Intel’s OpenVINO is a powerful deep learning toolkit that enables optimized neural network inference across multiple hardware platforms. In this article, we discuss the features and benefits of OpenVINO, and how it integrates with Viso Suite. By integrating OpenVINO into a computer vision infrastructure, businesses can build and deliver scalable applications.
In particular, you will learn more about:
- What is OpenVINO?
- Why should you use the toolkit?
- How OpenVINO works
- Flagship features of the toolkit
- OpenVINO Model Zoo
- Use cases and advantages
About us: viso.ai provides Viso Suite, the world’s only end-to-end Computer Vision Infrastructure. Our solution supports OpenVINO out of the box and enables large businesses to manage all computer vision applications.
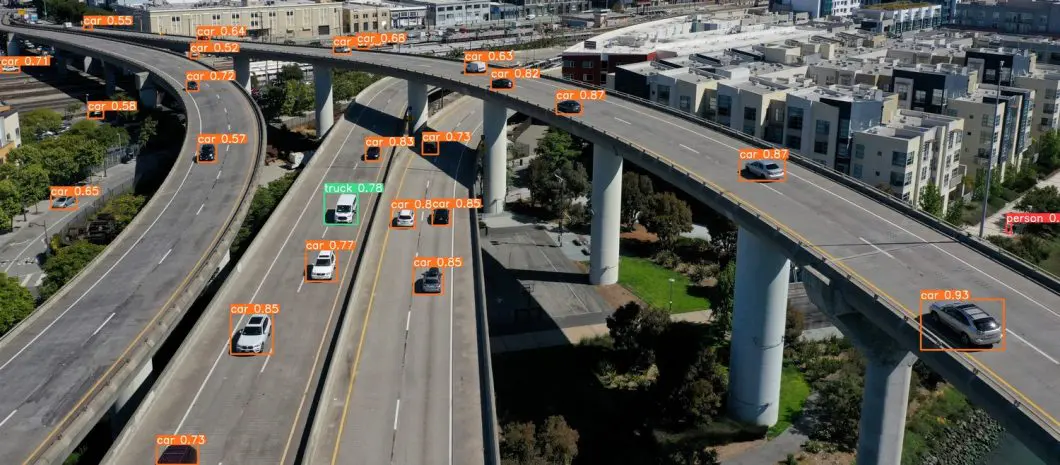
What is OpenVINO?
OpenVINO is a cross-platform deep learning toolkit developed by Intel. The name stands for “Open Visual Inference and Neural Network Optimization.” OpenVINO focuses on optimizing neural network inference with a write-once, deploy-anywhere approach for Intel hardware platforms. This also includes a post-training optimization tool.
The open-source toolkit is free for use under Apache License version 2.0 and has two versions:
- OpenVINO toolkit, supported by the open-source community
- Intel Distribution of OpenVINO toolkit, supported by Intel.
Using the OpenVINO toolkit, software developers can select models. This includes those in popular model formats like YOLOv3, ResNet 50, YOLOv8, etc. Then, you can deploy pre-trained models through a high-level C++ Inference Engine API with application logic.
OpenVINO offers integrated functionalities for expediting the development of applications and solutions that solve several tasks using computer vision. These include automatic speech recognition, natural language processing (NLP), recommendation systems, machine learning, and more.
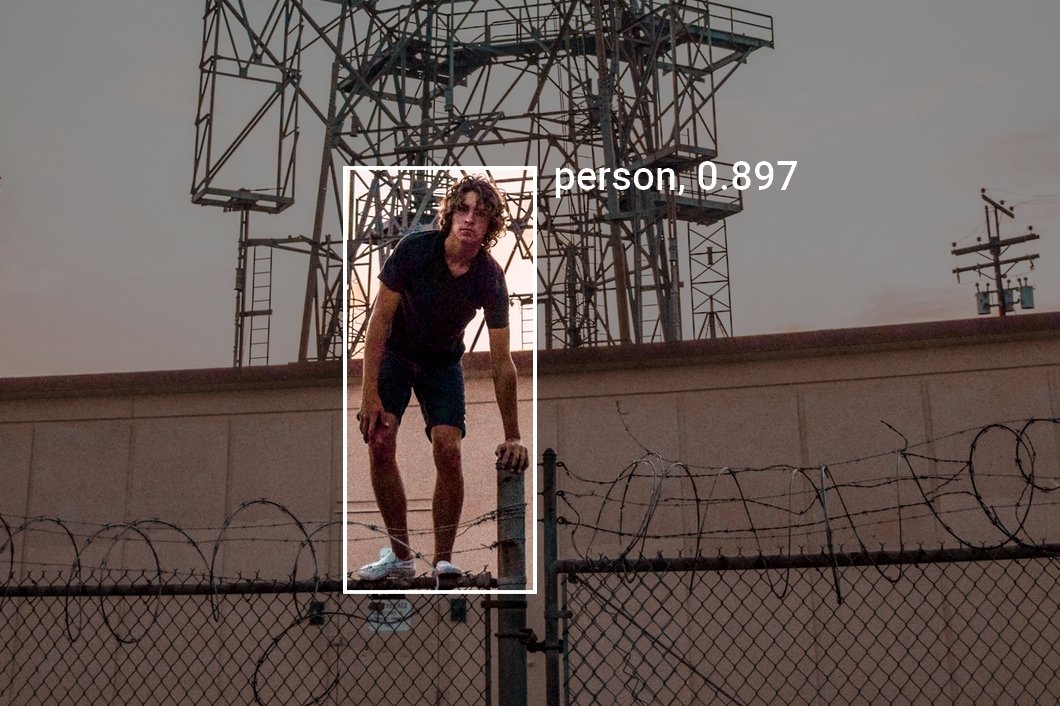
OpenVINO Platform for Enterprises
Viso Suite infrastructure leverages OpenVINO with a powerful pipeline and end-to-end features. Our platform helps large enterprises worldwide to build, deploy, and operate computer vision applications faster.
We partner with Intel to offer the integrated capabilities of OpenVINO as ready-made building blocks. In addition, Viso Suite provides everything around OpenVINO:
- Image annotation
- Model management
- Edge device management
- Automated deployments
- Zero-trust security
- Data privacy
- Full control over applications and data
To learn more, explore the features of Viso Suite or read the Whitepaper.
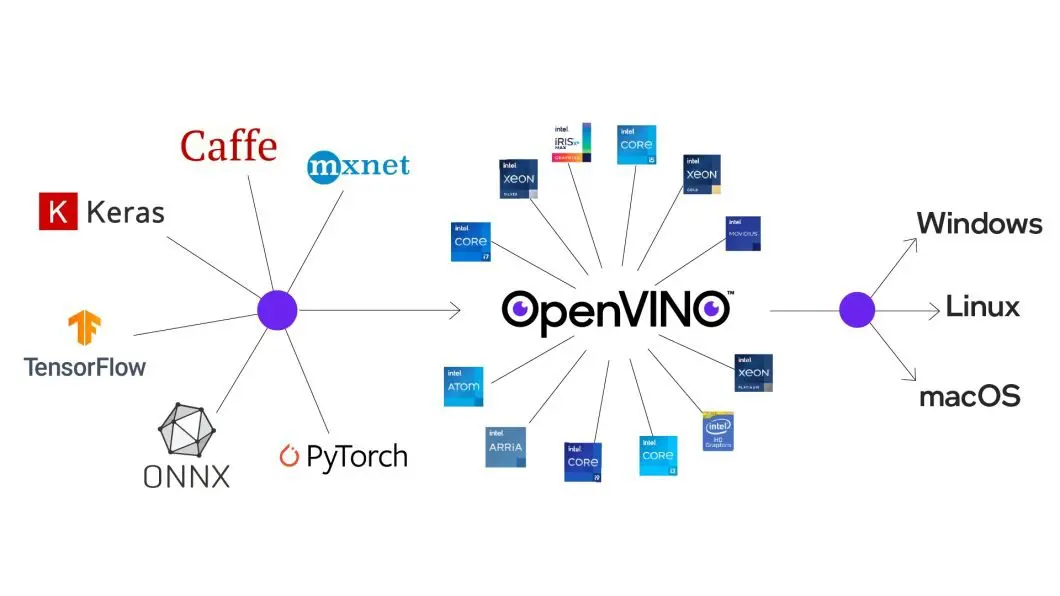
Why Use OpenVINO?
Deep Neural Networks (DNNs) advance in many industrial domains, leveling up the accuracy of computer vision algorithms. However, deploying and producing such accurate and useful models requires adaptations for the hardware and computational methods.
OpenVINO allows inference optimization of DNN models for process efficiency through the integration of various tools. This allows for reading models in popular formats, ensuring optimal performance, and reducing inference latency on Intel hardware.
Artificial Neural Networks (ANN) lay the groundwork for the OpenVINO toolkit. These ANNs include Convolutional Neural Networks (CNN) and recurrent and attention-based networks. To learn more about ANNs and their role in computer vision, we suggest reading our article on ANNs vs. CNNs.
The OpenVINO toolkit covers both computer vision and non-computer vision workloads across Intel hardware. It maximizes performance and accelerates application development. OpenVINO accelerates AI workloads and time-to-market with a library of predetermined functions and pre-optimized kernels. The OpenVINO toolkit includes other computer vision tools such as OpenCV, OpenCL kernels, and more.
The OpenVINO toolkit also provides a streamlined intermediate representation (IR) for efficient optimization and deployment of deep learning models across diverse hardware platforms.
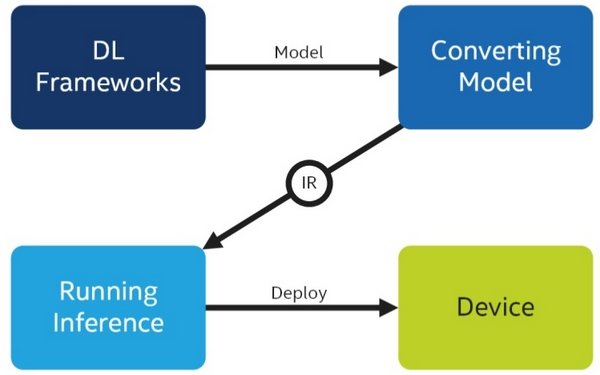
What Are the Benefits?
- Accelerate Performance: by enabling simple execution methods on Intel processors and accelerators. I.e. CPU, GPU/Intel Processor Graphics, VPU (Intel AI Stick NCS2 with Myriad X), and FPGA.
- Streamline Deployment: with CNN-based functions using one common API and 30+ pre-trained models and documented code samples. 100+ public and custom models streamline innovation with a centralized method for deep learning model implementation.
- Extend and Customize: with OpenCL (Open Computing Language) Kernels and other tools offer an open, royalty-free standard way. This allows for adding custom code pieces straight into the workload pipeline, customizing deep learning model layers without the burden of framework overheads, and implementing parallel programming of various accelerators.
- Innovate Artificial Intelligence: with the complete Deep Learning Deployment Toolkit within OpenVINO. It allows users to extend private applications and optimize AI “all the way to the cloud.” Model Optimizer, Intermediate Representation, nGraph Integration, and more support this.
- Full Viso Suite Integration (End-To-End): OpenVINO fully integrates with Viso Suite infrastructure. Viso Suite provides pre-built modules to fetch the video feed of any digital camera with multi-camera support. Visual programming with logic workflows allows fast building and updating of computer vision applications for deployment to edge devices.
What to Use the OpenVINO Toolkit For
The toolkit can
- Deploy computer vision inference on various hardware (more below)
- Import and optimize models from various frameworks such as PyTorch, TensorFlow, etc.
(Post-training to accelerate inference) - Run deep learning models outside of computer vision
- Perform “traditional” computer vision tasks (such as background subtraction)
The toolkit can not
- Training a machine learning model (although there are Training Extensions)
- Run “traditional” machine learning outside of computer vision
(such as Support Vector Machine), check out OpenCV - Interpret the output of the model
How OpenVINO Works on a High-Level
The OpenVINO workflow primarily consists of four main steps:
- Train: A model learns with code.
- Model Optimizer: model quantization, freezing, or fusion to generate an Intermediate Representation (.xml + .bin files). We configure pre-trained models according to the framework and convert them to a simple, single-line command. You can choose from pre-trained models in the OpenVINO Model Zoo, containing options for various applications.
- Inference Engine: we feed the Intermediate Representation, along with input data, to the Inference Engine. It checks for model compatibility based on the model training framework and hardware (otherwise known as the environment). Frameworks supported by OpenVINO include TensorFlow, TensorFlow Lite, Caffe, MXNet, ONNX (PyTorch, Apple ML), and Kaldi.
- Deployment: we deploy the application, along with the optimized model and input data, to devices. For enterprise-grade solutions, Viso Suite provides complete device management for automated and robust deployment at scale.
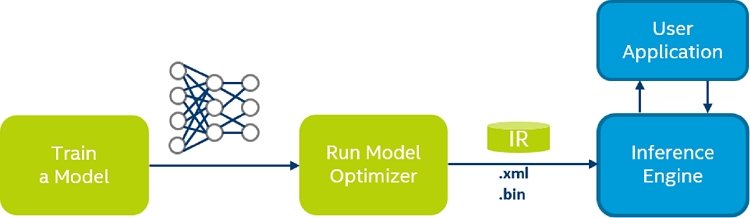
The Most Important Features
OpenVINO includes a variety of features specific to the toolkit. We will cover a few of the most notable ones, but these are just a portion of those offered by the toolkit.
Multi-Device Execution
Intel processors include strong x86 cores powered by integrated graphics and prime hardware for computation offload. Examples of computation offload include when integrated graphics allow users to move computations to the Intel-integrated CPU. This is already built-in while using the CPU processor for small, interactive, or low-latency functions.
AI workloads can take advantage of these computation offload functions by using tools OpenVINO toolkit offerings. Intel Open VINO toolkit runtime performs inference tasks on integrated graphics like any other supported target (like CPU).
One of the signature features of the OpenVINO™ toolkit is “multi-device” execution. Multi-device compatibility allows developers to run inference on several “compute devices” transparently on one system. This methodology enables computer vision creators to maximize inferencing performance.
The multi-device plugin further allows users to take full advantage of combining hardware and software fixtures. Multi-device mode uses the available CPU and integrated GPU for complete system utilization.
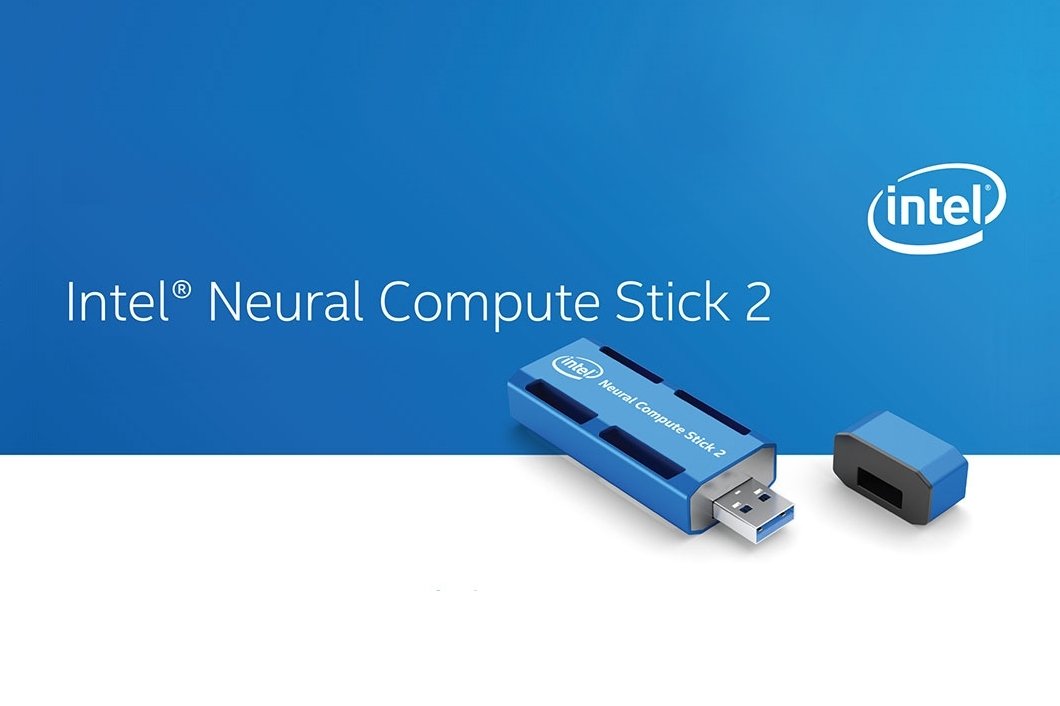
Application Footprint Reduction
Tools within the OpenVINO toolkit, i.e. Deployment Manager, allow users to quickly and easily reduce application footprint. The application footprint refers to the space and latency an application takes up in the user’s computing device. Therefore, reducing the application footprint was an important objective during the OpenVINO development process.
Depending on the use case, inferencing can require a substantial amount of memory to execute. To reduce this, the OpenVINO toolkit released further updates. They include Custom Compiled Runtimes, Floating-point Model Representation, and decreased model sizes within pre-included libraries.
While the OpenVINO toolkit contains all necessary components verified on target platforms, users can also create custom runtime libraries. The open-sourced version allows users to compile runtime with modifications reducing the size. I.e. Enable Link Time Optimizations (ENABLE_LTO).
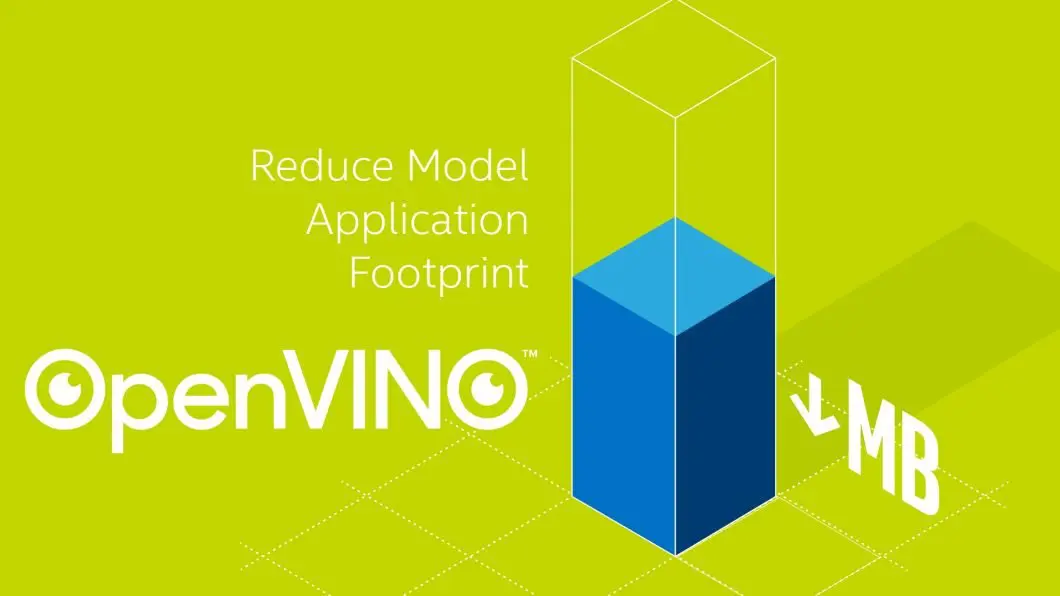
OpenVINO Training Add-On – NNCF
The toolkit download does not include 100% of user-built add-ons for OpenVINO. There are OpenVINO add-ons available for specific tasks and purposes. For example, there is a specific component for fine-tuning the accuracy of new deep learning models. Other pre-downloaded techniques do not allow for the desired accuracy.
This component is the Neural Network Compression Framework (NNCF). The supported optimization techniques and models in this add-on come directly from the OpenVINO toolkit.
Features of NNCF Add-on for the OpenVINO Toolkit
- Automatic Model Transformation: Using the optimization method ensures that there is no necessary user-implemented modification for transforming the deep learning model at hand.
- Unified API: This refers to the unified API (Application Programming Interface) for methods of optimization. Specific abstractions introduced within the framework are the basis of all compression methods.
- Algorithm Combination: allows for the application of several algorithms simultaneously. Thus, enabling the production of one optimized model per fine-tuning stage.
I.e. algorithms performing optimization for sparsity and lower precision are deployable at once by combining two separate algorithms in the pipeline. - Distributed Training Support: we can organize the deep learning model fine-tuning on the multi-node distributed cluster.
- Uniform configuration: optimization methods are configurable in a standardized way through the use of the JSON configuration file. A JSON configuration file provides a simpler setup of the compression parameters for the deep learning model.
- ONNX Exportation: is the open standard for machine learning interoperability. This is an informal standard for NN representation. Such optimized models are convertible to OpenVINO Intermediate Representation, discussed in the previous section of this article.
A novel advantage of the NNCF framework add-on is automatic model transformation when applying optimization methods. OpenVINO computer vision engineers Alexander Kozlov, Yury Gorbachev, and others created this specific add-on.
OpenVINO Model Zoo
The OpenVINO model zoo contains a variety of pre-trained deep-learning models optimized for Intel hardware. The model zoo offers developers a centralized model cache allowing for the accelerated development of intelligent applications. The zoo contains pre-trained models for computer vision tasks including image classification, object detection, and image segmentation.
The OpenVINO model zoo documentation provides thorough demos and step-by-step procedures for building and deploying AI applications.
OpenVINO Industry Use Cases and Advantages
The OpenVINO toolkit facilitates the development, creation, and deployment of high-performance inference applications across widely-used Intel platforms.
Uses range from security surveillance to smart cities, and more. To see more examples and find computer vision ideas, explore our extensive List of Computer Vision Applications. We introduce enterprise-grade computer vision solutions to build and operate using OpenVINO with Viso Suite.
We support multiple case studies on how AI-powered by the OpenVINO toolkit solves real-world problems.
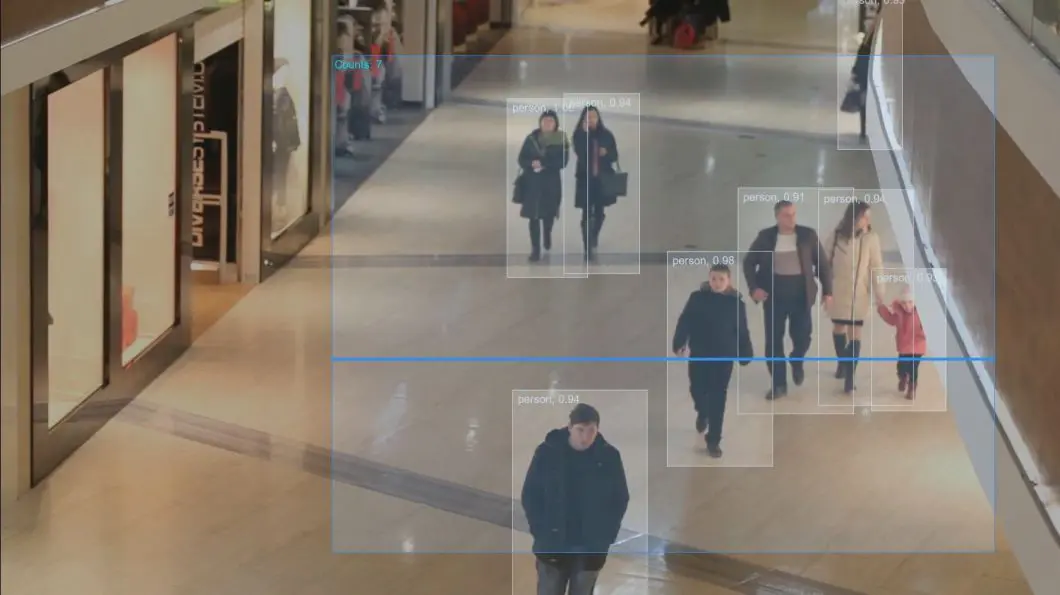
The toolkit is useful for a multitude of purposes, from solutions for industrial manufacturing to city-wide transportation. Some specific examples include:
Workplace Hazards and Personal Protective Equipment Detection
Businesses use Viso Suite to develop PPE recognition applications to protect against workplace hazards and ensure safety compliance. The Viso Suite platform utilizes Open VINO to extend deep learning capabilities and inference to the edge.
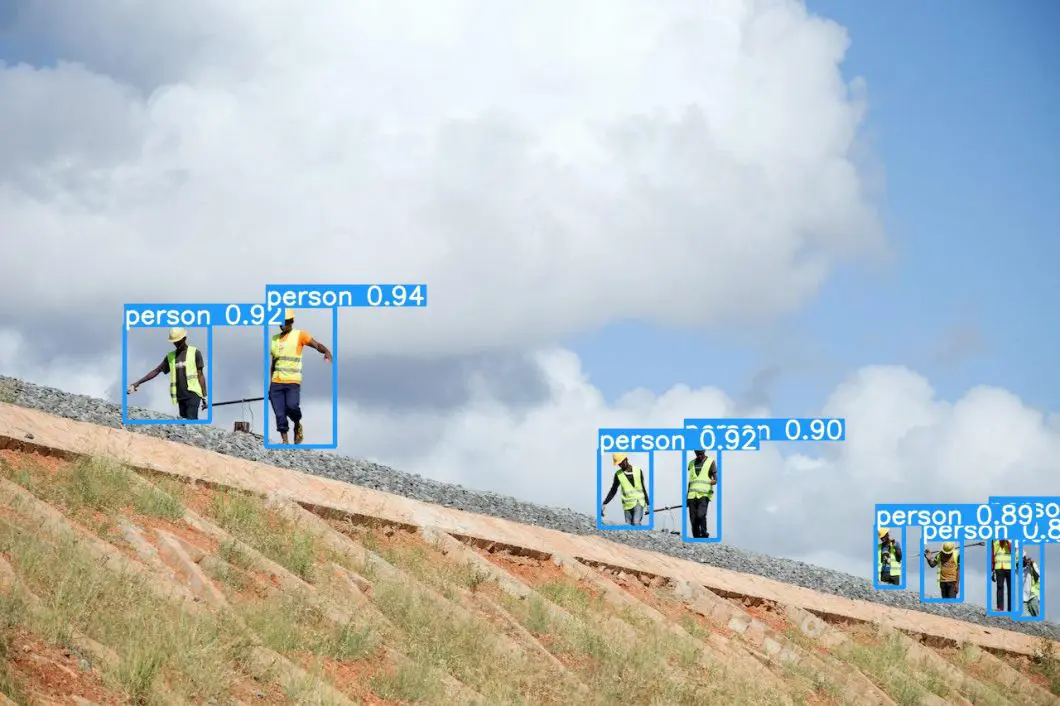
The PPE recognition application captures video footage from workspace surveillance, such as CCTV cameras. It uses the footage to identify whether employees are wearing the required PPE for the task at hand.
In case of a violation, the application can disperse information to company staff. We can set up these alerts as integrated notifications, alerting, and logging features. Thus, allowing employees to take prompt action to address the safety hazard.
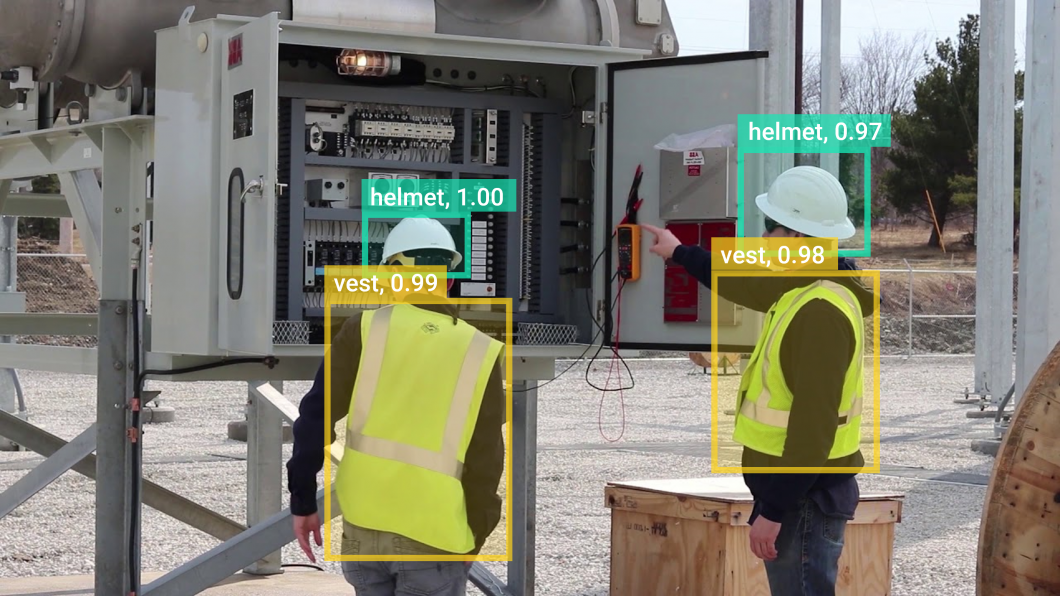
Manufacturing Defect Detection
Using Viso Suite, manufacturing companies can build and deliver custom AI inspection and defect detection applications. In heavy machinery manufacturing, deep learning applications can detect porosity or defects in castings or welded parts.
Therefore, computer vision pipelines use algorithms to analyze video frames and detect quality issues automatically. Such real-time AI image analysis methods can identify defects not visible to the human eye.
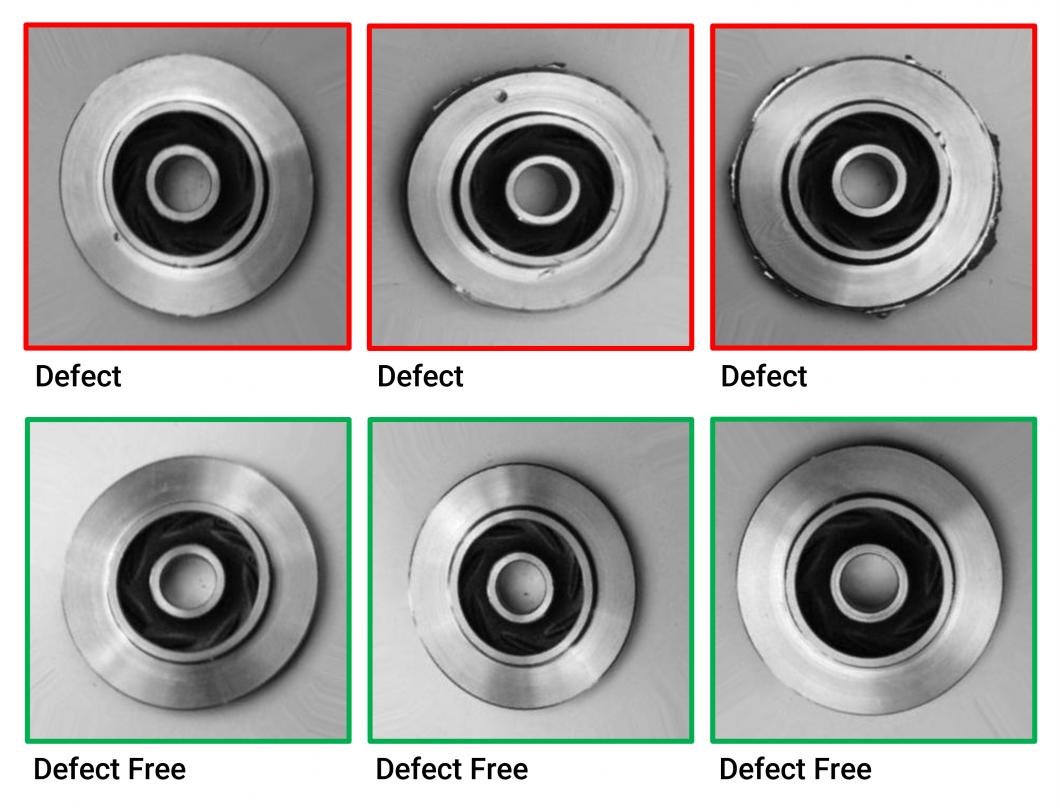
Industrial manufacturers use Viso Suite for automated defect detection solutions. This helps ensure consistent quality control while reducing product defect risk. Through high-performance computer vision, manufacturers can improve their production efficiency, product quality, customer satisfaction, and operational safety.
Sports Mega Event Security
A major tennis event used Viso Suite Infrastructure to construct an integrated video surveillance system. It meets the AI video monitoring needs across diverse sites, with mega crowds of more than 1 million visitors.
To safeguard players, spectators, venues, and equipment, the organizers deployed AI video surveillance across dozens of cameras and sensors. The OpenVINO toolkit implemented multiple AI vision applications for person detection, crowd counting, suspicious object recognition, parking, and traffic analysis. This is because it supports the delivery of deep learning software and inferencing for video analytics at the edge.
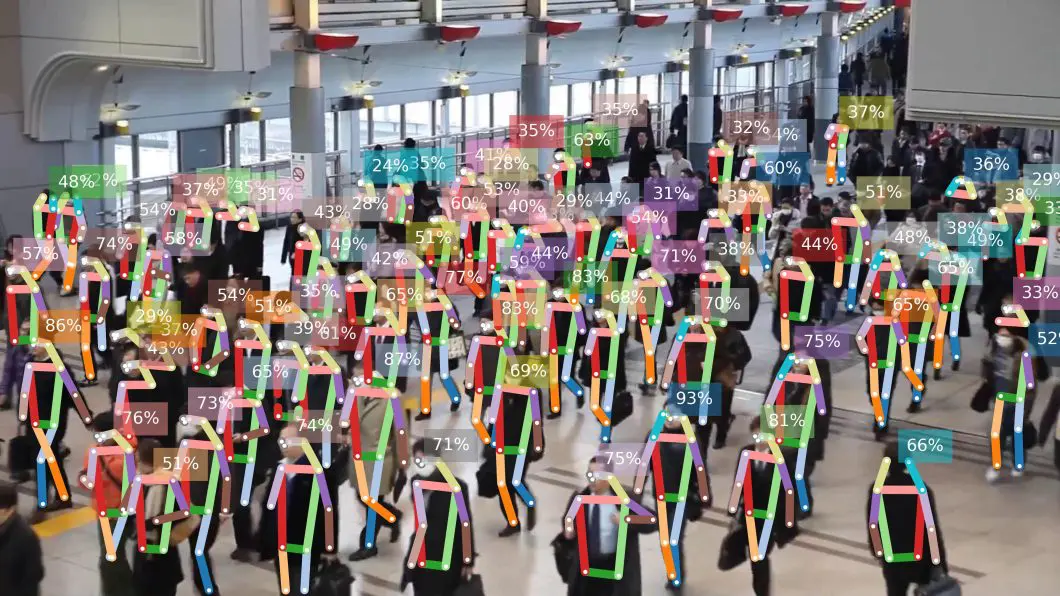
Restaurant Industry Analytics With OpenVINO
Viso Suite is deployable in the restaurant industry for comprehensive video surveillance. With OpenVINO toolkit integration, restaurants can utilize video analytics at the edge for enhanced safety, security, and operational efficiency. Viso Suite makes it possible to build several AI vision applications, including:
- Facial recognition for staff access control
- Occupancy monitoring to adhere to social distancing guidelines
- Queue management to improve customer flow and service delivery
Additionally, OpenVINO and Viso Suite enable real-time analysis of video feeds for various applications. These include table occupancy detection, wait time estimation, and food quality monitoring. With AI-driven video analytics, operators can:
- Make data-driven decisions to enhance customer experiences
- Improve operational workflows
- Ensure compliance with health and safety regulations
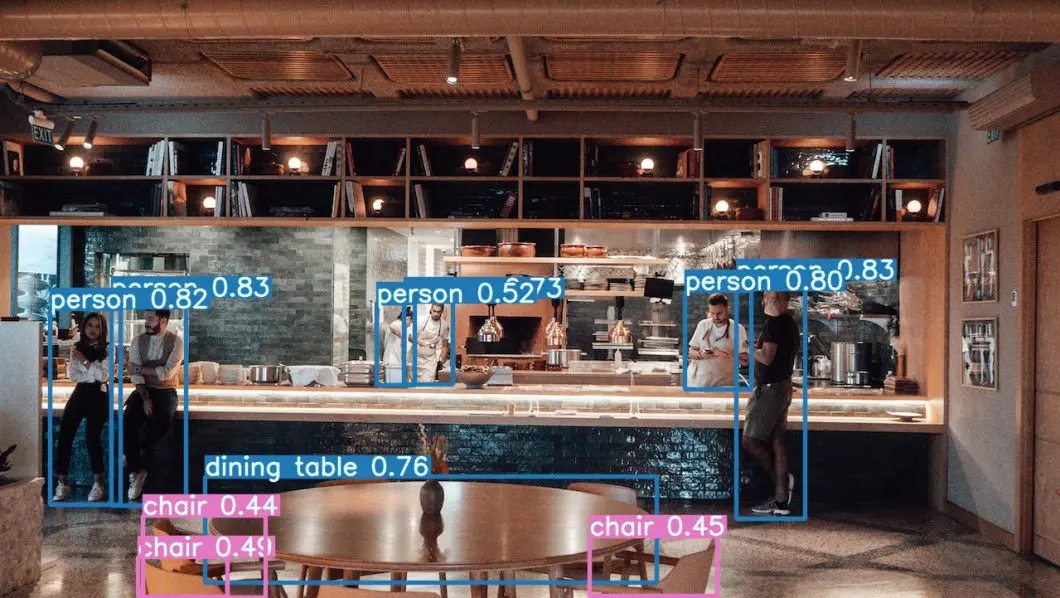
OpenVINO General Information
Download OpenVINO
We can download the OpenVINO toolkit after filling out the form on this landing page: here. For help downloading, visit the OpenVINO download documentation, a PDF that is downloadable directly onto your computer.
The OpenCL 3.0 Finalized Specification is usable as part of the OpenVINO toolkit. The complete documentation site is available here: OpenVINO Toolkit Overview.
OpenVINO 2022.1 introduces a new version of OpenVINO API (API 2.0). Legacy API versions of OpenVINO before 2022.1 require changes in the app logic. This is when migrating an application from other frameworks, such as TensorFlow, PyTorch models, ONNX Runtime, and PaddlePaddle.
OpenVINO 2022
OpenVINO 2022 is a comprehensive toolkit for developing applications and solutions based on deep learning tasks. It provides high-performance and rich deployment options from edge to cloud. It enables CNN-based and transformer-based deep learning inference on the edge or cloud. Additionally, it supports various execution modes across Intel technologies.
The installation package of OpenVINO 2022 includes two parts:
- OpenVINO Runtime (the core set of libraries to run ML tasks)
- OpenVINO Development Tools (set of utilities for working with OpenVINO models)
We can install the package via different methods depending on the user’s needs. New features and enhancements include improved performance and compatibility with more frameworks.
Find tutorials and demos here to help users start with OpenVINO.
OpenVino 2024 Updates
The OpenVINO toolkit’s version 2024.0 release makes generative AI more accessible. It does this by improving large language model (LLM) performance and expanding model coverage. OpenVINO now supports Mixture of Experts (MoE), a new architecture for processing more effective generative models via the pipeline. It also improves portability and performance for deployment anywhere, including the edge, the cloud, and locally.
Build a Large-Scale Computer Vision Solution with OpenVINO
At viso.ai, we are partners of Intel, the developer of OpenVINO. We power Viso Suite, an end-to-end computer vision infrastructure providing out-of-the-box Open VINO capabilities. As an all-in-one solution, Viso Suite provides everything around OpenVINO. This includes development, deployment, monitoring, scaling, and securing.
This includes the integration of any digital camera (surveillance cameras, CCTV cameras, webcams). Viso Suite offers a robust infrastructure for enterprises to deliver secure, robust, and scalable Edge AI vision systems:
- Viso Suite provides an all-in-one solution to build, deploy, and monitor computer vision systems.
- Use visual programming and an automated infrastructure to deliver computer vision 10x faster.
- To avoid integration hassles and writing code from scratch; please refer to solutions.
Starting with CV: Get in touch with our team of AI experts and request a demo for your organization.
Thanks for reading this overview of the OpenVINO toolkit. For more information about computer vision: