In 2021, companies in logistics and parcel delivery face high-cost pressure and prioritize innovation to save costs or increase revenues to stay competitive. In the following, we focus on a case study featuring a computer vision-based aftermarket truck collision avoidance system.
The on-device deep learning system is based on viso.ai, a cloud platform for AI vision applications that enables businesses to build, manage, and operate their own AI vision applications. For collision avoidance, we leverage the latest AI hardware technologies such as AI chips specialized for video processing (Vision Processing Unit, VPU), and take advantage of edge computing capabilities in the resource-constrained environment of a delivery van.
The case study was developed with Intel and a leading multinational postal organization to digitize delivery logistics, collision avoidance AI, and mitigation systems work prevent accidents with computer vision.
About us: Viso Suite is the end-to-end computer vision system consistently delivering 695% ROI for enterprises automating their business processes. By consolidating the entire machine learning lifecycle into an easy-to-use infrastructure, Viso Suite eliminates the need for point solutions and delivers quality application support at the highest level. To learn more, book a demo with our team.
The need for collision avoidance systems
The global parcel delivery market is growing at an unprecedented speed due to online sales. As a result, traditional postal companies face enormous cost pressure and must take action to stay competitive.
Delivery vans make up a significant share of the postal vehicle fleets. Delivery vans are a key cost driver due to expensive maintenance and upkeep. This primarily stems from inefficiencies, disconnected systems, collision accidents, property damage, and personal injuries.
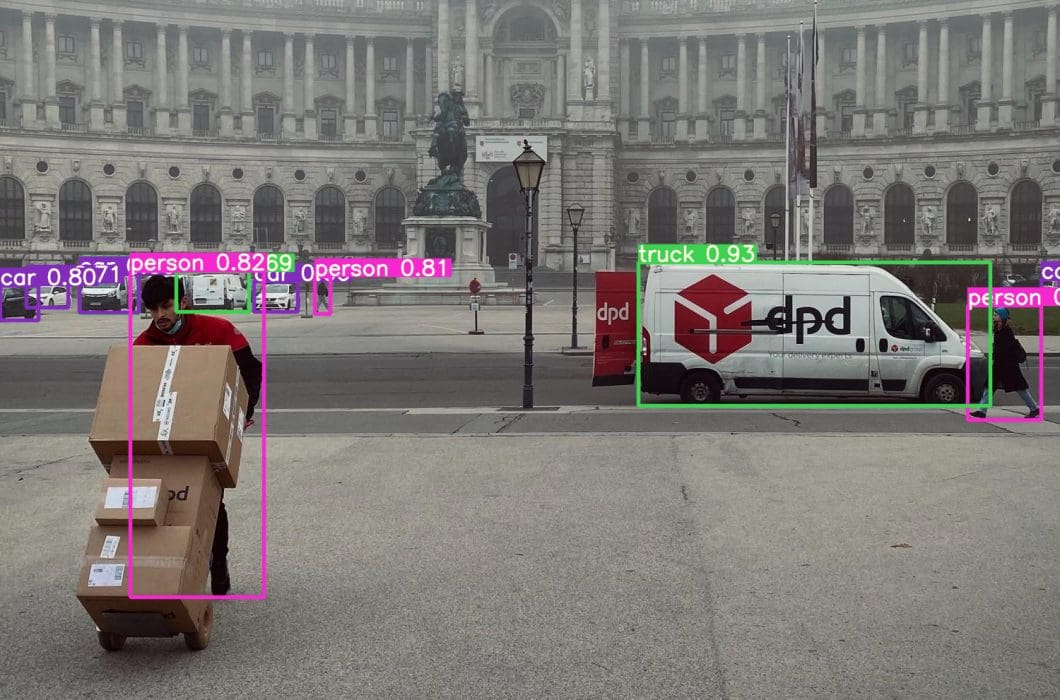
To manage the rapidly growing number of dispatched parcels, the fleets are generally expected to grow in accordance. At the same time, delivery vans are the direct touchpoint to the end client, hence crucial for the company’s reputation and perceived product quality.
Cost-related problems
- High costs due to accidents and damage. As time pressure increases with a growing number of packages out for delivery, the number of accidents and associated direct costs for repair are at an all-time high. Additionally, van damage directly impacts the company’s reputation – especially if an accident causes bodily harm.
- High costs due to time-consuming processes. Along with accidents come time-consuming processes such as legal fees, indirect costs to fill in damage reports, or arranging replacement vehicles to avoid interruption to delivery service. Moreover, current reporting accidents often face media discontinuity (e.g., fill in forms by hand and on paper, copy them into another system).
- High costs due to parcel theft. Parcel delivery vans are a popular target for theft. As a result, urban areas face increasing numbers of stolen packages, mainly electronic goods. While postal organizations try to control this, there’s still no effective way to prevent and solve these crimes. Often, the only way is to increase staffing for certain routes, hence driving up costs and further impacting profitability.
A multi-functional collision avoidance system for trucks in delivery logistics will help to solve these challenges.
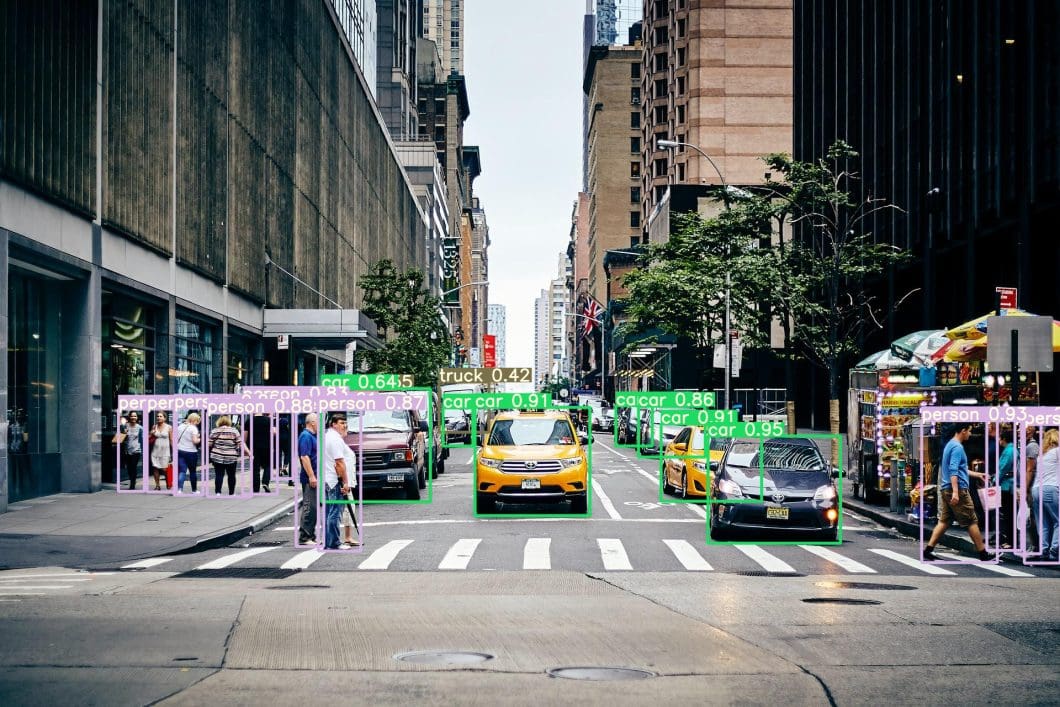
Challenges of in-vehicle computer vision
The environment in delivery vans is harsh, causing technical challenges to implementing a vision-based system:
- High AI processing power is required. Vision-based applications are resource-intensive. Especially the real-time video processing of multiple streams in parallel requires powerful computing hardware. In addition, in-vehicle computer vision applications face space constraints that affect cooling and require a small form factor.
- Power and vibration constraints. Van batteries are exposed to continuous stop-and-start activity and are easily depleted, so additional device power consumption must be minimized. In addition, the hardware is exposed to continuous vibration inside the delivery van, so it must be vibration-proof.
- Scalability and cost effectiveness. While the system must be scalable to thousands of vans, it must have a low enough cost to achieve ROI. Therefore, expensive technologies or non-modular systems cannot be used. The total cost of implementation matters as the possibility to dynamically replace or upgrade single parts of the system.
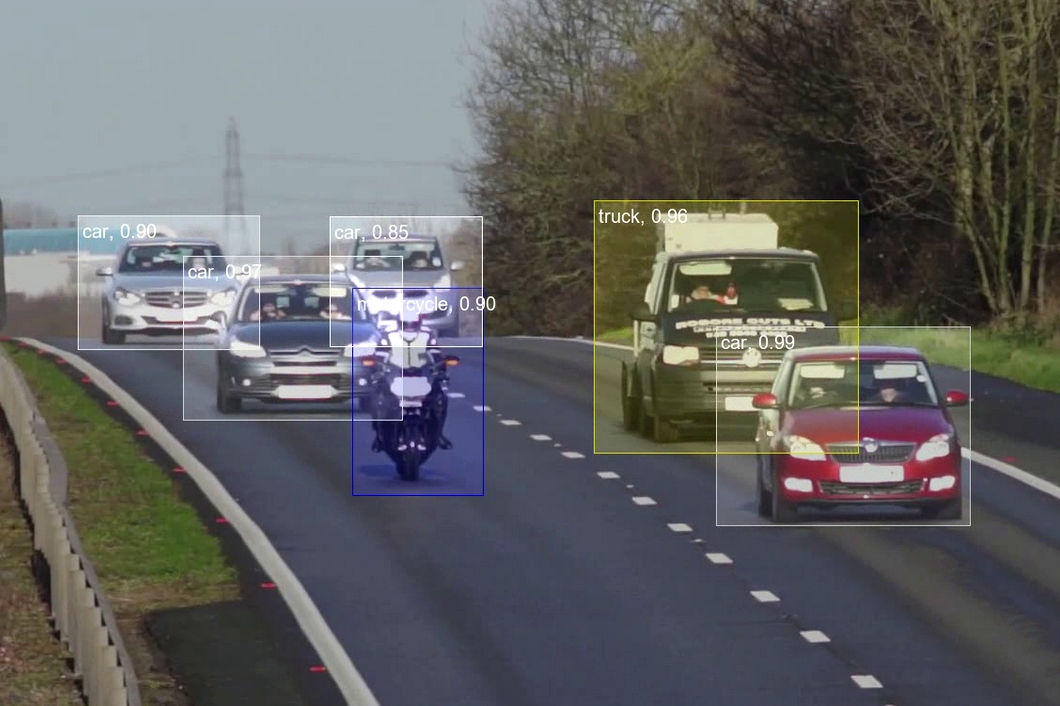
Edge computing enables near real-time AI and analytics
Today, many transportation providers rely on disaggregated data platforms and independent point solutions. Logistics companies seek to connect the fleet with the environment and turn data into insights, achieving fast, efficient, and informed use of logistics systems.
Viso.ai technologies enable AI and computer vision analytics in near-real-time, helping support public safety or digitize business processes. For example, with Edge Computing and inference, logistics companies benefit from fast response times, free up bandwidth, and help keep sensitive data private.
Features of the next-gen aftermarket collision avoidance system
While vehicles become smarter, operations often cannot benefit from automotive safety systems because of sensitive business information, certification and development processes, or operative challenges (e.g., leased fleet, changing manufacturer).
A vision-based solution, based on the viso.ai platform powered by Intel AI technologies such as the Intel Neural Compute Stick 2, uses optical sensors around delivery vans to connect the vehicles with the environment, hence automating last-mile delivery processes. The business automation system is independent of any van manufacturer and can be equipped at or after van procurement in less than two hours.
As the vans are now connected to the cloud and have computing resources locally available, the system can easily integrate future innovations. The initial product focuses on decreasing the costs related to delivery vans by providing the following main functionalities:
- Accident Prevention. The van driver gets a 360° view of the delivery van without blind spots on the tablet. This allows for real-time, ease of access to any video stream. Compared to automotive-grade systems, the cameras are mounted at optimized positions (above the surroundings) providing high-resolution streams and warning systems.
- People Safeguard functionality. The deep learning model detects people around the delivery van for accident prevention and warns the driver in real time based on an alert system about potential obstacles.
- Automated workflows. All workflows related to damage management are digitized and can be completed directly on the tablet before the report is synced on a cloud-based damage management portal.
- Theft detection. The system detects anomalies around the van, flags the situation, and starts recording automatically. If an incident happens, the video file can be exported.
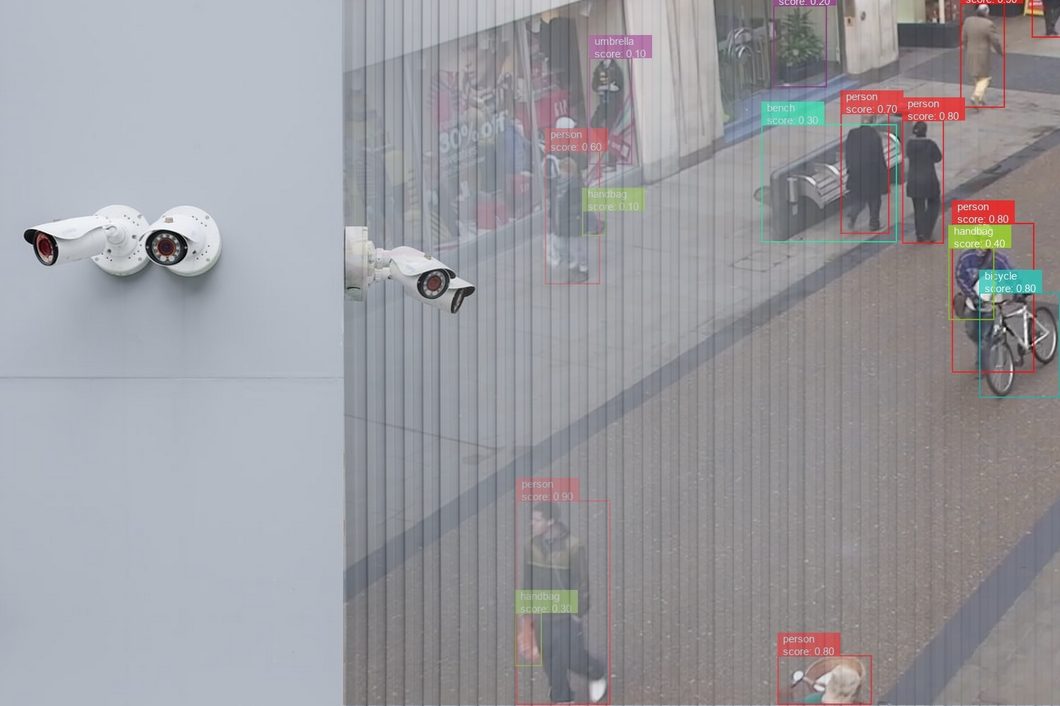
The use case setup
Viso.ai software leverages the latest hardware and software technology from Intel to meet these diverse and challenging requirements. The use case runs on Intel Core processors, combined with the Intel Movidius Myriad X VPU for Deep Learning inference, in a robust and industrial housing. Additionally, the Deep Learning models are optimized using the Intel Distribution of OpenVINO Toolkit, a software kit that helps developers and data scientists speed up computer vision workloads, streamline deep learning inference and deployments, and enable execution across a range of Intel’s AI hardware.
The computer is mounted inside the delivery vans. The Movidius Myriad X Vision Processing Unit provides enough power to perform object detection on three input camera streams in real-time at low electrical power consumption. The output is used to warn the driver about potential obstacles. In addition, the Intel Core i3 processor performs geometrical transformation tasks displaying live video streams to the driver inside the delivery van.
The use of the latest optimized Intel AI technologies has resulted in significant damage-related cost reduction. This is according to initial KPI assessments after the first month of system usage. Further features are now planned to be integrated into the same system to scale the benefits observed even more.
According to Arkadiusz Hruszowiec, Intel Business Development Regional Manager, “this project is a proof-point that our strategy of enabling AI on edge devices works in practice. By using the Intel Distribution of OpenVINO toolkit and Intel Vision Accelerator Solutions, viso.ai can take video streams, analyze them in a deep-learning model, and draw insights in real-time at the edge, which results in real business impact for the end customer.”
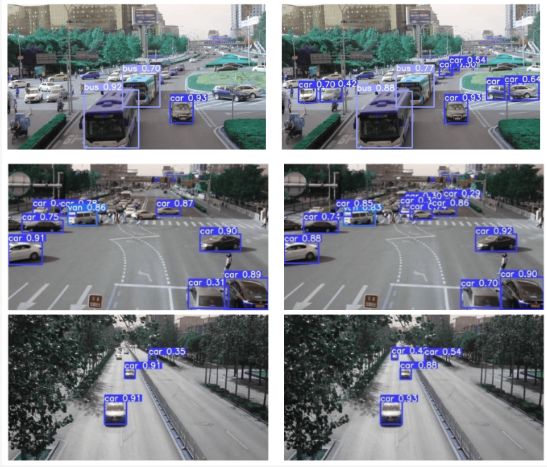
What’s next for AI collision avoidance rechnologies?
By orchestrating visual sensors and algorithms to avoid potential collisions, computer vision systems enable safe and secure parcel delivery. With environmental analysis and obstacle identification, computer vision systems can provide timely warning alerts or automatically adjust the vehicle trajectory.