Pose estimation is a fundamental task in computer vision and artificial intelligence (AI) that involves detecting and tracking the position and orientation of human body parts in images or videos.
This article will explore the latest advances in pose analytics algorithms and AI vision techniques, their applications and use cases, and their limitations.
- Definition: What is pose estimation?
- Variations: Head pose estimation, animal pose estimation, etc.
- How pose estimation works: Deep learning methods
- Use Cases and pose estimation applications
- How to get started with AI motion analysis
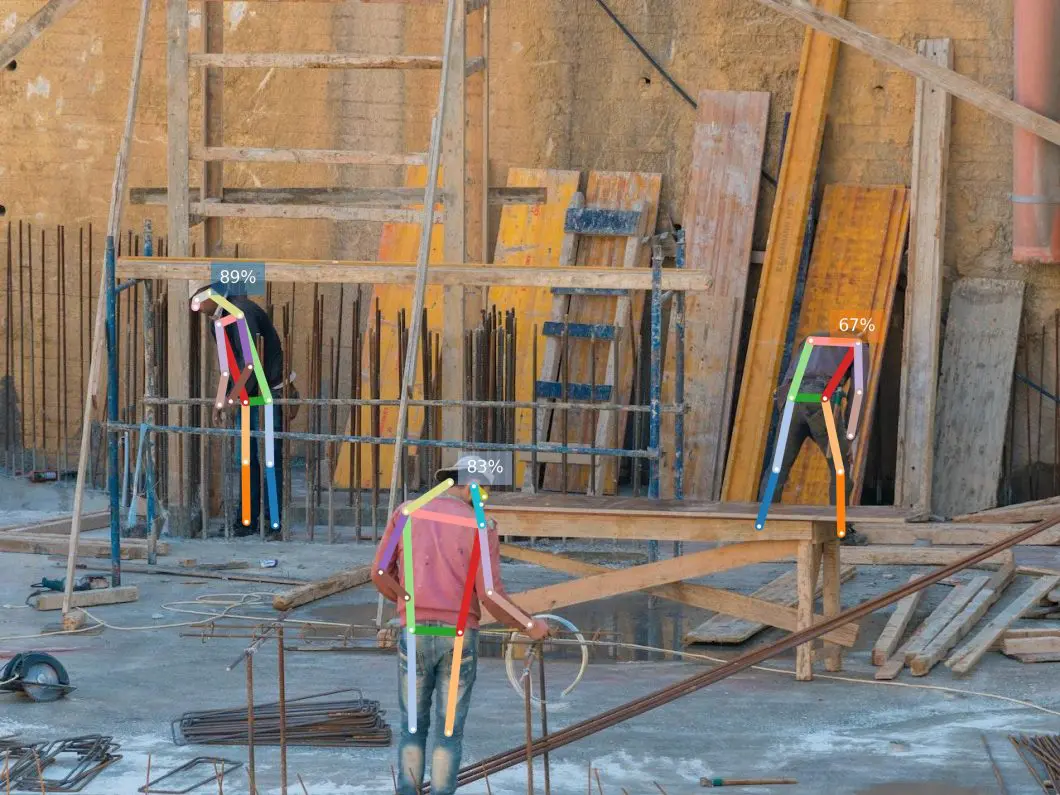
About us: Viso.ai provides the leading end-to-end Computer Vision Platform Viso Suite. Our solution enables organizations worldwide to build and deliver computer vision applications at scale. Get a demo for your organization.
What Is Pose Estimation?
Human pose estimation and tracking is a computer vision task that includes detecting, associating, and tracking semantic key points. Examples of semantic key points are “right shoulders,” and “left knees.”
Object pose estimation uses a trained model to detect and track the key points of objects such as cars. Examples of such key points are “left brake lights of vehicles.”
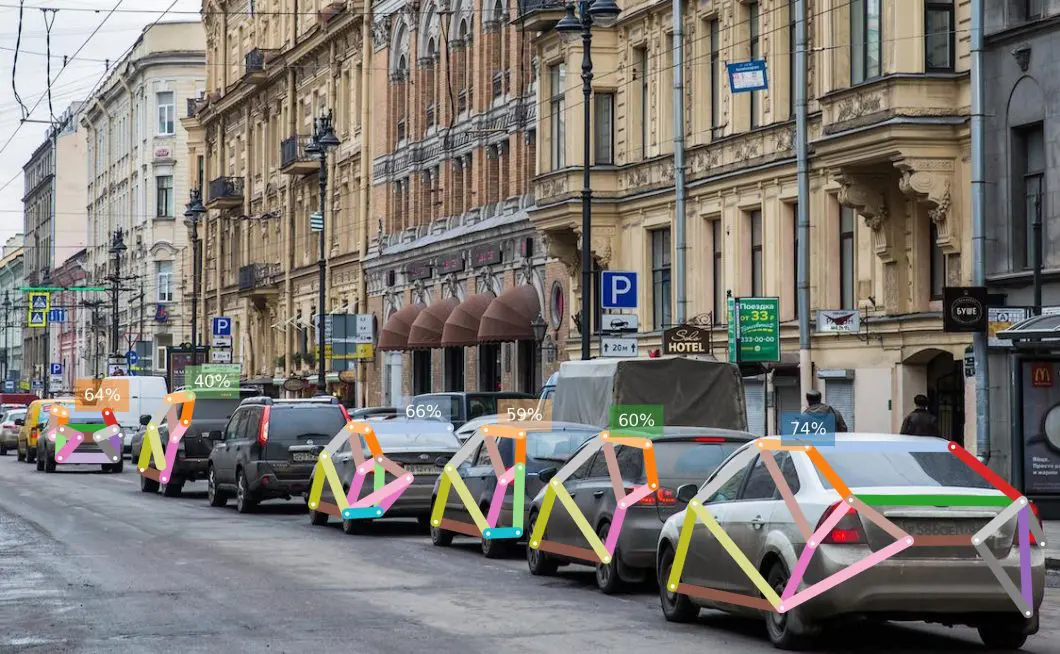
The performance of semantic keypoint tracking in live video footage requires high computational resources, which has been limiting the accuracy of pose understanding. With the latest advances in hardware and model efficiency, new applications with real-time requirements are becoming possible and economically feasible.
Today, the most powerful image processing models are based on convolutional neural networks (CNNs). Hence, state-of-the-art methods are typically based on designing the CNN architecture tailored particularly for human or objector pose detection systems.
Bottom-up vs. Top-down methods
All approaches for pose estimation can be grouped into bottom-up and top-down methods.
Bottom-up methods estimate each body joint first and then group them to form a unique pose. Bottom-up methods were pioneered with DeepCut (a method we will cover later in more detail).
Top-down methods run a person detector first and estimate body joints within the detected bounding boxes.
The Importance of Pose Estimation
In traditional object detection, people are only perceived as a bounding box (a square). By performing pose detection and pose tracking, computers can develop an understanding of human body language. However, conventional pose-tracking methods are neither fast enough nor robust to occlusions.
High-performing real-time pose detection and tracking will drive some of the biggest trends in computer vision. For example, tracking a large number of keypoints will enable computers to develop a finer-grained and more natural understanding of human behavior.
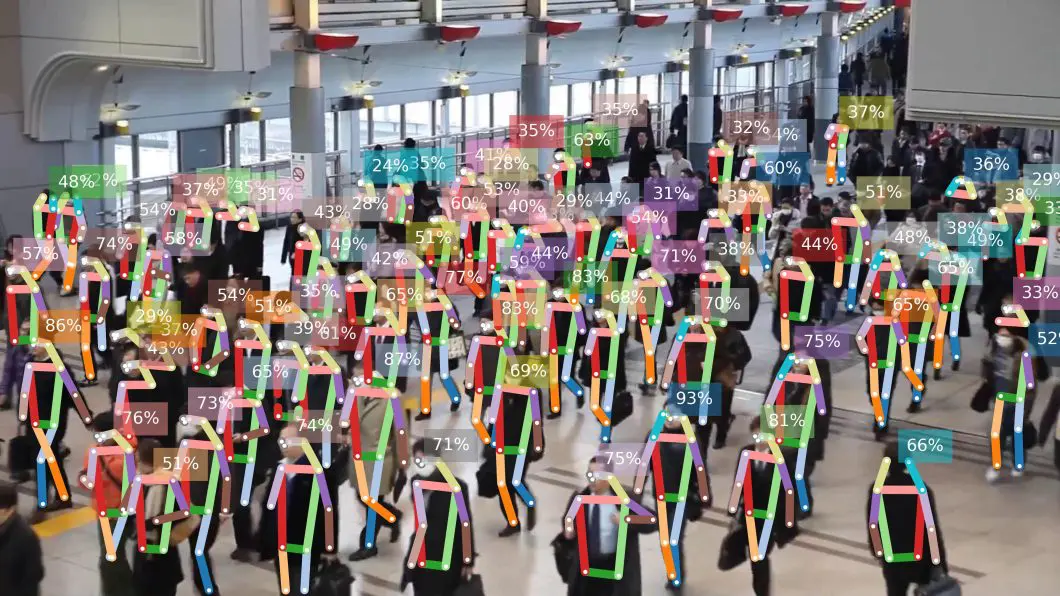
This will have a big impact on various fields, for example, autonomous driving, sports, healthcare, and many more. Today, the majority of self-driving car accidents are caused by “robotic” driving, where the self-driving vehicle conducts an allowed but unexpected stop and a human driver crashes into the self-driving car. With real-time body pose detection and tracking, the computers can understand and predict pedestrian behavior much better – allowing more natural driving.
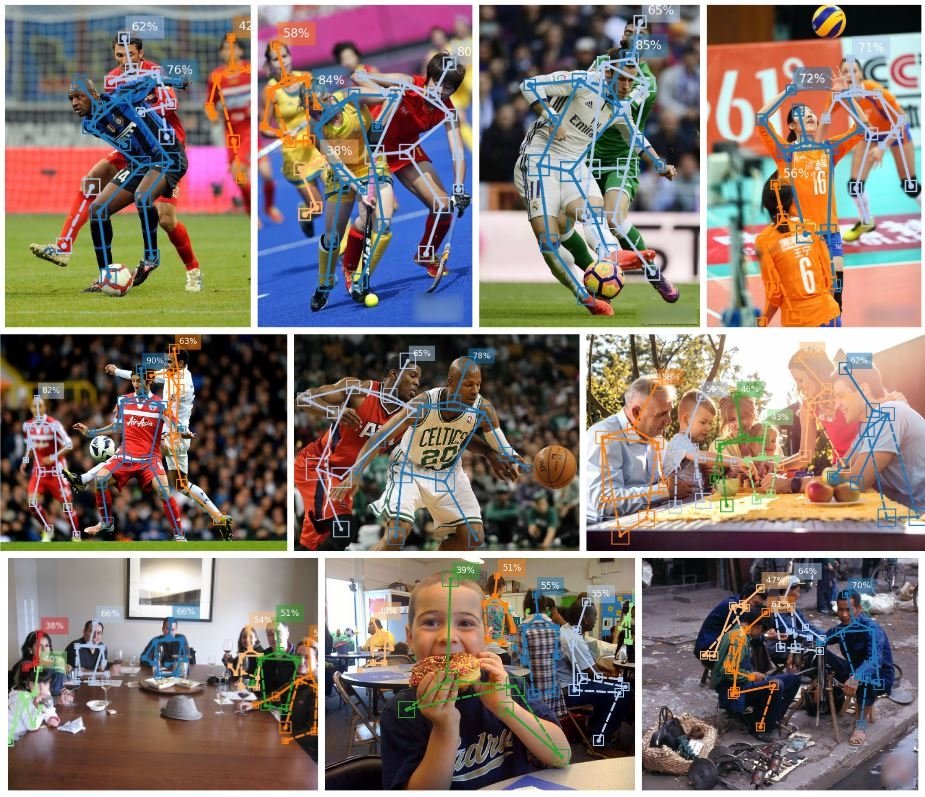
What is Human Pose Estimation?
Human pose estimation aims to predict the poses of human body parts and joints in images or videos. Since pose motions are often driven by some specific human actions, knowing the body pose of a human is critical for action recognition and video understanding.
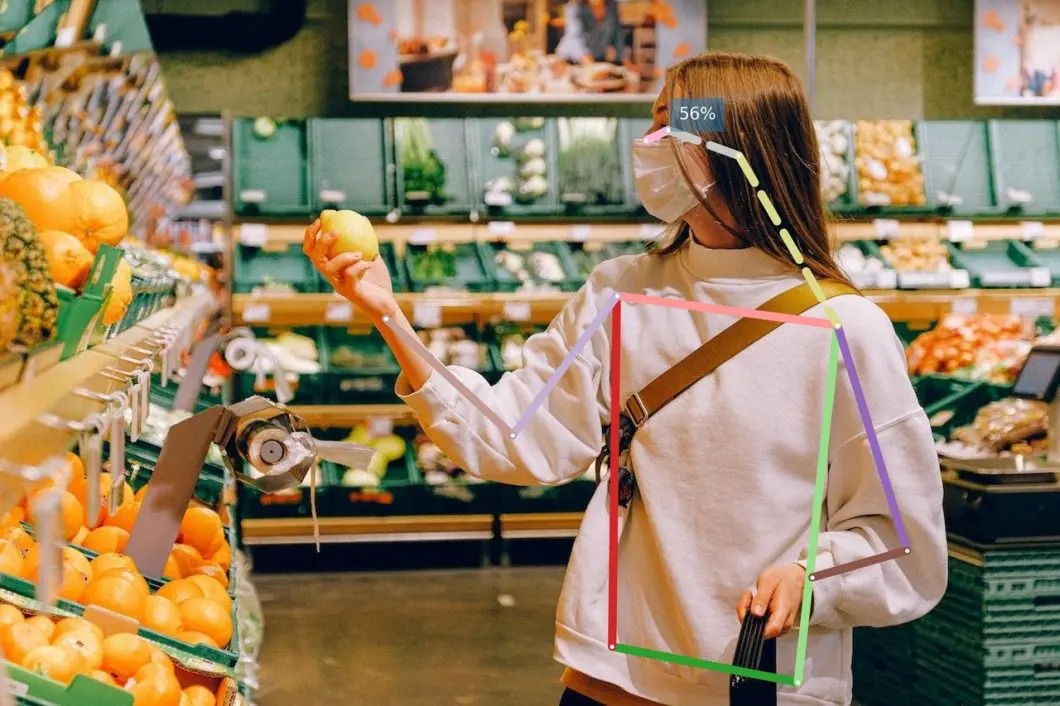
What is 2D Human Pose Estimation?
2D human pose estimation is used to estimate the 2D position or spatial location of human body keypoints from visuals such as images and videos. Traditional 2D human pose estimation methods use different hand-crafted feature extraction techniques for the individual body parts.
Early computer vision works described the human body as a stick figure to obtain global pose structures. However, modern deep learning-based approaches have achieved major breakthroughs by improving the performance significantly for both single-person and multi-person pose estimation. Some popular 2D human pose estimation methods include OpenPose, CPN, AlphaPose, and HRNet (we will cover them and others later in this article).
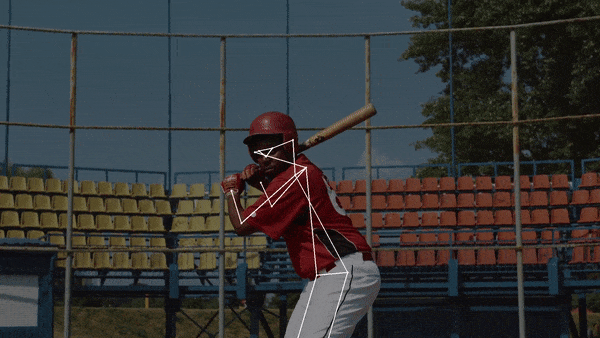
What is 3D Human Pose Estimation?
3D human pose estimation is used to predict the locations of body joints in 3D space. Besides the 3D pose, some methods also recover 3D human mesh from images or videos. This field has attracted much interest in recent years since it is used to provide extensive 3D structure information related to the human body.
It can be applied to various applications, such as 3D animation industries, virtual or augmented reality, and 3D action prediction. 3D human pose analysis can be performed on monocular images or videos (normal camera feeds).
Using multiple viewpoints or additional sensors (IMU or LiDAR), 3D pose estimation can be applied with information fusion techniques, which is a very challenging task. While 2D human datasets can be easily obtained, collecting accurate 3D pose image annotation is time-consuming, and manual labeling is not practical and expensive.
Therefore, although 3D pose tracking has made significant advancements in recent years, especially due to the progress made in 2D human pose estimation, there are still several challenges to overcome: Model generalization, robustness to occlusion, and computation efficiency.
A popular library that uses neural networks for real-time human pose estimation in 3D, even for multi-person use cases, is named OpenPose.
3D Human Body Modeling
In human pose estimation, the location of human body parts is used to build a human body representation (such as a body skeleton pose) from visual input data. Therefore, human body modeling is an important aspect of human pose estimation. It is used to represent features and key points extracted from visual input data. Typically, a model-based approach is used to describe and infer human body poses and render 2D or 3D poses.
Most methods use an N-joints rigid kinematic model where a human body is represented as an entity with joints and limbs, containing body kinematic structure and body shape information.
There are three types of models for human body modeling.
Kinematic Model
The Kinematic Model, also called the skeleton-based model, is used for 2D and 3D pose estimation. This flexible and intuitive human body model includes a set of joint positions and limb orientations to represent the human body structure. Therefore, skeleton pose estimation models are used to capture the relations between different body parts. However, kinematic models are limited in representing texture or shape information.
Planar Model
The Planar Model, or contour-based model, is used for 2D pose estimation. The planar models are used to represent the appearance and shape of a human body. Usually, body parts are represented by multiple rectangles approximating the human body contours. A popular example is the Active Shape Model (ASM), which is used to capture the full human body graph and the silhouette deformations using principal component analysis.
Volumetric Model
A volumetric model is used for 3D pose estimation. Multiple popular 3D human body models exist that are used for deep learning-based pose analysis for recovering 3D human mesh. For example, GHUM & GHUML(ite), are fully trainable end-to-end deep learning pipelines trained on a high-resolution dataset of full-body scans of over 60’000 human configurations to model statistical and articulated 3D human body shapes and poses.
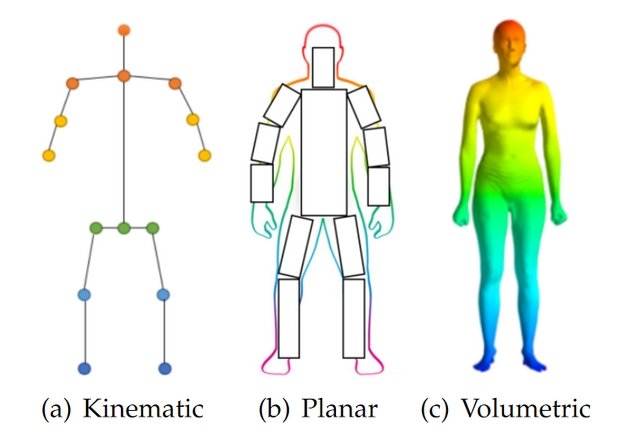
Main Challenges of Pose Detection
Detecting the human pose is a challenging task because the body’s appearance changes dynamically due to diverse forms of clothing, arbitrary occlusion, occlusions due to the viewing angle, and background contexts. Pose estimation needs to be robust to challenging real-world variations such as lighting and weather.
Therefore, it is challenging for image processing models to identify fine-grained joint coordinates. It is especially difficult to track small and barely visible joints.
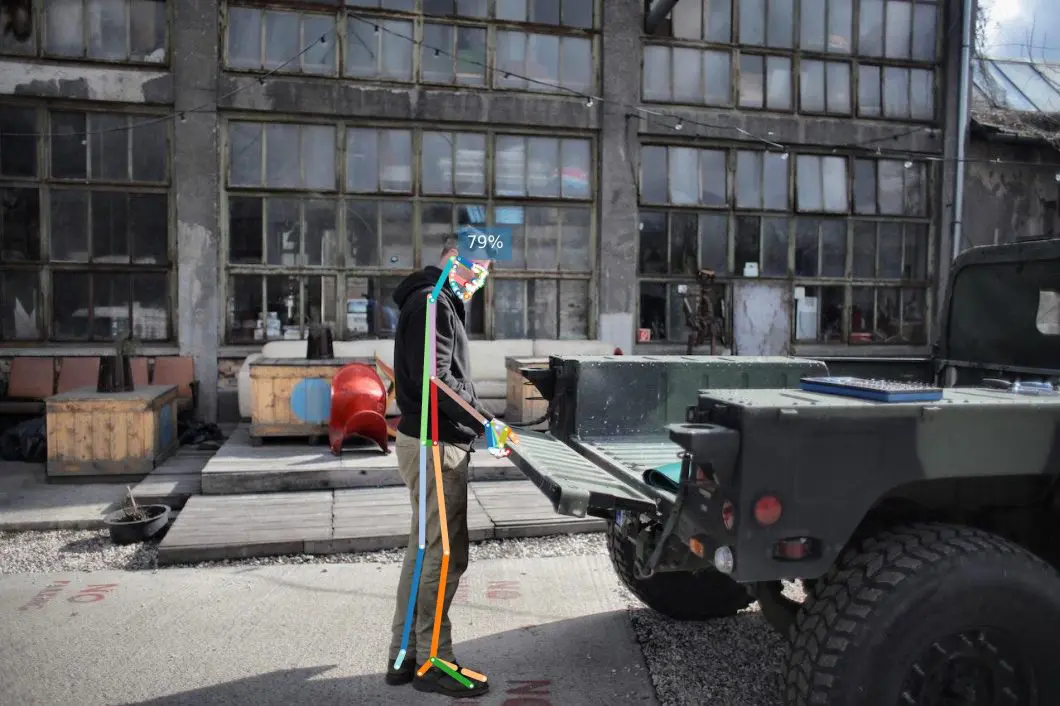
Head Pose Estimation
Estimating the head pose of a person is a popular computer vision problem. Head pose estimation has multiple applications, such as aiding in gaze estimation, modeling attention, fitting 3D models to video, and performing face alignment.
Traditionally head pose is computed with the use of keypoints from the target face and by solving the 2D to 3D pose correspondence problem with a mean human head model.
The ability to recover the 3D pose of the head is a by-product of keypoint-based facial expression analysis that is based on the extraction of 2D facial key points with deep learning methods. Those methods are robust to occlusions and extreme pose changes.
Animal Pose Estimation
Most state-of-the-art methods focus on human body pose detection and tracking. However, some models were developed to be used with animals and cars (object pose estimation).
Animal pose estimation comes with additional challenges, such as limited labeled data (need to collect data and annotate images manually) and a large number of self-occlusions. Therefore, datasets for animals are usually small and include limited animal species.
When working with limited data available and small datasets, active learning and data augmentation provide useful methods. Both techniques help to train vision algorithms more effectively and reduce the annotation work for custom AI model training.
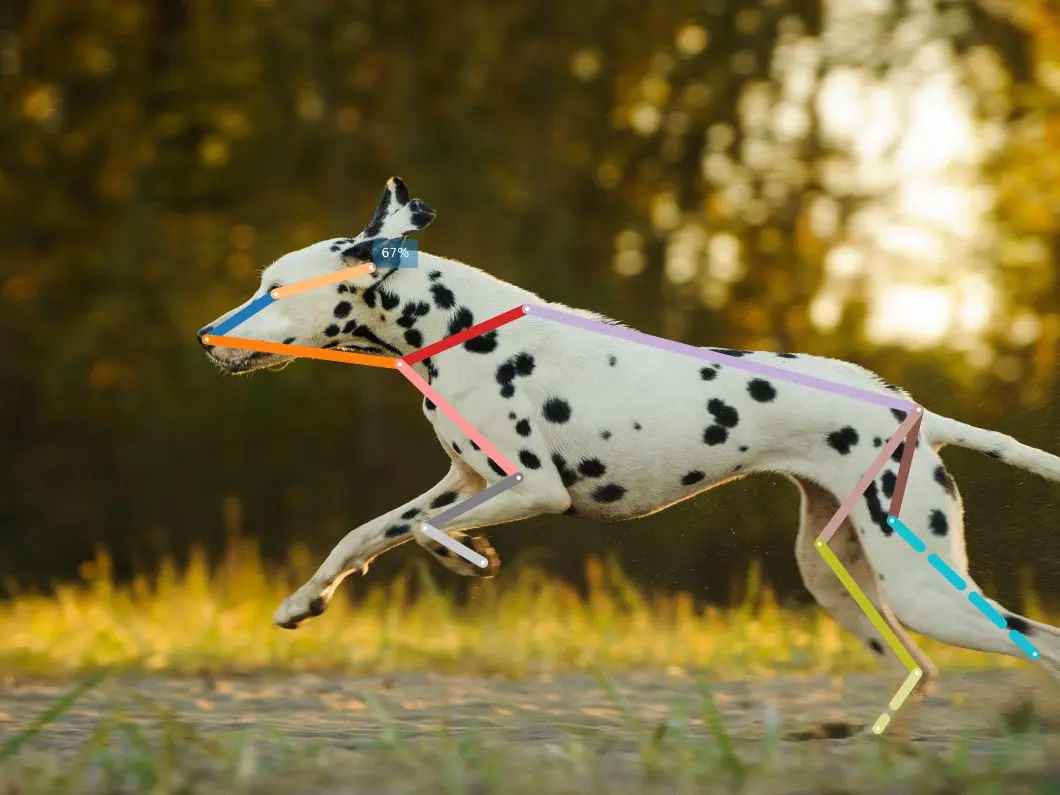
Also, estimating the pose of multiple animals is a challenging computer vision problem due to frequent interactions that cause occlusions and complicate assigning detected key points to the correct individual. Also, it is challenging to have very similar-looking animals that interact more closely than humans typically would.
To address those issues, transfer learning techniques have been developed to re-apply methods from humans to animals. An example is multi-animal movement tracking with DeepLabCut, a state-of-the-art, popular open-source pose estimation toolbox for animals and humans. Read more about animal pose tracking in our article about applications of computer vision in agriculture.
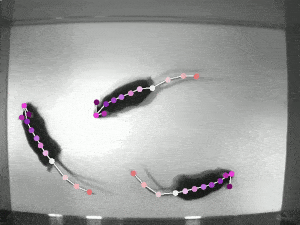
Video Person Pose Tracking
Multi-frame human pose estimation in complicated situations is complex and requires high computing power. While human joint detectors show good performance for static images, their performances often come short when the ML models are applied to video sequences for real-time pose tracking.
Some of the biggest challenges include handling motion blur, video defocus, pose occlusions, and the inability to capture temporal dependency among video frames.
Applying conventional Recurrent Neural Networks (RNN) incurs empirical difficulties in modeling spatial contexts, especially for dealing with pose occlusions. State-of-the-art multi-frame human pose estimation frameworks, such as DCPose, leverage abundant temporal cues between video frames to facilitate keypoint detection.
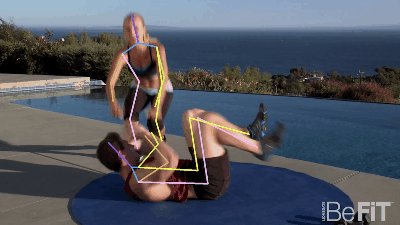
How Does Human Pose Estimation Work?
Pose estimation utilizes pose and orientation to predict and track the location of a person or object. Accordingly, the ML technique allows programs to estimate spatial positions (“poses”) of a body in an image or video. In general, most pose estimators are 2 steps frameworks that detect human bounding boxes and then estimate the pose within each box.
Pose estimation operates by finding key points of a person or object. Taking a person, for example, the key points would be joints like the elbow, knees, wrists, etc. There are two types: multi-pose and single-pose. Single-pose estimation is used to estimate the poses of a single object in a given scene, while multi-pose estimation is used when detecting poses for multiple objects.
Human pose estimation on the popular MS COCO Dataset can detect 17 different keypoints (classes). Each keypoint is annotated with three numbers (x,y,v), where x and y mark the coordinates, and v indicates if the keypoint is visible.
"nose", "left_eye", "right_eye", "left_ear", "right_ear", "left_shoulder", "right_shoulder", "left_elbow", "right_elbow", "left_wrist", "right_wrist", "left_hip", "right_hip", "left_knee", "right_knee", "left_ankle", "right_ankle"
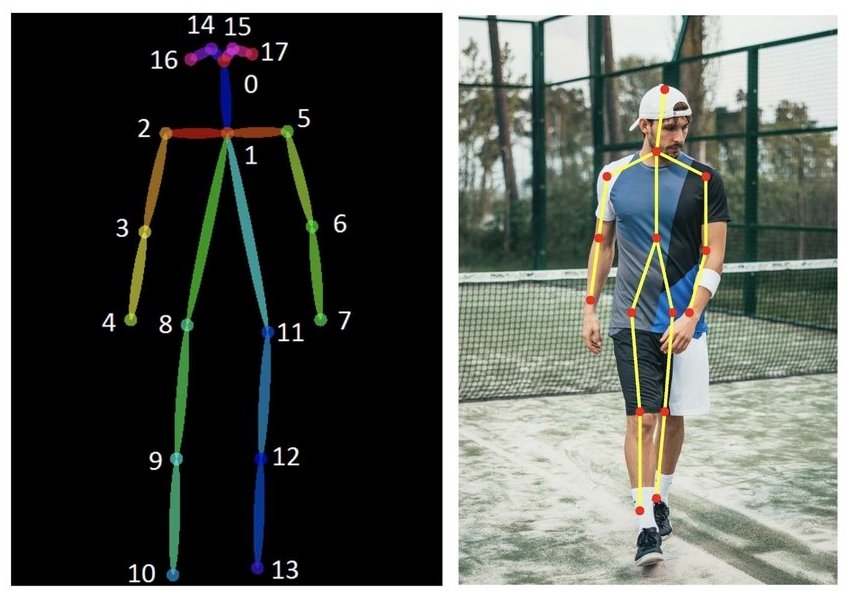
Human Pose Estimation with Deep Learning
With the rapid development of deep learning solutions in recent years, deep learning has been shown to outperform classical computer vision methods in various tasks, including image segmentation or object detection. Therefore, deep learning techniques brought significant advances and performance gains in pose estimation tasks.
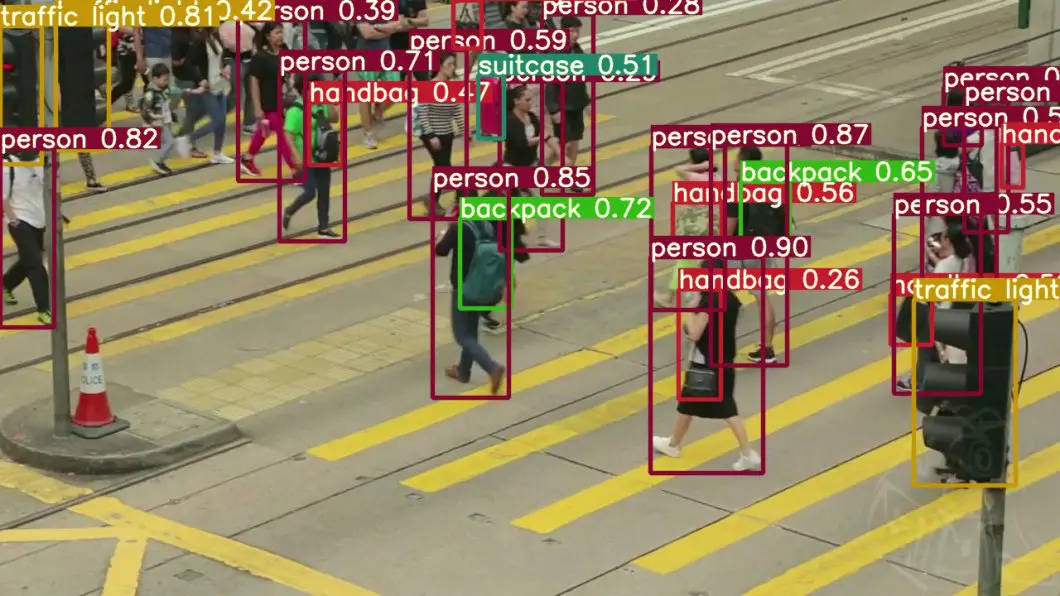
Next, we will list and review the popular human pose detection and tracking methods.
The Most popular Pose Estimation methods
- Method #1: OpenPose
- Method #2: High-Resolution Net (HRNet)
- Method #3: DeepCut
- Method #4: Regional Multi-Person Pose Estimation (AlphaPose)
- Method #5: Deep Pose
- Method #6: PoseNet
- Method #7: Dense Pose
- Method #8: TensorFlow
- Method #9: OpenPifPaf
- Method #10: YoloV8
Deep Learning based Pose Detection methods
Because pose estimation is an easily applicable computer vision technique, we can implement a custom pose estimator using existing architectures. The existing architectures for getting you started with developing a custom pose estimator include:
OpenPose
It is one of the most popular bottom-up approaches for real-time and multi-person pose estimation. OpenPose is an open-sourced framework that is suitable for achieving high accuracy in detecting body, foot, hand, and facial key points. An advantage of OpenPose is that it is an API that gives users the flexibility of selecting source images from camera fields, webcams, and others, more importantly for embedded system applications (for instance, integration with CCTV cameras and systems).
It supports different hardware architectures, such as CUDA GPUs, OpenCL GPUs, or CPU-only devices. The lightweight version is efficient enough for Edge inference applications with on-device processing in real-time with edge devices.
HRNet
High-Resolution Net (HRNet) is a neural network for human pose estimation. It is an architecture used in image processing problems to find what we know as key points (joints) for the specific object or person in an image. One advantage of this architecture over other architectures is that most existing methods match high-resolution representations of postures from low-resolution representations with respect to using high-low-resolution networks.
In place of this bias, the neural network maintains high-resolution representations when estimating postures. For instance, this HRNet architecture is helpful for the detection of human posture in televised sports.
DeepCut
This is another popular bottom-up approach for multi-person human pose estimation. DeepCut is used for detecting the poses of multiple people. The model works by detecting the number of people in an image and then predicting the joint locations for each image. DeepCut can be applied to videos or images with multi-persons/objects, for example, football, basketball, and more.
AlphaPose
Regional Multi-Person Pose Estimation (AlphaPose) is a popular top-down method of pose estimation. It is useful for detecting poses in the presence of inaccurate human bounding boxes. That is, it is an optimal architecture for estimating human poses via optimally detected bounding boxes. AlphaPose architecture is applicable for detecting both single and multi-person poses in images or video fields.
DeepPose
DeepPose is a human pose estimator that leverages the use of deep neural networks (DNN). The DNN of DeepPose captures all joints, hinges a pooling layer, a convolution layer, and a fully-connected layer to form part of these layers.
PoseNet
This is a pose estimator architecture built on tensorflow.js to run on lightweight devices such as browsers or mobile devices. Hence, PoseNet can be used to estimate either a single pose or multiple poses.
DensePose
This is a pose estimation technique that maps all human pixels of an RGB image to the 3D surface of the human body. DensePose can also be used for single and multiple-pose estimation problems.
TensorFlow
Tensorflow Lite provides pose estimation with a lightweight ML model optimized for low-power edge devices.
OpenPifPaf
OpenPifPaf is an open-source computer vision library and framework for pose understanding, which involves identifying and localizing human body parts in images or videos. It is built on top of the PyTorch deep learning framework and uses a multi-task learning approach to achieve accurate and efficient pose estimation. OpenPifPaf has gained popularity for its ease of use, robustness, and ability to handle challenging movement tracking scenarios, such as occlusion and cluttered backgrounds.
YOLOv8
These YOLOv8 pose models use the -pose suffix (for example, yolov8n-pose.pt). Those real-time optimized models are trained on the COCO key points dataset and are suitable for a variety of pose estimation tasks.
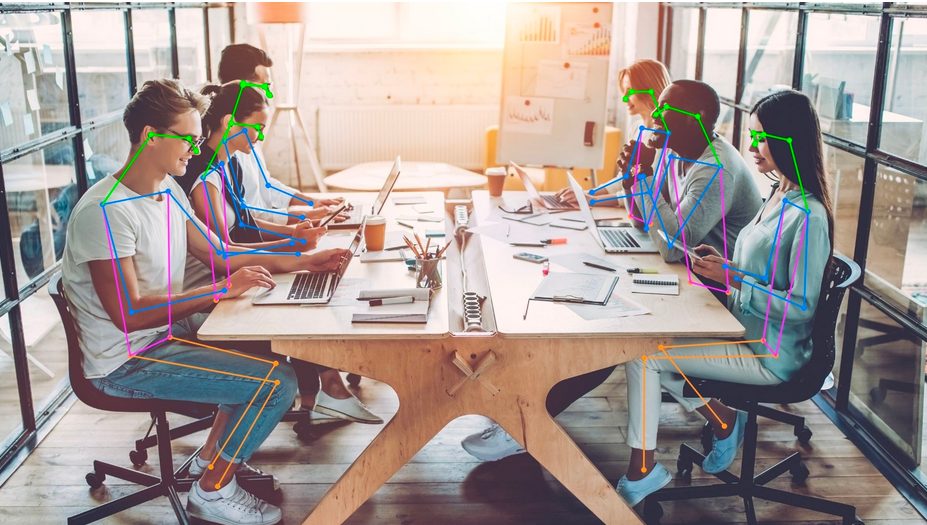
Use Cases and Applications of Human Pose Estimation
Human pose estimation has been utilized in a wide range of applications, including human-computer interaction, action recognition, motion capture, movement analysis, augmented reality, sports and fitness, and robotics. AI pose understanding enables applications in various fields and industries, some of which are listed below.
Most Popular Pose Estimation Applications
- Application #1: Human Activity Estimation
- Application #2: Motion Transfer and Augmented Reality
- Application #3: Motion Capture for Training Robots
- Application #4: Motion Tracking for Consoles
- Application #5: Human Fall Detection
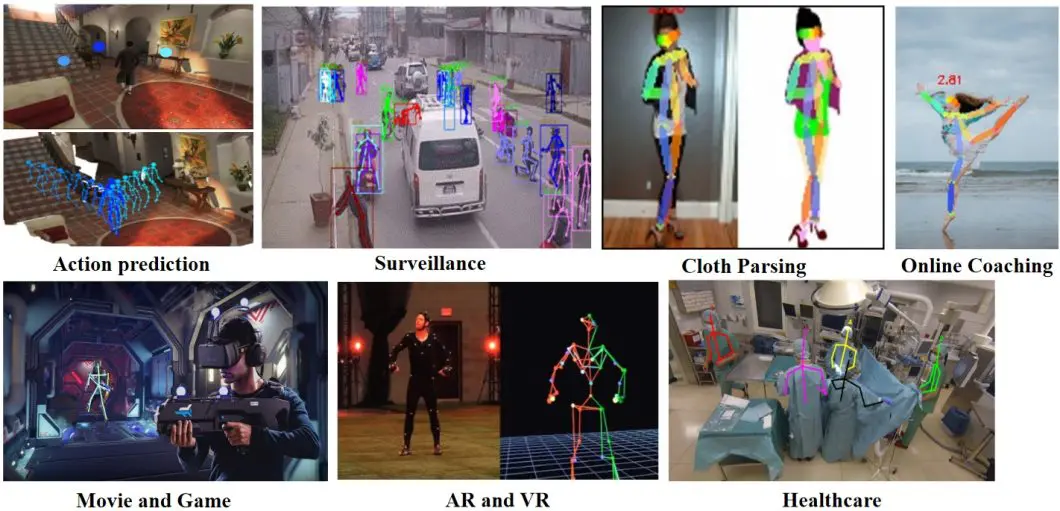
Human Activity Estimation
A rather obvious application of pose estimation is tracking and measuring human activity and movement. Architectures like DensePose or PoseNet are often used for activity, gesture, or gait recognition. Examples of human activity tracking via the use of pose estimation include:
- Application for detecting sitting gestures, recognizing hand gestures, or analyzing facial expressions
- AI-based analysis of players and athletes in football, basketball, cricket, etc.
- Applications to analyze dance techniques (for example, in ballet dances)
- Computer vision systems to assess the quality of surgeries in medical applications.
- Fitness applications to detect the execution form of exercises and count repetitions.
- Full body/sign language communication (for example, traffic policemen signals).
- Intelligent applications to detect people falling or the process of certain diseases.
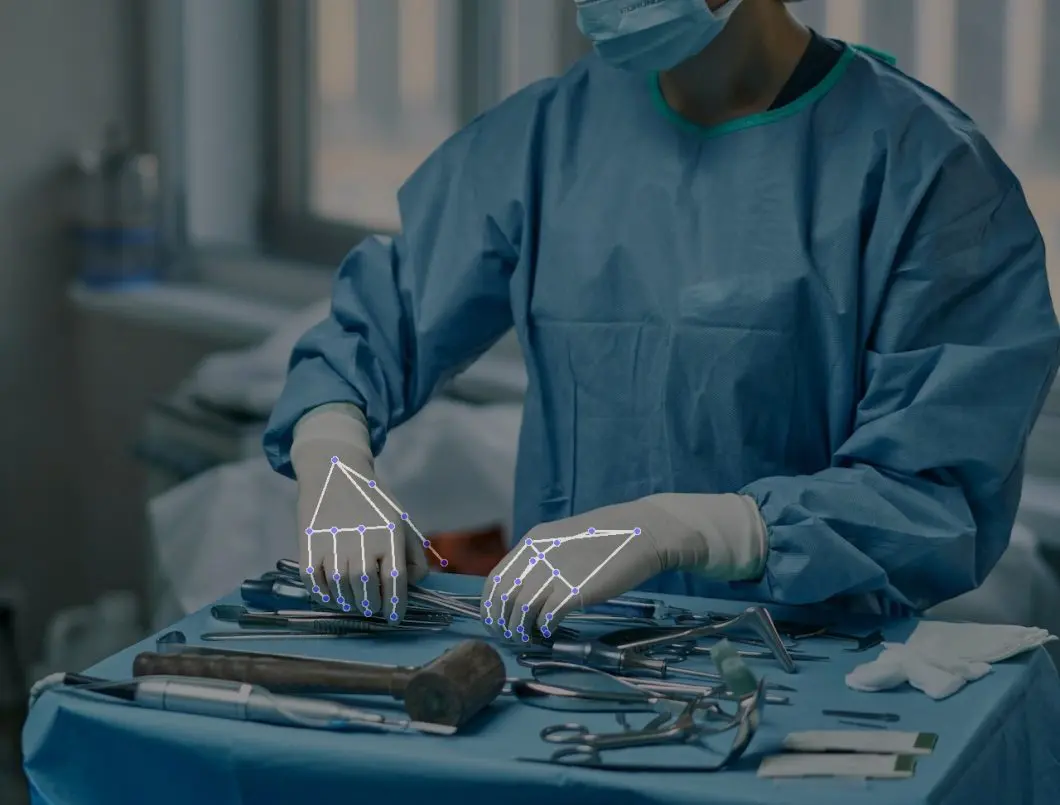
Augmented Reality and Virtual Reality
As of today, pose estimation interfaced with augmented and virtual reality applications gives users a better online experience. For instance, users can virtually learn how to play games like tennis via virtual tutors who are pose-represented.
More so, pose estimators can also be interfaced with augmented reality-based applications. For example, The United States Army experiments with augmented reality programs to be used in combat. These programs aim to help soldiers distinguish between enemies and friendly troops, as well as improve night vision.
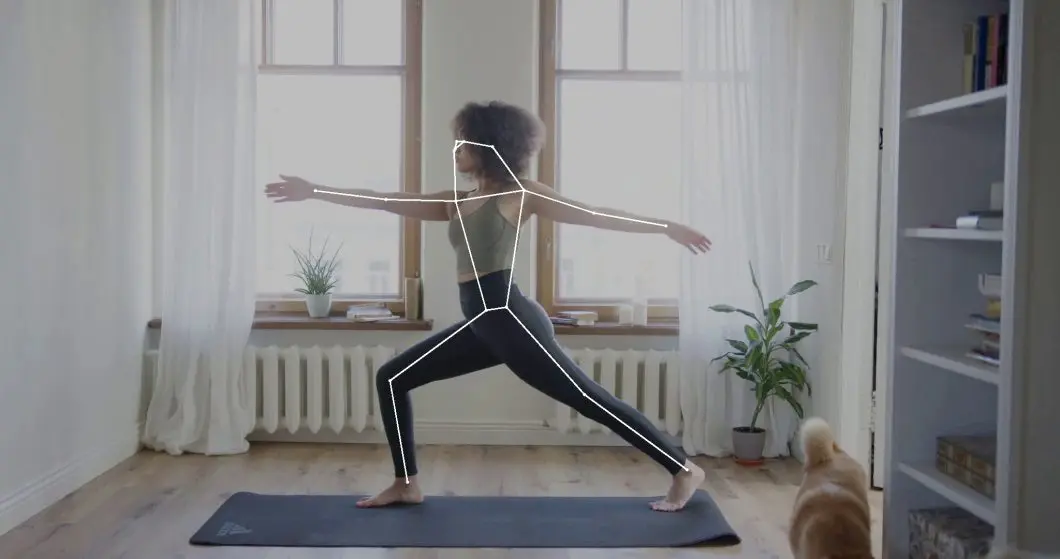
Training Robots With Human Pose Tracking
Typical use cases of pose estimators are in the application of making robots learn certain crafts. In place of manually programming robots to follow trajectories, robots can be made to learn actions and movements by following the tutor’s posture, look, or appearance.
Human Motion Tracking for Consoles
Other applications of pose estimation are in-game applications, where human subjects auto-generate and inject poses into the game environment for an interactive gaming experience. For instance, Microsoft’s Kinect used 3D pose estimation (using IR sensor data) to track the motion of the human players and to use it to render the actions of the characters virtually into the gaming environment.
Outlook and Future Trends
Pose estimation for objects is a major trend in computer vision. Object pose estimation allows a more detailed understanding of objects than two-dimensional bounding boxes. Until now, pose tracking is still computationally very intensive and requires expensive AI hardware (often multiple NVIDIA GPUs) that is not practical for real-world use.
Edge AI technology
New technologies and methods make it possible to decrease the size of AI models, making pose estimation algorithms less “heavy” and much more efficient. This is the basis for the real-world implementation of human pose detection.
As a result, it becomes possible to deploy pose estimation algorithms to edge devices and perform on-device machine learning (Edge AI). Edge Inference makes the technology scalable, more robust for mission-critical applications (offline capability), and private (no visuals need to be sent to the cloud). An example of a fast and efficient pose estimation model for Edge ML is Lightweight OpenPose.
How to get started
The enterprise computer vision platform Viso Suite makes it possible to build and deliver pose estimation systems rapidly and at scale. The end-to-end solution is used by global leaders to implement real-world pose estimation applications using common CCTV or USB cameras with various AI hardware and the latest processors.
What’s Next?
Pose estimation is a fascinating aspect of computer vision that can be applied in multiple fields, including technology, healthcare, business, and others. Aside from its prominence in modeling human characters via Deep Neural Networks that learn various key points, it is also used for security and surveillance systems.
Other popular applications of computer vision include image classification, image segmentation, face detection, and object detection.
If you enjoyed reading this article, we recommend exploring the following topics:
- Read our definitive guide to object detection
- View the most popular computer vision use cases
- Introduction to computer vision and what you need to know about it
- Learn about the latest AI hardware and AI accelerators