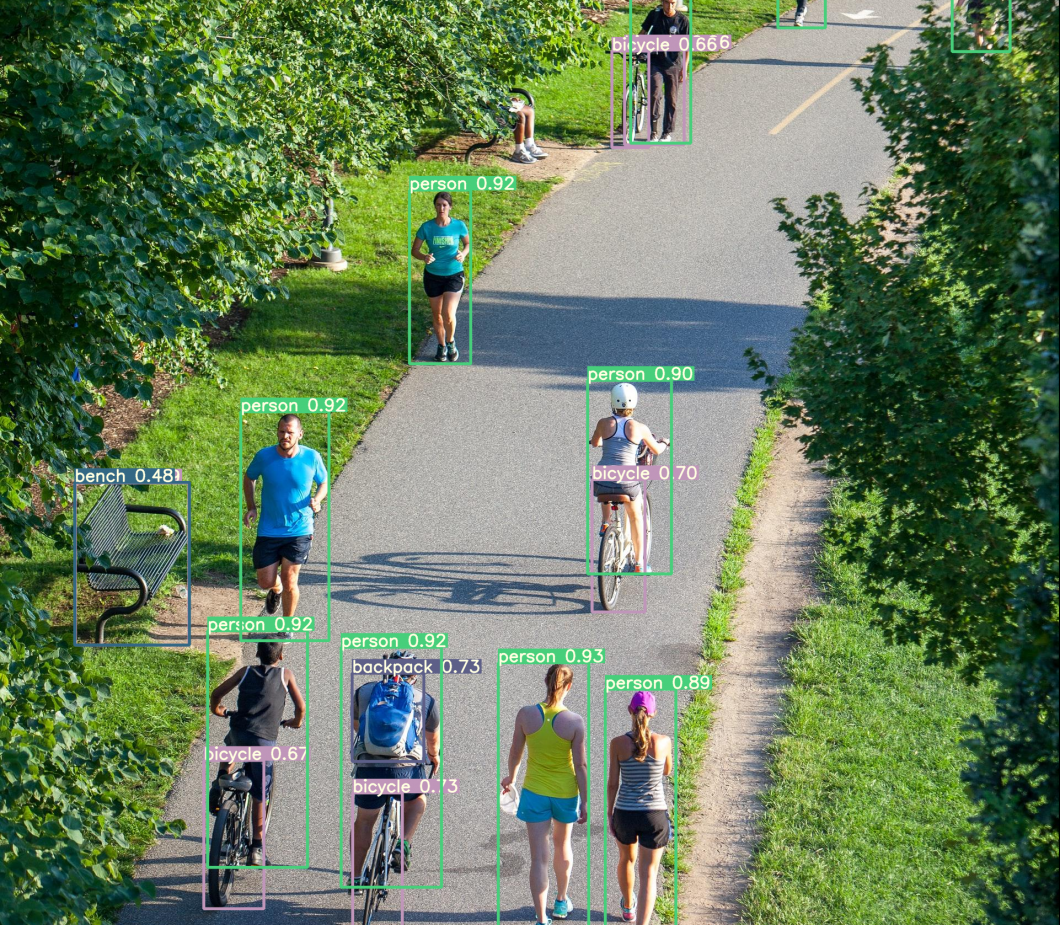
City agencies can gain more citizen engagement and optimize operations through real-time data intelligence and intra-agency collaboration. From an economic standpoint, smart city facilitations drive new revenue streams and economic development by enhancing customer activity and behavior awareness.
Video analytics are useful for cities and towns that manage crowds of people and are part of the smart city model. Automatic Number Plate Recognition and traffic monitoring are two examples of video analytics being used within cities. These applications streamline otherwise cumbersome processes that require sufficient human intervention.
Vehicle License Plate Recognition
Automatic Number Plate Recognition (ANPR) consists of accurate systems capable of reading vehicle number plates without human intervention. Using high-speed image capture while supporting the illumination makes it possible for video analytic systems to detect and read plate numbers in near real-time.
Therefore, the characters of the license plates are recognized using Optical Character Recognition (OCR), converting the images into digital text strings. This makes it possible for video analytic systems to detect and record plate numbers. Modern ANPR programs create metadata sets for every detected license plate for the authorities to reuse in other systems. ANPR is useful for recording cars running red lights, traffic mishaps, and more.
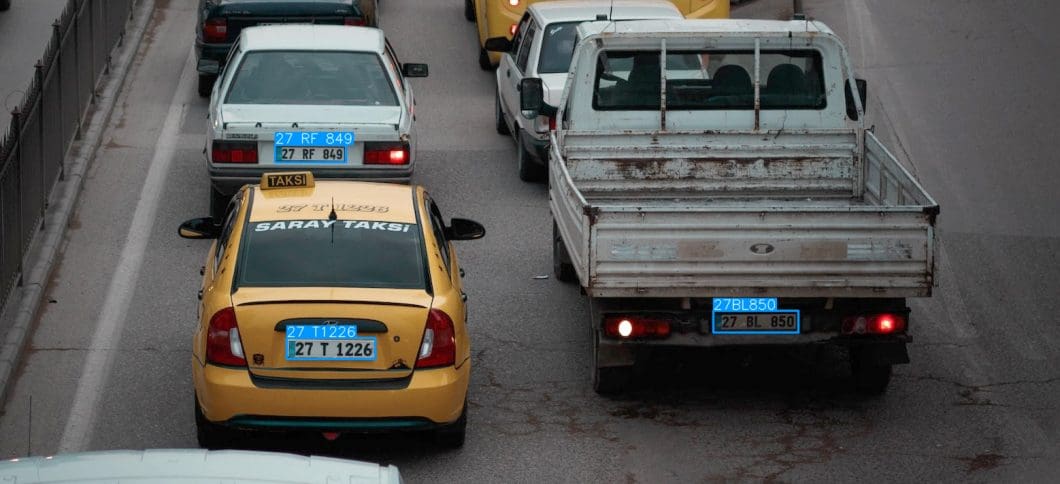
Intelligent Traffic Monitoring
Video analytics can provide insights useful for analyzing traffic and monitoring traffic jams. In addition to detecting dangerous accidents and situations, traffic monitoring gives quantitative insights into the number of vehicles in areas at specific times and traffic patterns.
In the case of an accident, these analytical systems involving traffic analysis later provide police with assistance for collecting evidence in case of litigation.
Vehicle Counting
This aspect of video analytics involves differentiating between cars, trucks, buses, and taxis to generate helpful statistics used to obtain insights about traffic. Network cameras can record the concentration of fast-moving cars in one area compared to another, which can be helpful for the city to know which implementations of traffic control are effective. Vehicle counting also provides insights into when future road maintenance needs to occur.
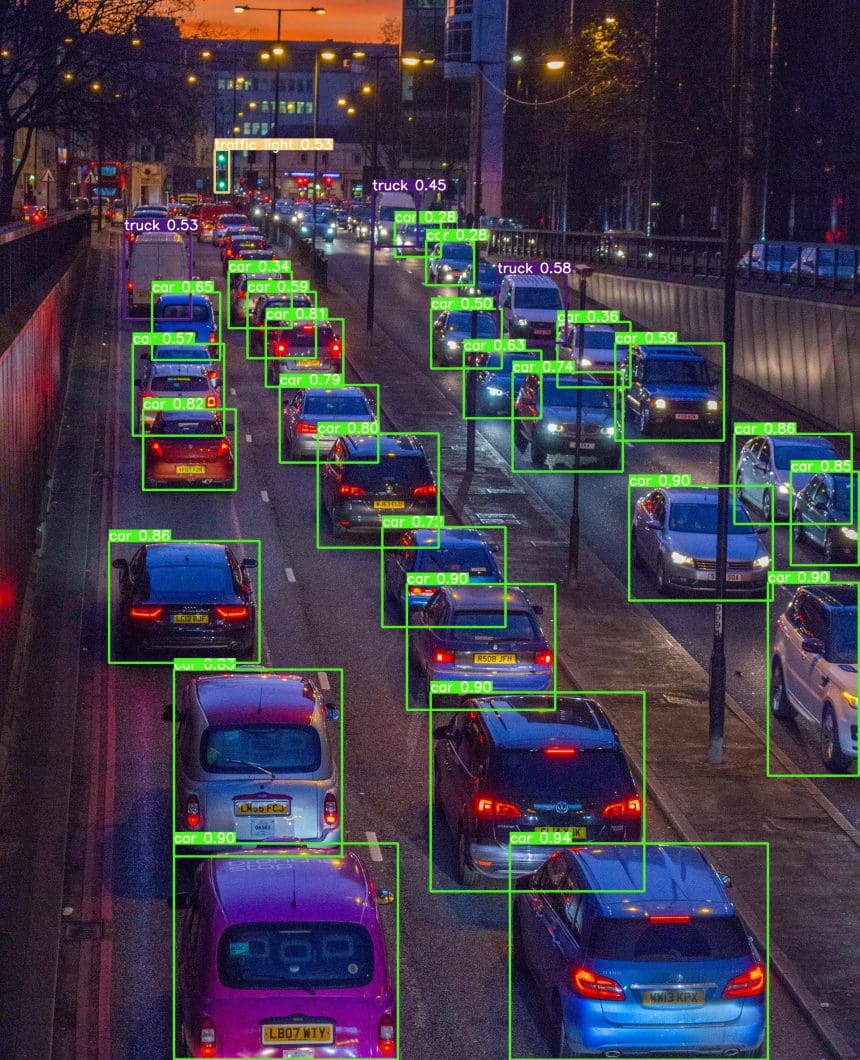
What’s Next? – Your AI Video Analytics Project
Video analytics remains an interesting aspect and application of computer vision as a part of visual artificial intelligence. Explore more video analysis solutions that you can build, customize, deploy, and operate with the Viso Suite platform.
If you enjoyed this article, we suggest you read more about the applications of computer vision. Articles we recommend include:
- Learn about Computer Vision, the technology behind video analytics
- Video Understanding With Deep Learning – PyTorchVideo
- Everything you need to know about Image Recognition (Guide)
- MediaPipe: Open Source Framework for ML pipelines