Computer Vision is a key Artificial Intelligence (AI) technology rapidly entering the oil and gas industry. In oil and gas, computer vision has created significant potential for innovation and growth, promising to revolutionize industry operations. Companies aim to create value using AI technology instead of relying on traditional, human-centered processes.
As AI changes the rules of competition, organizations race to build internal capabilities, create custom AI vision applications, and gather learnings with early adoption to iteratively optimize and operate AI technology at scale. In the following, we will cover:
- Technology Trends in the Industry
- Computer Vision Technology Application in Oil and Gas
- List of top AI vision applications
About us: Viso.ai provides the end-to-end Computer Vision Infrastructure Platform Viso Suite. Industry leaders use it to rapidly build, deliver, and scale their real-world computer vision use cases. Get a demo for your company.
Technology trends of AI in oil and gas
Emerging technology and breakthroughs in the field of computer vision and Edge AI allow the highly scalable use of distributed computer vision applications. Those applications are possible because the workloads from computer vision and AI algorithms have moved from the cloud to the network edge. We can also refer to this concept as edge computing.
The combination of the Internet of Things with on-device machine learning allows processing the video streams of distributed cameras in real-time, at high computational efficiency. Those technological advances make it possible to build large-scale deep learning applications with a large number of connected endpoints (AIoT).
As a result, it becomes possible to build mission-critical, large-scale computer vision systems with remote cameras connected to computing devices. I recommend reading our article about Edge AI to learn more about Edge AI technology.
Compared to conventional IoT sensors and low-power devices, cameras provide a contactless method that provides rich information about complex objects and situations. With computers that can see, it becomes possible to automate human tasks and accelerate processes, increase operational efficiency, and reduce human error or subjectivity.
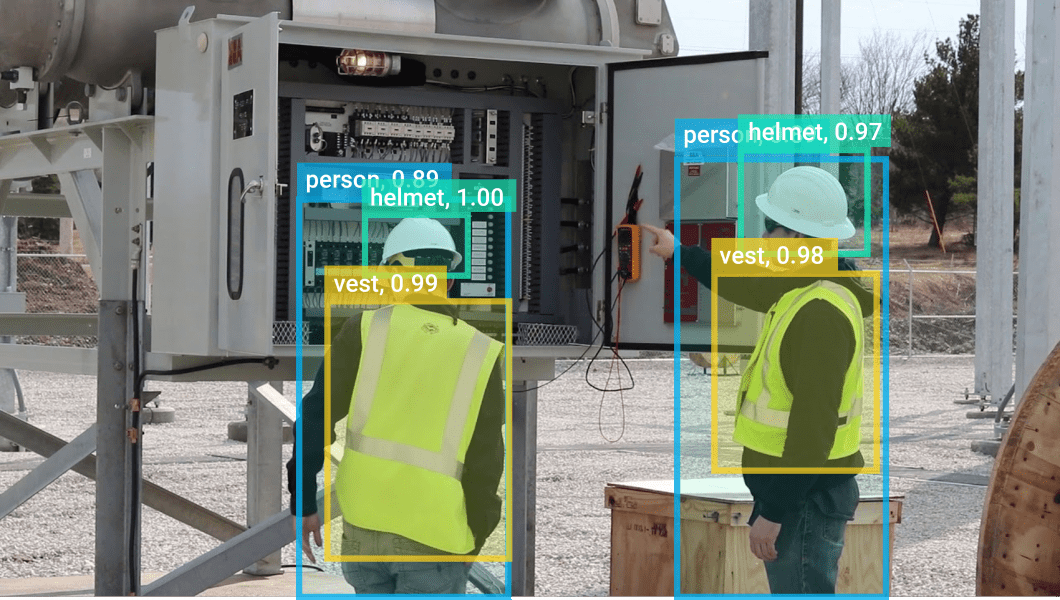
AI solutions for computer vision in oil and gas
In the oil and gas sector, companies generally adopt AI technologies to improve operational efficiency across the supply chain through industrial automation (Industry 4.0). This typically translates to accelerating processes, improving cost efficiencies, and reducing operational risks.
The major application types include:
- AI maintenance and service life prediction
- Safety and compliance monitoring
- Reliability, reduce business interruption
- AI risk evaluation, structural health monitoring
- Sustainability and resource optimization
- Non-destructive testing and inspection
- Analyze fatigue and corrosion of systems
In the following, we will highlight some popular use cases of AI vision in more detail.
Oil or gas pipeline inspection at scale
Assessing large-scale infrastructure systems to determine their condition and health status under in-service or extreme hazard events poses great challenges to the operators. Deep learning approaches leverage computer vision models for the conditional assessment of large-scale systems by extracting critical information from the remote sensing data of cameras.
Firstly, the visuals need to be pre-processed on pixel level with traditional computer vision methods. Next, deep learning models (e.g., R-CNN) can evaluate the condition of different critical components. Application experiments demonstrated that DL models can rapidly and accurately detect the damage location and level. Hence there is a high potential for large-scale oil/gas pipeline assessment at both spatial and temporary scales over conventional models.
Remote oil and gas field monitoring
Real-time oil and gas field monitoring with cameras to automate and digitize oil development sites for maintenance of offshore oil and gas fields. Such systems aim to increase oil and gas productivity by monitoring and predicting the condition of load pumps with machine learning techniques.
The digital transformation of the oil and gas industry is driven by low-cost sensors and high-performance computing with distributed systems to extract high-value information from big data, directly at the source of data (Edge Intelligence). The multi-dimensional value and comparably low costs of cameras allow large-scale video analysis without the need to attach physical sensors.
Automatic recognition of analog instruments
Computer vision can be used to read analog gauges at power substations and other equipment. Cameras with computer vision are used to read oil level gauges, winding temperature gauges automatically, and SF6 gas density gauges (find the study here).
Thereby, vision algorithms use color segmentation to detect the position of the pointers and scale marks. Such applications work much faster and more accurately than humans and help to avoid dangerous accidents and expensive production interruptions.
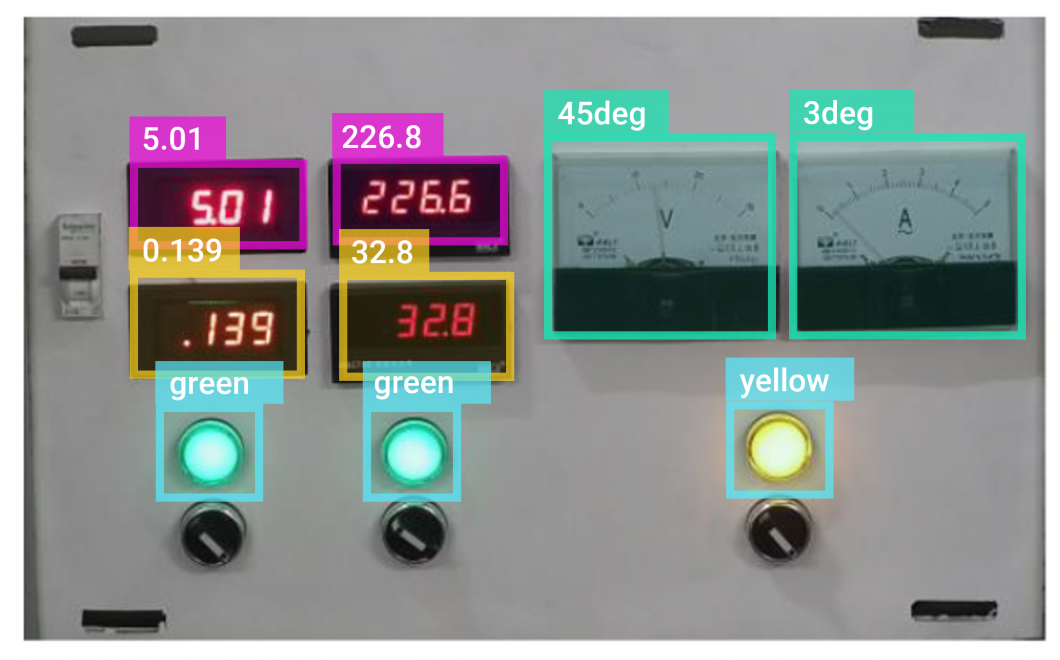
Wireline spooling automation with computer vision
In the oil and gas industry, wirelines are used for well intervention and reservoir evaluation. While the tool string is retrieved from the well, the wireline cable, typically under tension, is spooled on a drum. Improper spooling could cause severe cable damage.
New computer vision applications have been implemented to detect spooling anomalies with advanced data analytics (Inception-V3 base network) and to predict the cable position in real-time (VGG-19 network).
Leak detection with computer vision
Machine vision is used to detect methane gas emissions using regular infrared cameras. For example, a use case for deep learning-based methane detection was recently developed. The automated approach simplifies the leak detection analysis with very high accuracy, as high as 95-99%.
Traditional optical gas imaging (OGO) methods to detect methane leaks are labor-intensive and unable to provide leak detection results without the judgment of a human operator. Computer vision approaches for optical gas imaging with convolutional neural networks (CNN) require training with methane leak images to enable automatic detection.
Visual inspection for corrosion detection
Corrosion is a major defect in structural systems; it has a significant economic impact and can pose safety risks if left untended. Inspection tasks that must be performed periodically are often carried out manually, sometimes in hazardous conditions.
In addition, the manual interpretation process is usually very expensive, time-consuming, and subjective. Therefore, deep learning methods analyze the video images of cameras to automate inspection tasks.
A key indicator during inspections is the presence of corrosion. Hence, computer vision has been successfully applied in use cases for automatic rust detection. This leads to cost savings and faster, better decision-making of preventative or corrective measures based on quantitative insights at scale.
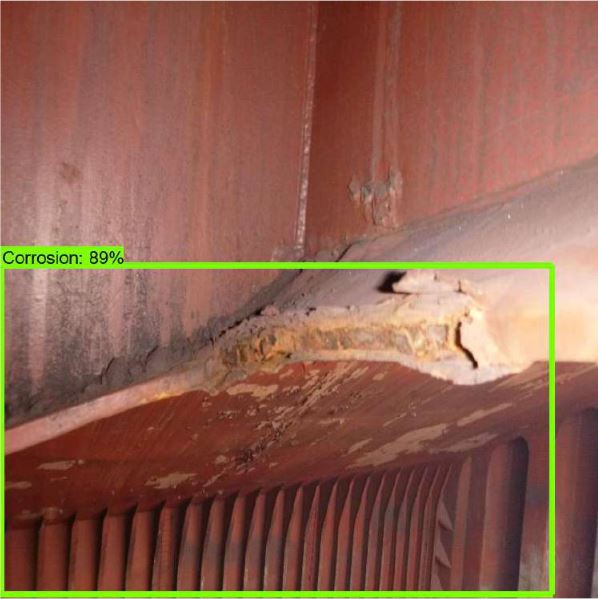
Geological assessment and AI-aided exploration
Computer vision tools can perform rock typing based on images of rock samples extracted from the wells. Therefore, deep neural networks (DNN) are applied. Traditional methods of petrophysical interpretation are time-consuming, and the results depend strongly on the human expert (subjectivity).
In tests, the ML model’s accuracy was 92% compared to manual interpretation and about 1,000 times faster than the manual method. Interestingly, the study found that the second manual interpretation showed an accuracy of 91% compared to a second manual interpretation.
This shows how AI methods are the obvious way to accelerate the process and, even more critically, exclude subjectivity in the interpretation process.
Intelligent fire detection with AI vision
Fire is one of the most severe causes of accidents that may lead to casualties, considerable production loss, and equipment damage. Human operators traditionally performed fire detection through video cameras, especially in petroleum and chemical facilities. However, it’s almost impossible for human operators to spot fires in time with hundreds of video cameras installed in large-scale settings. Human subjectivity, distraction, and visual perception limit the accuracy of human safety supervisors.
Intelligent fire detection applies computer vision methods to video cameras to detect fires. Some methods have shown better results when focusing on smoke detection. The method uses background subtraction to detect motion and reduce computational complexity. The object detection and image classification models perform fire detection at a rate of 98.4%, with a false alarm rate of 99.9%, at 27.4ms detection time per frame.
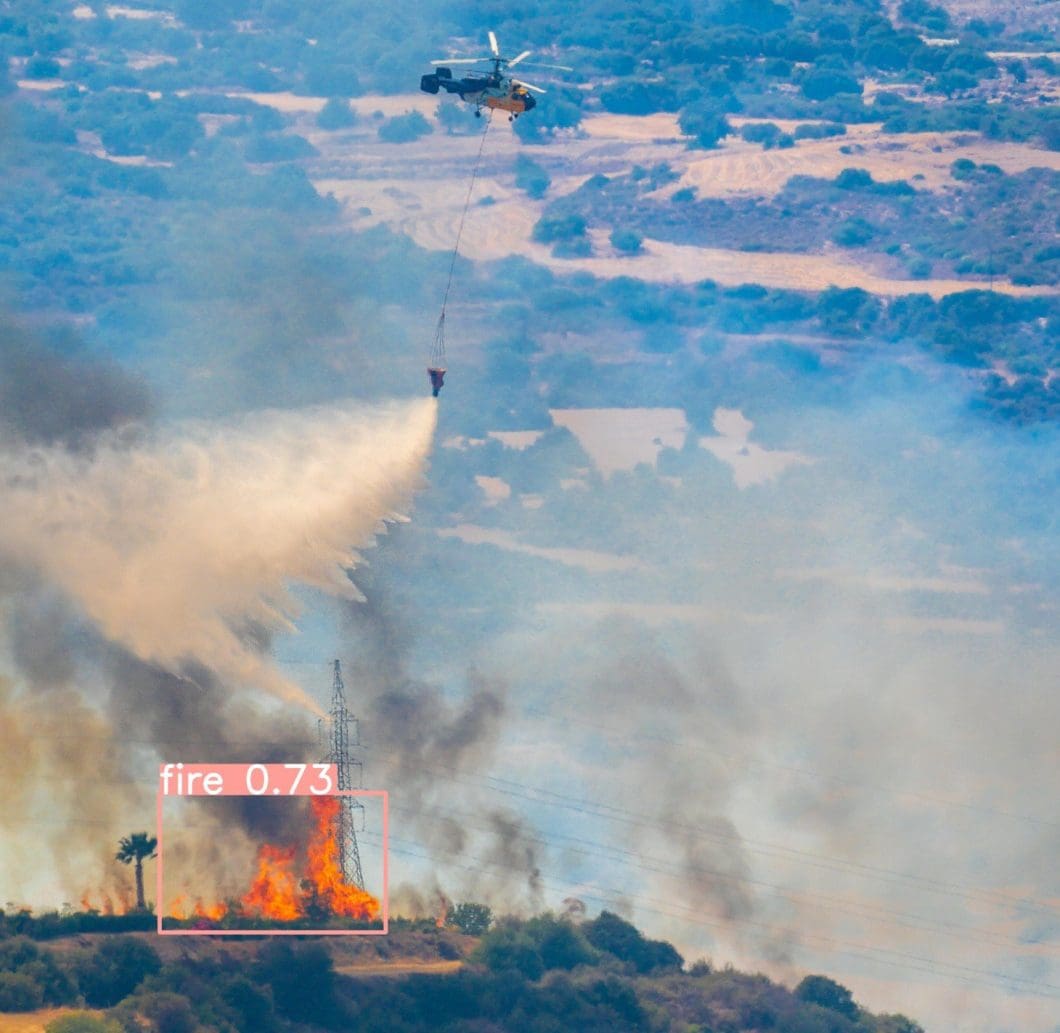
Predictive maintenance and equipment failure detection
In oil plants, deep learning models can detect equipment failures or deterioration. Therefore, custom neural networks are trained to detect anomalies during automated equipment inspection. If an AI model detects a potential issue, an image can be automatically sent to a human supervisor for manual review.
By continuously monitoring equipment performance, they can predict potential failures, allowing for proactive maintenance to minimize downtime and prevent accidents.
Getting starting with computer vision for oil and gas
Today, we are only seeing the beginning of the era of AI-driven applications. Edge AI made it possible to move AI vision capabilities from the cloud to the field, enabling large-scale applications. Because of strategic importance and distinct operational workflows, most oil and gas companies aim to build and operate custom computer vision systems.
New applications for computer vision and artificial intelligence in the oil and gas industry primarily aim to improve maintenance, workplace safety, management, life-cycle sustainability, quality, and operational efficiency.
To find related applications, check out our industry report about computer vision in energy and utilities industry applications.
Integrating Viso Suite to existing tech stacks
If your organization is looking for a full-stack computer vision infrastructure to build, deploy, and monitor custom and enterprise-grade computer vision applications, check out Viso Suite. Our platform leverages the latest Edge AI with features accelerating the building and operating of large-scale deep learning systems.
Get in touch with sales and get a personal demo.
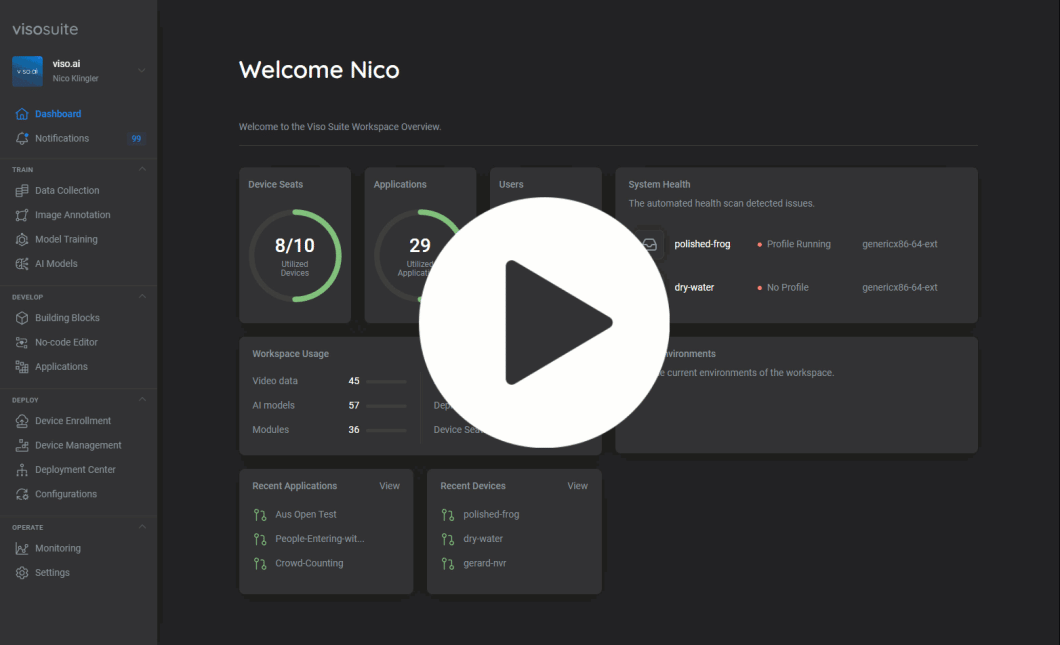