What is computer vision, and how does it work? This article provides a complete guide to Computer Vision (CV), one of the key fields of artificial intelligence (AI).
In the following, we will cover everything you need to know about visual AI technology and Computer Vision (CV):
- What is Computer Vision?
- How does Computer Vision work?
- The history of Computer Vision
- Current Trends
- Applications
- Start a Computer Vision Project
About us: Viso.ai provides the leading end-to-end Computer Vision Platform Viso Suite. Our technology automates how teams can build, deliver, and scale their computer vision applications. Get a demo for your company.

What is Computer Vision AI?
Computer Vision (CV) is a field of Artificial Intelligence (AI) that deals with computational methods to help computers understand and interpret the content of digital images and videos. Hence, CV aims to make computers see and understand visual data input from cameras or sensors.
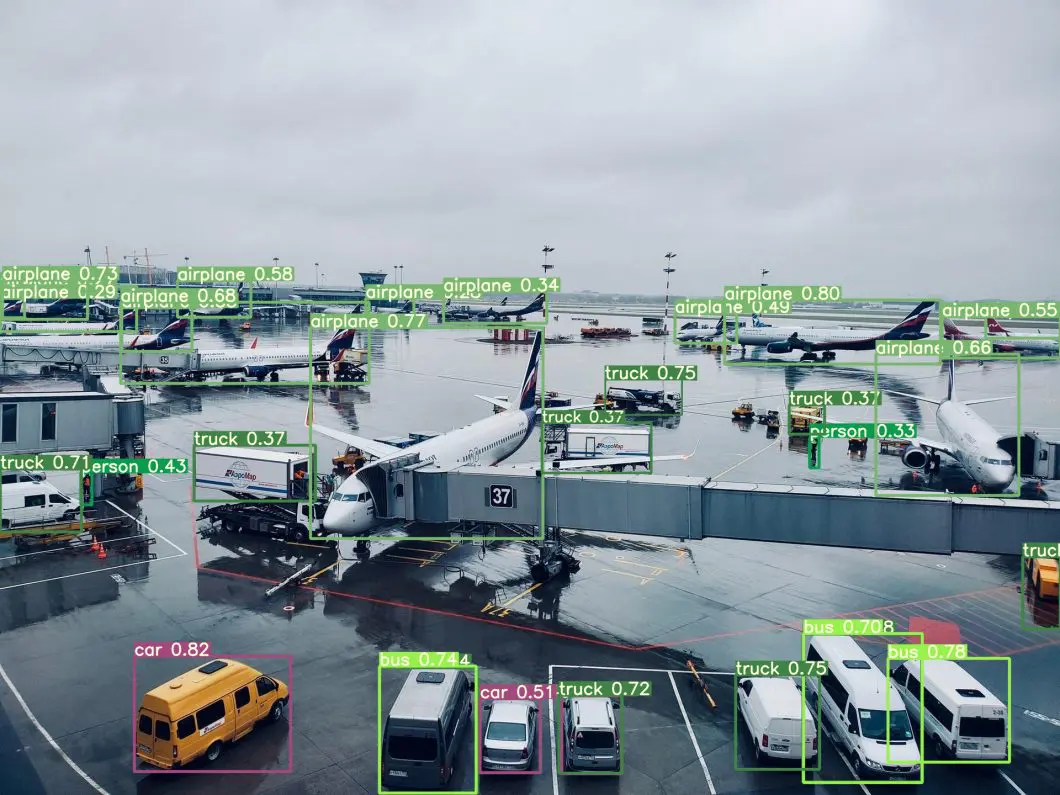
Definition of Computer Vision
Computer vision tasks seek to enable computer systems to automatically see, identify, and understand the visual world, simulating human vision using computational methods.
Human Vision vs. Computer Vision
Computer vision aims to artificially imitate human vision by enabling computers to perceive visual stimuli meaningfully. It is, therefore, also called machine perception, or machine vision.
While the problem of “vision” is trivially solved by humans (even by children), computational vision remains one of the most challenging fields in computer science, especially due to the enormous complexity of the varying physical world.
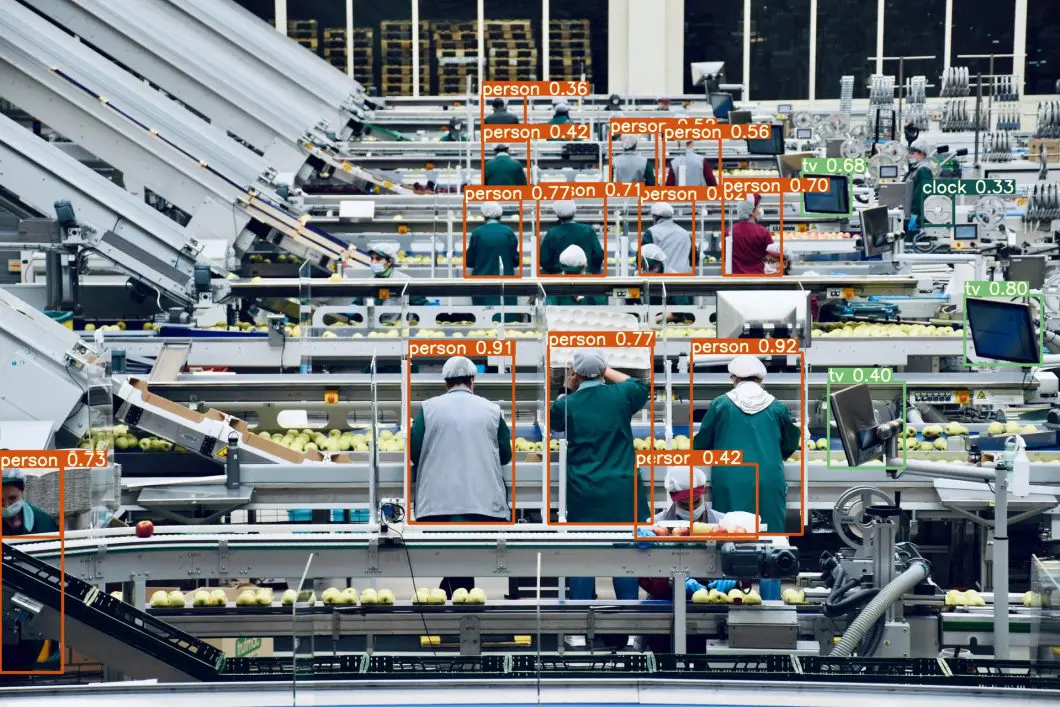
Human sight is based on a lifetime of learning with context to train how to identify specific objects or recognize human faces or individuals in visual scenes. Hence, modern artificial vision technology uses machine learning and deep learning methods to train machines how to recognize objects, faces, or people in visual scenes.
As a result, computer vision systems use image processing algorithms to allow computers to find, classify, and analyze objects and their surroundings from data provided by a camera.
What Is the Value of Computer Vision?
Computer vision systems can perform product inspection, infrastructure monitoring, or analyze thousands of products or processes in real time for defection detection. Due to their speed, objectivity, continuity, accuracy, and scalability, computer vision systems can quickly surpass human capabilities.
The latest deep learning models achieve above human-level accuracy and performance in real-world image recognition tasks such as facial recognition, object detection, and image classification.
Computer vision applications are used in various industries, ranging from security and medical imaging to manufacturing, automotive, agriculture, construction, smart city, transportation, and many more. As AI technology advances and becomes more flexible and scalable, more use cases become possible and economically viable.
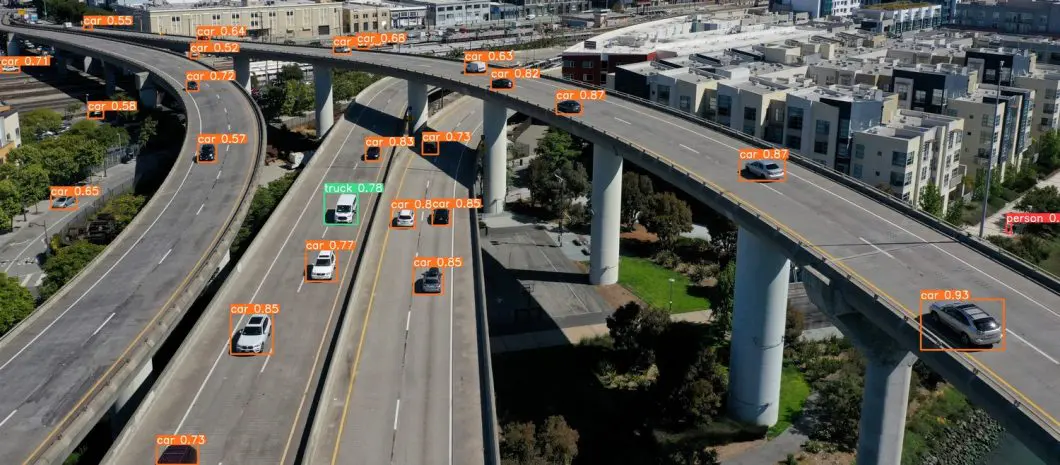
Check out our blog to learn more about the business value of computer vision.
Computer Vision Market Size
According to an analysis of the AI vision market by Verified Market Research (Nov 2022), the AI in Computer Vision Market was valued at USD 12 Billion in 2021 and is projected to reach USD 205 Billion by 2030. Accordingly, the computer vision market is rapidly growing at a CAGR of 37.05% from 2023 to 2030.
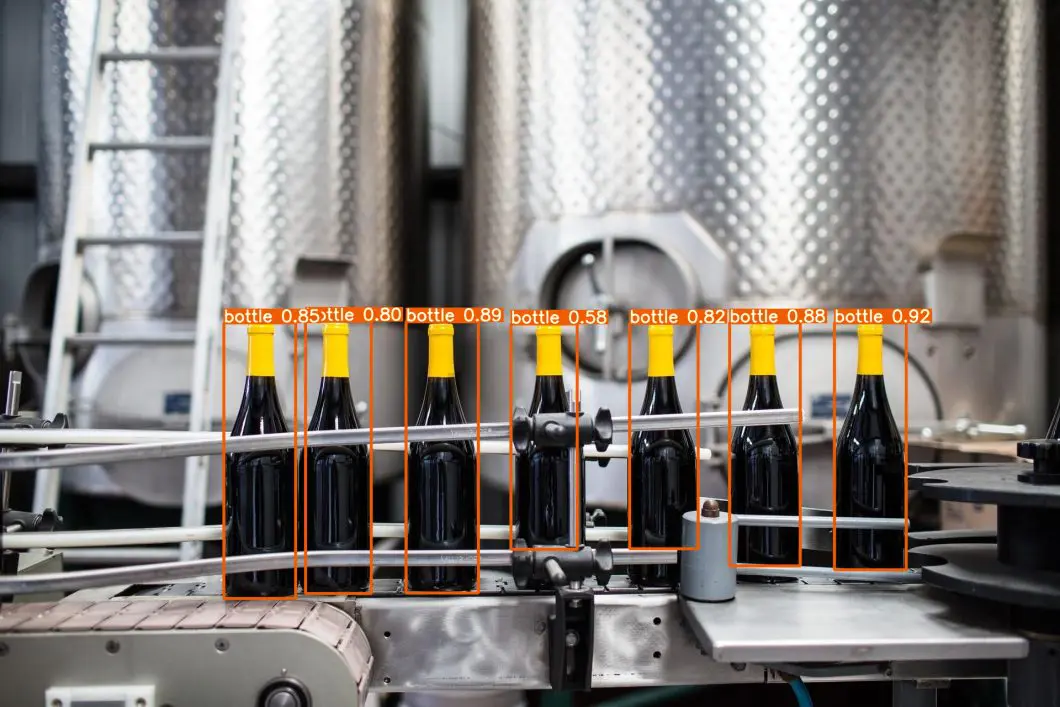
Computer Vision Platform to Build Applications
The computer vision platform Viso Suite enables leading organizations worldwide to develop, scale, and operate their AI vision applications. As the world’s only end-to-end AI vision platform, Viso Suite provides software infrastructure to dramatically accelerate the development and maintenance of computer vision applications across industries (Get the Economic Impact Study).
Viso Suite covers the entire lifecycle of computer vision, from image annotation and model training to visual development, one-click deployment, and scaling to hundreds of cameras. The platform provides critical capabilities such as real-time performance, distributed Edge AI, Zero-Trust Security, and Privacy-preserving AI out-of-the-box.
The extensible architecture of Viso Suite helps companies to re-use and integrate existing infrastructure (cameras, AI models, etc.) and connect computer vision with BI tools (PowerBI, Tableau) and external databases (Google Cloud, AWS, Azure, Oracle, etc.).
How Does Computer Vision AI Work?
Generally, computer vision works in three basic steps:
- Step #1: Acquiring the image/video from a camera,
- Step #2: Processing the image, and
- Step #3: Understanding the image.
A Practical Example of Computer Vision
Computer vision machine learning requires a massive amount of data to train a deep learning algorithm that can accurately recognize images. For example, to train a computer to recognize a helmet, it needs to be fed large quantities of helmet images with people wearing helmets in different scenes to learn the characteristics of a helmet.
Next, the trained algorithm can be applied to newly generated images, for example, videos of surveillance cameras, to recognize a helmet. This is, for example, used in computer vision applications for equipment inspection to reduce accidents in construction or manufacturing.
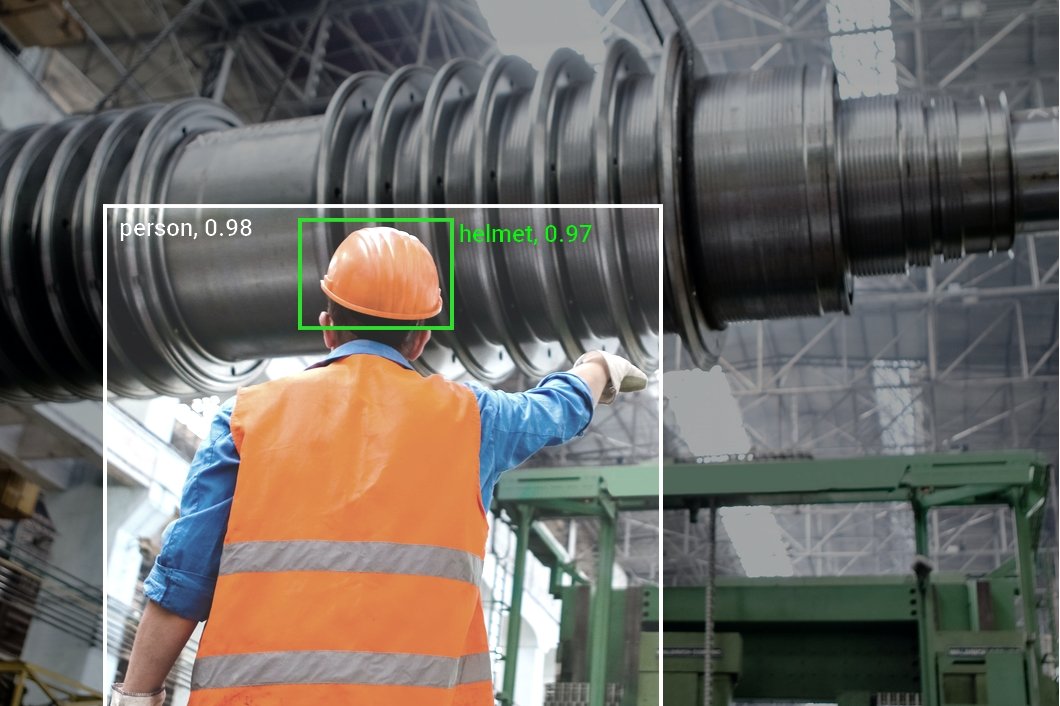
How Computer Vision Technology Works
To train an algorithm for computer vision, state-of-the-art technologies leverage deep learning, a subset of machine learning. Many high-performing methods in modern computer vision software are based on a convolutional neural network (CNN).
Such layered neural networks enable a computer to learn about the context of visual data from images. If enough data is available, the computer learns how to tell one image from another. As image data is fed through the model, the computer applies a CNN to “look” at the data.
The CNN helps a machine learning/deep learning model to understand images by breaking them down into pixels that were given labels to train specific features, so-called image annotation. The AI model uses the labels to perform convolutions and make predictions about what it is “seeing” and checks the accuracy of the predictions iteratively until the predictions meet the expectation (start to come true).
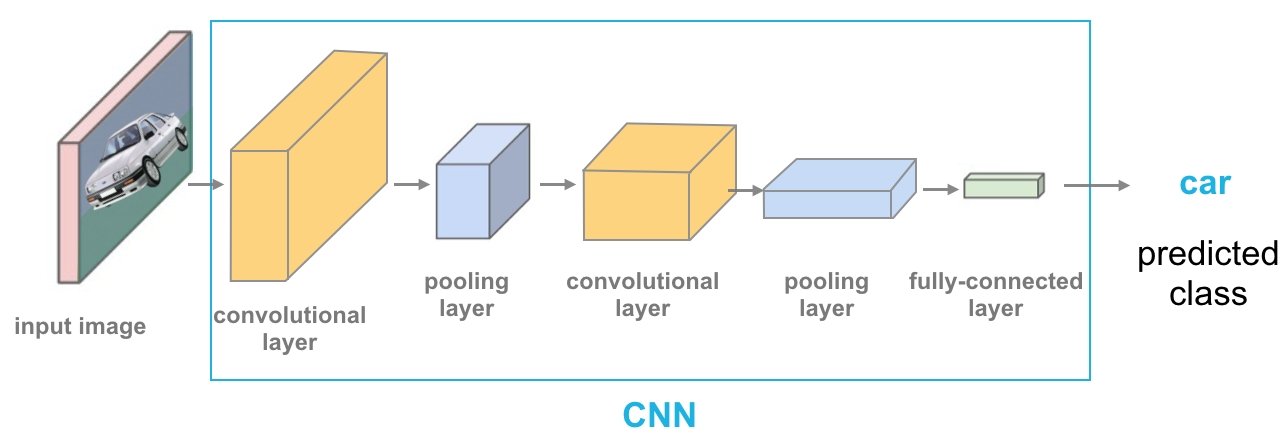
Computational Vision Inspired by the Human Brain
Hence, computer vision works by recognizing images or “seeing” images similar to humans, using learned features with a confidence score. Therefore, neural networks essentially simulate human decision-making, or neuron activation mechanisms, and deep learning trains the machine to do what the human brain does naturally.
The characteristic layered structure of deep neural networks is the foundation of Artificial Neural Networks (ANN). Each layer adds to the knowledge of the previous layer.
Human-level Performance of Computer Vision AI
Deep learning tasks are computationally heavy and expensive, depending on significant computing resources, and require massive datasets to train models on. Compared to traditional image processing, deep learning algorithms enable machines to learn by themselves, without a developer programming it to recognize an image based on pre-determined features. As a result, deep learning methods achieve very high accuracy.
Today, deep learning enables machines to achieve human-level performance in image recognition tasks. For example, in deep face recognition, AI models achieve a detection accuracy (e.g., Google FaceNet achieved 99.63%) that is higher than the accuracy humans can achieve (97.53%).
Computational vision with deep learning has also achieved human performance in classifying skin cancer with a level of competence comparable to dermatologist experts.
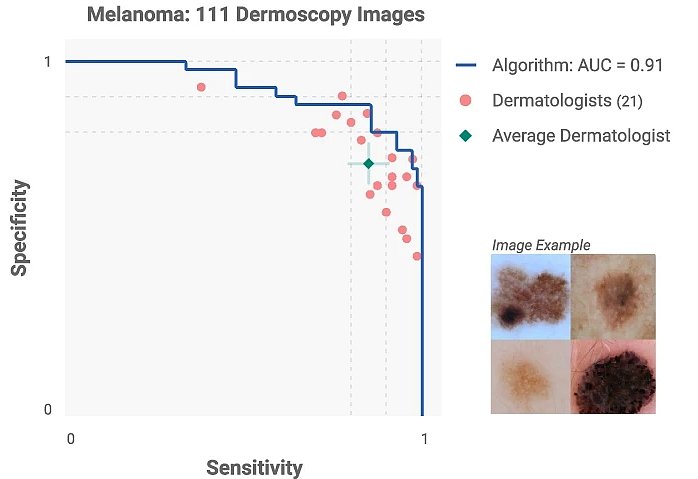
What Is a Computer Vision System?
Modern computer vision systems combine image processing with machine learning and deep learning techniques. Hence, developers combine different software (etc., OpenCV or OpenVINO) and AI algorithms to create a multi-step process, a computer vision pipeline.
The organization and setup of a computer vision system vary based on the application and use case. However, all computer vision systems contain the same typical functions:
- Step #1: Image acquisition. The digital image of a camera or image sensor provides the image data or video. Technically, any 2D or 3D camera or sensor can be used to provide image frames.
- Step #2: Pre-processing. The raw image input of cameras needs to be preprocessed to optimize the performance of the subsequent computer vision tasks. Pre-processing includes noise reduction, contrast enhancement, re-scaling, or image cropping.
- Step #3: Computer vision algorithm. The image processing algorithm, most popularly a deep learning model (DL model), performs image recognition, object detection, image segmentation, and image classification on every image or video frame.
- Step #4: Automation logic. The AI algorithm output information needs to be processed with conditional rules based on the use case. This part performs automation based on information gained from the computer vision task. For example, pass or fail for automatic inspection applications, match or no-match in recognition systems, and flag for human review in insurance, surveillance and security, military, or medical recognition applications.
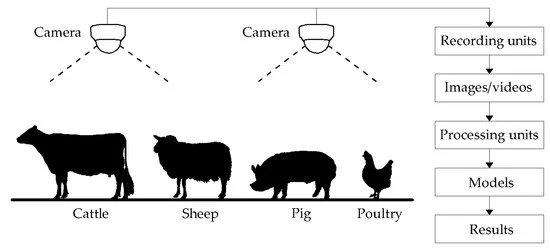
The Best Computer Vision AI Deep Learning Models Today
In computer vision, specifically, real-time object detection, there are single-stage and multi-stage algorithm families.
- Single-stage algorithms aim for real-time processing and the highest computational efficiency. The most popular algorithms include SSD, RetinaNet, YOLOv3, YOLOR, YOLOv5, YOLOv7, and YOLOv8.
- Multi-stage algorithms perform multiple steps and achieve the highest accuracy but are rather heavy and resource-intensive. The widely used multi-stage algorithms include Recurrent Convolutional Neural Networks (R-CNN) such as Mask-RCNN, Fast RCNN, and Faster RCNN.
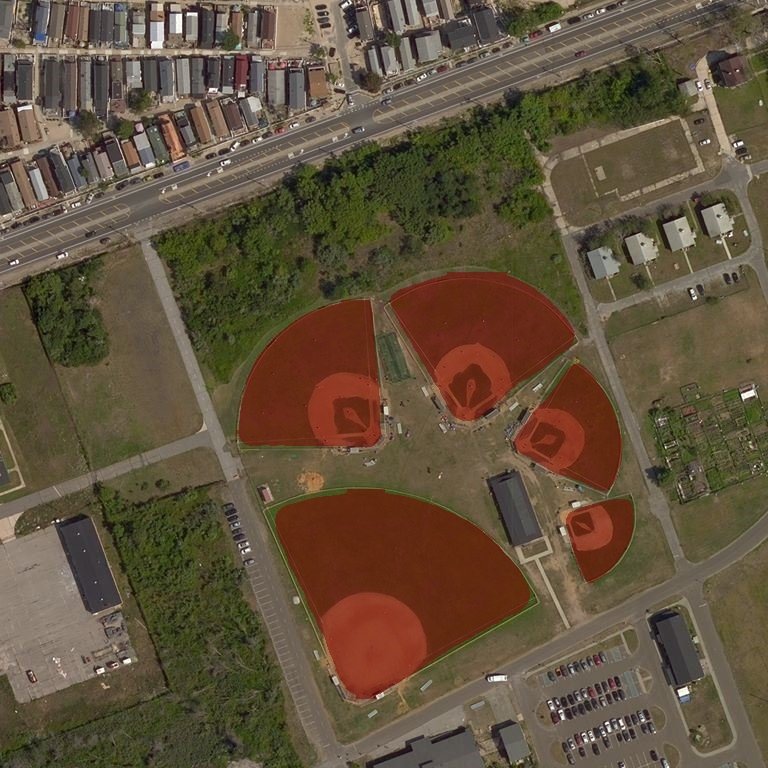
History of Computer Vision AI Technology
In recent years, new deep learning technologies achieved great breakthroughs, especially in image recognition and object detection.
- 1960 – The beginnings. Computer vision came to light in the 1960s when computer scientists tried to mimic human eyesight using computing mechanics. Although computer vision research has spent several decades teaching machines how to see, the most advanced machine at that time could only perceive common objects and struggled to recognize multiple natural objects with infinite shape variations.
- 2014 – The era of Deep Learning. Researchers achieved great breakthroughs by training computers with the 15 million images of the largest image classification dataset, ImageNet using deep learning technology. In computer vision challenges and benchmarks, deep learning demonstrated overwhelming superiority over traditional computer vision algorithms that treat objects as a collection of shape and color features.
- 2016 – Near real-time Deep Learning. Deep learning, a particular class of machine learning algorithms, simplifies the process of feature extraction and description through a multi-layer convolutional neural network (CNN). Powered by massive data from ImageNet, modern central processing units (CPU), and graphics processing units (GPU), deep neural networks bring an unprecedented development of computer vision and achieve state-of-the-art performance. Especially, the development of single-stage object detectors made deep learning AI vision much faster and more efficient.
- 2020s – Deep Learning deployment and Edge AI. Today, CNN has become the de-facto standard computation framework in computer vision. Numbers of deeper and more complex networks were developed to make CNNs deliver near-human accuracy in many computer vision applications.
Optimized, lightweight AI models make it possible to perform computer vision on inexpensive hardware and mobile devices. Edge AI hardware, such as deep learning hardware accelerators, enables highly efficient Edge Inference.
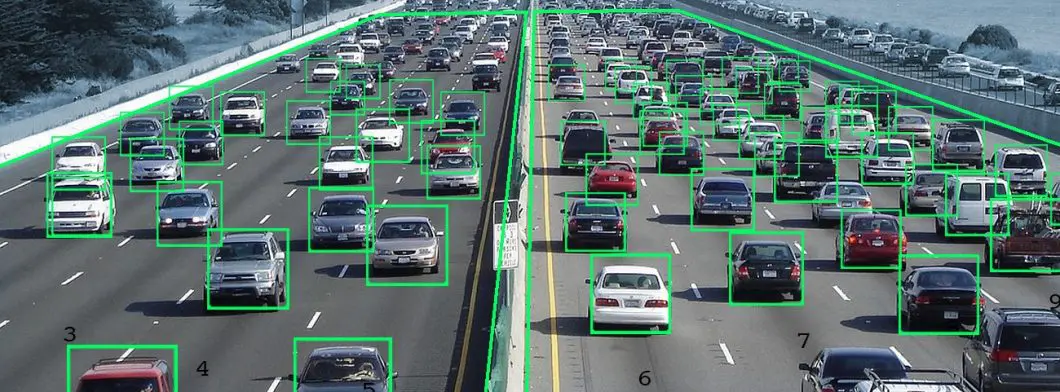
Current Trends and State-of-the-Art Technology in Computer Vision AI
The latest trends combine Edge Computing with on-device Machine Learning, a method also called Edge AI. Moving AI processing from the cloud to edge devices makes it possible to run computer vision machine learning everywhere and build scalable applications.
We see a trend in falling computer vision costs, driven by higher computational efficiency, decreasing hardware costs, and new technologies. As a result, more and more CV applications have become possible and economically feasible – further accelerating adoption.
The most important Computer Vision trends right now are:
- Trend #1: Real-Time Video Analytics
- Trend #2: AI Model Optimization and Deployment
- Trend #3: Hardware AI Accelerators
- Trend #4: Edge Computer Vision
- Trend #5: Real-world computer vision applications
Real-Time Video Analytics
Traditional machine vision systems commonly depend on special cameras and highly standardized settings. In contrast, modern deep learning algorithms are much more robust, easy to re-use and re-train, and allow the development of applications across industries.
Modern deep-learning computer vision methods can analyze video streams of common, inexpensive surveillance cameras or webcams to perform state-of-the-art AI video analytics.
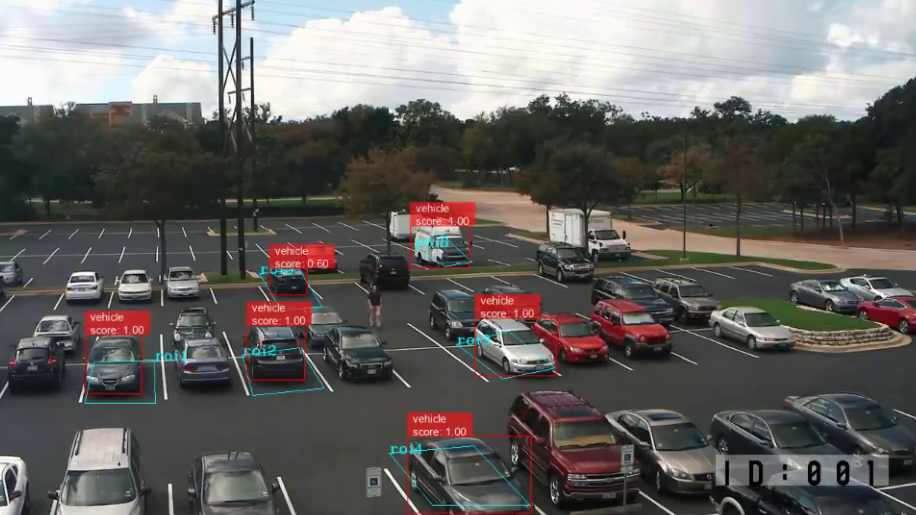
AI Model Optimization and Deployment
After a decade of deep learning training, aiming to improve the accuracy and performance of algorithms, we now enter the era of deep learning deployment. AI model optimization and new architectures made it possible to drastically reduce the size of machine learning models while increasing computational efficiency. This makes it possible to run deep learning computer vision without depending on expensive and energy-consuming AI hardware and GPUs in data centers.
Hardware AI Accelerators
Meanwhile, we face a boom in high-performance deep learning chips that are increasingly energy-efficient and run on small form-factor devices and edge computers. Current popular deep learning AI hardware includes edge computing devices such as embedded computers and SoC devices, including the Nvidia Jetson Tx2, Intel NUC, or Google Coral.
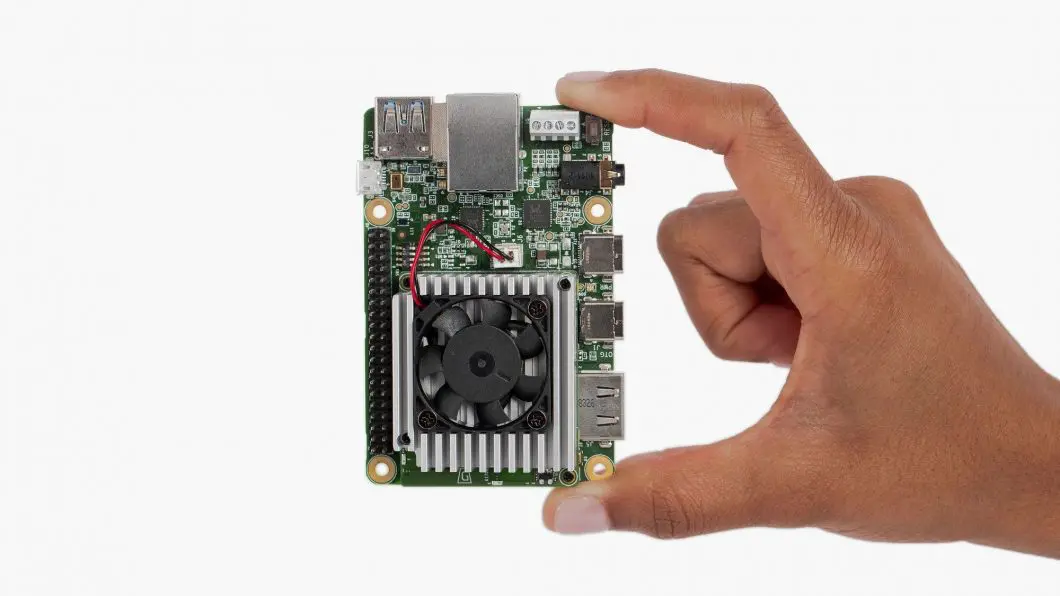
AI accelerators for neural networks can be attached to embedded computing systems. The most popular hardware neural network AI accelerators include the Intel Myriad X VPU, Google Coral, or Nvidia NVDLA.
Edge Computer Vision
Traditionally, CV and AI, in general, were pure cloud solutions due to the unlimited availability of computing resources and easy scalability to increase resources. Web or cloud CV solutions require uploading all images or photos to the cloud, either directly or using a computer vision API such as AWS Rekognition, Google Vision API, Microsoft image recognition API (Azure Cognitive Services), or Clarifai API.
In mission-critical use cases, data offloading with a centralized cloud design is usually not possible because of technical (latency, bandwidth, connectivity, redundancy) or privacy reasons (sensitive data, legality, security), or because it is too expensive (real-time, large-scale, high-resolution, bottlenecks cause cost spikes). Hence, edge computing concepts are used to overcome the limits of the cloud; the cloud is extended to multiple connected edge devices.
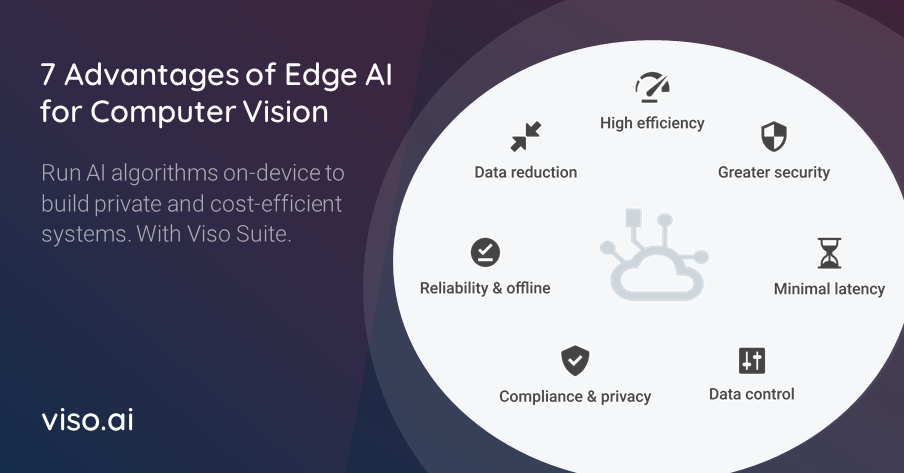
Edge AI, also called Edge Intelligence or on-device ML, uses edge computing and the Internet of Things (IoT) to move machine learning from the cloud to edge devices near the data source such as cameras. With the massive, still exponentially growing amount of data generated at the edge, AI is required to analyze and understand data in real-time without compromising the privacy and security of visual data.
Real-world Computer Vision Applications
Hence, CV at the edge leverages the advantages of the cloud and the edge to make AI vision technology scalable, and flexible. This supports the implementation of real-world applications. On-device CV does not depend on data offloading and inefficient centralized image processing in the cloud.
Also, Edge CV does not fully depend on connectivity and requires much lower bandwidth and reduced latency, especially important in video analytics. Therefore, Edge CV allows the development of private, robust, secure, and mission-critical real-world applications.
Since Edge AI involves the Internet of Things (AIoT) to manage distributed devices, the superior performance of Edge CV comes at the cost of increased technical complexity.
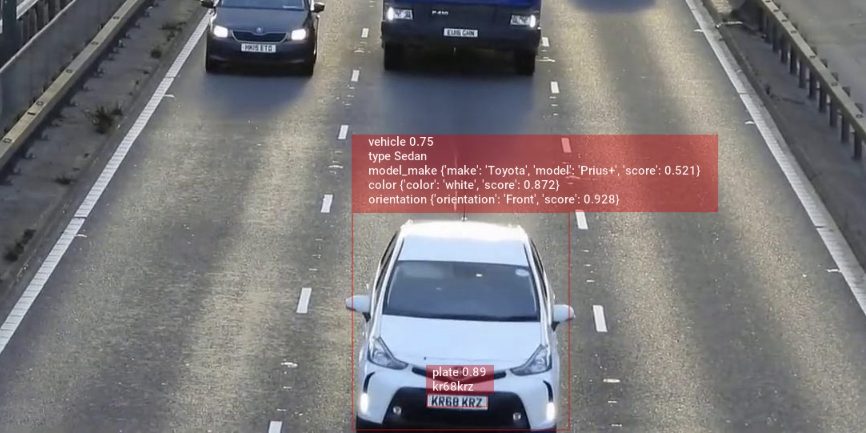
Computer Vision AI Applications and Use Cases
Companies are rapidly introducing CV technology across industries to solve automation problems with computers that can see. Visual AI technology is quickly advancing, making it possible to innovate and implement new ideas, projects, and applications including:
Manufacturing
Industrial computer vision is used in manufacturing industries on the production line for automated product inspection, object counting, process automation, and to increase workforce safety with PPE detection and mask detection.
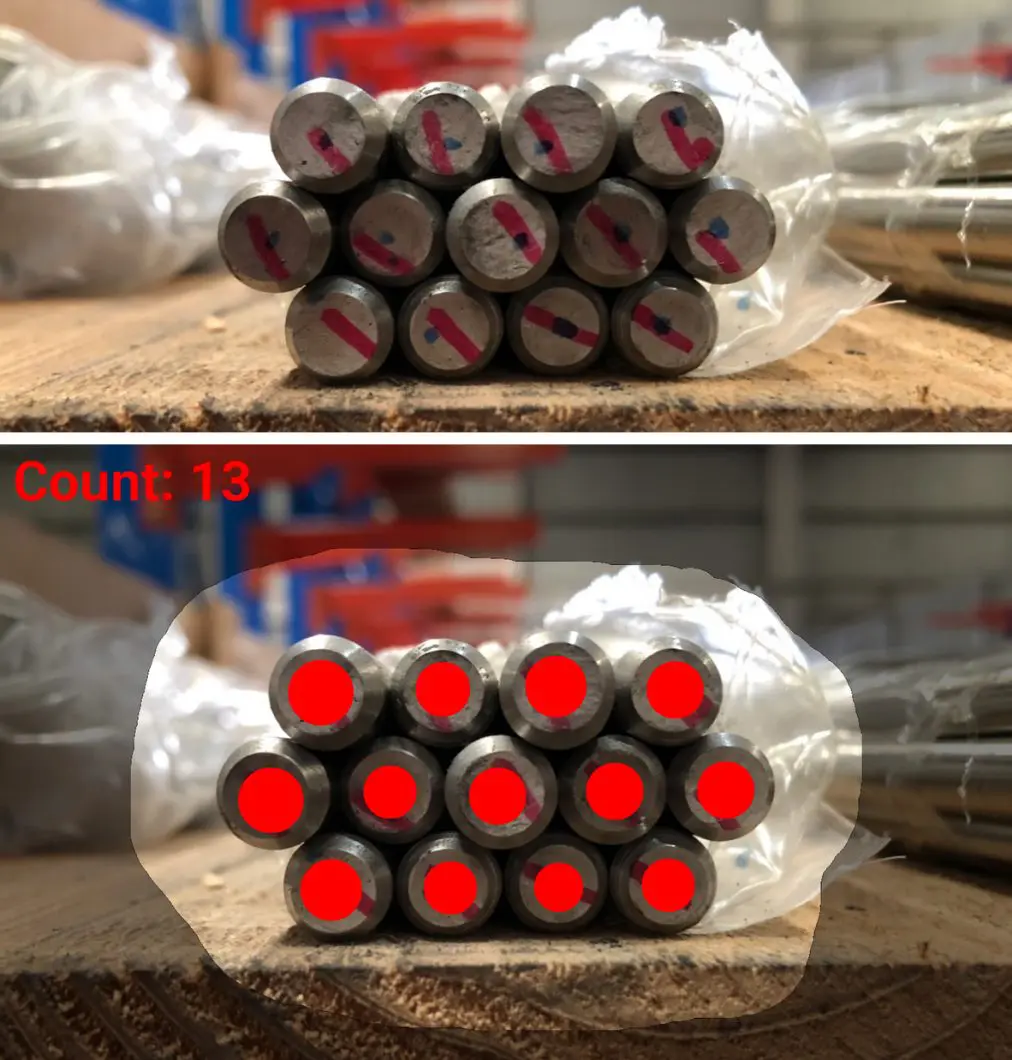
Healthcare
Among applications of computer vision in healthcare, a prominent example is automated human fall detection to create a fall risk score and trigger alerts.
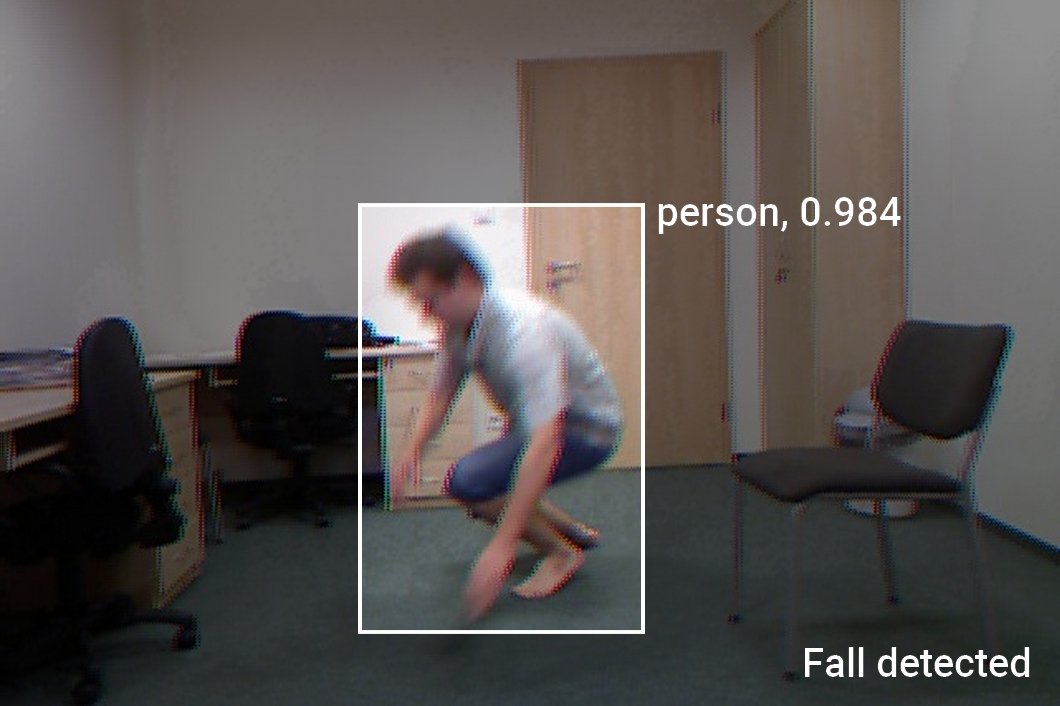
Security
In video surveillance and security, person detection is performed for intelligent perimeter monitoring. Another popular use case is deep face detection and facial recognition with above-human-level accuracy.
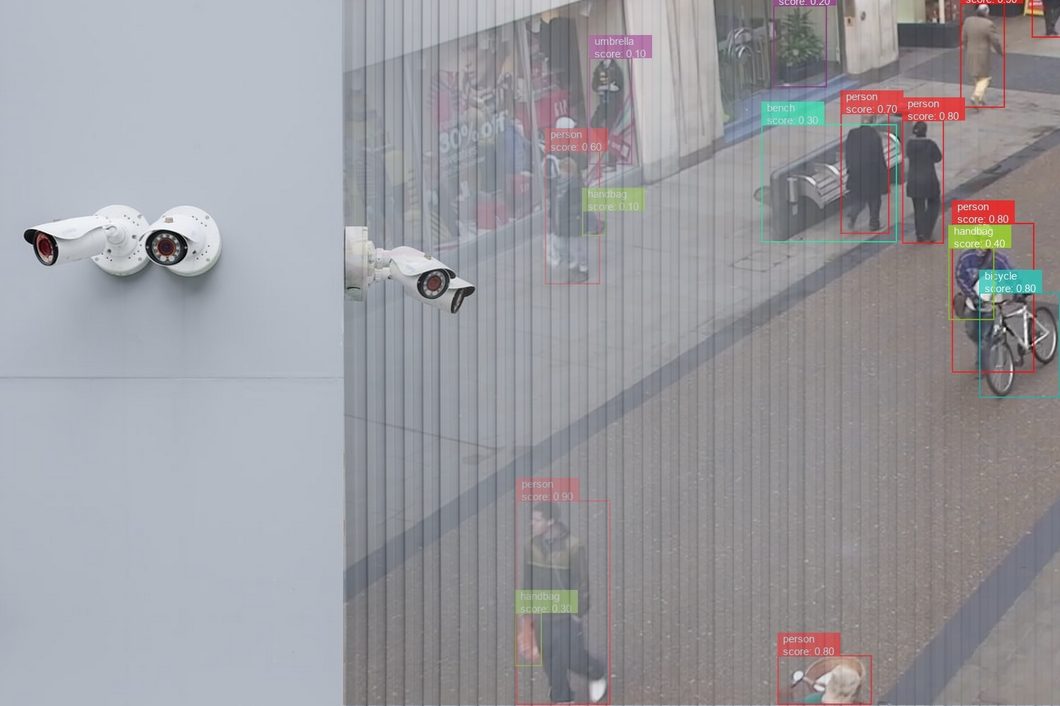
Agriculture
Use cases of computational vision in agriculture and farming include automated animal monitoring to detect animal welfare, and early detect diseases and anomalies.
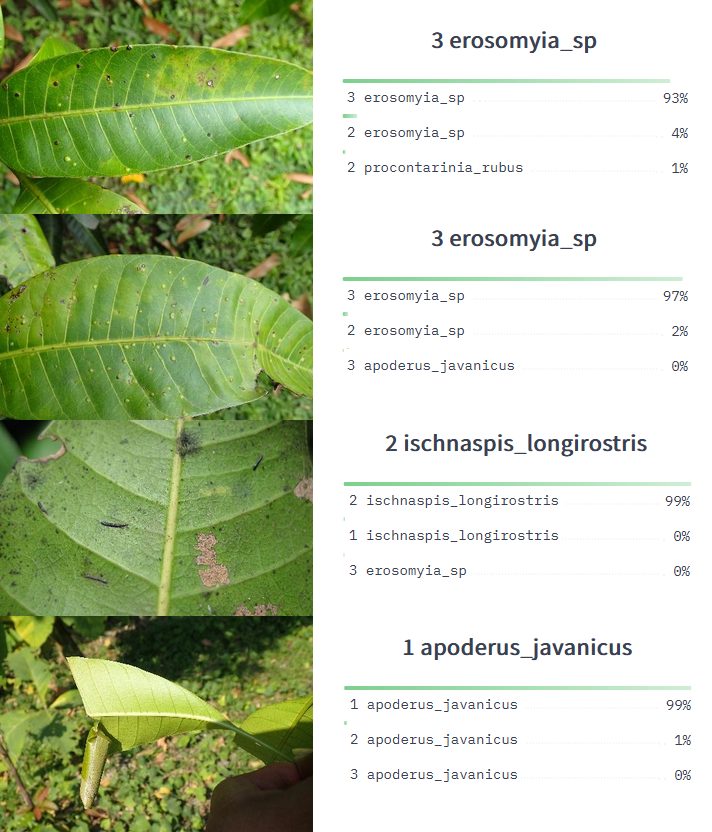
Smart Cities
Used as a key strategy in smart cities for crowd analysis, weapon detection, traffic analysis, vehicle counting, self-driving cars/autonomous vehicles, and infrastructure inspection.
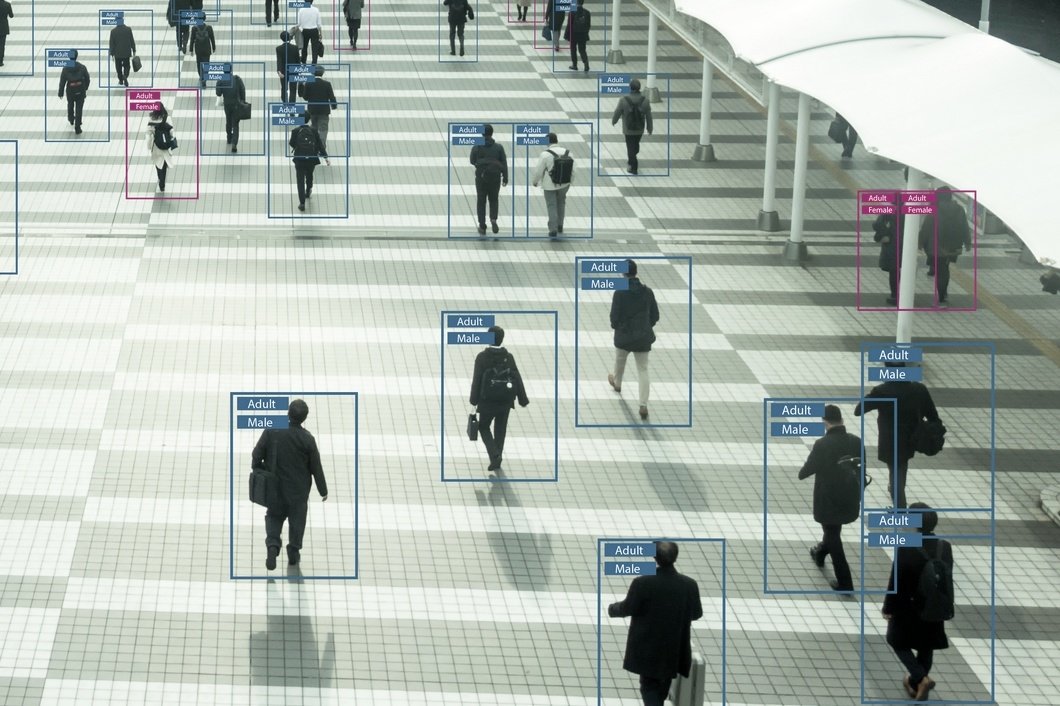
Retail
For example, video surveillance cameras in retail stores track movement patterns of customers and perform people counting or footfall analysis to identify bottlenecks, customer attention, and waiting times.
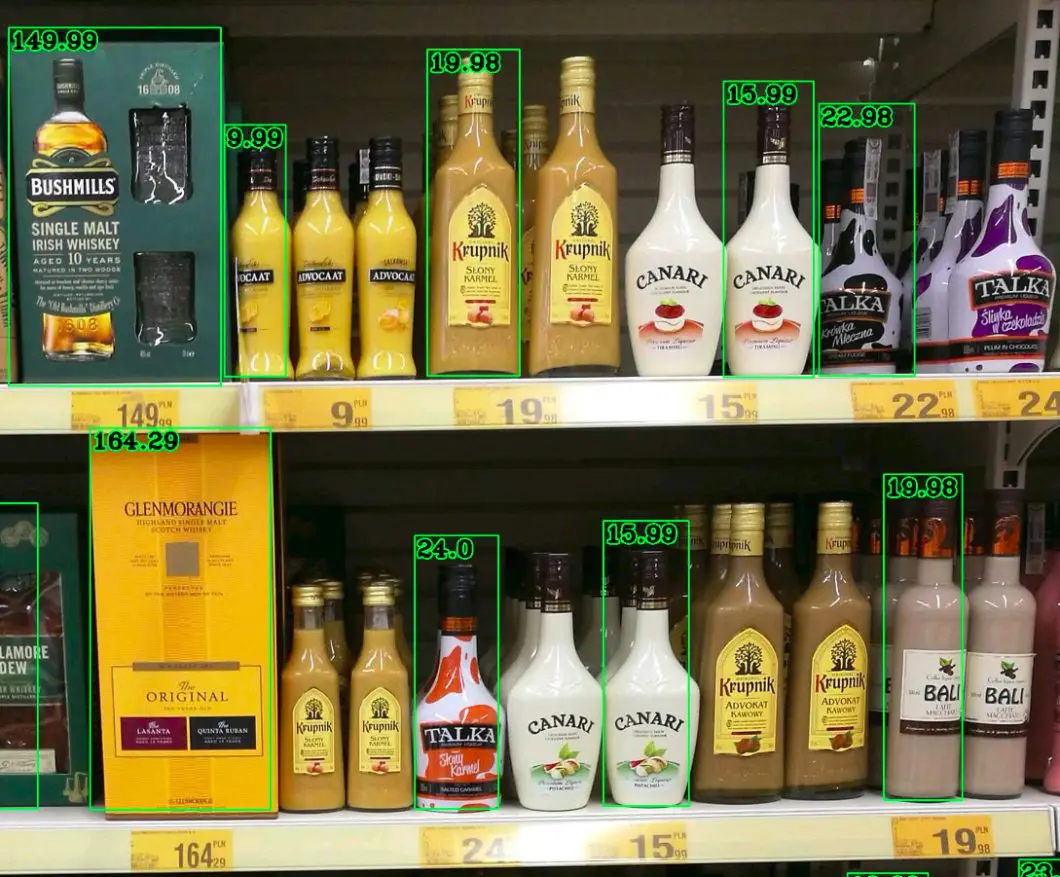
Insurance
Computer vision in Insurance leverages AI vision for automated risk management and assessment, claims management, visual inspection, and forward-looking analytics.
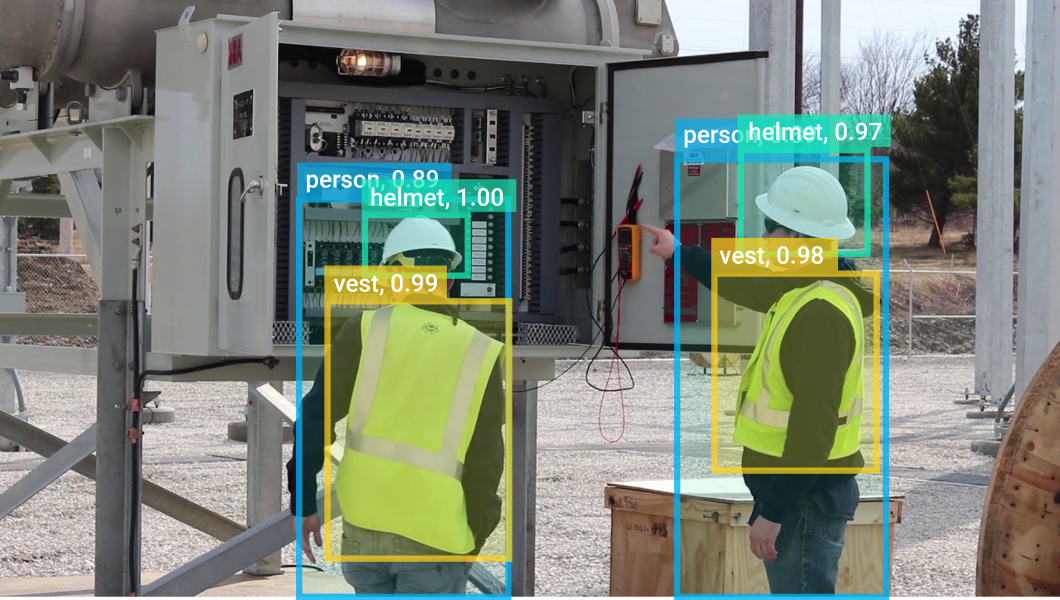
Logistics
AI vision in Logistics applies deep learning to implement AI-triggered automation and save costs by reducing human errors, predictive maintenance, and accelerating operations throughout the supply chain.
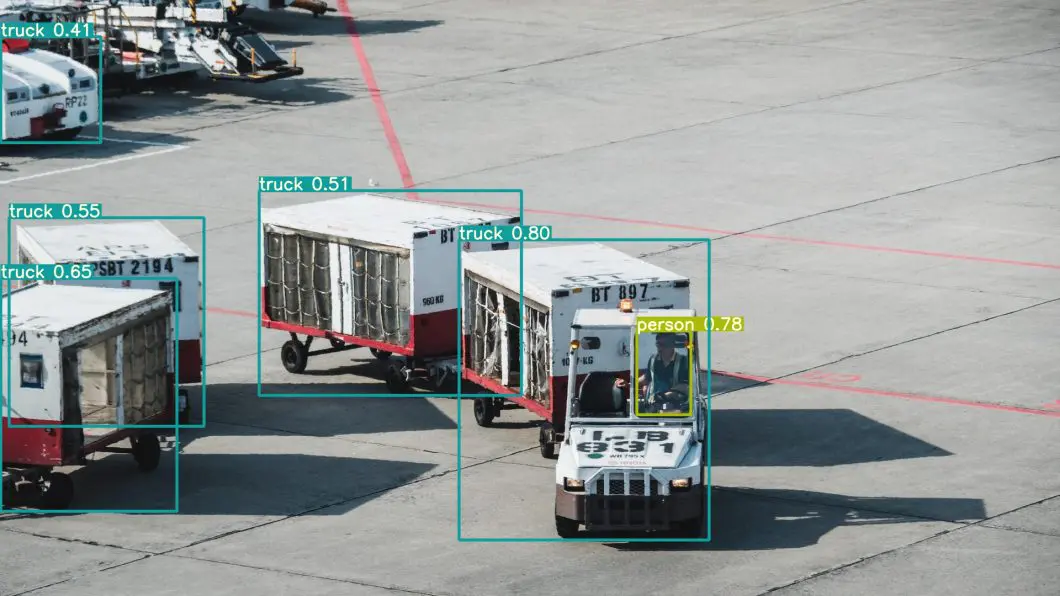
Pharmaceutical
Computer vision in pharmaceutical industries is used for packaging and blister detection, capsule recognition, and visual inspection for equipment cleaning.
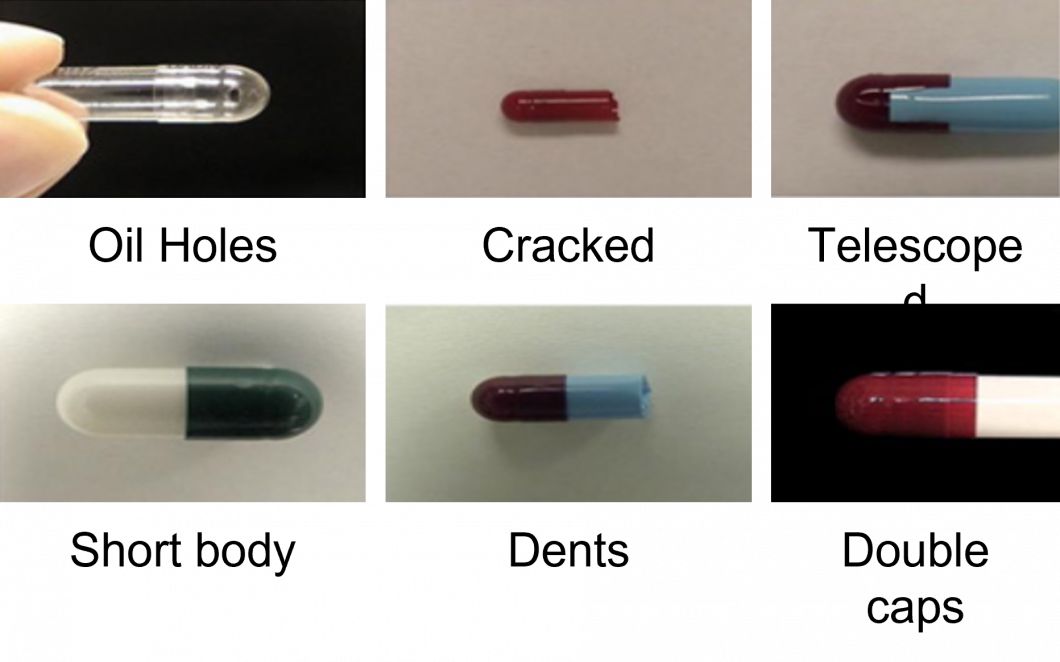
Augmented Reality and Virtual Reality
Computer vision for augmented and virtual reality creates immersive experiences by integrating real-world or virtual environment perception, thus allowing users to interact with virtual surroundings in real-time.
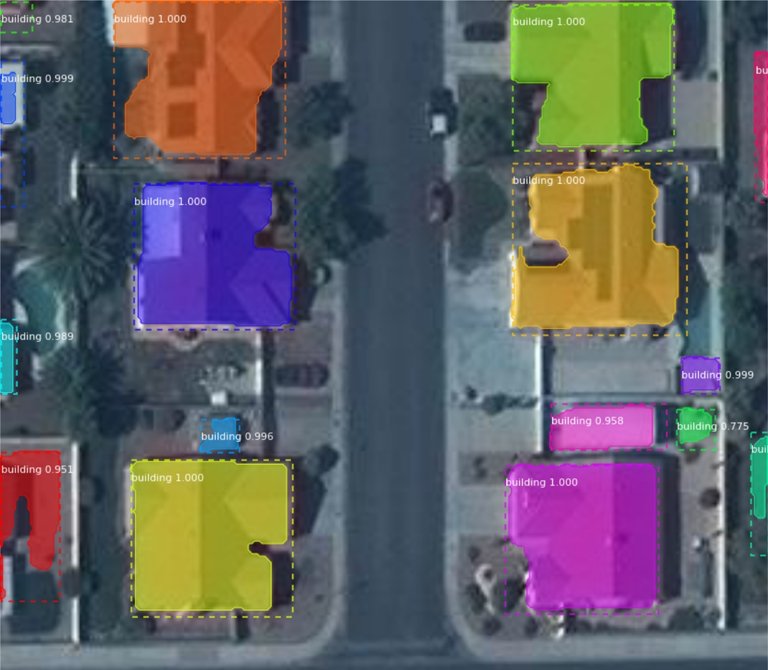
Computer Vision AI Research
Key fields of research involve the fundamental visual perception tasks:
- Object recognition: Determine whether image data contains one or multiple specified or learned objects or object classes.
- Facial recognition: Recognize an individual instance of a human face by matching it with database entries.
- Object detection: Analyze image data for a specific condition, and localize instances of semantic objects of given classes.
- Pose estimation: Estimate the orientation and position of a specific object relative to the camera.
- Optical character recognition (OCR): Identify characters in images (numberplates, handwriting, etc.), usually combined with encoding the text in a useful format.
- Scene understanding: Parse an image into meaningful segments for analysis.
- Motion analysis: Tracking the movement of interest points (key points) or objects (vehicles, objects, humans, etc.) in an image sequence or video.
- Pattern recognition: Identification of patterns and regularities within data.
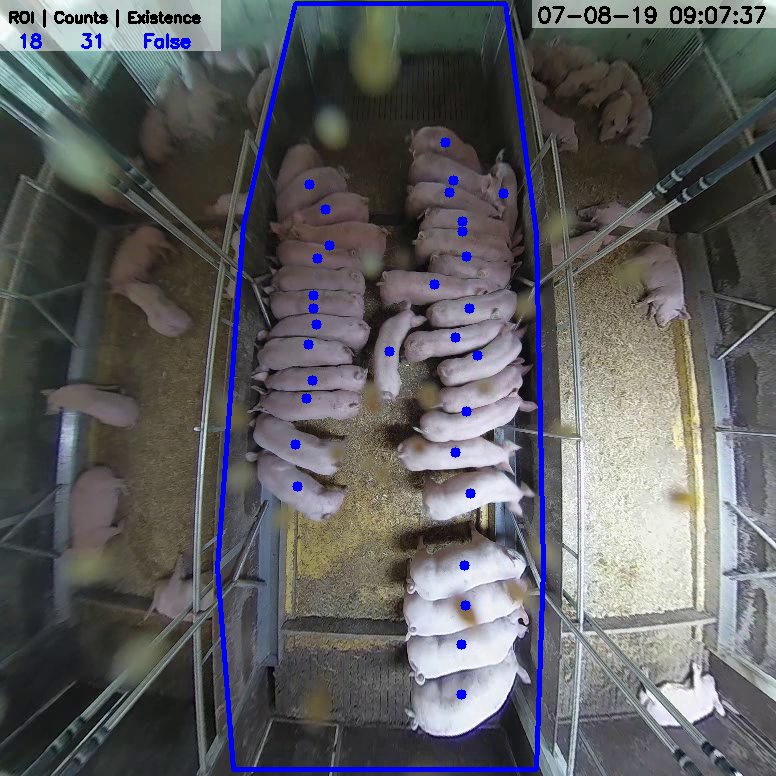
What is Image Classification?
Image classification forms the fundamental building block of Computer Vision. CV engineers often start with training a Neural Network to identify different objects in an image (Object Detection). Training a network to identify the difference between two objects in an image implies building a binary classification model. On the other hand, if it is more than two objects in an image, then it is a multi-classification problem.
It is important to note that to successfully build any image classification model that can scale or be used in production, the model has to learn from enough data. Transfer learning is an image classification technique leveraging existing architectures that have been trained to learn enough from huge data samples. The learned feature or task is then utilized to identify similar samples. Another term for this is knowledge transfer.
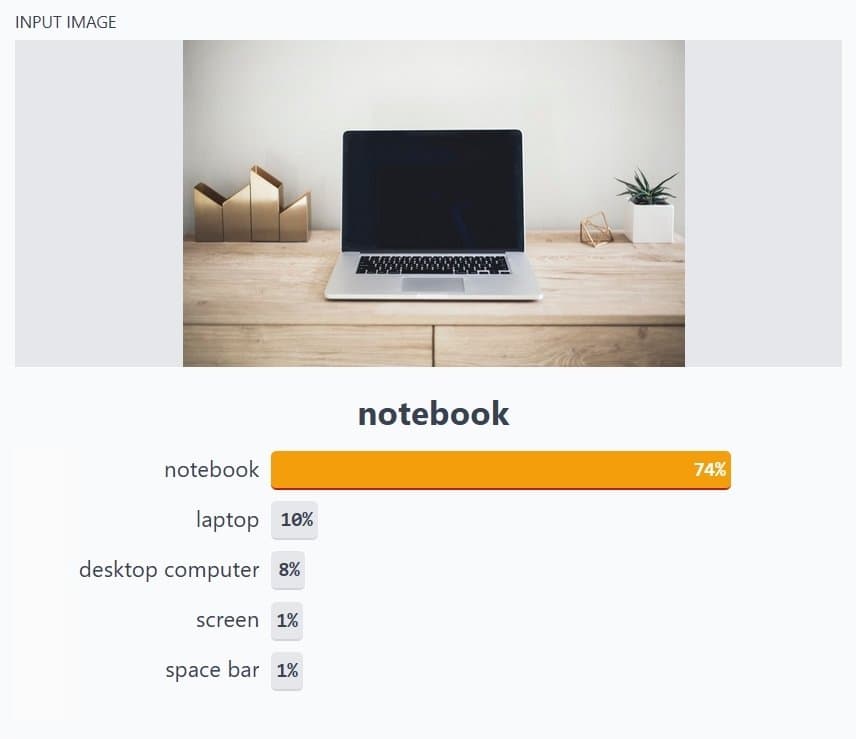
With the idea of transfer learning, Computer Vision engineers have built scalable solutions in the business world with a small amount of data. Existing architectures for image classification include ResNet-50, ResNet-100, ImageNet, AlexNet, VggNet, and more.
What is Image Processing?
Image processing is a key aspect of AI vision systems since it involves transforming images to extract certain information or optimize it for subsequent tasks in a CV system. Basic image processing techniques include smoothing, sharpening, contrasting, de-noising, or colorization.
The de facto standard tool for image processing is OpenCV, initially developed by Intel and currently used by Google, Toyota, IBM, Facebook, and so on.
Image preprocessing removes unnecessary information and helps the AI model learn the images’ features effectively. The goal is to improve the image features by eliminating unwanted falsification and achieving better classification performances.
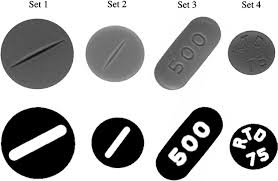
A common application of image processing is super-resolution. This technique typically transforms low-resolution images into high-resolution images. Super-resolution is a major challenge most CV engineers encounter because they often get the model information from low-quality images.
What is Optical Character Recognition?
Optical character recognition or optical character reader (OCR) is a technique that converts any kind of written or printed text from an image into a machine-readable format.
Existing architectures for OCR extractions include EasyOCR, Python-tesseract, or Keras-OCR. These machine learning software tools are popularly used for Number Plate Recognition as an example.
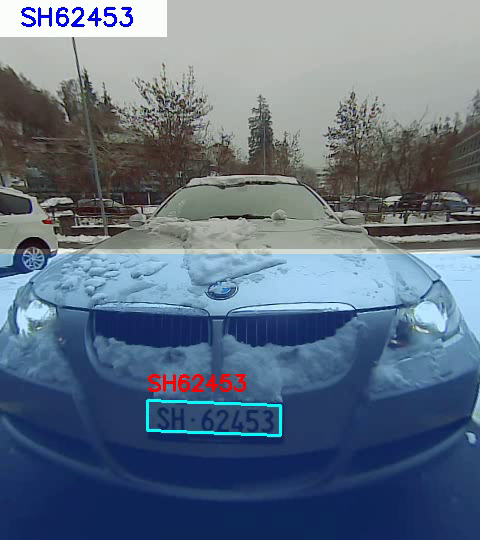
What is Image Segmentation?
While image classification aims to identify the labels of different objects in an image, instance segmentation tries to find the exact boundary of the objects in the image.
There are two types of Image Segmentation techniques: Instance segmentation and semantic segmentation. Instance segmentation differs from semantic segmentation in the sense that it returns a unique label to every instance of a particular object in the image.
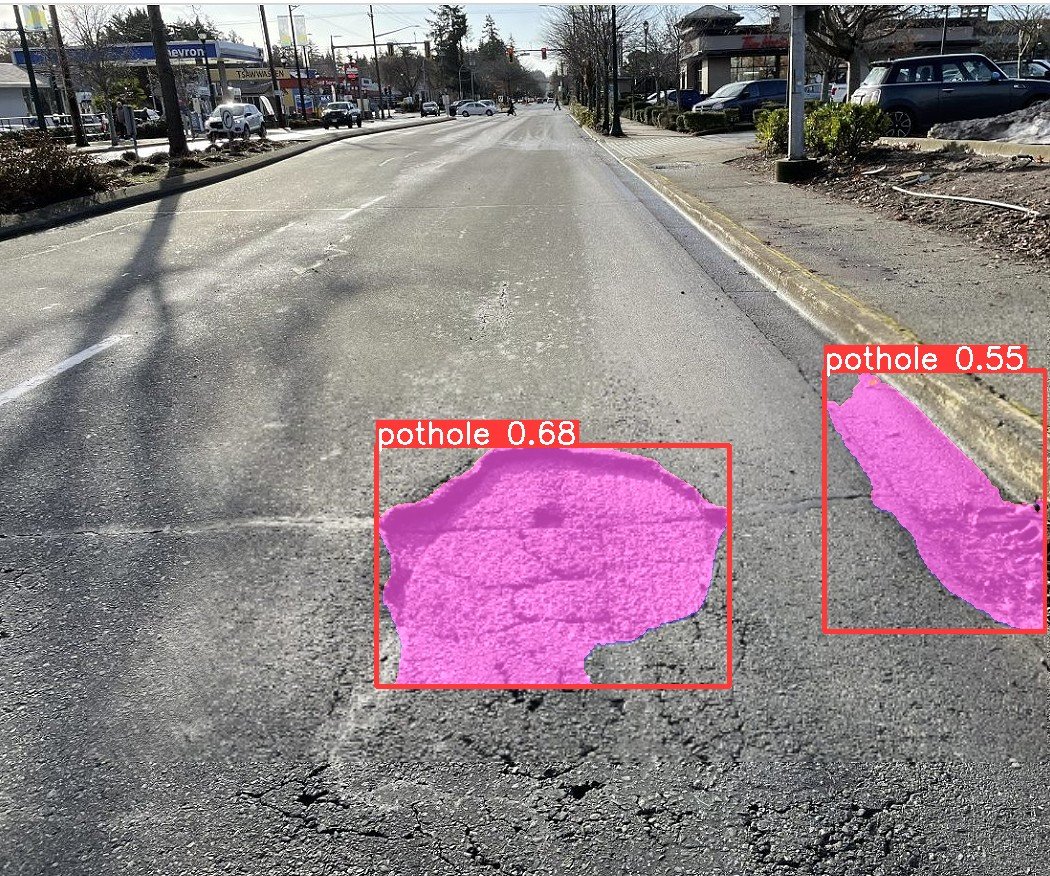
What is Object Detection with Vision AI?
Object detection focuses on detecting an object in an image and then tracking the object through a series of frames.
Object Detection is often applied to video streams, whereby the user tracks multiple objects simultaneously with unique identities. Popular architectures of object detection include the AI vision algorithms YOLO, R-CNN, or MobileNet.
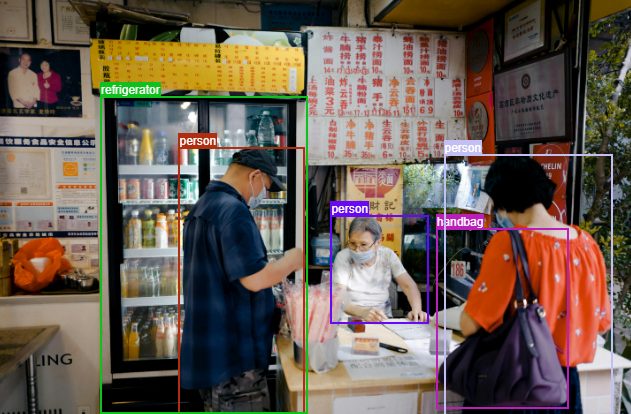
What is Pose Estimation?
Pose Estimation makes computers understand the human pose. Popular architectures around Pose Estimation include OpenPose, PoseNet, DensePose, or MeTRAbs. These are useful for real-world problems including crime detection via poses or ergonomic assessments to improve organizational health.
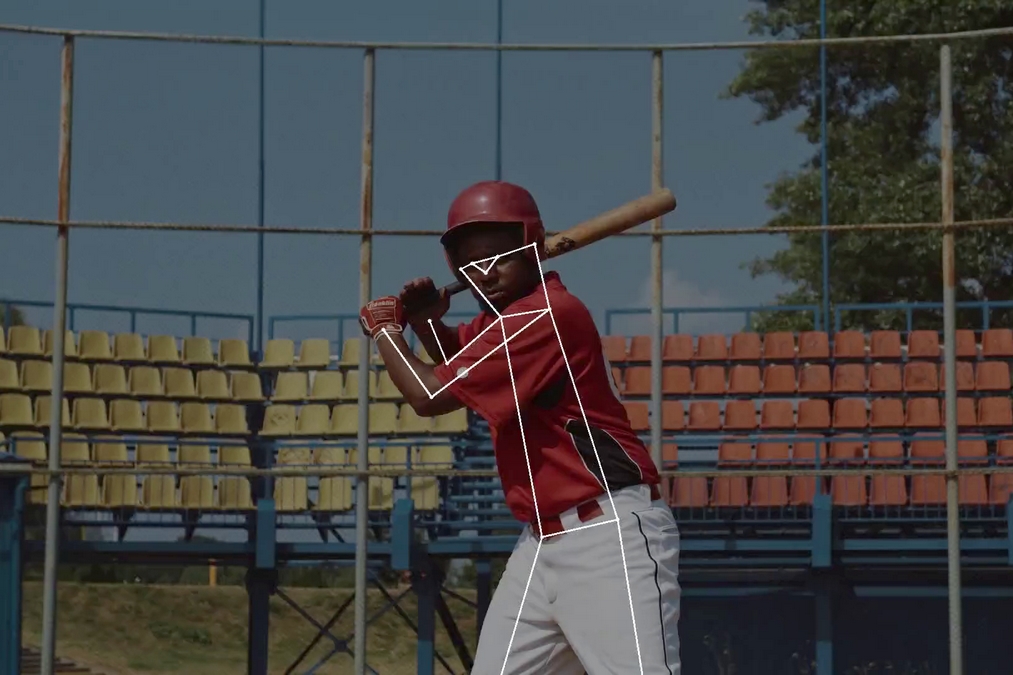
Read More Expert Articles related to Computer Vision AI
Computer vision is an imperative aspect of companies using AI today. If you enjoyed this article, we suggest you read more about the topic:
- The 11 Most Popular Computer Vision Tools
- Learn about reasons why computer vision projects fail – and how to succeed.
- View an extensive list of real-world AI vision applications across industries.
- Read an easy-to-understand guide about what is machine learning and deep learning.
Implement Computer Vision AI Projects
Industry leaders worldwide deliver their computer vision projects with Viso Suite infrastructure to build, deploy, and monitor computer vision applications.
- Viso Suite is the leading enterprise computer vision platform that provides visual programming to develop applications.
- One platform provides automated tools and robust infrastructure to build scalable Edge Computer Vision systems.
- The best AI models and AI hardware are supported out of the box, while the applications are fully customizable and scalable to suit business needs.
The business value of Viso Suite stems from the fact that it is the only truly end-to-end computer vision infrastructure. When implementing computer vision solutions, businesses typically start with point solutions. These point solutions only take care of a specific step in the application development and management process. This could be model training, data annotation, or security.
While this provides a short-term solution, it is not realistic for evolving with the needs of the use case or business. Additionally, stringing together multiple tools and platforms quickly becomes complicated and expensive.
Omitting the need for point solutions, Viso Suite provides a unified computer vision infrastructure. This infrastructure allows businesses to retain complete control over all building, deploying, and maintenance of their computer vision applications.