Waste management is a growing problem that localities and organizations must hurry to tackle as the world’s population continues to expand. Increasing rates of consumption from our growing population means more waste production requiring efficient and effective management. As per the World Bank, 2.01 billion tons of municipal solid waste was generated globally in 2016 with experts predicting a steep rise to 3.40 billion tons in 2050.
However, truly effective waste management is no simple task. Typically, different types of waste are often mixed and disposed of together. Separating these waste types is a hazardous process, as each requires specific disposal methods. For example, residential waste collected could contain several different types of plastics, requiring segregation before any can be properly recycled.
The segregation process quickly becomes inefficient due to these labor-intensive tasks resulting in human error. This is where computer vision technology can help identify waste, separate it, and ensure its proper disposal.
In this article, we will propose computer vision as an effective tool for waste management. We will look at its applications across waste sorting, recycling, and improvement of the overall disposal processes for smart cities in an increasingly visual world.
Artificial Intelligence in Waste Management
The field of Artificial Intelligence (AI) and machine learning has evolved significantly in recent years. Many industries began adopting these technologies to improve projects’ cost-effectiveness and efficiency while maintaining identifying a tool to give them a leg up on the competition. One such industry is the waste management industry – companies mainly focus on segregating the waste, disposing it safely, and appropriately recycling the waste with the assistance of intelligent tools.
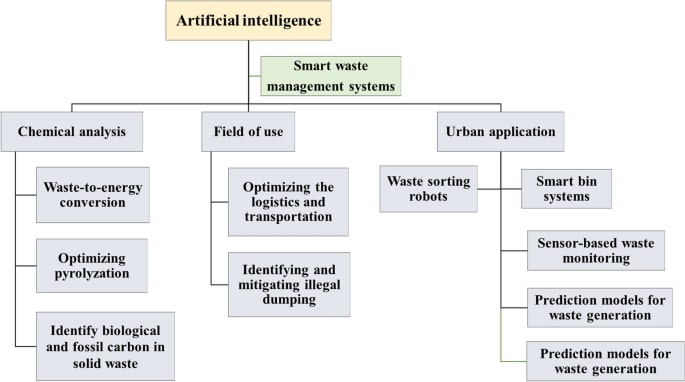
The illustration above depicts the various ways that AI can optimize waste touchpoints from from object identification to object sorting to predictive analysis. Each method involves the application of a specific domain in AI. For example, we can estimate the amount of waste production using historical data.
But, what if we want to identify what waste items are put in the garbage bin in real time? And, what can we do to prevent bin overfill before it occurs?
For truly solving real-world scenarios, organizations require more than just a computer vision tool or algorithm. Fully end-to-end solutions help us solve overarching challenges and implement production-ready applications. Before we delve into these applications, let’s revise the basis of computer vision and its various tasks.
What is Computer Vision?
Computer Vision is a field of Artificial Intelligence (AI) that mainly consists of processing and analyzing images and videos from the real world and helps make decisions based on it. This enables machines to have a human vision. Computer vision can be used to perform various tasks such as object detection, image classification, image recognition, and image segmentation, thereby solving a range of challenges.
Computer vision mainly uses neural networks under the hood. It powers autonomous drones, self-driving vehicles, face recognition in CCTV cameras, etc. Let us now see how computer vision can improve waste management.
To learn more about computer vision as a field of AI, we suggest checking out our Introduction to computer vision guide.
Computer Vision in Waste Management
Computer vision gives us the ability to analyze and process images and videos as mentioned above. These techniques can be applied to identify and analyse visual data. A few applications of computer vision in waste management are:
- Automated Waste Sorting robots
- Smart Waste Bins
- Detecting illegal waste dumping and disposal
- Detecting valuable resources in the waste
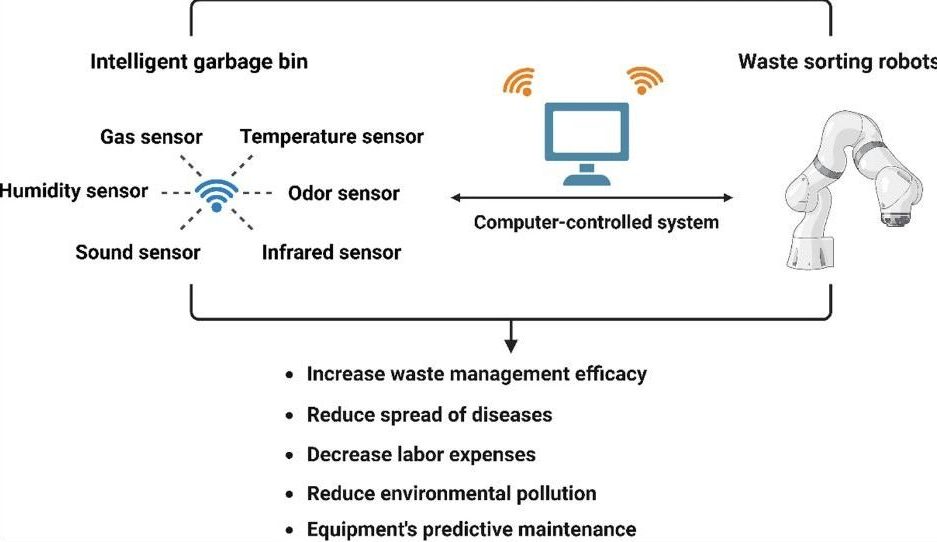
Application 1: Automated Waste-Sorting Robots
Every day, human workers collect various types of waste from different locations. However, human error often plays a role in unmet efficiency standards in recycling and disposal, namely when waste is not properly sorted. This is where we find opportunities for combining robotics with computer vision.
Implementing object detection, computer vision systems can identify and classify different types of waste. Waste-sorting robots equipped with cameras and sensors detect these materials in real time. Trained on extensive datasets, these robots accurately sort waste and direct it to the appropriate path for recycling or disposal.
Here is an example of what the full-scale intelligent waste disposal system could look like:
- Image Capture: Cameras capture images of waste items on a conveyor belt in real-time.
- Preprocessing: Images are cleaned and enhanced (e.g., noise reduction, normalization) to improve model accuracy.
- Object Detection: Computer vision algorithms, such as convolutional neural networks (CNNs), analyze the images to identify and classify waste types (i.e., plastic, metal, paper).
- Data Analysis: The system compares detected objects with a pre-trained model to accurately label each item.
- Waste Categorization: Based on the classification, the waste is sorted into predefined categories (e.g., recyclable, non-recyclable, hazardous).
- Robotic Arm Activation: The robotic system receives commands based on the waste type and uses grippers or suction to pick up the item.
- Sorting: The robotic arm places the sorted waste into the appropriate bin or compartment for further processing or recycling.
- Feedback Loop: The system continuously monitors sorting accuracy and updates the computer vision model with new data to improve future performance.
- Integration with IoT: Sensors and IoT devices track bin levels, report operational issues, and optimize the system’s efficiency in real-time.
Application 2: Smart Waste Bins
Sometimes, in regular garbage bins, we see the trash level more than what the bin could handle. Aside from being unsightly, overflowing bins can pose health risks and obstruction of paths. Manual inspection of trash levels in the garbage bins is also laborious and increases the cost of waste management. So, what if we could have a bin that could solve all these problems? And if such a bin needs to exist what technology should power it?
Smart bins extensively employ computer vision algorithms mainly utilizing object detection and object recognition. These systems coordinate sensors and visual algorithms to monitor garbage levels. Once the bin has enough level of garbage they send messages to sanitation workers and lock the bin.
This is important for several reasons focusing on environmental and public health:
- Stops the spread of the diseases.
- Increases waste management efficiency.
- Reduces the environmental pollution.
- Maintaining the hygiene of surroundings.
Application 3: Regulating Dumping and Disposal
Illegal waste dumping in unauthorized areas creates significant problems, such as health hazards, the spread of diseases, conflicts over dumping sites, and environmental damage. Computer vision is instrumental in detecting and addressing illegal waste disposal.
Here are some ways computer vision-equipped devices can help:
- Smart City Street-Level Surveillance: Security cameras using CNNs can monitor streets, identifying waste and pinpointing its location.
- Human Activity Detection: Cameras integrated with algorithms like OpenPose or YOLO can detect individuals dumping waste in public areas.
- Aerial Monitoring: High-resolution images from drones and satellites can identify illegal dumping sites from a broader perspective.
Application 4: Identifying and Removing Valuable Waste Items
Waste often contains reusable and recyclable materials, making recycling one of the most effective ways to reduce disposable waste and minimize environmental impact. However, recycling is not as simple as processing waste directly. First, different materials must be accurately classified for proper recycling.
While human workers can perform this task, it presents several challenges:
- Inefficient classification
- Rising labor costs
- Increased time required for segregation
These issues can be addressed by using computer vision-equipped devices in recycling facilities for the sorting process. Researchers have developed various models to automate these tasks and thus improve recycling rates.
For example, a team of researchers from the University of Manchester, East China University of Science and Technology, and Donghua University developed a two-stage Waste Recognition-Retrieval algorithm to improve waste classification accuracy.
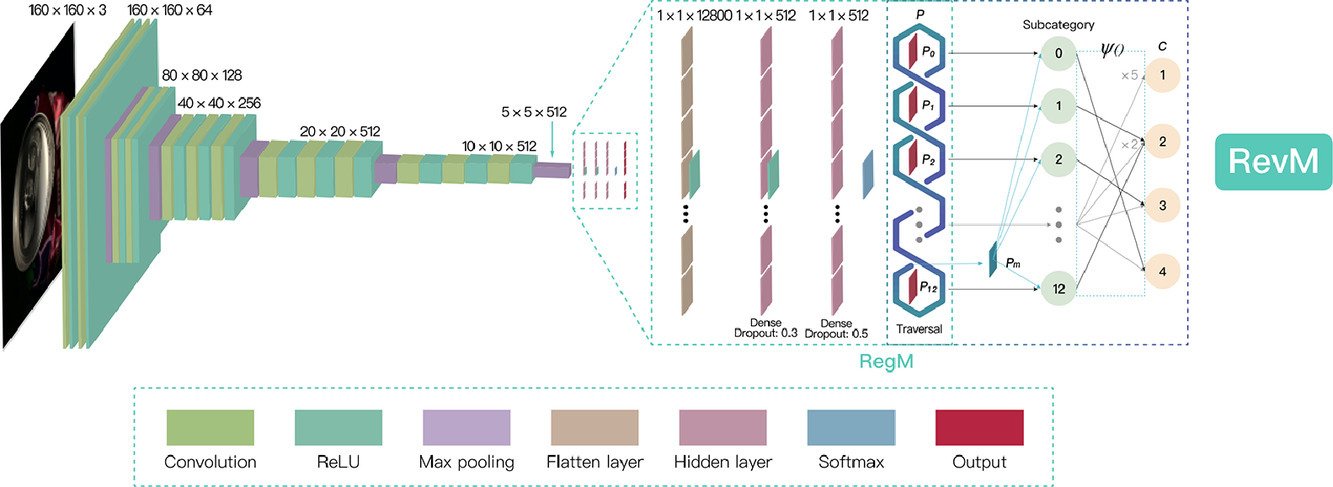
In this model, the first stage worst waste into thirteen categories, and the second classifies the waste into four categories. It has an accuracy of around 94.71%.
However, this algorithm has few limitations. It can identify only one type of waste in mixed waste. It also has low accuracy in classifying paper, tissue, and fabric.
Researchers at the University of Porto, Portugal developed a hierarchical deep learning method for sorting and identifying waste in food trays.
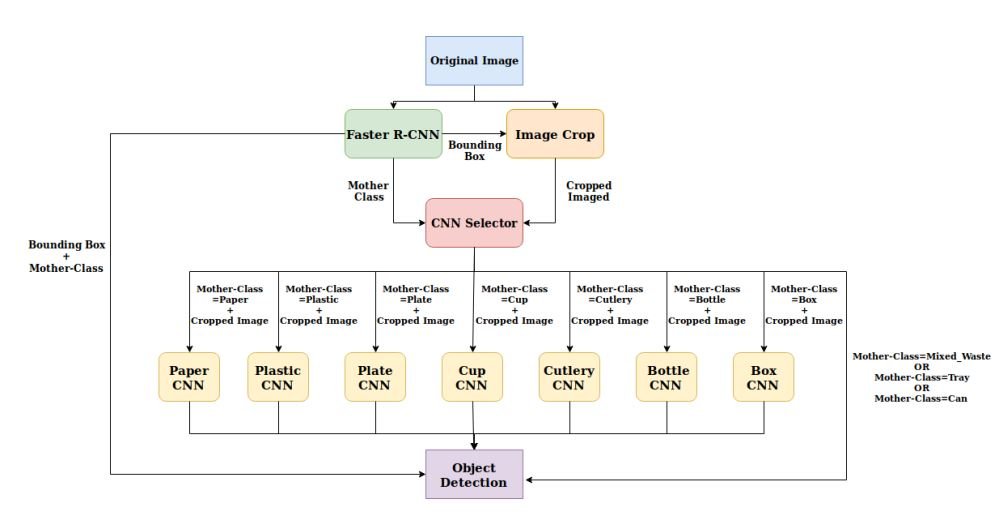
Multi-layer hybrid CNN, for waste classification, achieved an accuracy of 92.6% for classifying waste into six categories based on the material. This breakthrough is important, as it omits the need for human labor to perform these traditionally tedious tasks.
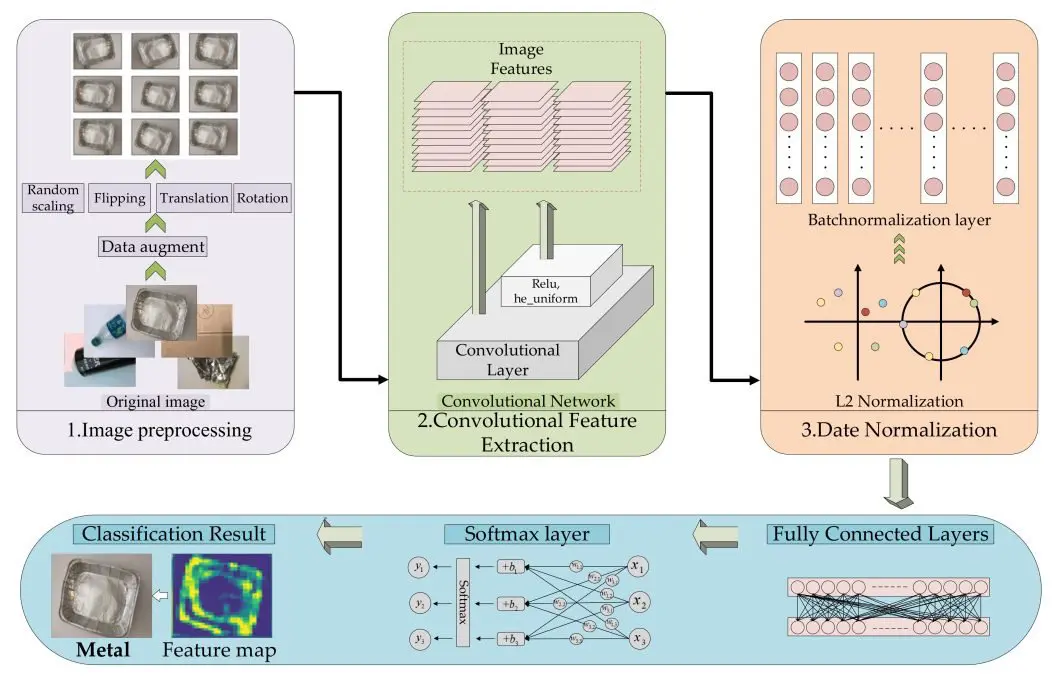
Another group of researchers from China proposed a method that uses online spectroscopy and CNNs to detect textiles in the waste. The model classifies textiles in the waste with an accuracy of 95.4%. It classifies textile waste into thirteen categories based on the material.
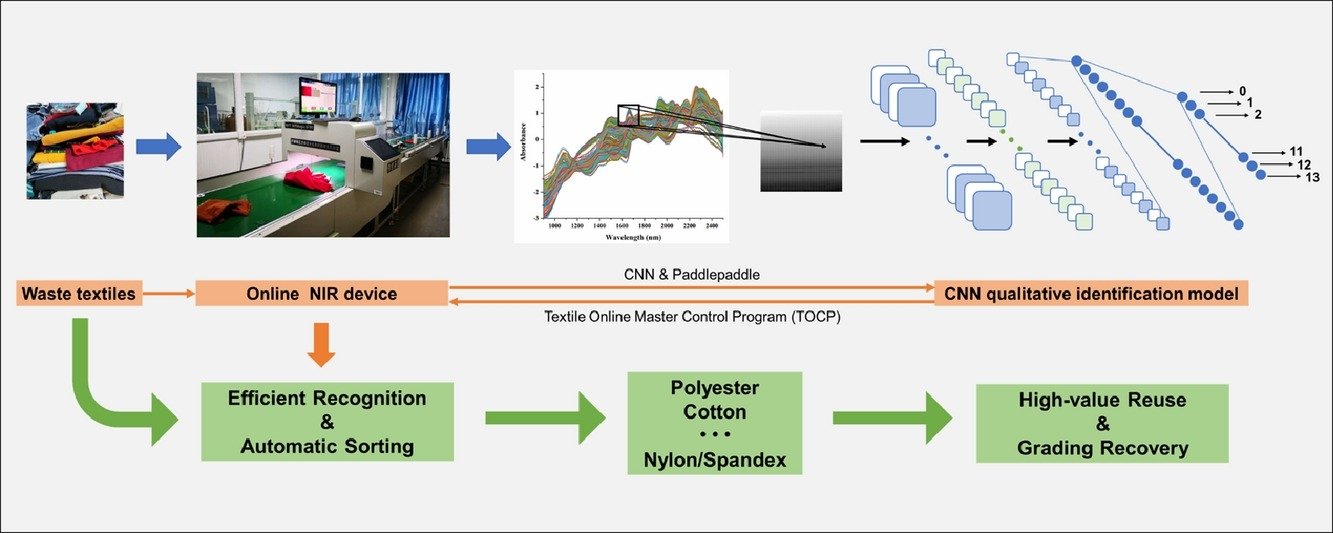
Limitations and Future Prospects
While computer vision algorithms can significantly improve the efficiency of waste management, they also come with certain limitations.
Convolutional neural network-based systems often require expensive hardware and consume high amounts of energy. Additionally, image processing systems may struggle during sunrise and sunset due to poor lighting conditions. Acquiring quality data for model training can also be challenging, as image quality varies at different times of the day, leading to less accurate models.
These limitations can be addressed by enhancing model performance and increasing efficiency through the integration of other technologies, such as the Internet of Things (IoT).
Waste Management With Computer Vision System Implementation
AI-powered waste management systems enhance the quality of life and promote the sustainability of communities. Computer vision plays a crucial role in modern waste management, significantly optimizing disposal methods.
Recent advances in computer vision are transforming how waste is managed. However, while innovations continue to improve these processes, we expect the quality and instances of these smart systems to increase in the coming years.
Viso Suite for Smart Cities and Intelligent Waste Management
Viso Suite is used by both private firms and governmental organizations around the world for waste management. IT leaders choose Viso Suite for both its ability to seamlessly integrate into existing tech stacks and grow with the evolving project needs. To learn more about how Viso Suite can solve waste management challenges, book a demo with our team of experts.
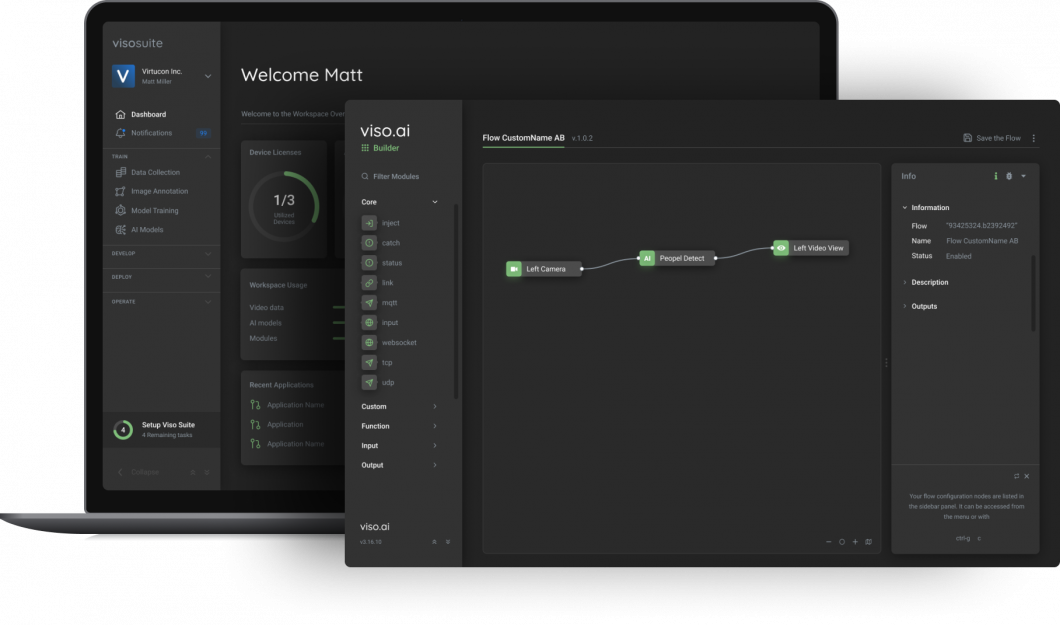