This article provides vital insight into key applications of computer vision in the pharmaceutical industry. Computer vision, especially deep learning, is one of the key emerging technologies that help digitize the fields of medicine, biology, life sciences, and manufacturing.
AI vision applications leverage Artificial Intelligence with the Internet of Things (AIoT) to process the image of camera sensors for image recognition and object detection.
AI technology in the pharmaceutical industry
Industry 4.0 for pharmaceutical manufacturing
Along with the evolution of medicines in the last two centuries, the manufacturing methods for their production have advanced from small-scale manual processing to large-scale production as part of a trillion-dollar pharmaceutical industry.
Today’s pharmaceutical manufacturing technologies continue to evolve rapidly as the Internet of Things (IoT), artificial intelligence (AI), robotics, and advanced computing begin to challenge the traditional approaches, methods, and business models for the manufacture of pharmaceuticals. The application of these new technologies is expected to define the next generation of manufacturing, also described as Industry 4.0 or Pharma 4.0.
New thinking will be required to realize Industry 4.0 for pharmaceuticals and overcome the inertia of current manufacturing infrastructure, operations, and regulatory requirements. While implementing these new technologies will certainly not be easy, it may well be worthwhile as they bring the potential for higher output, increased manufacturing safety, improved quality, increased agility, better value, new flexibility, and reduced waste.
Computer vision in pharmaceutical industry automation use cases
Artificial Intelligence leverages advanced computing and machine learning for data analysis to enable machines to replicate and surpass human intelligence. Computer Vision is the sub-discipline of AI that focuses on image processing with algorithms to imitate human vision with the use of sensors and cameras.
State-of-the-art methods in computer vision are mainly based on deep learning, a subset of machine learning (ML). Deep learning methods leverage deep neural networks (DNN) to train a model on annotated ground truth data and then apply the model to gain an understanding of new images.
The application of AI vision in pharmaceutical manufacturing has already begun with prominent use cases, including machine vision technology to replace human visual inspection of packaging, labels, caps, and glass vials. Predictive equipment maintenance and monitoring are used to reduce disturbances, production downtime, and safety risks. Automated computer vision quality control is used to enable continuous process quality assurance, for example, in equipment cleaning.
AI applications in the pharma industry aim to generally improve and optimize manufacturing processes while also reducing human intervention in the production process. The application of emerging technologies has the disruptive potential to dramatically increase the efficiency, agility, flexibility, and quality of the industrial production of medicines.
Key challenges of industry 4.0 adoption
Today, the pharma industry is still very much transitioning into Industry 3.0 by adopting continuous manufacturing and employing advanced PAT (Process Analytical Technology). Intelligent and AI-enabled systems may enable end-to-end automation and customer demand-driven manufacturing, operations, and even dispensing.
Need for new regulatory frameworks
The need to operate in existing regulatory standards can be a significant barrier to technological innovation in pharmaceutical industries. Especially the lack of regulatory precedence can lead the industry to maintain conventional processes even when new technologies would reduce the overall regulatory compliance burden and lead to increasing quality and efficiency.
Another regulatory challenge is the burden of filing regulatory applications across multiple global jurisdictions that have different regulatory expectations, especially for new manufacturing technologies.
Need for new technology platforms
Most challenges related to Industry 4.0 adoption will be technical challenges that early adopters need to solve. The ability to implement AI systems requires new software and hardware infrastructure. AI-based automation systems require capturing, processing, and retrieving large amounts of stored and real-time data.
The technical complexity of AI systems is based on the need to integrate numerous platforms and tools, incompatible data models, and different architectures. Modern computing paradigms combine Edge Computing with Artificial Intelligence (Edge AI) to overcome the limitations of the cloud by processing data in real time with on-device machine learning. The advent of Edge AI allows the implementation of robust, distributed, large-scale AI systems.
Especially in pharmaceutical AI applications, digital information is usually highly sensitive, such as info on the drug discovery process. This proprietary data or patient data requires integrated security and data protection. In most computer vision use cases, real-time and local data processing is needed to avoid sending videos to the cloud.
Top computer vision in pharmaceutical applications
Computer vision provides the ability to automate a wide range of complex tasks that traditionally depend on trained operators. Compared to other sensor technologies, camera solutions are highly efficient, contactless, and have a minimal operational footprint that impacts existing processes and equipment while providing a great amount of information. Compared to traditional machine vision systems, deep learning allows using conventional cameras and a very flexible and scalable system design.
There are numerous applications of computer vision during the pharmaceutical production process. One of the most urgent problems from the point of view of industry productivity and competitiveness is automatic inspection. Early detection of defects in the production process means lower cost and faster feedback on the production line to eliminate the causes of defects. Example applications include label detection, batch number monitoring, liquid medicine volume detection, and so on.
In simple terms, computer vision systems acquire the images and video streams from cameras and analyze them using AI software to detect specific objects, conditions, events, anomalies, or deviations.
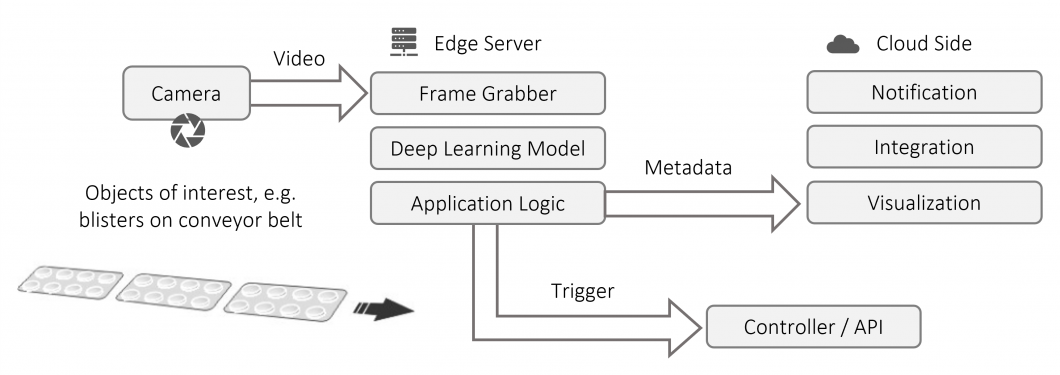
In the following, we will discuss the following computer vision applications for pharmaceutical manufacturing:
- Application #1: Quality inspection of capsules using AI vision
- Application #2: Pharmaceutical bottle-packaging detection and counting
- Application #3: Automated defect detection for blister packaging
- Application #4: Blister package recognition and identification with Deep Learning
- Application #5: Detection and classification of imprinted pharmaceutical tablets
- Application #6: Infusion bag detection with Deep Learning models
- Application #7: AI vision inspection of cleaning in pharmaceutical manufacturing
Computer vision quality inspection of capsules
Real-time quality inspection of gelatin capsules in pharmaceutical applications is an important issue from the point of view of industry productivity and competitiveness. The two-part gelatin capsules (body and cap) are sensitive to common flaws caused by equipment problems and inconsistencies in the manufacturing process that make them unacceptable for marketing and shipment. Defects include incorrect size, color, dents, cracks, holes, size, bubbles, dirt, double caps, or missing caps.
Quality inspection is critical; issues cause revenue loss, waste of manufacturing time, increase in manufacturing and resource costs, and uncertainty about product quality that could affect a manufacturer’s reputation. Traditionally, trained workers monitor a conveyor belt of capsules and remove any defective products manually. However, the drawbacks of manual inspection are consistency, costs, quality, and objectivity.
The automatic quality inspection with computer vision provides an automated solution to address those problems. Therefore, industrial image processing software analyzes digital video images from one or multiple cameras. Due to the real-time requirement of the application, a piece has to be inspected within a few milliseconds.
There are different approaches to inspecting capsules with camera sensors. Conventional machine vision systems apply a set of complex image processing techniques such as gray-level thresholding. Cutting-edge deep learning techniques train and deploy machine learning models. Industrial deep learning software applications are significantly more robust, flexible, and scalable.
The AI vision application works by acquiring the image of the capsule, applying a deep learning model to perform the quality inspection by classifying the capsules, and a custom system controller to pass the accepted and rejected capsules to the appropriate container.
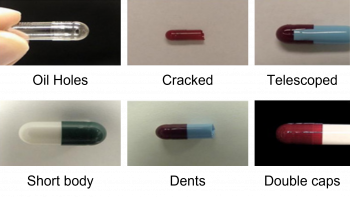
Pharmaceutical bottle-packaging detection
Computer vision technology is used for the packaging detection of pharmaceutical glass bottles. Machine learning methods play an increasingly important part in the automation of packaging line detection systems. It allows real-time detection and large-scale quality control across multiple locations.
In a bottle detection system, the computer vision system collects glass bottle images and uses AI vision software with deep learning models for bottle detection and counting, rule-based judgment, and decision-making. Automated quality analysis plays a critical role in pharmaceutical production processes; the goal is to improve the quality and accuracy of defect detection in the control process.
Automated visual inspection for blister packaging
In pharmaceutical blister packaging, part of the recommended security standard involves inspecting tablets in each blister before it is sealed. An automated visual inspection system helps to detect missing and broken tablets, and tablet fragments, as well as the color, size, and shape of individual tablets.
Intelligent detection based on ML technology provides a rapid and automated method for drug production quality detection and control in drug production. For this AI application, images of defect-free blisters are used to train a deep learning model for detecting the nonuniformity of blisters, detecting the positions of tablets in blisters, and detecting the color of tablets.
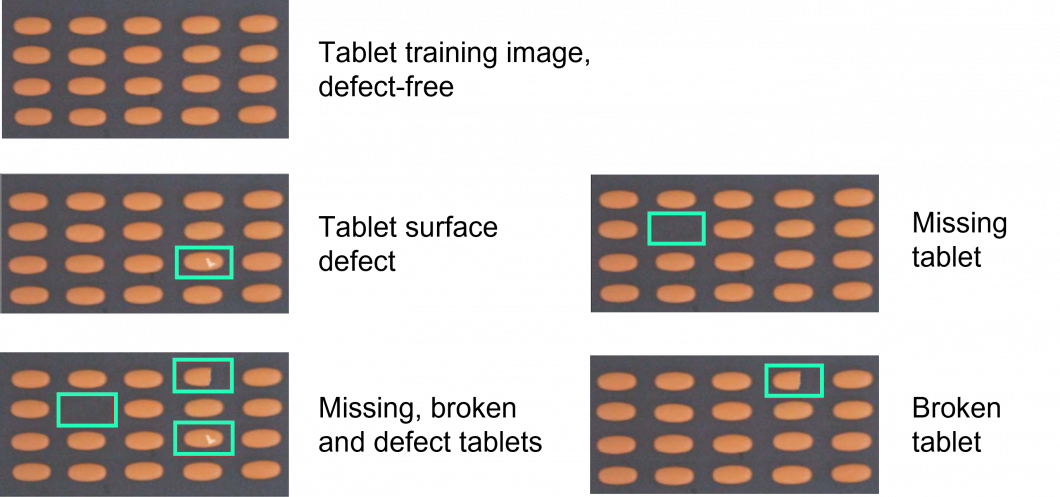
Blister package identification with deep learning
Correct identification of blister packages carries utmost importance at dispensing stations, where numerous prescriptions are to be efficiently dispensed by pharmacists. The usual presence of several hundreds of similar-looking, but completely different types of blister packages, in a crowded dispensing station makes it prone to human error, posing serious safety and health concerns for a patient’s life. Furthermore, human errors are inevitable due to various reasons, including fatigue, stress, heavy workload, or distraction.
To automate and facilitate the correct identification of blister packages, deep learning methods have been introduced to accurately detect and identify blister packages. Researchers used the highlighted deep learning (HDL) method to automatically detect and then segment the raw blister pack images irrespective of position and lighting.
Subsequently, a convolutional neural network (CNN) is used to perform image classification to identify the correct blister pack type. Using a ResNet CNN classifier, a database of 272 blister packages was used to test the application in a real scenario, yielding almost 100% accuracy over the entire testing set.
Automated visual inspection of imprinted pharmaceutical tablets
Automated visual inspection of tablets can, in contrast to manual tablet sorting, provide objective and reproducible tablet quality assurance. Hereby, visual inspection of the ever-increasing numbers of produced imprinted tablets is especially demanding. The goal is to ensure that the regulations are enforced for the unambiguous identification of active ingredients and dosage strength of each tablet.
Deep learning models are trained on annotated images of tablet images with imprinted tablets, with and without typical production defects. The algorithm is trained on manually classified tablets into good (defect-free) and multiple defective classes. Field tests have shown image analysis methods to provide a promising tool for automating visual inspection of imprinted tablets.
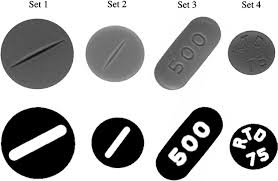
Infusion bag detection with convolutional neural networks
In the production of pharmaceutical infusion bags, manual quality inspection is of great importance. However, it is very challenging because defects may be difficult to recognize.
Applications use neural networks to detect infusion bags placed on a try, even when overlapped. The transparent plastic filled with a transparent fluid is challenging to detect and requires a set of image pre-processing steps to facilitate the object detection tasks.
Cleaning pharmaceutical equipment
In pharmaceutical manufacturing, the control of cross-contamination is of great importance. Pharmaceutical manufacturers must validate their cleaning process to ensure compliance with regulations. Batch-to-batch cleaning is required to ensure that any built-up residues of previous batches are not transferred to the next batches of the same product. It further ensures that equipment is adequately cleaned to manufacture further batches.
Vision systems can be trained to detect fragments, residues, and dirt to increase the effectiveness and consistency in the cleaning of pharmaceutical production equipment. Hence, computer vision applications can help to ensure cleaning procedures and improve the safety, efficacy, and quality of the subsequent batches of drug products.
Vision systems can help to detect cleaning mechanisms, classify physical activities, and monitor equipment use. Specialized AI vision applications can detect the occurrence of specific activities such as brushing, scrubbing, or manual cleaning. The advantages of automated cleaning inspection include assurance of quality and safety, compliance with government regulations, reduction of quality costs, less downtime, and higher operational efficiency.
The bottom line
Traditionally, pharmaceutical factories depend on manual or semi-automatic quality inspection that depends on manual operators, training, and experience. The downsides of manual quality assurance are limited accuracy, subjectivity, and consistency. In the meanwhile, the stakes are high and quality issues can cause expensive business interruptions and threaten competitiveness. For pharma companies, zero-defect quality products are highly required in competitive markets.
In recent years, with the development of computer vision technology, digital image processing has advanced dramatically. Deep learning is closing the gap between humans and computers, with machines already achieving human-level performance and above for certain tasks. Given the disruptive impact of AI on manufacturing processes, it is only a matter of time before custom computer vision systems will become a widely adopted mainstream technology.
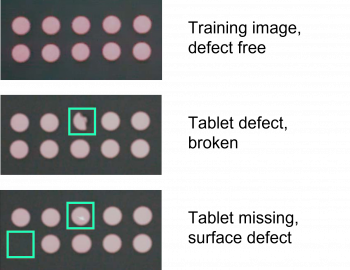
Getting started
At viso.ai, we enable pharmaceutical companies to build and operate their computer vision applications on next-gen software infrastructure. Industry and technology leaders worldwide trust Viso technology to deliver powerful AI vision systems.
The end-to-end platform Viso Suite provides an integrated set of tools to cover the full application lifecycle. From image annotation and training of specific object detection models to secure deployment and privacy-preserving deep learning at the edge, Viso Suite provides ML teams with full-scale control. Use cutting-edge visual programming with automated development features to bridge the gap between business and IT teams.
Get in touch with our team and learn about the costs, timeframe, and scope of an initial proof-of-concept that suits your needs.