This article will provide an introduction to object detection and provide an overview of the state-of-the-art computer vision object detection algorithms. Object detection is a key field in artificial intelligence, allowing computer systems to “see” their environments by detecting objects in visual images or videos.
In particular, you will learn about:
- What object detection is and how it has evolved over the past 20 years
- Types of computer vision object detection methods
- We list examples, use cases, and object detection applications
- The most popular object detection algorithms today
- New deep-learning object recognition algorithms
About: At viso.ai, we provide the end-to-end computer vision platform Viso Suite. The platform enables teams to build and deliver all their real-world computer vision applications in one place. Get the whitepaper and a demo for your company.
What is Deep Learning Object Detection?
Object detection is an important computer vision task used to detect instances of visual objects of certain classes (for example, humans, animals, cars, or buildings) in digital images such as photos or video frames. The goal of object detection is to develop computational models that provide the most fundamental information needed by computer vision applications: “What objects are where?”.
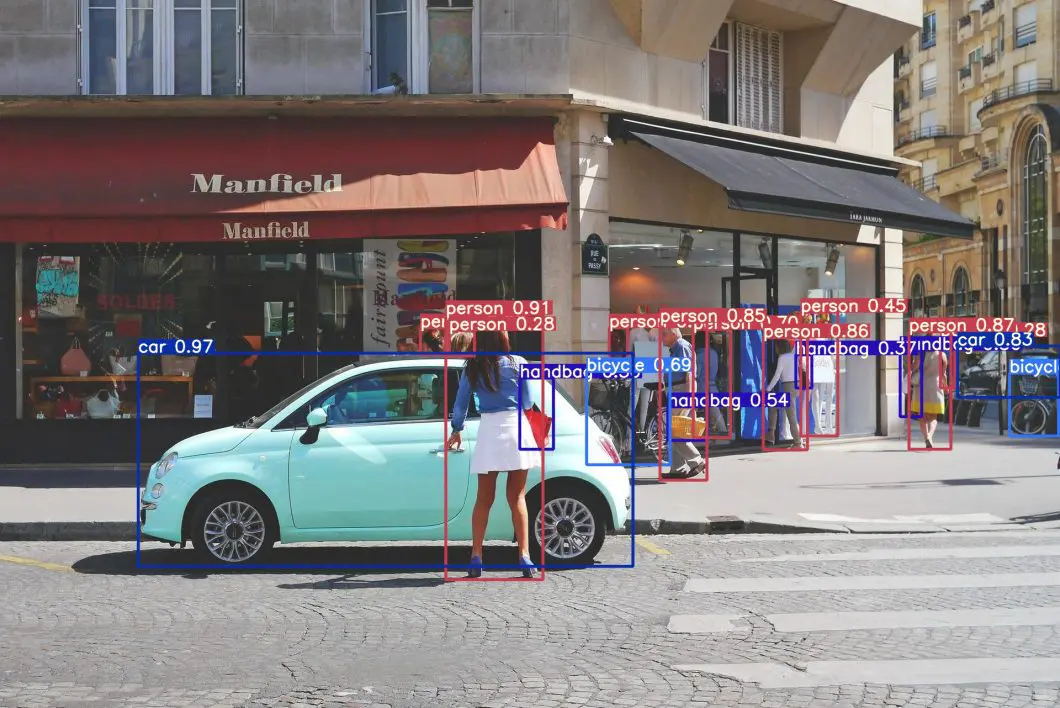
Person Detection
Person detection is a variant of object detection used to detect a primary class “person” in images or video frames. Detecting people in video streams is an important task in modern video surveillance systems. The recent deep learning algorithms provide robust person detection results. Most modern person detector techniques are trained on frontal and asymmetric views.
However, deep learning models such as YOLO that are trained for person detection on a frontal view data set still provide good results when applied for overhead view person counting (TPR of 95%, FPR up to 0.2%). See how companies use Viso Suite to build a custom people counting solution with deep learning for video analysis.
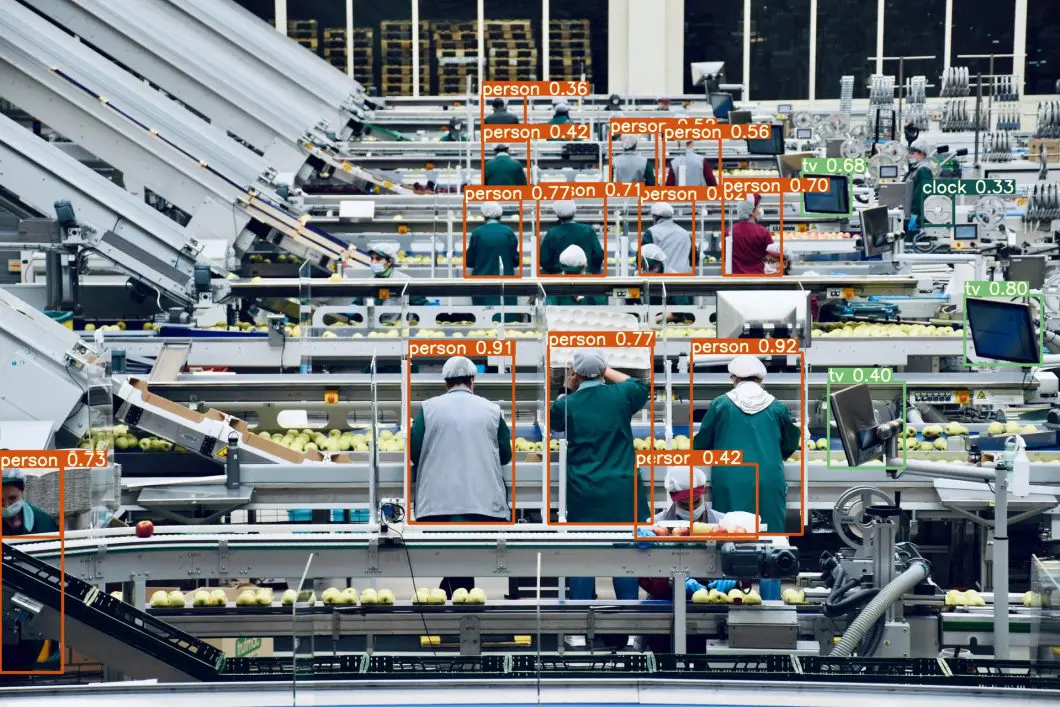
Why is Object Detection Deep Learning Important?
Object detection is one of the fundamental problems of computer vision. It forms the basis of many other downstream computer vision tasks, for example, instance and image segmentation, image captioning, object tracking, and more. Specific object detection applications include pedestrian detection, animal detection, vehicle detection, people counting, face detection, text detection, pose estimation, or number-plate recognition.
As computer vision matures, it has transitioned from the “Slope of Enlightenment” toward the “Plateau of Productivity” on the Gartner Hype Cycle. With advancements in technology, increased adoption, and practical applications across industries, computer vision, including object detection, is entering a phase of stability and widespread integration. The focus now shifts from experimental stages to refining and optimizing existing applications, marking a crucial step toward its full realization and impact on enterprises in various sectors.
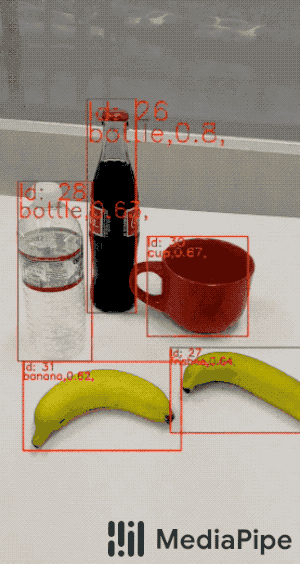
Objects Detection and Deep Learning
In the last few years, the rapid advances in deep learning techniques have greatly accelerated the momentum of object detection technology. With deep learning object detection networks and the computing power of GPUs, the performance of object detectors and trackers has greatly improved, achieving significant breakthroughs in object detection.
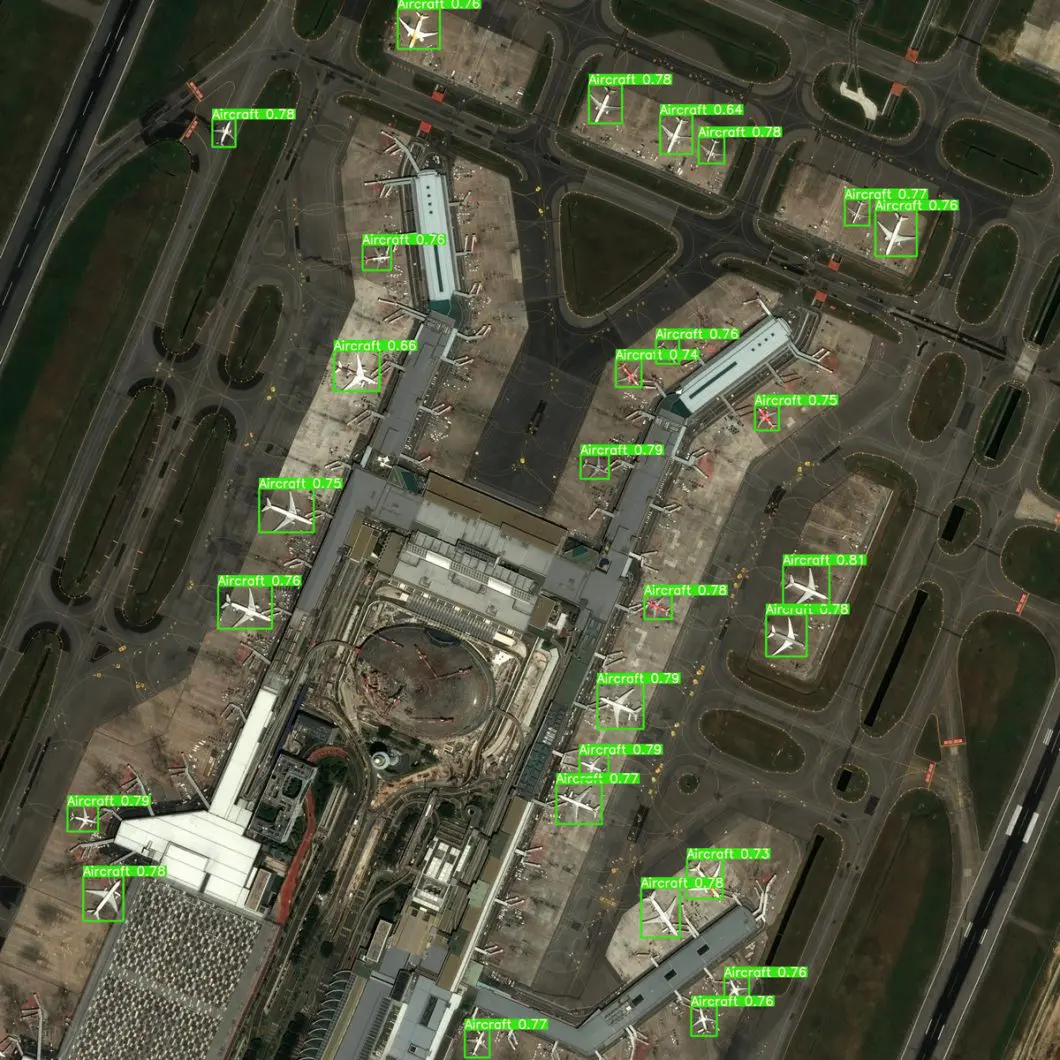
Machine learning (ML) is a branch of artificial intelligence (AI), and it essentially involves learning patterns from examples or sample data as the machine accesses the data and can learn from it (supervised learning on annotated images).
Deep Learning is a specialized form of machine learning which involves learning in different stages. To learn more about the technological background, check out our article: What’s the difference between Machine Learning and Deep Learning?
Computer Vision Software Advances
Deep Learning object detection and tracking are the fundamental basis of a wide range of modern computer vision applications. For example, the detection of objects enables intelligent healthcare monitoring, autonomous driving, smart video surveillance, anomaly detection, robot vision, and much more. Each AI vision application usually requires a combination of different algorithms that form a flow (pipeline) of multiple processing steps.
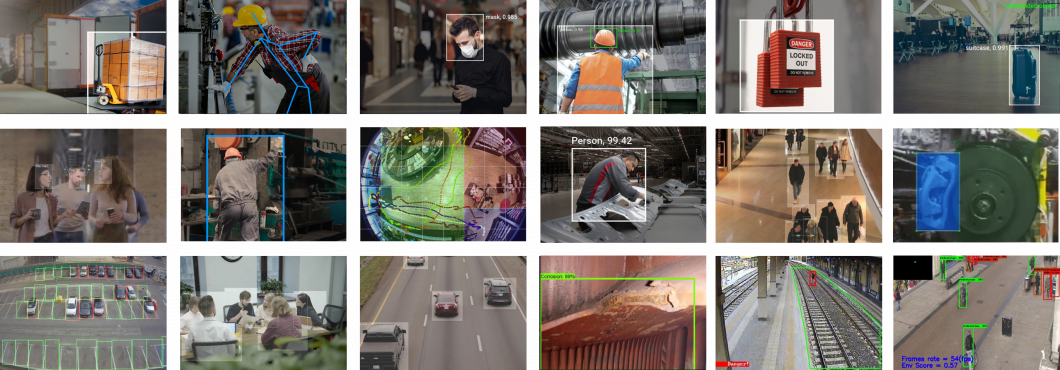
AI imaging technology has greatly progressed in recent years. A wide range of cameras can be used, including commercial security and CCTV cameras. By using a cross-compatible AI software platform like Viso Suite, there is no need to buy AI cameras with built-in image recognition capabilities, because the digital video stream of essentially any video camera can be analyzed using object detection models.
As a result, applications become more flexible as they no longer depend on custom sensors, expensive installation, and embedded hardware systems that must be replaced every 3-5 years.
Computer Vision Hardware Advances
Meanwhile, computing power has dramatically increased and is becoming much more efficient. In past years, computing platforms moved toward parallelization through multi-core processing, graphical processing units (GPU), and AI accelerators such as tensor processing units (TPU)
Such hardware allows applying computer vision for object detection and tracking in near real-time environments. Hence, rapid development in deep convolutional neural networks (CNN) and GPU’s enhanced computing power are the main drivers behind the great advancement of computer vision-based object detection.
Those advances enabled a key architectural concept called Edge AI. This concept is also called Intelligent Edge or Distributed Edge. It moves heavy AI workloads from the Cloud closer to the data source. This results in distributed, scalable, and much more efficient systems that allow the use of computer vision in business and mission-critical systems.
Edge AI involves IoT or AIoT, on-device machine learning with Edge Devices, and requires complex infrastructure. At viso.ai, we enable organizations to build, deploy, and scale their object detection applications while taking advantage of all those cutting-edge technologies. You can get the Whitepaper here.
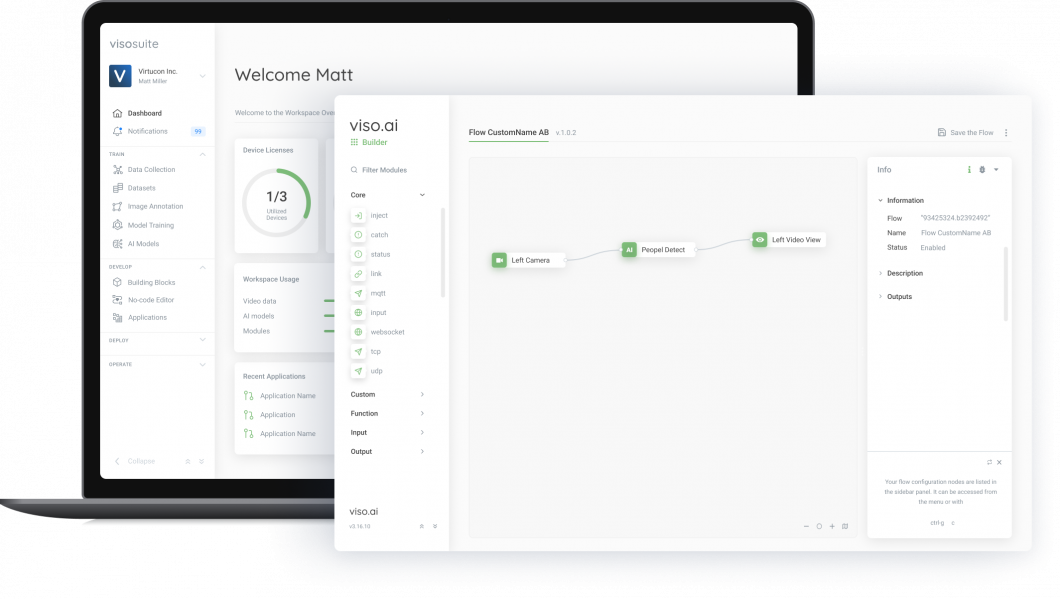
Disadvantages and Advantages of Object Detection
Object detectors are incredibly flexible and can be trained for a wide range of tasks and custom, special-purpose applications. The automatic identification of objects, persons, and scenes can provide useful information to automate tasks (counting, inspection, verification, etc.) across the value chains of businesses.
However, the main disadvantage of object detectors is that they are computationally very expensive and require significant processing power. Especially, when object detection models are deployed at scale, the operating costs can quickly increase and challenge the economic viability of business use cases. Learn more in our related article What Does Computer Vision Cost?
Deep Learning Object Recognition vs. Object Detection
While similar, object detection and object recognition are two different computer vision tasks. Object recognition, also referred to as image classification, involves identifying the class of an object found in an image. Unlike outright object detection, object recognition does not provide localization information.
Object recognition algorithms output class labels that indicate objects found in the image. It is commonly used for applications like image tagging, content-based image retrieval, and visual search engines.
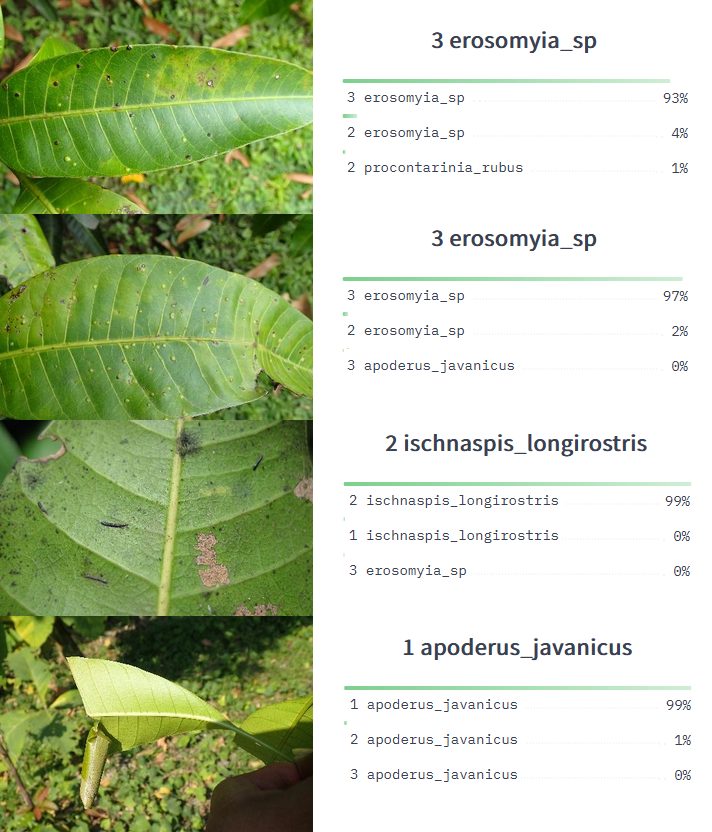
How Deep Learning Object Detection Algorithm Works
Object detection can be performed using either traditional (1) image processing techniques or modern (2) deep learning networks.
- Image processing techniques generally don’t require historical data for training and are unsupervised. OpenCV is a popular tool for image processing tasks.
- Pros: Hence, those tasks do not require annotated images, which humans labeled data manually (for supervised training).
- Cons: These techniques are restricted to multiple factors, such as complex scenarios (without unicolor background), occlusion (partially hidden objects), illumination and shadows, and clutter effect.
- Deep Learning methods generally depend on supervised or unsupervised learning, with supervised methods being the standard in computer vision tasks. The performance is limited by the computation power of GPUs, which is rapidly increasing year by year.
- Pros: Deep learning object detection is significantly more robust to occlusion, complex scenes, and challenging illumination.
- Cons: A huge amount of training data is required; the process of image annotation is labor-intensive and expensive. For example, labeling 500’000 images to train a custom DL object detection algorithm is considered a small dataset. However, many benchmark datasets (MS COCO, Caltech, KITTI, PASCAL VOC, V5) provide the availability of labeled data.
Today, deep learning object detection is widely accepted by researchers and adopted by computer vision companies to build commercial products.
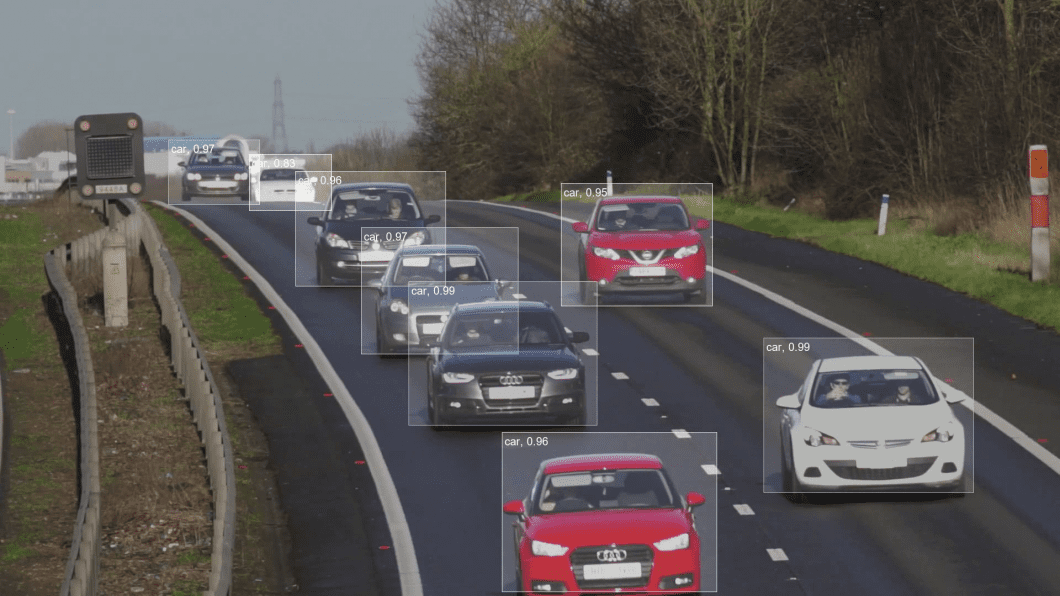
The Best Image Detection Algorithm Today
The field of object detection is not as new as it may seem. In fact, object detection has evolved over the past 20 years. The progress of object detection is usually separated into two separate historical periods (before and after the introduction of Deep Learning):
Object Detector Before 2014 – Traditional Object Detection period
- Viola-Jones Detector (2001), the pioneering work that started the development of traditional object detection methods
- HOG Detector (2006), a popular feature descriptor for object detection in computer vision and image processing
- DPM (2008) with the first introduction of bounding box regression
Object Detector After 2014 – Deep Learning Detection period
The most important two-stage object detection algorithms
- RCNN and SPPNet (2014)
- Fast RCNN and Faster RCNN (2015)
- Mask R-CNN (2017)
- Pyramid Networks/FPN (2017)
- G-RCNN (2021)
The most important one-stage object detection algorithms
- YOLO (2016)
- SSD (2016)
- RetinaNet (2017)
- YOLOv3 (2018)
- YOLOv4 (2020)
- YOLOR (2021)
- YOLOv7 (2022)
- YOLOv8 (2023)
- YOLOv9 (2024)
The creators of the original YOLO algorithms did not release YOLOv8. It’s important to note that it was published under an AGPL-3.0 License, a strong copyleft license that limits commercial use.
It is important to understand the main characteristics to understand which model is best for a given use case. First, we will look into the key differences between the relevant image recognition models for object detection before discussing the individual models.
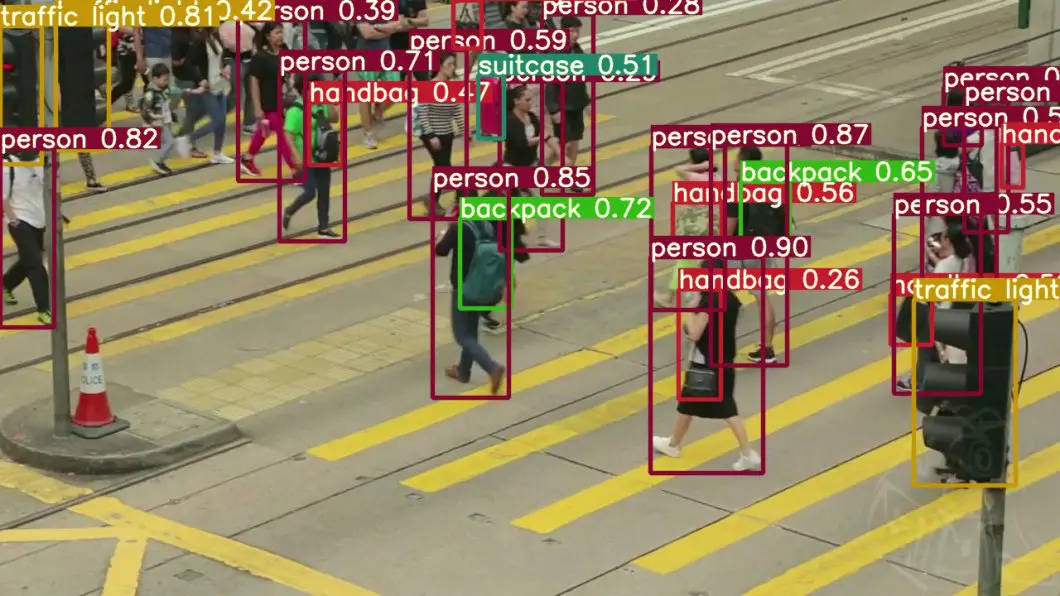
One-Stage vs. Two-Stage Deep Learning Object Detection
As you can see in the list above, state-of-the-art object detection methods can be categorized into two main types: One-stage vs. two-stage object detectors.
In general, deep learning-based object detectors extract features from the input image or video frame. An object detector solves two subsequent tasks:
- Task #1: Find an arbitrary number of objects (possibly even zero), and
- Task #2: Classify every single object and estimate its size with a bounding box.
To simplify the process, you can separate those tasks into two stages. Other methods combine both tasks into one step (single-stage detectors) to achieve higher performance at the cost of accuracy.
Two-stage Detectors
In two-stage object detectors, the approximate object regions are proposed using deep features before these features are used for the image classification and bounding box regression for the object candidate.
- The two-stage architecture involves (1) object region proposal with conventional Computer Vision methods or deep networks, followed by (2) object classification based on features extracted from the proposed region with bounding-box regression.
- Two-stage methods achieve the highest detection accuracy but are typically slower. Because of the many inference steps per image, the performance (frames per second) is not as good as one-stage detectors.
- Various two-stage detectors include region convolutional neural network (RCNN), with evolutions Faster R-CNN or Mask R-CNN. The latest evolution is the granulated RCNN (G-RCNN).
- Two-stage object detectors first find a region of interest and use this cropped region for classification. However, such multi-stage detectors are usually not end-to-end trainable because cropping is a non-differentiable operation.
One-stage Detectors
One-stage detectors predict bounding boxes over the images without the region proposal step. This process consumes less time and can therefore be used in real-time applications.
- One-stage object detectors prioritize inference speed and are super fast but not as good at recognizing irregularly shaped objects or a group of small objects.
- The most popular one-stage detectors include the YOLO, SSD, and RetinaNet. The latest real-time detectors are YOLOv7 (2022), YOLOR (2021), and YOLOv4-Scaled (2020). View the benchmark comparisons below.
- The main advantages of object detection with single-stage algorithms include a generally faster detection speed and greater structural simplicity and efficiency compared to multi-stage detectors.
How to Compare Image Detection Algorithms
The most popular benchmark is the Microsoft COCO dataset. Different models are typically evaluated according to a Mean Average Precision (MAP) metric. In the following, we will compare the best real-time object detection algorithms.
It’s important to note that the algorithm selection depends on the use case and application; different algorithms excel at different tasks (e.g., Beta R-CNN shows the best results for Pedestrian Detection).
The Best Real-Time Object Detection Algorithm (Accuracy)
On the MS COCO dataset and based on the Average Precision (AP), the best real-time object detection algorithm is YOLOv7, followed by Vision Transformer (ViT) such as Swin and DualSwin, PP-YOLOE, YOLOR, YOLOv4, and EfficientDet.
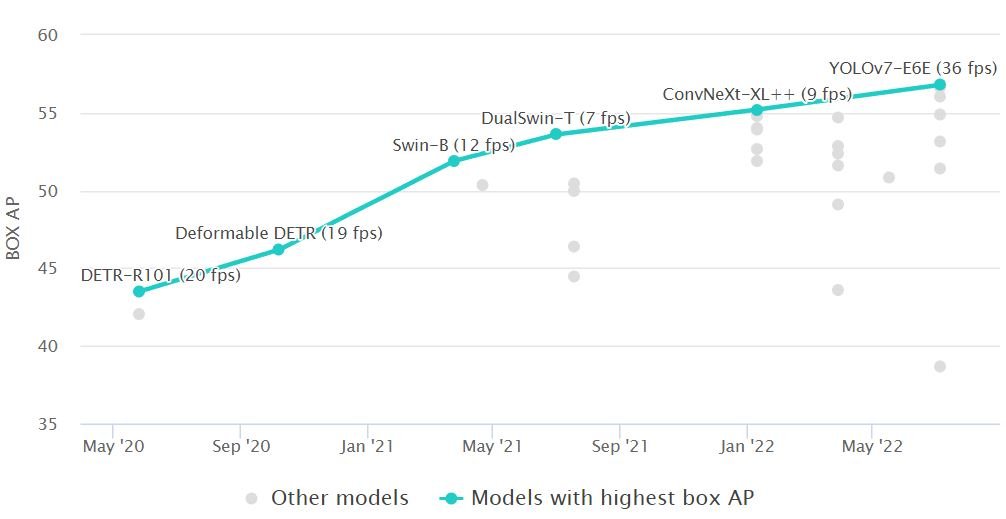
The Fastest Real-Time Object Detection Algorithm (Inference Time)
Also, on the MS COCO dataset, an important benchmark metric is inference time (ms/Frame, lower is better) or Frames per Second (FPS, higher is better). The rapid advances in computer vision technology are very visible when looking at inference time comparisons.
Based on current inference times (lower is better), YOLOv7 achieves 3.5ms per frame, compared to YOLOv4 12ms, or the popular YOLOv3 29ms. Note how the introduction of YOLO (one-stage detector) led to dramatically faster inference times compared to any previously established methods, such as the two-stage method Mask R-CNN (333ms).
On a technical level, it is pretty complex to compare different architectures and model versions in a meaningful way. Edge AI is becoming an integral part of scalable AI solutions, and newer models come with lighter-weight edge-optimized versions (see YOLOv7-lite or TensorFlow Lite).
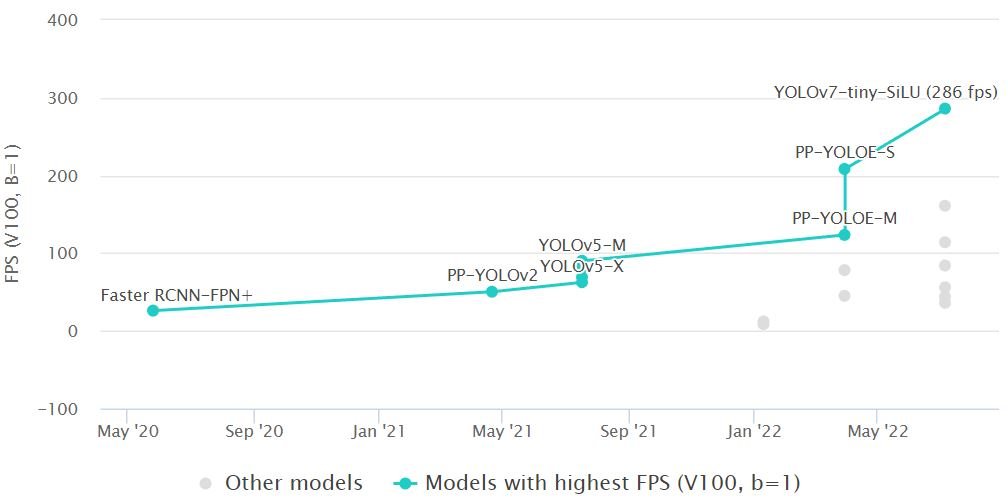
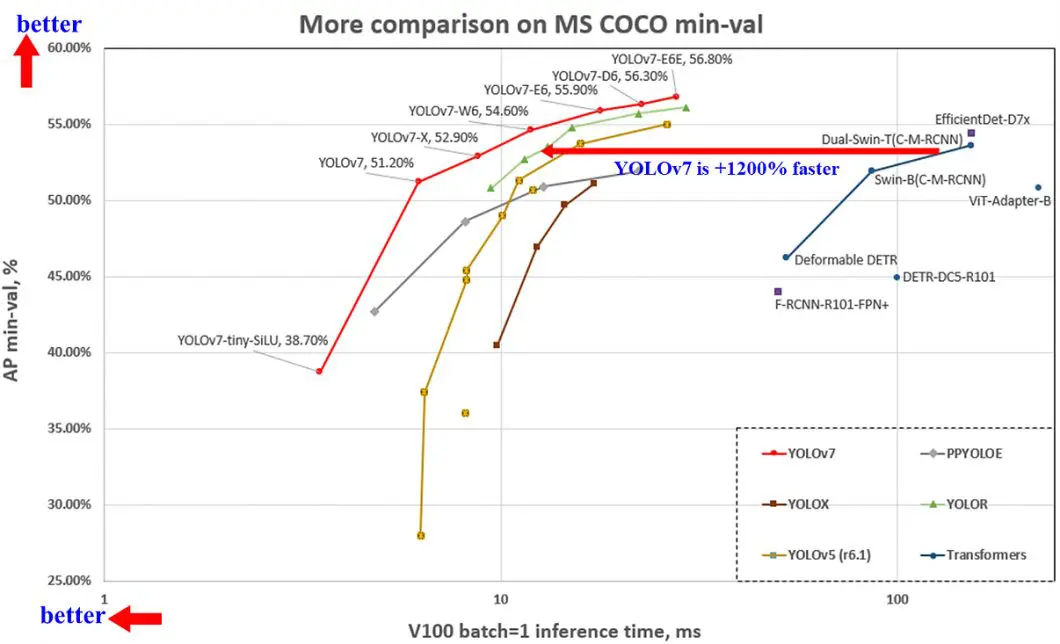
In comparison of the latest YOLO versions – YOLOv8 vs. YOLOv7 and YOLOv6 – the latest release (YOLOv8) shows the best performance in real-time benchmarks published by the creator.
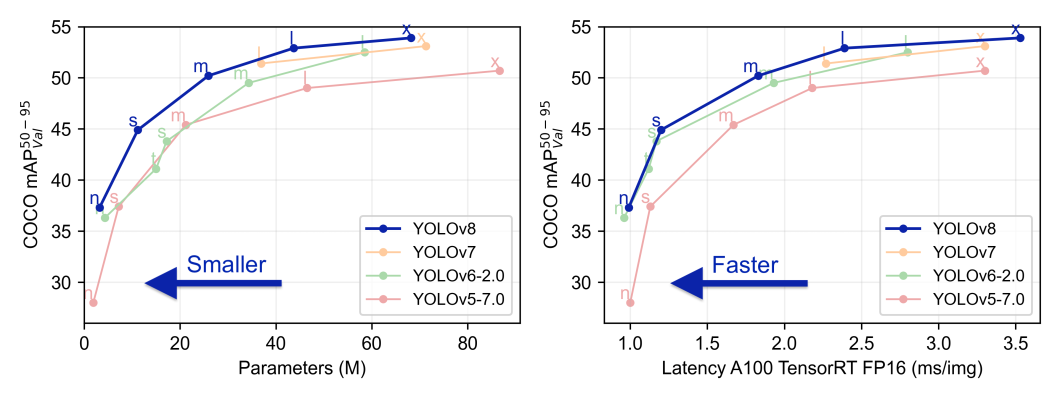
Deep Learning Object Detection Use Cases and Applications
The use cases involving object detection are very diverse; there are almost unlimited ways to make computers see like humans to automate manual tasks or create new, AI-powered products and services. It has been implemented in computer vision programs used for a range of applications, from sports production to productivity analytics. To find an extensive list of recent computer vision applications, I recommend you check out our article about the most popular computer vision applications today.
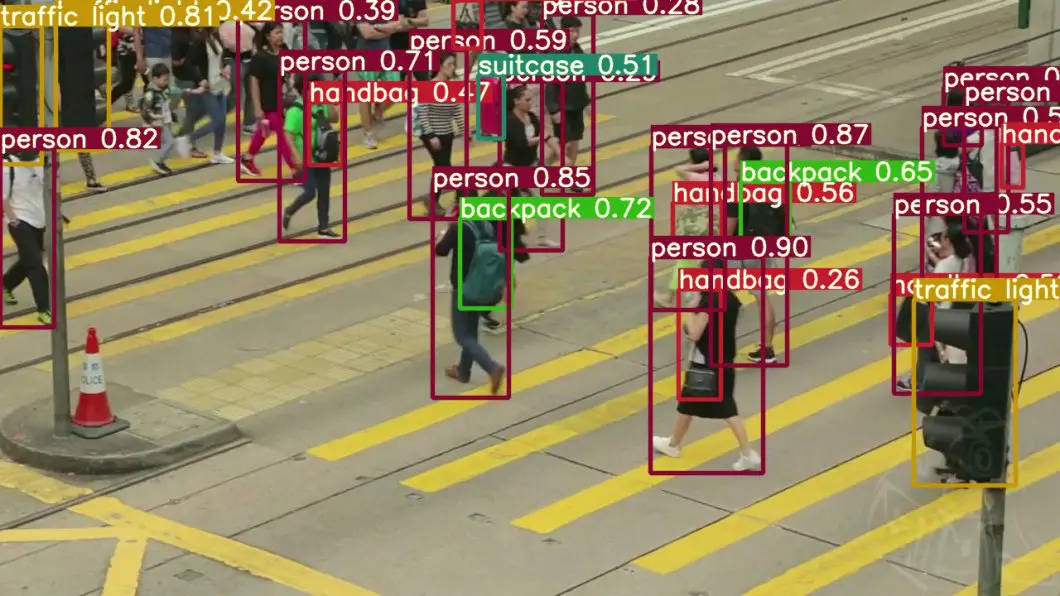
Today, deep learning object recognition is the core of most vision-based AI software and programs. Object detection plays an important role in scene understanding, which is popular in security, construction, transportation, medical, and military use cases.
Object Detection in Retail
Strategically placed people counting systems throughout multiple retail stores are used to gather information about how customers spend their time and customer footfall. AI-based customer analysis to detect and track customers with cameras helps to gain an understanding of customer interaction and customer experience, optimize the store layout, and make operations more efficient. A popular use case is the detection of queues to reduce waiting time in retail stores.
Autonomous Driving
Self-driving cars depend on object detection to recognize pedestrians, traffic signs, other vehicles, and more. For example, Tesla’s Autopilot AI heavily utilizes object detection to perceive environmental and surrounding threats, such as oncoming vehicles or obstacles.
Animal Detection in Agriculture
Object detection is used in agriculture for tasks such as counting, animal monitoring, and evaluation of the quality of agricultural products. Damaged produce can be detected while it is in processing using machine learning algorithms.
People Detection in Security
A wide range of security applications in video surveillance are based on object detection, for example, to detect people in restricted or dangerous areas, suicide prevention, or automating inspection tasks in remote locations with computer vision.
Vehicle Detection with AI in Transportation
Object recognition is used to detect and count vehicles for traffic analysis or to detect cars that stop in dangerous areas, for example, on crossroads or highways.
Medical Feature Detection in Healthcare
Object detection has allowed for many breakthroughs in the medical community. Because medical diagnostics rely heavily on the study of images, scans, and photographs, object detection involving CT and MRI scans has become extremely useful for diagnosing diseases, for example, with ML algorithms for tumor detection.
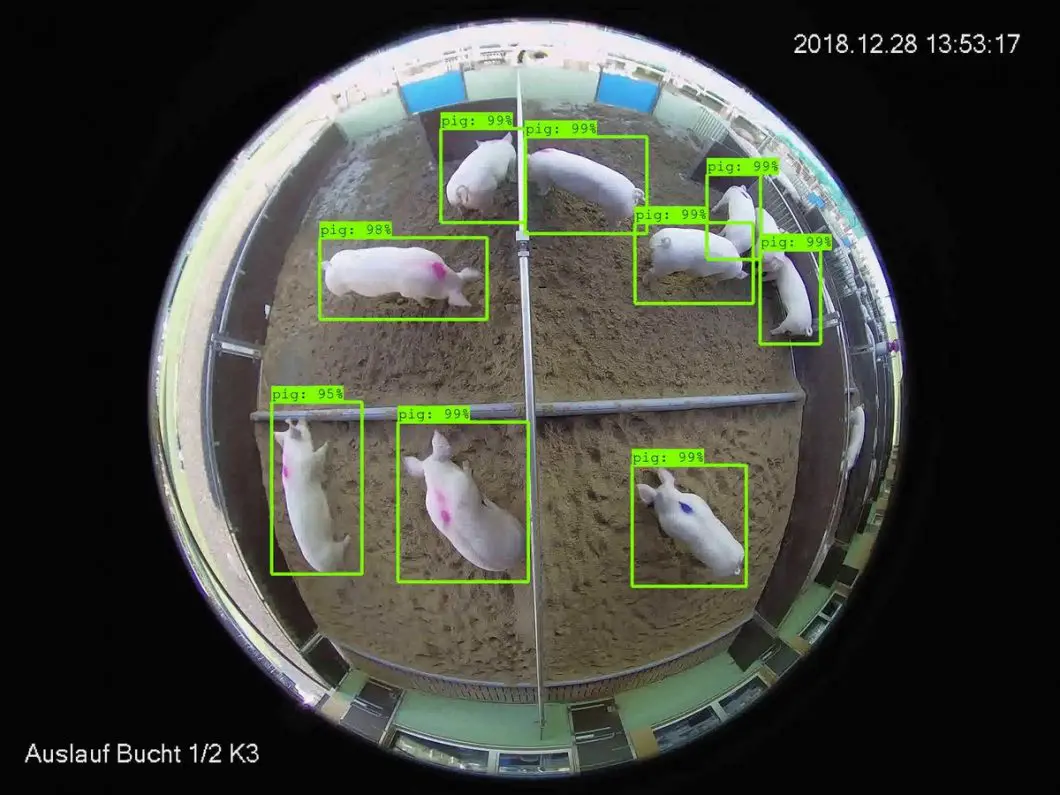
Most Popular Object Detection Algorithms
Popular algorithms used to perform object detection include convolutional neural networks (R-CNN, Region-Based Convolutional Neural Networks), Fast R-CNN, and YOLO (You Only Look Once). The R-CNNs are in the R-CNN family, while YOLO is part of the single-shot detector family. In the following, we will introduce these models and discuss the differences between the popular object detection algorithms.
YOLO – You Only Look Once
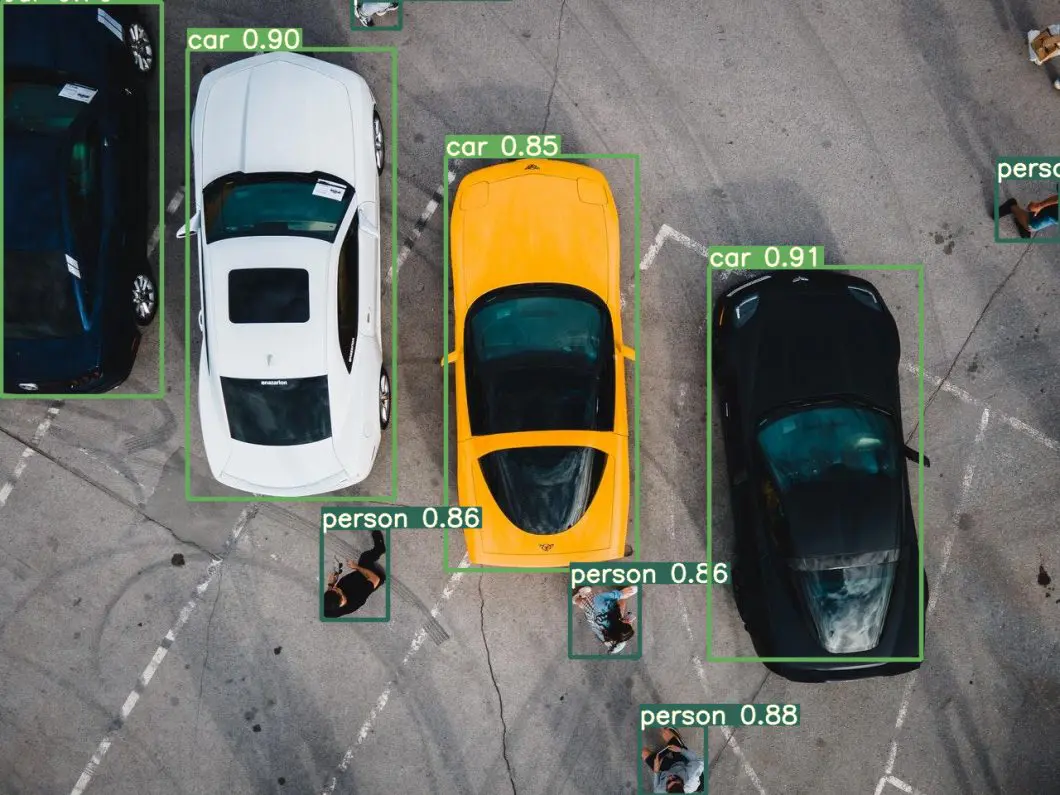
SSD – Single-Shot Detector
SSD is a popular one-stage detector that can predict multiple classes. The method detects objects in images using a single deep neural network by discretizing the output space of bounding boxes into a set of default boxes over different aspect ratios and scales per feature map location.
The image object detector generates scores for the presence of each object category in each default box and adjusts the box to better fit the object shape. Also, the network combines predictions from multiple feature maps with different resolutions to handle objects of different sizes.
The SSD detector is easy to train and integrate into software systems that require an object detection component. In comparison to other single-stage methods, SSD has much better accuracy, even with smaller input image sizes.
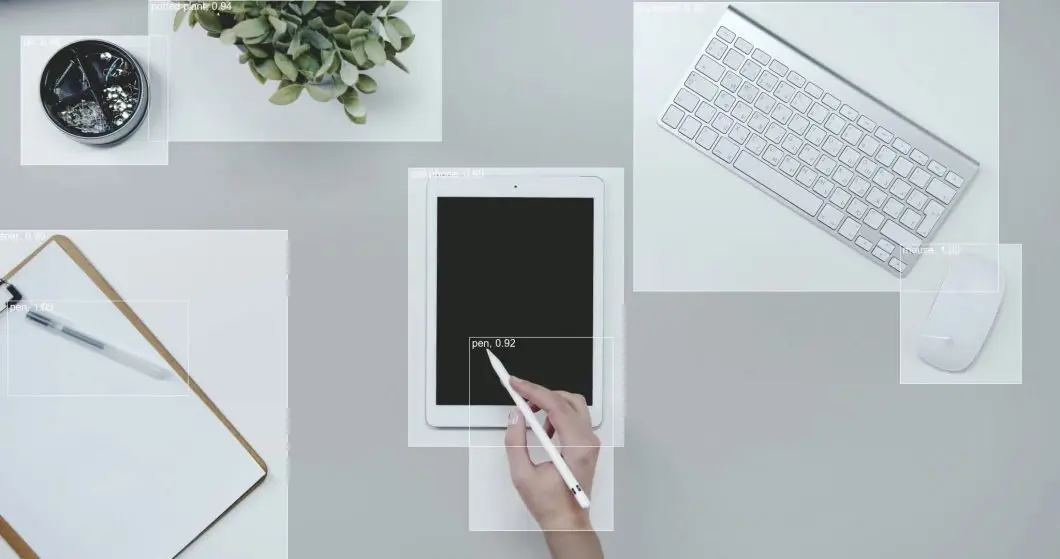
R-CNN – Region-Based Convolutional Neural Networks
Region-based convolutional neural networks or regions with CNN features (R-CNNs) are pioneering approaches that apply deep models to object detection. R-CNN models first select several proposed regions from an image (for example, anchor boxes are one type of selection method) and then label their categories and bounding boxes (e.g., offsets). These labels are created based on predefined classes given to the program. They then use a convolutional neural network (CNN) to perform forward computation to extract features from each proposed area.
In R-CNN, the input image is first divided into nearly two thousand region sections, and then a CNN is applied for each region, respectively. The size of the regions is calculated, and the correct region is inserted into the neural network. We can infer that a detailed method like that can produce time constraints. Training time is significantly greater compared to YOLO because it classifies and creates bounding boxes individually, and a neural network is applied to one region at a time.
In 2015, Fast R-CNN was developed to significantly cut down train time. While the original R-CNN independently computed the neural network features on each of as many as two thousand regions of interest, Fast R-CNN runs the neural network once on the whole image. This is very comparable to YOLO’s architecture, but YOLO remains a faster alternative to Fast R-CNN because of the simplicity of the code.
At the end of the network is a novel method known as Region of Interest (ROI) Pooling, which slices out each Region of Interest from the network’s output tensor, reshapes, and classifies it (Image Classification). This makes Fast R-CNN more accurate than the original R-CNN. However, because of this recognition technique, fewer data inputs are required to train Fast R-CNN and R-CNN detectors.
Mask R-CNN
Mask R-CNN is an advancement of Fast R-CNN. The difference between the two is that Mask R-CNN added a branch for predicting an object mask in parallel with the existing branch for bounding box recognition. Mask R-CNN is simple to train and adds only a small overhead to Faster R-CNN; it can run at 5 fps. Read more about Mask R-CNN here.
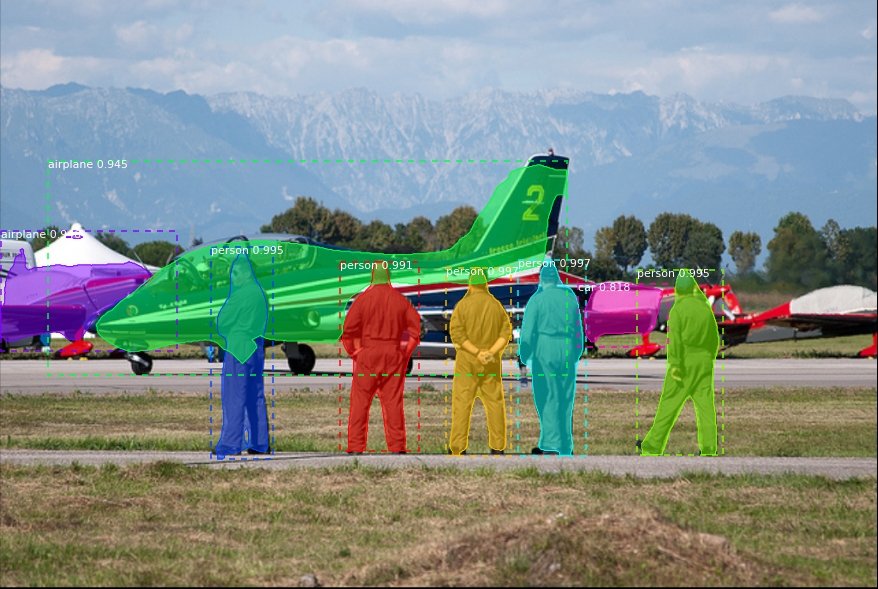
SqueezeDet
SqueezeDet is the name of a deep neural network for computer vision that was released in 2016. It was specifically developed for autonomous driving, where it performs object detection using computer vision techniques. Like YOLO, it is a single-shot detector algorithm.
In SqueezeDet, convolutional layers are used not only to extract feature maps but also as the output layer to compute bounding boxes and class probabilities. The detection pipeline of SqueezeDet models only contains single forward passes of neural networks, allowing them to be extremely fast.
MobileNet
MobileNet is a single-shot multi-box detection network used to run object detection tasks. This model is implemented using the Caffe framework. The model output is a typical vector containing the tracked object data, as previously described.
YOLOR
YOLOR is a novel object detector introduced in 2021. The algorithm applies implicit and explicit knowledge to the model training at the same time. Therefore, YOLOR can learn a general representation and complete multiple tasks through this general representation.
Implicit knowledge is integrated into explicit knowledge through kernel space alignment, prediction refinement, and multi-task learning. Through this method, YOLOR achieves greatly improved object detection performance results.
Compared to other object detection methods on the COCO dataset benchmark, the MAP of YOLOR is 3.8% higher than the PP-YOLOv2 at the same inference speed. Compared with the Scaled-YOLOv4, the inference speed has been increased by 88%, making it the fastest real-time object detector available today. Read more about the advantages of object detection, YOLOR – You Only Learn One Representation.
What’s Next for Deep Learning Object Detection?
Object detection is one of the most fundamental and challenging problems in computer vision. As probably the most important computer vision technique, it has received great attention in recent years. Especially with the success of deep learning methods that currently dominate the recent state-of-the-art detection methods.
One of the use cases of object detection is product detection. This is mainly used by retailers to improve operational efficiency and save costs. Product detection methods automate the process of identifying and classifying products using AI algorithms with deep learning. Read more to learn more about how Viso Suite can implement product detection technology in your business.
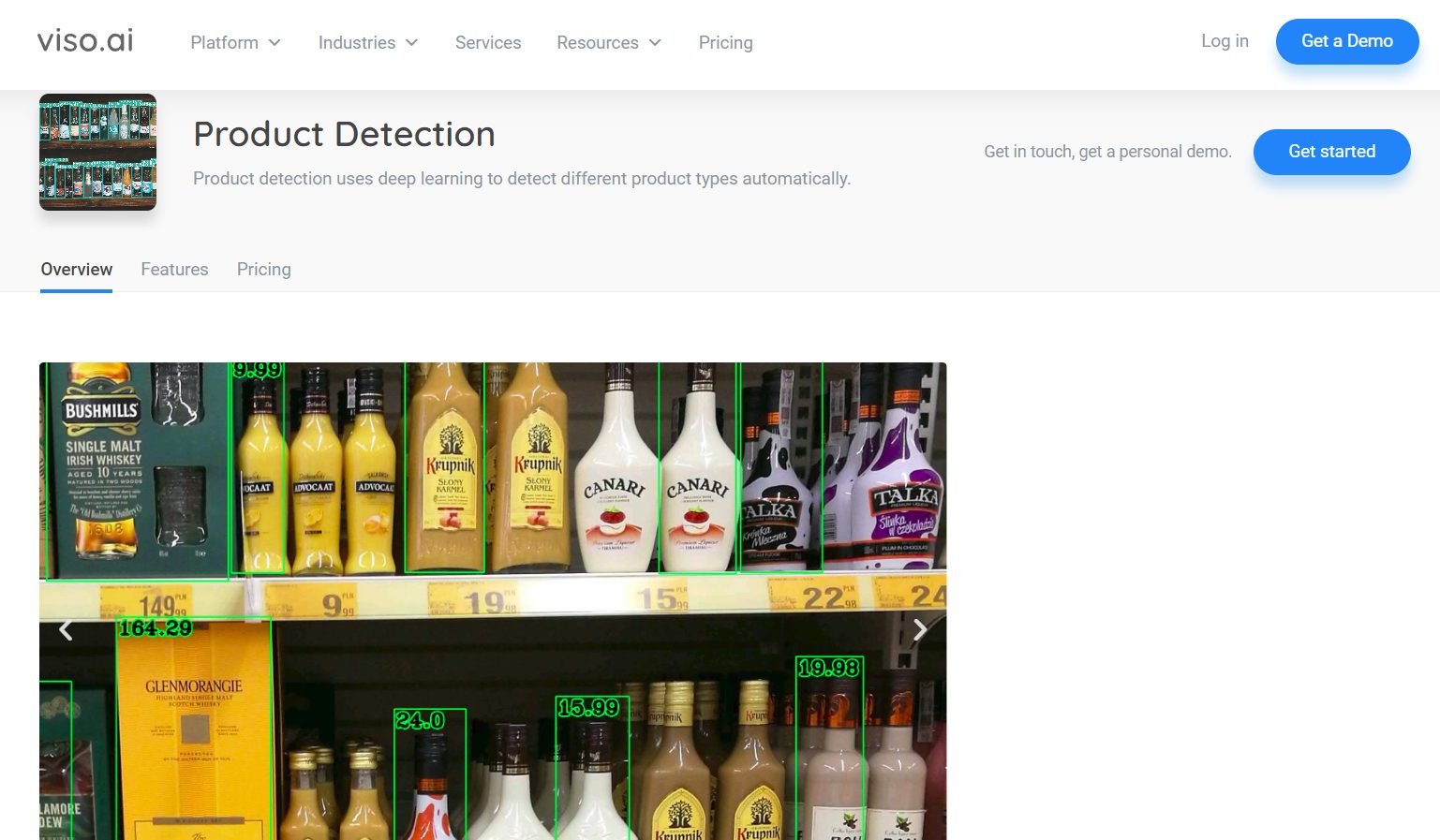
Further Reads about Object Detection
Object detection methods are increasingly important for computer vision applications in any industry. If you enjoyed reading this article, I would suggest reading:
- An Introduction to MLOps – Methods To Deliver Machine Learning
- Explore the fast Segment Anything Model (SAM) that can identify objects without training
- Read more about Convolutional Neural Networks (CNNs)
- See the top 5 Deep Learning Frameworks You Need To Know
- How to build a People Counting system in 10 minutes