Technology continues to evolve at a rapid pace, with huge leaps in the advancement of artificial intelligence (AI). These advancements open a world of new possibilities for the application of computer vision. This article will explore what lies ahead for computer vision trends. We will dive into what this will mean for the industry, the businesses that adopt it, and the wider society.
Update: for our latest report, check out our Computer Vision Trends in 2025 article.
The Rise of Generative AI
The recent popularity of Generative AI systems has seen organizations everywhere rush to explore the technology’s transformative capabilities. AI tools such as OpenAI’s ChatGPT and Dall-E have improved operations and tackled problems that would once have been impossible to solve.
Generative AI has entered the mainstream. A host of startups, including Hugging Face, Anthropic, Stability AI, Midjourney, and AI21 Labs, will join market leader OpenAI.
The field of computer vision will be among those exploring its potential. Over the next 12 months, we can expect to see Generative AI further enable synthetic data creation.
Generative AI can be used to create outputs across a variety of domains. These can include large language models like text-to-image, text-to-video, text-to-audio, and more.
The output data from generative models can be used to train computer vision models, such as those for object detection or facial recognition. Not only will this minimize the risk of privacy violations, but make the model training process significantly less expensive and time-consuming. This is because it labels training data faster and more efficiently than humans.
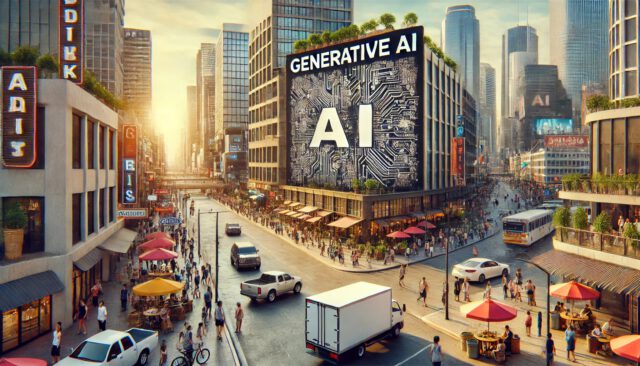
Greater Insights from Multimodal AI
Until recently, AI models have tended to focus on processing information from a single modality. This would be a single source of data, such as text, images, or video.
Multimodal deep learning, however, makes it possible to train models to recognize relationships between different modalities, translating text to audio, text to images, images to videos, and so on. More importantly, AI is now capable of combining multiple modalities. Thus, treating them as a unified source for insights and predictions.
Consider the impact of AI in healthcare, for example. Traditionally, assessing a patient’s health was dependent on a single modality, typically either textual or visual. A combination of both, i.e. a doctor’s notes and data from an examination, will result in faster, more accurate diagnoses.
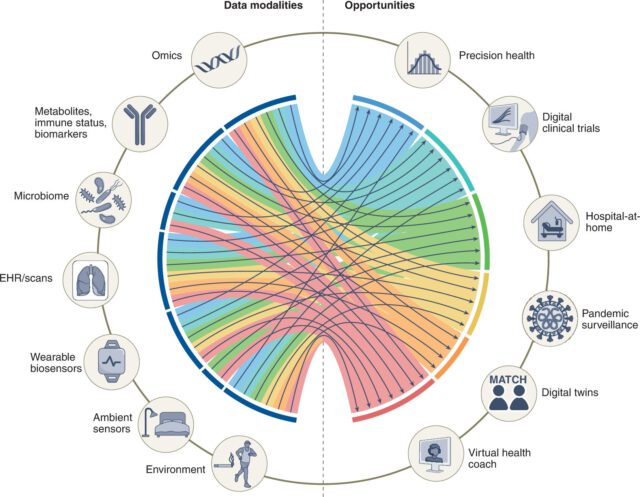
Computer Vision in Healthcare
The impact of AI in healthcare will go far beyond improving the speed and efficiency of health assessments.
Doctors and researchers have been using computer vision algorithms to differentiate between healthy and cancerous tissue. This speeds up the analysis of medical imaging and scans. In turn, doctors can quickly identify and diagnose serious diseases and ensure accurate and timely record-keeping. This paper, for example, proposes an application of AI and computer vision to enable medical practitioners to promptly and effectively diagnose breast cancer.
Computer vision will also play a variety of roles in operating theatres, such as monitoring surgical procedures. This can track the location of instruments and ensure the correct performance of surgeries. In turn, this minimizes the risk of surgical instruments left inside the patient. And, increasingly, healthcare professionals will use augmented reality to guide – and even perform – remote surgery.
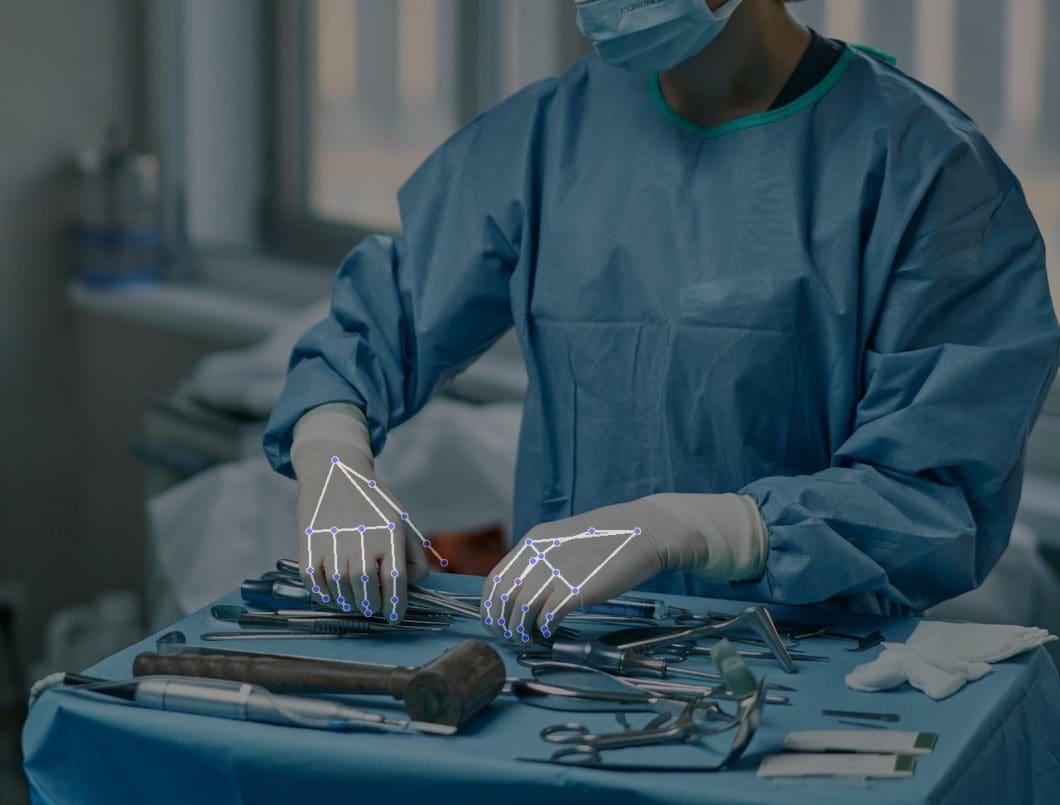
Edge Computing and Lightweight Architecture
We will continue to see an increased focus on edge computing within the computer vision space. Processing visual data directly on edge devices such as smartphones, drones, and IoT sensors, where that data is captured, reduces latency. This enables real time visual data processing, essential for use cases across industries.
Looking ahead, it’s likely that the growing adoption of edge computing architecture will lead to the development of small, efficient computer vision applications. These small applications can run on low-power devices, a boon to manufacturing and security operations.
However, these smaller, more efficient computer vision applications will require lightweight AI models. These can be deployed on low-power devices with limited processing power and memory.
R-CNN (Region-based Convolutional Neural Networks) is one of the most commonly used machine learning models. However, while R-CNN is highly accurate for object detection, it requires heavy – and expensive – computational resources.
In contrast, lightweight AI architectures like YOLO (You Only Look Once) require fewer powerful resources. These lightweight models are a more suitable option for edge devices.
Similarly, the high accuracy and real-time performance of the SSD (Single Shot Detector) object detection algorithm have made it a popular choice for a wide range of applications. These applications include AI in autonomous vehicles and surveillance systems, among others.
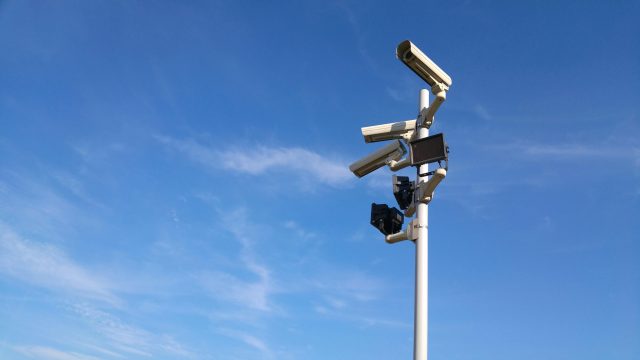
Enabling Autonomous Vehicles
Self-driving cars are high on the list of key use cases for computer vision technology. Currently, the technology used to navigate and operate autonomous vehicles relies on processing input from various sources, ranging from cameras and GPS to RADAR and LiDAR.
But as they become more prevalent, it’s only a matter of time before the computers in these vehicles can drive almost entirely by sight, the same way a human driver does. To this end, we can expect the incorporation of increasingly sophisticated computer vision technology into the design and production line process, as self-driving vehicles edge ever closer to becoming an everyday reality on our roads.
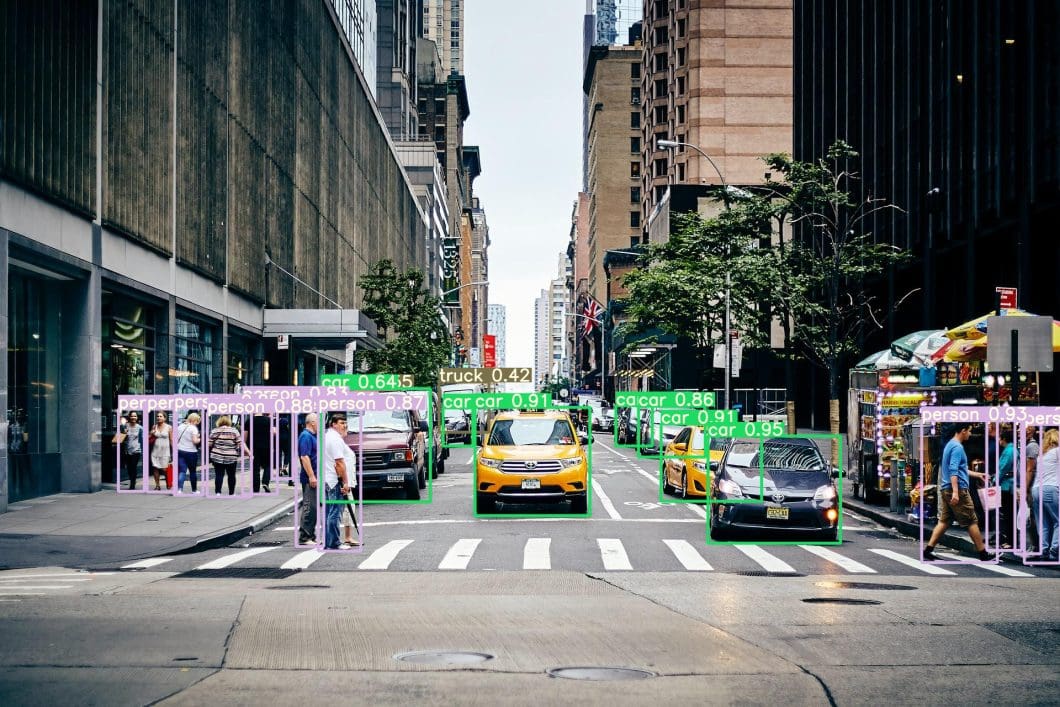
Tackling Deepfake Deception
AI-generated “deepfakes” are becoming so convincing that it can often be hard to differentiate between real and computer-generated video content. In a time of elevated political uncertainty, and particularly with UK and US elections on the horizon, the effect this could have on people’s ability to detect misinformation is highly concerning.
Computer vision will have an increasingly significant role in countering the threat of misinformation. Computer vision systems trained on vast datasets of real and fake digital images and videos are used to analyze media. These systems can identify signs of whether the media is artificially generated or manipulated. The diagram below illustrates the general architecture of such a deepfake detection system.
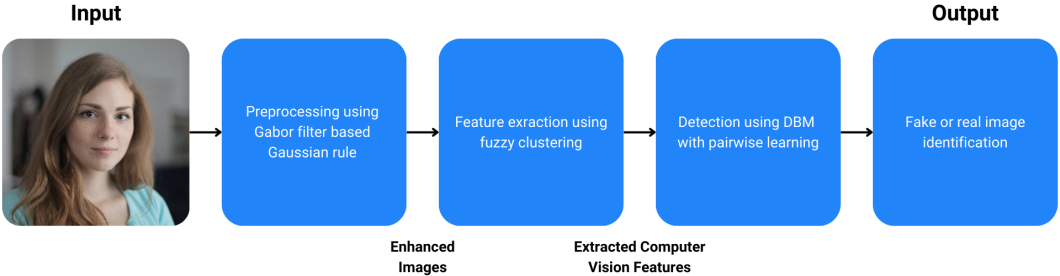
Following AI’s increasing prominence in our day-to-day lives, deepfakes become ever more realistic too. Improved deep learning systems will be essential in helping fight disinformation.
Focusing On Augmented Reality
Computer Vision is an essential component of augmented reality (AR) technology, enabling computers to understand visual information and overlay it with digital content.
A flurry of new consumer-grade AR devices from companies like Apple and Meta will hit the market. This means that we will see Computer Vision-augmented tools become more widely available.
Thanks to new AR devices, workers in manufacturing will be able to access real-time instructional and administrative information. In retail, AR enables consumers to visualize detailed product and pricing information. AR can supplement traditional teaching content with engaging immersive educational experiences in education.
Sophisticated Satellite Vision
Space technology is big business. NASA’s budget for 2023 was $25.4 billion – an increase of 5.6% from 2022. Thanks to advances in computer vision technology, images captured by satellites are more detailed and insightful than ever. With strong exploration and satellite imagery initiatives, we can expect to see machine learning aiding advances both in space and on Earth.
Object Detection in Outer Space
The high-resolution, high-sensitivity instruments carried by the James Webb Space Telescope were launched in 2021. By implementing computer vision AI technologies, the telescope can enhance, filter, and analyze the images and data taken in space. Additionally, machine vision technology allows the telescope to find more objects in space. These advancements allow it to view objects too old, distant, or faint for the Hubble Space Telescope.
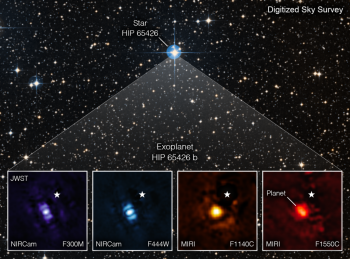
Improving Satellite Imagery on Earth With Computer Vision
With computer vision, the resolution of images taken with the telescope is also further improved. This vastly improved resolution makes it possible to effectively monitor a range of activities on Earth. Such activities include the spread of wildfires, deforestation, and urban sprawl on land. While also being able to measure the impact of factors such as migration and pollution on marine environments.
Advances in 3D Computer Vision
The recent development of sophisticated algorithms has opened more opportunities for the application of 3D computer vision. This includes using multiple cameras to capture various angles of objects or light sensors to measure the time for light to reflect off an object. At present, autonomous cars utilize both of these methods in their safety systems.
Whether spatial or time-based, advances in 3D computer vision will provide better-quality data on depth and distance. These advances allow for the creation of accurate 3D models for digital twins: precise replicas of an object, building, or person for use in simulations.
The depth of information provided by 3D computer vision will also improve accuracy. This can be done by leveraging depth data to distinguish between objects in a cluttered environment, as in the diagram below. Thereby, ensuring greater precision and reliability.
Ensuring Ethics in Computer Vision
While the rapid implementation of computer vision in society promises an exciting future, ethics must always remain a priority. Issues of bias and fairness have always been key considerations in AI.
To this end, governments worldwide have proposed strict regulations such as the EU’s AI Act. This measure aims to ensure AI’s responsible development. AI companies agree this is necessary while avoiding the risk of stifling innovation.
There will be a greater focus on addressing imbalances inherent in image recognition and facial recognition algorithms, for example. Thus, algorithms will require the creation of more diverse and representative datasets. These more diverse datasets can help overcome biases related to race, gender, and other distinguishing factors.
Furthermore, computer vision technology is increasingly being used in public areas. With this in mind, there will be a growing emphasis on privacy protection measures. For example, face-blurring to protect individuals’ identities.
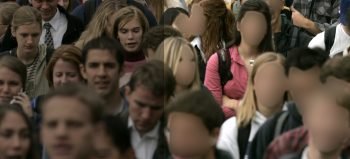
What’s Next in Computer Vision AI Trends?
Technology is advancing so quickly that it can often be hard to keep up. So much could happen over the next 12 months as companies fundraise and rapidly grow. Indeed, the list of trends and use cases above is far from exhaustive. However, computer vision will certainly have an increasingly vital role in the future of industry and society.