AI and computer vision will transform the whole future value chain of insurance. Use cases and opportunities abound everywhere. AI fosters more powerful risk assessment systems, gaining advantages from risk assessment, AI-triggered automation, and forward-looking analytics.
New risk management systems are so powerful and disruptive that they have the potential to flip insurance business models upside down. Key AI applications of computer vision include risk management, risk estimation, claims management, and real-time asset or process monitoring.
This article provides an overview of visual Artificial Intelligence (AI) in insurance to trigger efficiencies and new applications.
- Key insurance technology trends for the adoption of AI vision and deep learning
- New and valuable real-world use cases of computer vision model in Insurtech
- Practical examples of applications in the insurance industry
About us: Viso.ai provides the leading end-to-end Computer Vision Platform Viso Suite. Global organizations use it to develop, deploy, and scale all computer vision applications in one place, with automated infrastructure. Get a personal demo.
AI Technology Trends for Insurers
Digital transformation and value-creating AI are of rapidly increasing importance for the business model of insurance companies. In the insurance sector, innovation is driven by emerging AI technologies that impact the entire value chain. Hence, there is a huge interest by the leading insurers to push digitization to the next level by leveraging risk-relevant and behavioral data gathered with distributed sensors and machine learning.
Sensing the Physical World
The complex and massive amount of data gathered by sensors such as cameras requires machine learning to process information. AI tech is applied to transform data into insights and automation to trigger efficiencies and new applications in insurance.
Today, AI adoption in the insurance industry is still far beyond its full capabilities. An important reason is that machine learning applications rely on masses of data hardly available in insurance.
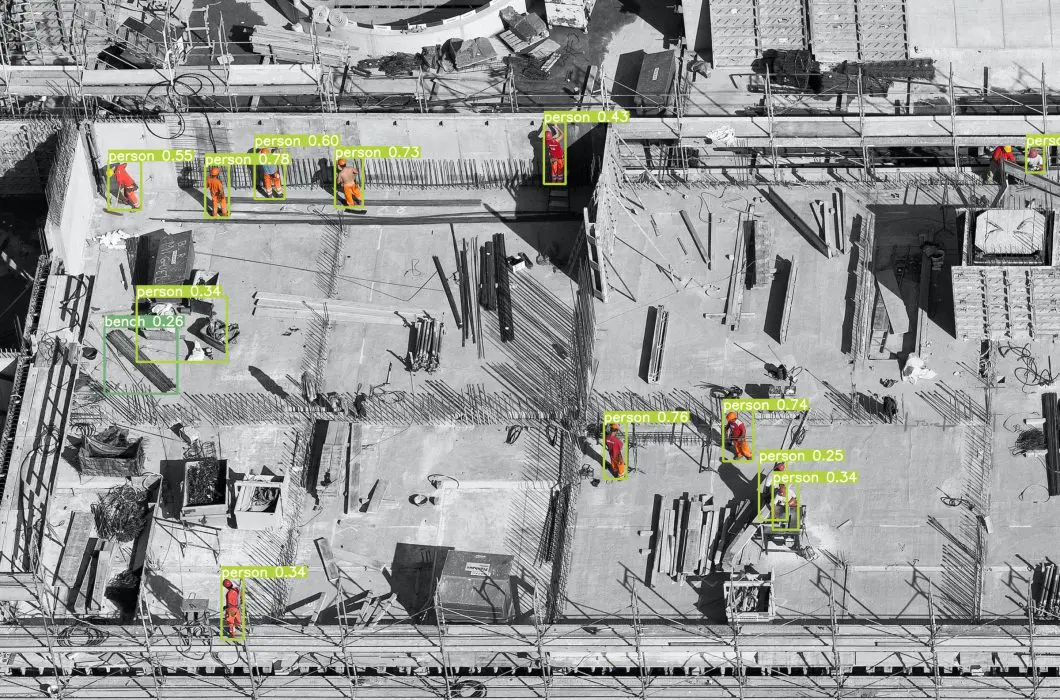
As we enter the era of the Internet of Things (IoT) and Artificial Intelligence (AIoT), AI adoption in insurance will benefit tremendously from real-world data generated by connected sensors. Visual sensors such as cameras and on-device computer vision provide a highly scalable method for AI vision intelligence.
In general, computer vision works in three basic steps:
- (1) obtaining image data/video from a camera,
- (2) processing the image with AI models, and
- (3) understanding the image.
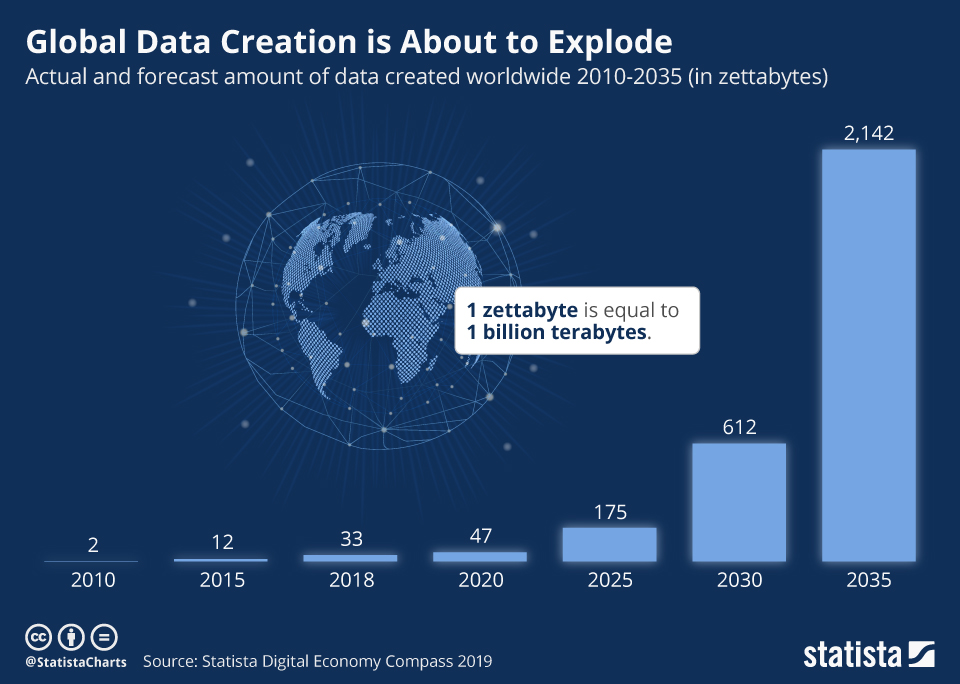
Key Technology Trends in Insurtech
Digitalization in the insurance industry is driven by a range of emerging technologies such as the Internet of Things and Big Data. The immense amount of data created at the edge (connected devices with sensors) requires AI to analyze and understand the data.
Traditional, cloud-based web applications require centralized processing in the cloud (data offloading), limiting adoption because of limited reliability, security, privacy, performance, connectivity, latency, and scalability. Therefore, recent megatrends around Edge Intelligence (Edge AI) move AI processing tasks from the cloud towards the edge, near the sensor that produces the data.
This approach of on-device machine learning helps overcome the limits of cloud computing and enables the implementation of ubiquitous real-world AI solutions.
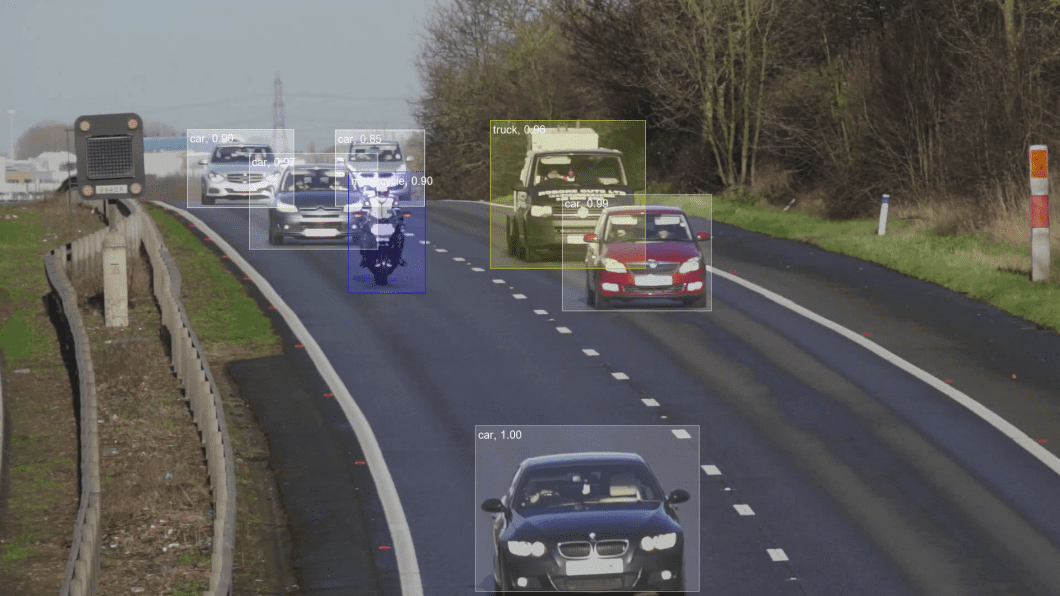
Benefits and Opportunities for Insurers
The benefits of those new technologies allow insurers to build large-scale AI solutions and better assess risks. This further results in benefits to improve customer experience and relationship management, data mining to detect periodicity in the context of underwriting cycles, and to better predict customer profitability.
AI Technology is Highly Disruptive
The relevance of big data and AI insurance applications is substantiated by the ability to collect, process, and understand large amounts of data. This is fundamental for gaining valuable insight from semi-structured and unstructured data stemming from different data sources.
AI systems leverage big data to gain a better understanding of customers and interactions between customers and insurers (customer service). For example, AI analytics convert big data to understandable results for insurers by explaining how customers interact or make buying decisions. AI enables methods to make it possible to interpret and understand a high volume of complex data, where conventional methods of analytics fail.
Ability to Scale Real-world AI Vision
Many applications of machine learning depend on massive amounts of data to train the ML model. Especially in computer vision, deep learning applications need image datasets to learn. As described earlier, the new megatrends shift AI closer to the edge. Hence deep learning is moved from the cloud to sensors and devices where data is generated and processed.
Edge AI extends the cloud and enables scalable real-world applications, reliable offline capabilities, decentralized system risks, and privacy-preserving data processing on-device.
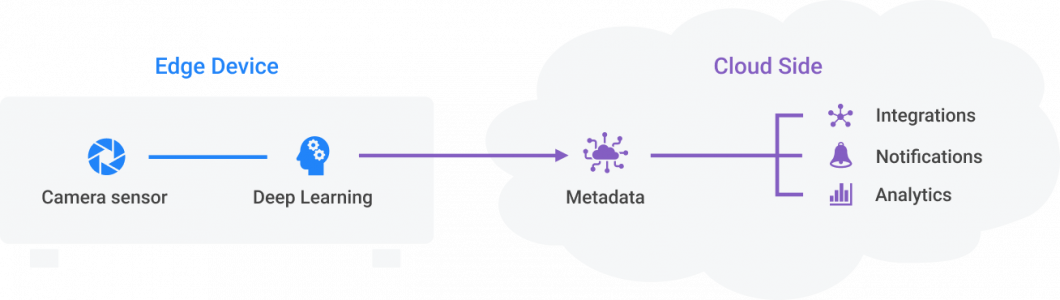
On such edge devices, however, computation power and storage capacity are typically scarce resources. Complex, data-hungry algorithms require high computing resources and are difficult to execute in constrained environments.
Hence, more powerful AI hardware, optimized edge devices, and neural network accelerators such as Vision Processing Units (VPU) or Tensor Processing Units (TPU) enable large-scale Edge AI use cases with fleets of connected edge devices. Edge computing requires the effective distributed deployment of down-scaled and edge-adapted algorithms (optimized models) such as Google TensorFlow Lite.
Solution-Specific AI Vision Systems
Sensing the real world with AI vision is the basis for a wide range of applications that leverage the data gained from AI models to automate operational workflows. However, data collection, deployment of AI models, and remote fleet monitoring required for edge AI applications are still very complex and challenging to scale.
Viso Suite helps companies build and deploy their own AI vision systems effectively with end-to-end edge computer vision infrastructure. It integrates over 20 tools and provides development features with automated infrastructure to build, deploy, and scale custom AI vision applications.
Large enterprises, state-owned organizations, and AI startups use cutting-edge technology to deliver their AI vision solutions much faster, with flexible modules. The following video shows how the computer vision platform can be used to build applications.
The following chapters will describe practical examples of insurtech computer vision applications.
Top Applications of Computer Vision in Insurance
- Application #1: Risk assessment with computer vision
- Application #2: Industrial IoT (IIoT) and Artificial Intelligence
- Application #3: Forward-looking Risk Estimation
- Application #4: AI vision in underwriting process automation
- Application #5: Fraud prevention with AI vision
- Application #6: AI to understand new and complex risks
Risk Assessment with Computer Vision
Computer vision technology is used to process real-world information to assess specific risks more precisely, faster, and more objectively than humans. AI can find patterns in various scenarios to use computer vision in risk assessment. A popular example is remote sensing, for example, to analyze flood risk.
AI vision can perform sentiment analysis for real-time risk scoring with deep learning. The data can be used for dynamic pricing based on individual risk factors.
While multiple academic examples have been discussed and implemented, insurers experience difficulties in realizing the opportunities in actual business processes. Only a few productive implementations are widely deployed as of today, something that is very likely to change.
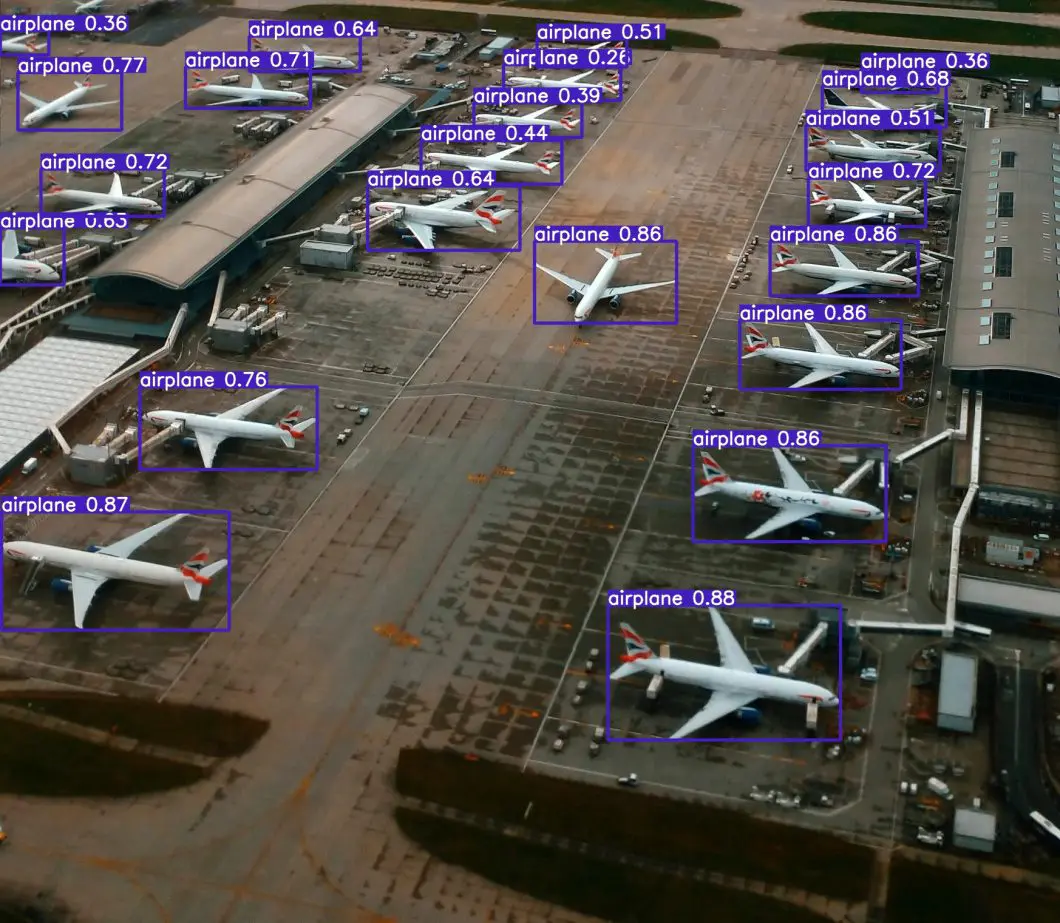
Industrial IoT (IIoT) with Edge Computer Vision
Information from IoT provides data that AI models can analyze to create vast opportunities in the insurance sector. Until now, and despite the high potential of IoT, insurers lack ways of finding relevant information in the mass of sensor data. Here, deep learning is expected to accelerate large-scale applications of industrial IoT with vision sensors (cameras).
Condition monitoring provides accurate information on an asset’s historical usage and current state. Asset tracking with physical devices includes RFID, LoraWAN, GSM network, or Bluetooth modules (“chips”) to enable an evaluation of the current risk exposure of assets or trade goods.
Here, computer vision has been using camera sensors as a very scalable method. Compared to other methods, there is no need to attach physical devices (contactless). Also, inexpensive, common security cameras (CCTV, etc.) can be used to provide video streams to cost-effectively monitor multiple objects and situations in parallel.
In combination with internal (ERP) and external data (weather, etc.), such information facilitates the quantification of complex risks such as business interruption. For example, the use of equipment on large construction sites can be tracked (for example, machinery or power hubs).
A wide range of information is important to the insurer: if eligible employees are using the equipment, if cases of accidents are covered, if processes are executed in a prescribed way, and if there are signs of failure that would cause insured damage to the site.
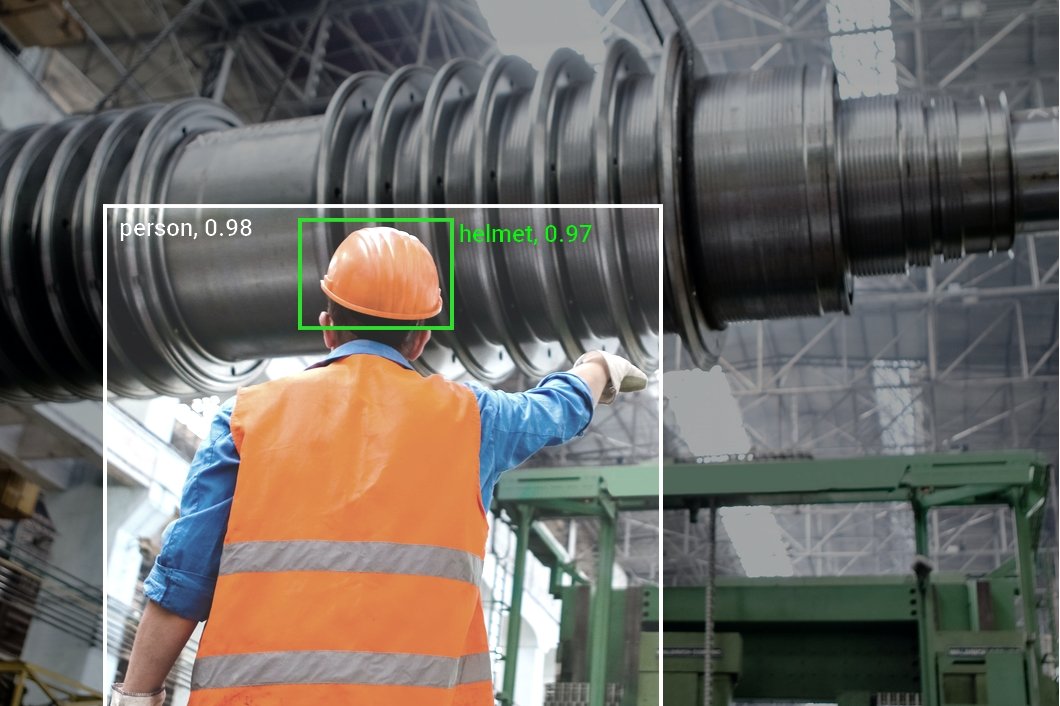
IIoT Digitization
Through digitization, the insurer obtains transparency and can use the data directly or use AI to detect patterns, spot impending failures, and predict real-time risk based on the number and type of contractors present.
In automobile insurance, the customer is awarded a premium reduction based on data from a drive recorder or crash recorder. Hence, the data of visual insights provide further relevant information.
In short-living goods logistics such as food transportation, insurance companies develop IoT-controlled parameterized products to ensure cargo ship freight and logistics delivery.
IIoT vision applications in agriculture allow contactless monitoring of livestock in large-scale farming to analyze risks of biological hazards by detecting dead animals and early indicators of disease.
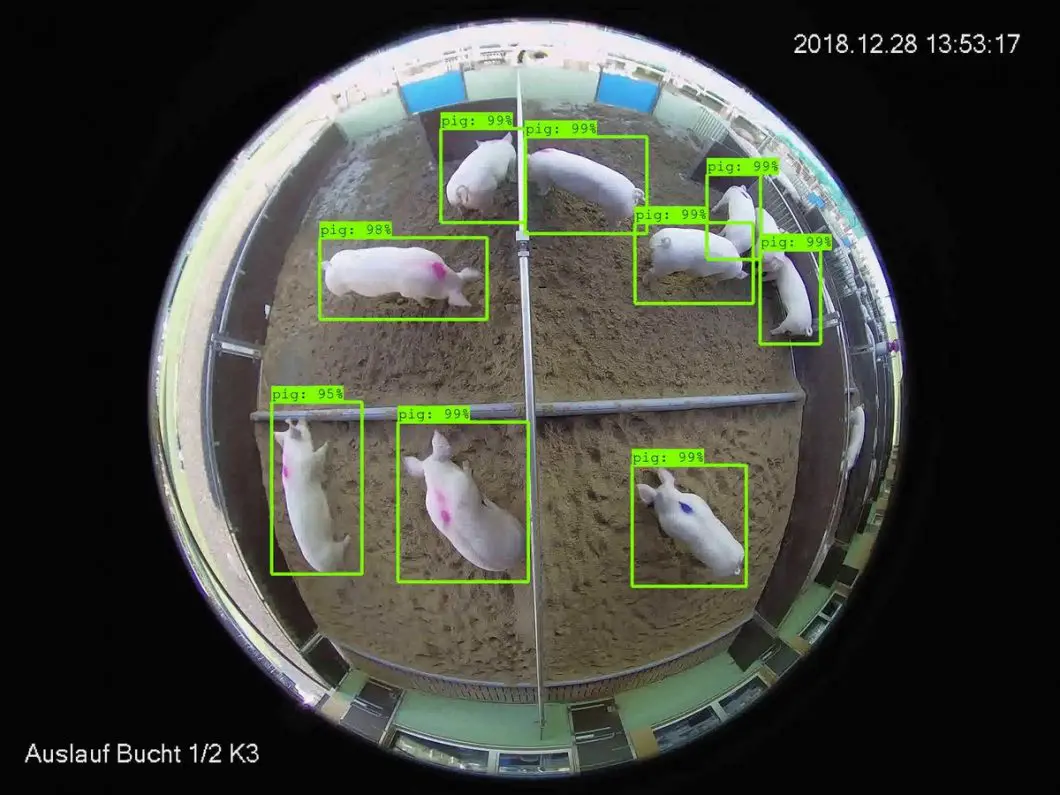
Forward-looking Risk Estimation
In industrial manufacturing, production machines are often insured yearly, with some carrying individual insurance contracts or contracts for entire production plants.
Industrial AIoT is gaining momentum to measure the machine state at all times to deliver a real-time understanding of machine operation and condition monitoring. With unknown risks getting transparent to better estimate and minimize risks of failure across production lines and plants.
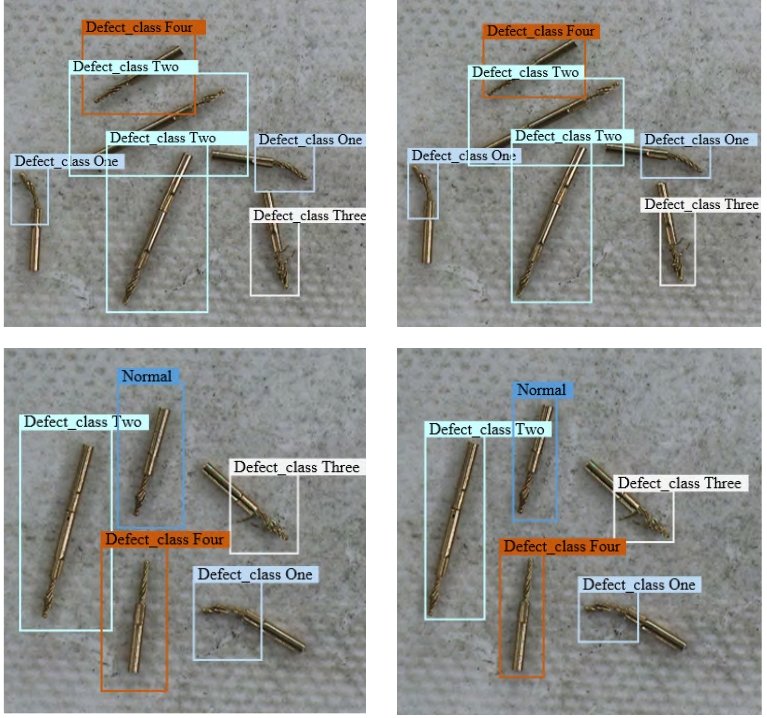
Vision-based condition monitoring helps to take preventative measures against machine failure and lower business interruption risk while increasing overall equipment effectiveness. In addition, insurance customers profit from lower premiums if an insurance company offers such a scheme. Key technologies are real-time object detection, situational monitoring, intrusion, or event detection with video AI analysis.
AI in Insurance for Underwriting Process Automation
The value chain of office processes is often characterized by a variety of different software applications. Hence, underwriters spend a considerable amount of their time manually transferring data from one software application to another while spending only a little time with higher-value tasks such as reasoning from information, selling, or engaging with brokers.
About 80% of data in today’s insurance companies is text. Hence, NLP (Natural language processing) is regarded as one of the most widely implemented AI technologies today. Here, artificial intelligence is used for automated interactions, cognitive applications, and automatically providing relevant information using semi-structured information.
Since underwriting tasks involve a high volume of documents, often paper-based, the extraction of structured information from scanned documents plays an important role. Therefore, AI-enabled optical character recognition (OCR) is used to save time and manual labor. The extracted information can be used for creating recommendations for the underwriter, such as referring to similar cases.
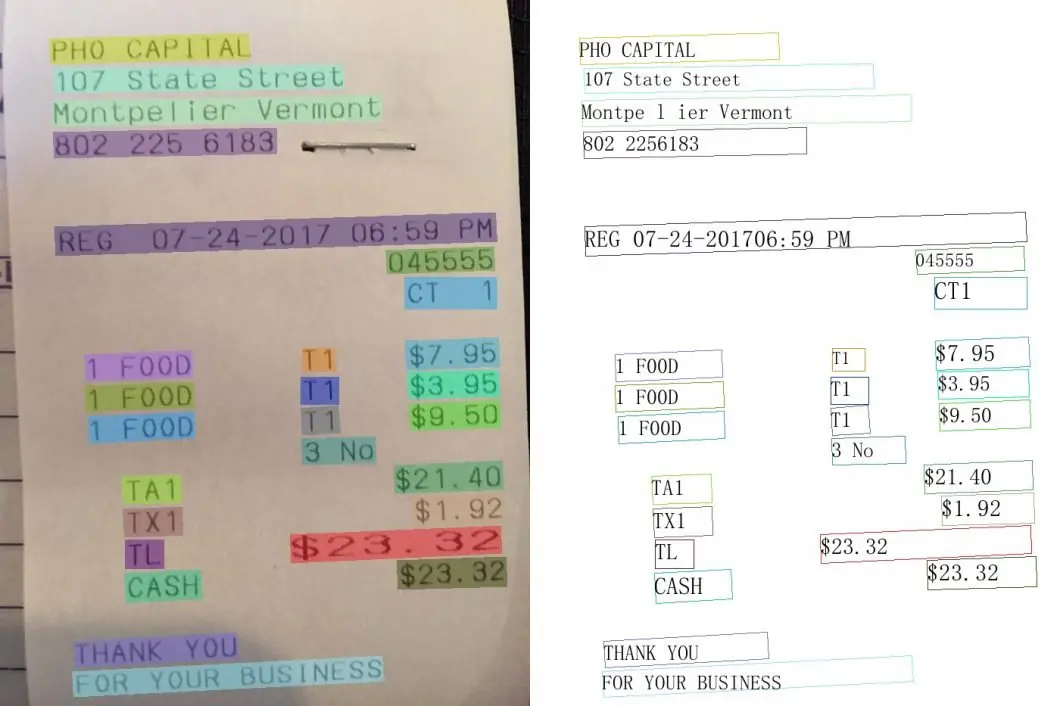
Fraud Prevention with Computer Vision
Exploiting behavioral data such as facial expressions or the tone of voice at the moment of underwriting is typical for fraud prevention with machine learning. For example, in life insurance or health insurance, it is expected that over 40% of risk information is obtainable from behavior monitoring alone.
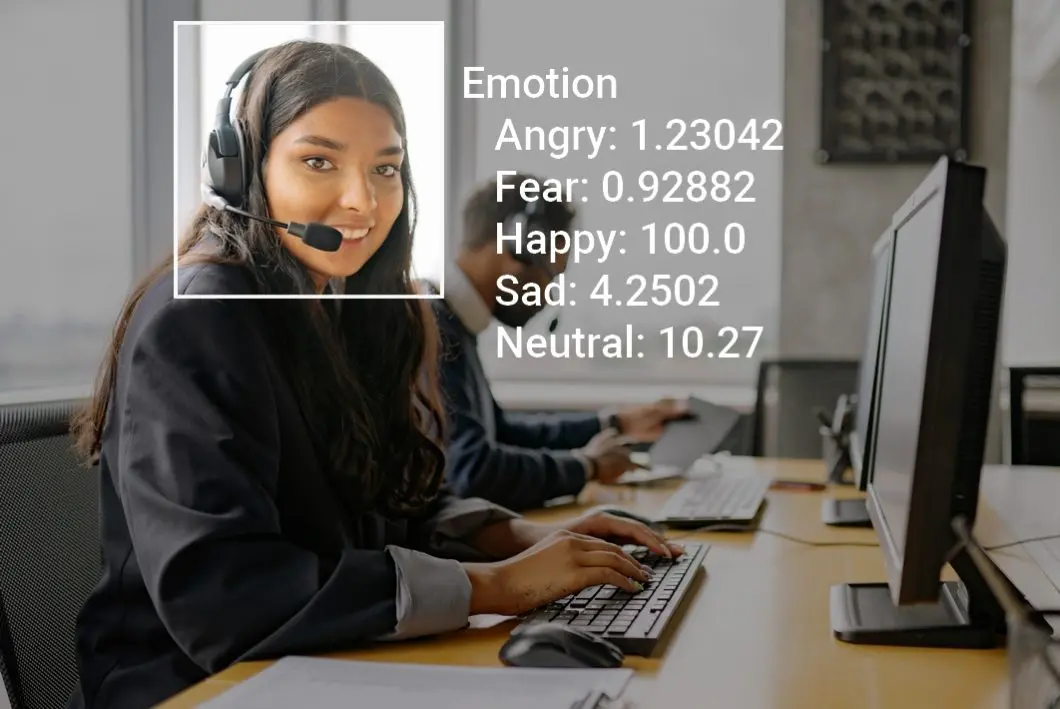
But also in non-life insurance, behavioral data is essential. For example, identifying certain patterns in how an employee operates a machine indicates process issues that could lead to insurance claims processing.
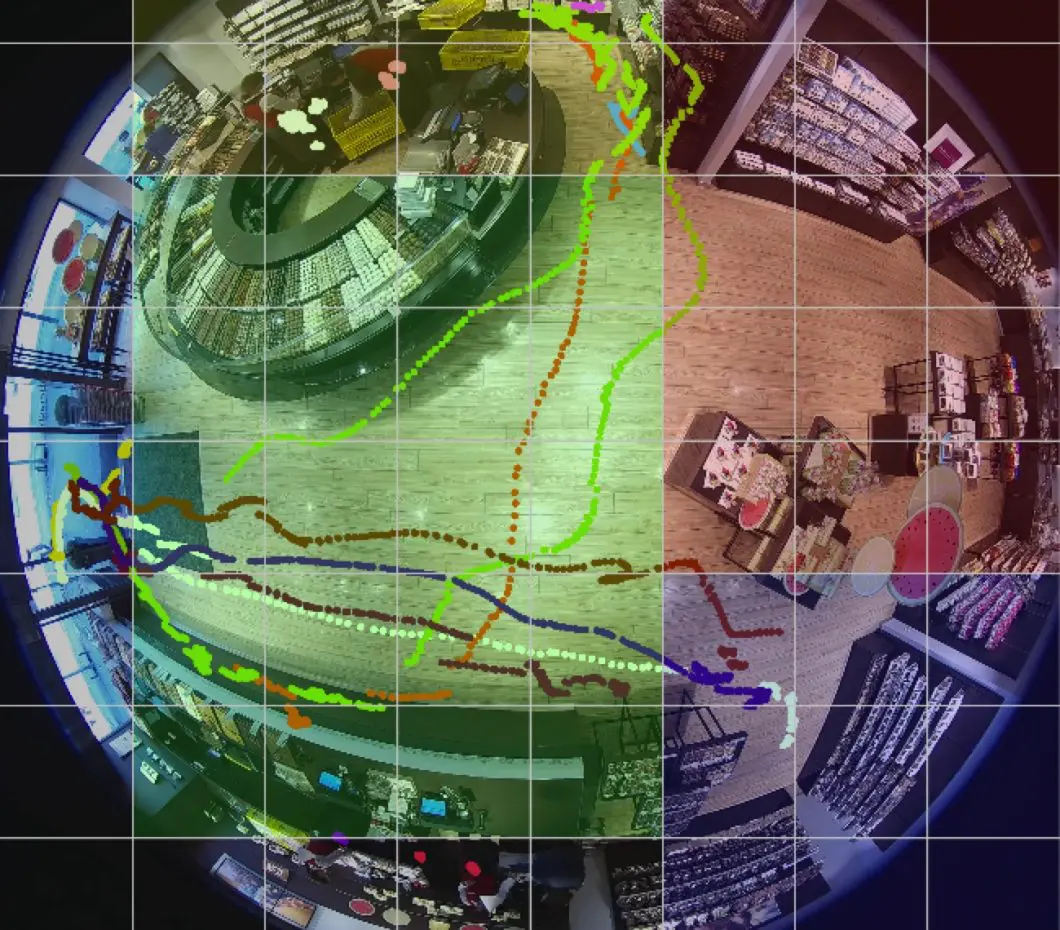
AI To Understand New and Complex Risks
According to the Allianz Risk Barometer, the most important global business risks of today are
- Cyber incidents
- Business interruption
- Natural catastrophes
- Pandemic outbreak
- Changes in legislation and regulation
- Climate change
- Fire, explosion
The complexity of risks continuously increases, and new risks cause unprecedented levels of disruption to businesses and their supply chains. Emerging technology and artificial intelligence will increase the interpretability of business risks by extracting patterns and making complex risks manageable.
Examples include intelligent systems to detect and mitigate factors of business interruption or natural catastrophes. Another example is automated monitoring of compliance with guidelines such as social distancing or mask detection. These applications provide a risk score to quantify and track risks across multiple locations (distributed AIoT systems).
Climate change is a major root cause of supply chain interruption, mostly resulting from high risks of extreme weather. Climate impacts company-internal processes that might cause business interruption. For example, lowered water levels prevent cooling in industrial manufacturing with a direct impact on production. The quantitative assessment of such risks is critical for the pricing of insurance products, and to design of parametric products.
How to Get Started
Building AI insurance applications with computer vision for large-scale systems is a highly complex problem; it requires stitching together numerous software and hardware platforms.
Therefore, we’ve built the premier computer vision infrastructure, Viso Suite. It allows insurance leaders to deliver enterprise-grade, secure, and scalable computer vision applications dramatically easier and faster. The end-to-end solution provides a comprehensive set of tools to cover the entire application lifecycle of deep learning vision systems. The streamlined infrastructure allows for 10x faster time to business value, without writing code from scratch that is difficult and costly to maintain.
Explore showcase applications to see what companies have built on Viso Suite. Reach out and request a live demo for your organization.
To read more about related topics, you might be interested in the following articles: