Shortly after the release of YOLOv4, YOLOv5 was brought into the world. The pace at which the open-source “You Only Look Once” models are progressing is exciting, but the community has questioned if it is too good to be true. Written in the PyTorch framework, YOLOv5 seemingly is faster and easier to use than previous versions. However, with the absence of a published paper, YOLOv5 has indeed experienced a mixed reception of intrigue and controversy.
Who Created YOLO?
Then-graduate student Joseph Redmon and advisor Ali Farhadi created the versions of YOLOv1 to YOLOv3. However, YOLOv4 was introduced by Alexey Bochkovskiy, who continued the legacy since Redmon stopped computer vision research due to ethical concerns.
In a tweet, Redmon claimed that although he “loved the work, the military applications and privacy concerns eventually became impossible to ignore”.
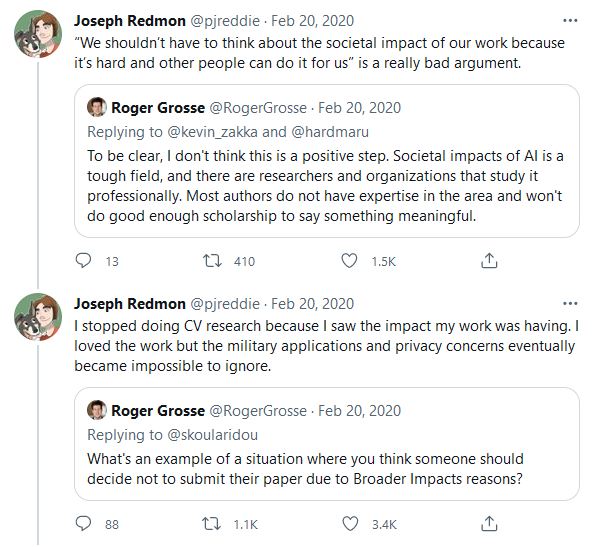
If you want to read more about privacy concerns in deep learning, I recommend reading our Article about privacy-preserving deep learning.
YOLO up to the 3rd version are considered to be large improvements of one another and have vastly different features, with each one being a significant improvement from the last. Joseph Redmon describes the efficiency and architecture of YOLO in his research papers, which provide legitimacy to its use and distinction.
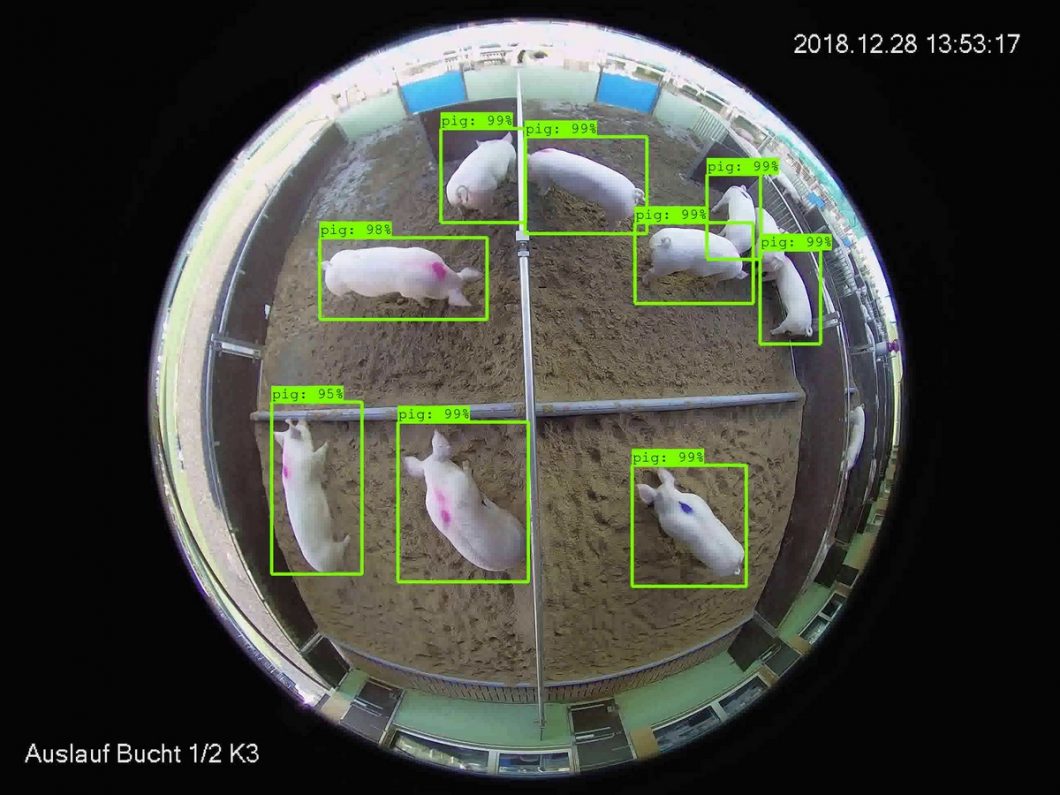
A Brief History of YOLO Models
The various contributors and creators have brought controversy and attention to the YOLO series of models.
YOLOv1
As previously mentioned, YOLOv1 was released in 2015 by Joseph Redmon et al. YOLOv1 made waves in the computer vision community as it proposed a single neural architecture. This new approach made it possible to directly predict bounding boxes and class probabilities from full images in one pass.
However, despite its breakthroughs, YOLOv1 struggled to detect small objects within images.
YOLOv2
Also known as YOLO9000, YOLOv2 was released in 2017 by Joseph Redmon et al. This version introduced various improvements on top of the 2016 YOLO iteration. These improvements included batch normalization, a high-resolution classifier, and anchor boxes. Notably, YOLOv2 was able to detect significantly more object categories by using detection data from the COCO dataset and the ImageNet classification custom dataset.
YOLOv3
YOLOv3, another YOLO version by Joseph Redmon and Ali Farhadi, used a single neural network to simultaneously predict bounding boxes and class probabilities for multiple objects in an image. It improved on the backs of v1 and v2 by introducing architectural enhancements, including feature pyramid networks (FPNs) and multiple scales for detection.
YOLOv4
YOLOv4 was the first YOLO version introduced by Alexey Bochkovskiy, Chien-Yao Wang, and Hong-Yuan Mark Liao. It implemented techniques such as CSPDarknet53 for feature extraction and Spatial Pyramid Pooling (SPP) for improved context modeling. YOLOv4’s architecture is made for faster run inference speed times with high precision, useful in real-time and edge applications.
YOLOv5: What Is Different?
YOLOv5 was released by a company called Ultralytics in 2020. It is published in a GitHub repository (https://github.com/ultralytics/yolov5) by Glenn Jocher, Founder & CEO at Ultralytics, and quickly gained traction soon after its publishing.
The Ultralytics YOLOv5 object detection model was also published on the iOS App Store under the app name “iDetection” and “Ultralytics LLC”. While the Ultralytics page on GitHub is supposedly said to be state of the art among all known YOLO implementations, the version’s legitimacy has remained questionable in the community.
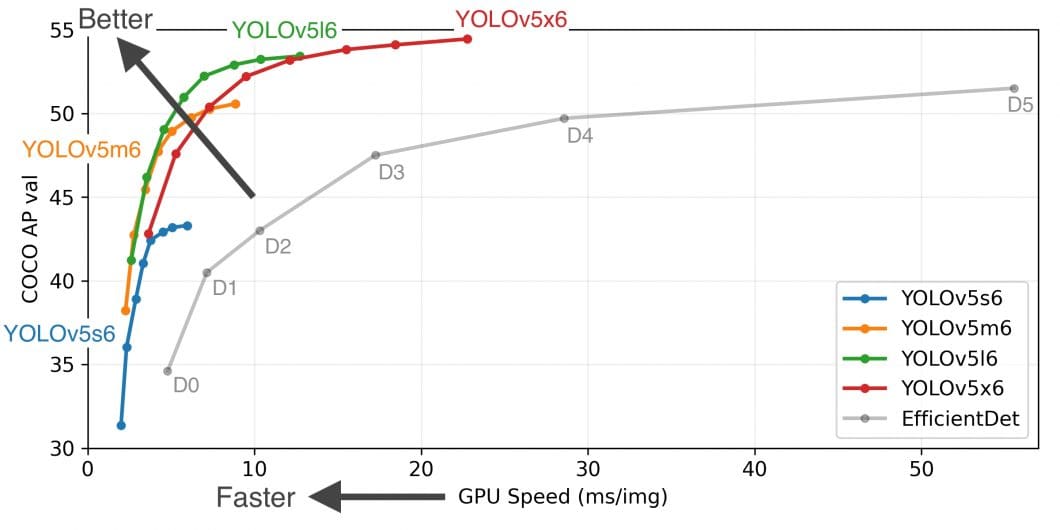
YOLOv5: Limited Literary Support
Redmon’s YOLO versions 1-3 and Bochkovskiy’s YOLOv4 were published in peer-reviewed research papers that supported their performance and architecture use.
The image below is taken from Bochkovskiy’s YOLOv4 research paper. In the paper, he compares the efficiency of YOLOv4 with YOLOv3 and other common object detection algorithms. The accuracy is measured using FPS and Average Precision (AP). YOLO versions 1-3 were discussed similarly in Redmon’s papers.
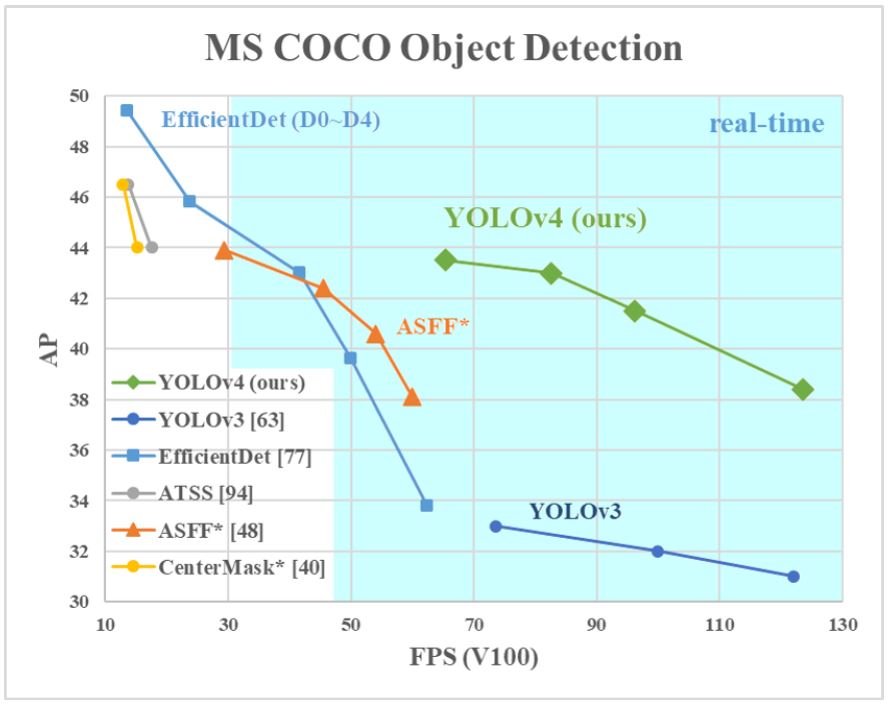
This kind of accuracy review is necessary for the community to understand the comparative efficiency of YOLO versus other algorithms. Unfortunately, YOLOv5 has not been discussed in a formal research paper yet.
When Ultralytics was asked about a potential research paper, Glenn Jocher replied in a GitHub thread in June 2020:
We are extremely limited in resources at the moment, and our priority is architecture and training experimentation to mature on a more stable set of both.
He also stated that Ultralytics has been to submit to the research paper platform arXiv by the end of the year. However, a paper has not been published as of today, but may be underway.
The community has instead been resorting to third-party articles to gain information on YOLOv5’s performance. Consequently, this drives concerns because some articles may not be entirely credible since the authors did not create the architecture, and therefore do not know it as well as the original creators would.
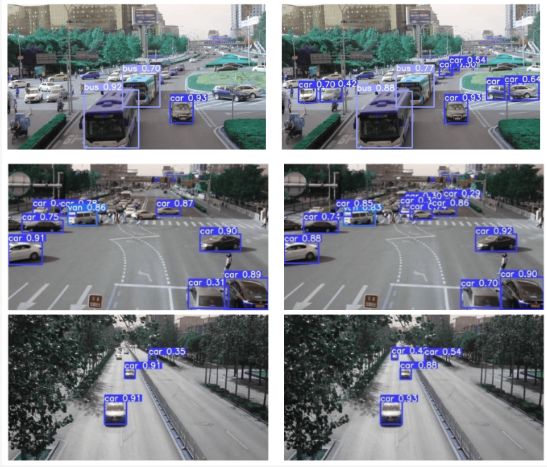
Roboflow YOLOv5 Article Controversy
YOLOv5 was incorrectly discussed by Roboflow, which has thus published another article correcting their mistake. In the original article “YOLOv5 is Here: State-of-the-Art Object Detection at 140 FPS”, multiple facts were misconstrued.
As there aren’t many articles out there on YOLOv5’s performance, this article was looked at by thousands of people and relied on for correct information. However, because it’s not a published paper, there was a margin of error in the calculations found in the article. This caused mass confusion among people wanting to use YOLOv5 as it came out and stained YOLOv5’s credibility.
Alexey Bochkovskiy, the creator of YOLOv4, pointed out that the article had invalid comparison results. He explained in a GitHub thread that the latency shouldn’t be measured with a batch size of 32 but rather with a batch equal to 1.
Latency is the time of a complete data processing cycle, and it cannot be less than processing a whole batch, which can take up to 1 second, depending on batch size. The higher the batch, the higher the latency, so the real latency of YOLOv5 can be up to 1 second.
His other critique was that the Roboflow article compared the speed of much smaller and less accurate versions of YOLOv5 with “very accurate and big YOLOv4”. He said that “they [Roboflow] did not provide the most critical details for comparison: what exactly YOLOv5 version was used, s,l,x,… what training and testing resolutions were used, and what test batch was used for both YOLOv4 vs. YOLOv5”.
Moreover, Roboflow did not test it on the generally accepted Microsoft COCO dataset, with the same settings. They did not test it on the Microsoft COCO CodaLab-evaluation server to “reduce the likelihood of manipulation”.
Naming YOLOv5
Because Bochkovskiy and others are not the original authors of YOLO, the names of YOLO’s subsequent versions have been contested and remain controversial.
In the versions YOLOv4 and YOLOv5, Redmon’s novel feature extraction, Darknet-53, is heavily altered, and so is much of the YOLOv3 architecture in general. The use of YOLOv5 as Ultralytics’ model name has also been frowned upon in the Computer Vision community.
However, YOLOv4 has been recognized by the general community as a valid improvement over YOLOv3, and the name is not so controversial. YOLOv5, on the other hand, has unvalidated data backing its improvement over YOLOv4.
The editors of the original Roboflow article, Joseph Nelson and Jacob Solawetz, eventually published an article called “Responding to the Controversy about YOLOv5”, where they address the issues raised by Bochkovskiy on the GitHub thread. They begin the article by saying they “appreciate the machine learning community’s feedback, and are publishing additional details on our methodology.”
Roboflow, however, although the publishers of misinformation around YOLOv5, are not the publishers of YOLOv5 itself, so the name YOLOv5 remains unchanged within their article as it is at the discretion of Glenn Jocher (founder of Ultralytics) to acknowledge that they may need to change YOLOv5’s name to something more unique.
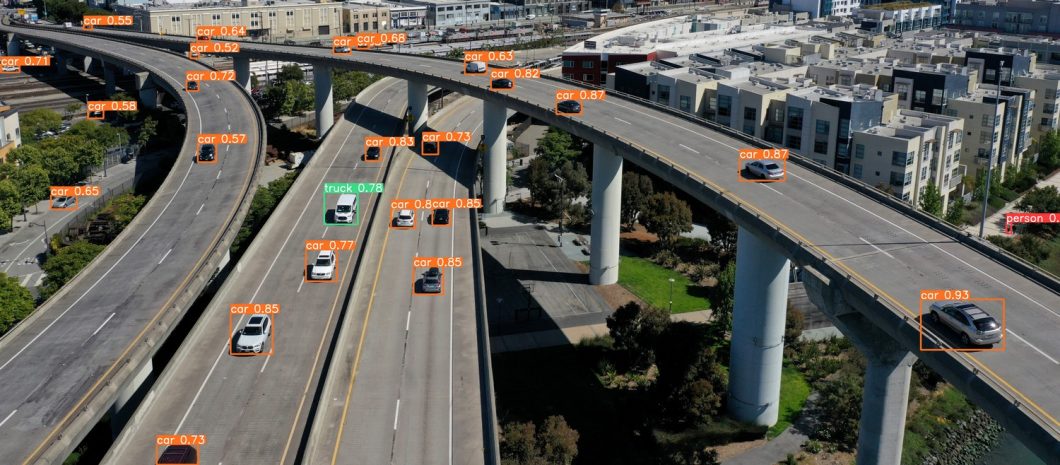
YOLO Licensing
Currently, all YOLO models from the Ultralytics repositories (YOLOv3, YOLOv5, and YOLOv8) are licensed under the AGPL-3.0 license. This license allows users to use, modify, and distribute software covered by the license. However, it imposes additional obligations, particularly for network-based services.
Users must provide access to the source code of any modified versions if they offer their creation as a service. Additionally, any derivative works or modifications must also fall under the AGPL-3.0 license.
On top of the other YOLO controversies from Ultralytics, many users have found the AGPL-3.0 licensing scheme more restrictive compared to the licensing of other computer vision models, including MMDetection and R-CNN. Other common open-source licenses include the Apache and MIT licenses. More information can be found in the Ultralytics YOLO docs and GitHub.
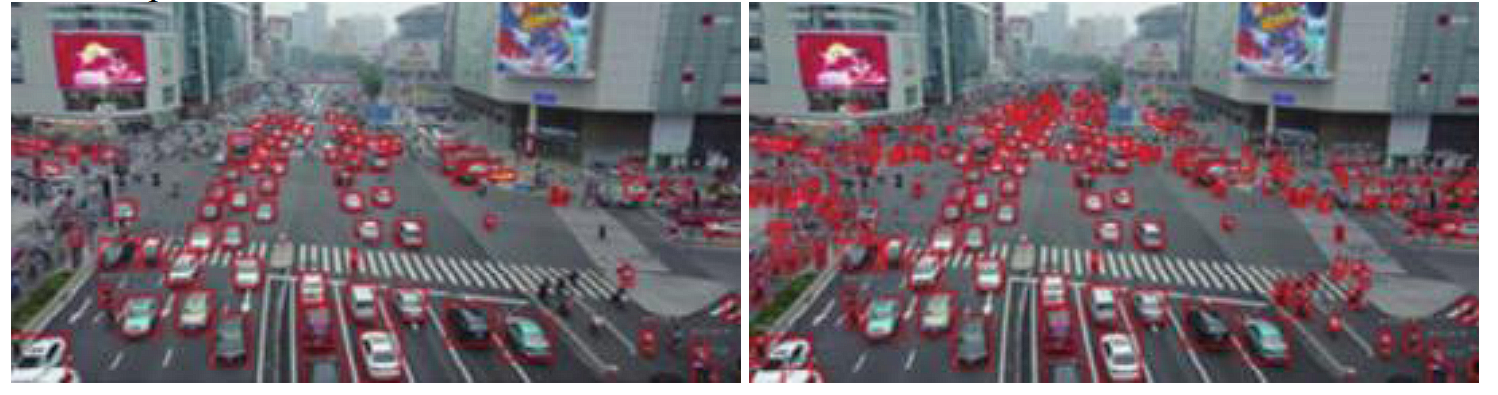
What’s Next With the YOLOv5 Model?
For all researchers, students, hobbyists, and developers, we suggest checking out the YOLO documentation from Ultralytics to get started. There is ample information for individuals working on research and passion projects there.
To get started using computer vision for enterprise-grade applications, please get in touch with a member of the viso.ai team. We will provide a demo and walk you through how Viso Suite can facilitate the intelligent production and management of your project needs. Request a Viso Suite demo
Our guides to the YOLO series: