The Gartner Hype Cycle on AI presents the pace of AI development both today and in the future. It emphasizes the opportunities for innovation and the potential risks. Companies can use the hype cycle to adopt new technologies or avoid adopting AI too early, or waiting too long. Gartner Hype Cycle on AI includes 5 phases:
- Innovation Trigger – an occurrence of a technology or a product launch, that people start talking about.
- Peak of Inflated Expectations – when product adoption starts to increase, but there’s still more hype than proof that the innovation will deliver the company’s need.
- The Trough of Disillusionment – when the original excitement fades off and early adopters report performance issues and low ROI.
- The slope of Enlightenment – when early adopters see initial benefits and others start to understand how to adopt the innovation in their organizations.
- Plateau of Productivity – marks the point at which more users see real-world benefits, the innovation is widely accepted, and mainstream adoption is seen.
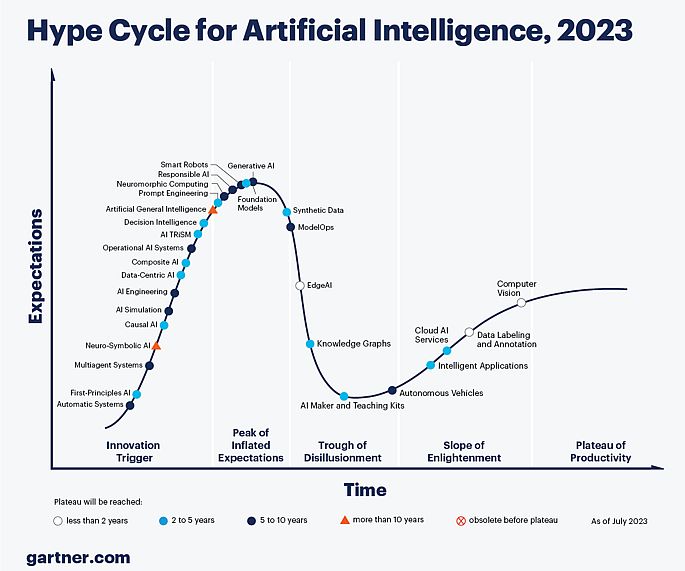
About us: Viso Suite is a flexible and scalable infrastructure developed for enterprises to integrate computer vision into their tech ecosystems seamlessly. Viso Suite allows enterprise ML teams to train, deploy, manage, and secure computer vision applications in a single interface. To learn more, book a demo with our team.
Hype Cycle Peaks and Predictions
To gain a bigger benefit, companies should plan future system architectures on composite AI techniques. They should adopt innovation services at all stages of the Hype Cycle.
In recent years we’ve faced a huge development in particular AI fields:
- Deep learning, based on Convolutional Neural Networks (CNNs), has enabled speech understanding and computer vision in our phones, cars, and homes.
- Due to AI and computer vision advancement, the gaming industry will surpass Hollywood as the biggest entertainment industry.
- Cognitive, or general AI aims to employ huge amounts of “static” data, to process that knowledge to solve real-life problems.
- Generative AI (ChatGPT) has surpassed expectations, although hype about it continues. In 2024, more value will derive from projects based on other AI techniques, (stand-alone or in combination with GenAI).
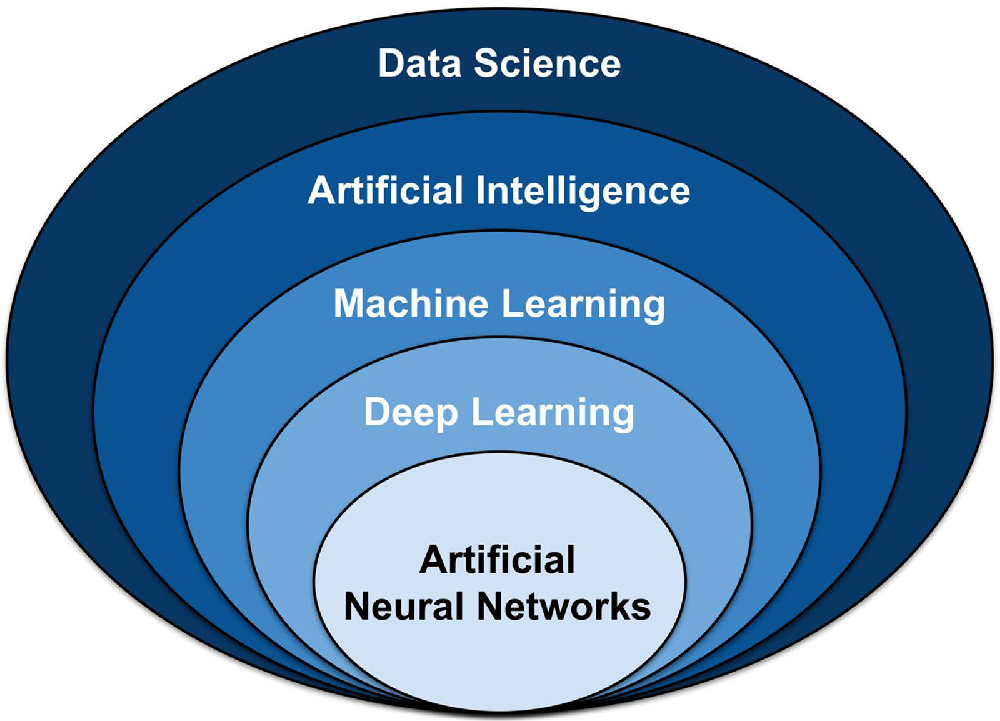
A year after ChatGPT’s debut, the AI market is still keeping strong. The appearance of text-to-video and text-to-music generation tools made consumer-related synthetic content tools advance further.
NVIDIA’s market cap launched it as the most valuable company (surpassing Amazon, Microsoft, and Apple). It is due to the global demand for its GPU chips designed to run large language models. At the moment, it appears that the AI bubble may continue to expand in the future.
State-of-the-Art in Different AI Fields
Generative AI (Nowadays)
AI tools have evolved and they can generate completely new texts, codes, images, and videos these days. GPT-4 (ChatGPT) has emerged as a leading exemplar of generative artificial intelligence systems within a short period.
The hype cycle states that it is often hard to recognize whether the content is created by man or machine. Generative AI is especially good and applicable in 3 major areas – text, images, and video generation.
GPT-4 model is trained on a large amount of multimodal data, including images and text from multiple domains and sources. This data is obtained from various public datasets, and the objective is to predict the next token in a document, given a sequence of previous tokens and images.
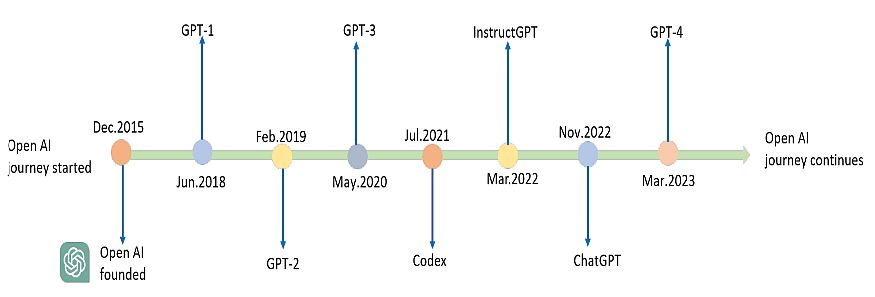
The GPT-4 model achieves human-level performance on the majority of professional and academic exams. Notably, it passes a simulated version of the Uniform Bar Examination with a score in the top 10% of test takers. Additionally, GPT-4 improves problem-solving capabilities by offering greater responsiveness with text generation that imitates the style and tone of the context.
Computer Vision (nowadays)
Computer vision (artificial sight) is the ability to recognize images and understand what is in them. It involves digital cameras, analog-to-digital conversion, and digital signal processing. After the image is taken, the particular steps within machine vision include:
- Image processing – stitching, filtering, and pixel counting.
- Segmentation – partitioning the image into multiple segments to simplify and/or change the representation of the image into something meaningful and easier to analyze.
- Blob checking – inspecting the image for discrete blobs of connected pixels (e.g. a black hole in a grey object) as image landmarks. These blobs frequently represent optical targets for observation, robotic capture, or manufacturing failure.
- Object detection algorithm includes template matching, i.e. finding and matching specific patterns using some Machine Learning method (neural network, deep learning, etc.). It also involves re-positioning of the object, or varying in size.
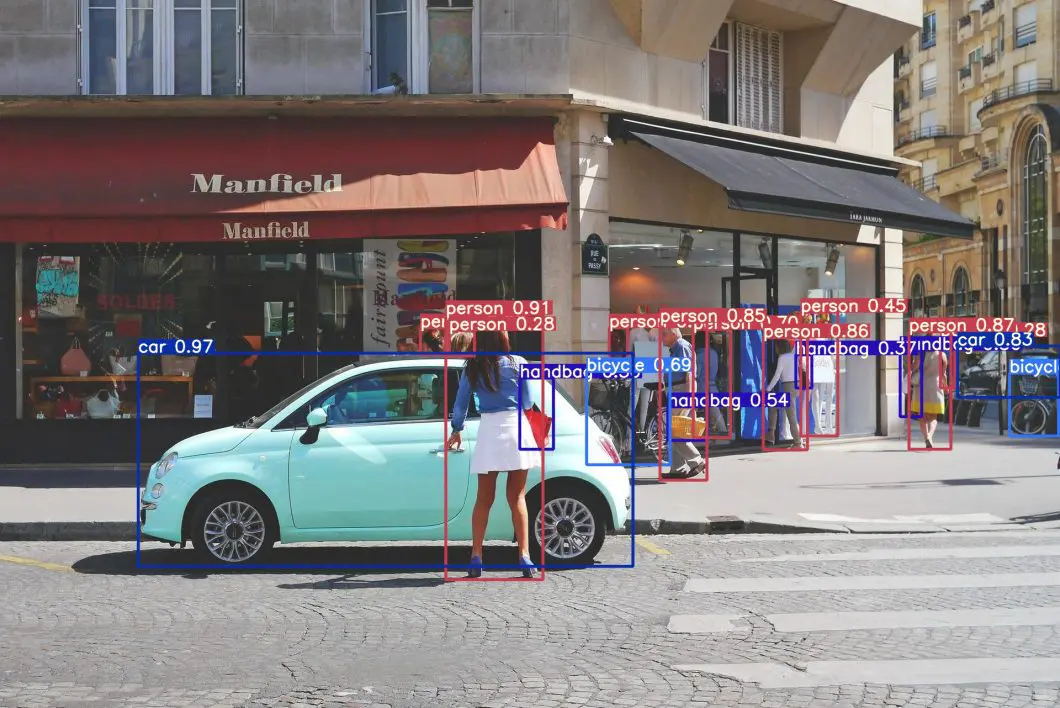
Deep Learning (nowadays)
Deep learning (DL) is a branch of machine learning based on complex data representations, at a higher degree of abstraction, by applying nonlinear transformations. DL methods are useful in areas of artificial intelligence such as computer vision, natural language processing, speech, sound comprehension, and bioinformatics.
This learning is based on advanced discriminative and generative deep models with particular emphasis on practical implementations. The key elements of deep learning are the classical neural networks, their building elements, regularization techniques, and deep model-specific learning methods.
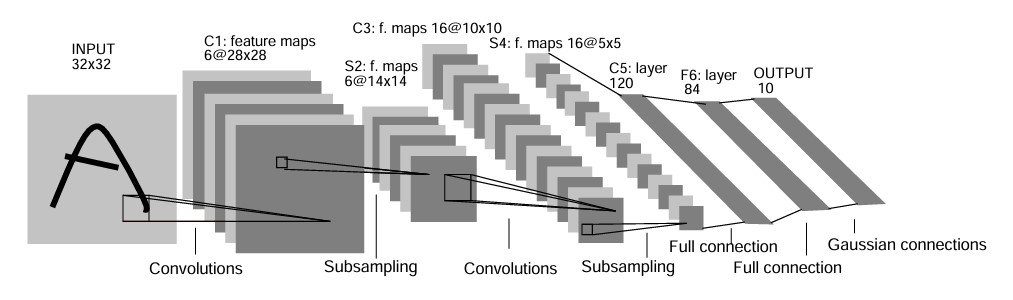
Additionally, image classification and natural language processing utilize Convolutional Neural Networks. All these techniques can lead to sequence modeling by deep feedback neural networks and create applications in robotics and self-driving cars.
Engineers implement deep learning methods using modern dynamic languages (Python, Lua, or Julia). Also, there are modern deep learning application frameworks (e.g. Theano, Tensor-flow, PyTorch).
General AI (Cognition) (~10 years)
Recently IBM has developed the IBM Watson Cognitive Computer, which is applicable in all areas, from making the most complex business decisions to the daily activities of the masses. In addition to its many abilities, Watson has won in the US Quiz TV show Jeopardy.
It’s one thing to learn a supercomputer to play chess, and something else to understand the complex strands of English sentences full of synonyms, slang, and logic, and to give the correct answer. The point is that Watson is not programmed.
For example, in cooking, after being “involved” with thousands of recipes, Chef Watson himself figures out which foods, spices, and other things go best and mix them. Then the model continues to study alone. It can also be a weather forecaster, airplane controller (pilot), chatbot, and many more.
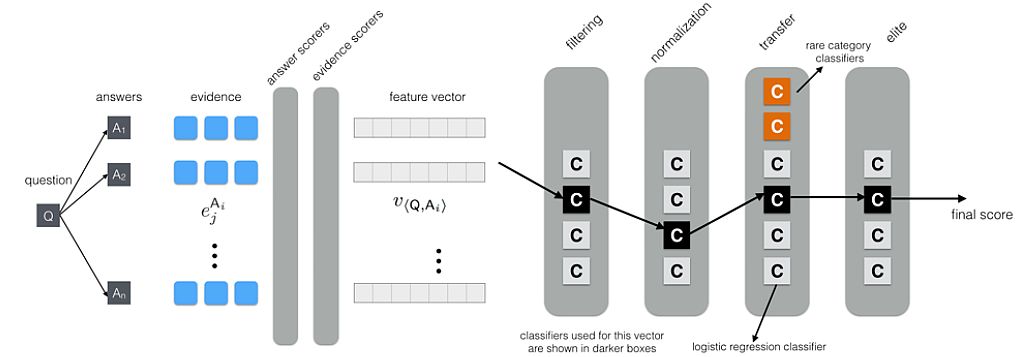
By developing these cognitive systems, IBM aims to extend human intelligence. Their technology, products, services, and policies will enhance and extend human capacity, expertise, and potential. Their attitude is based not only on principles but also on cognitive science.
The hype cycle on AI says: “Cognitive systems will not realistically reach consciousness or independent activity. Instead, they will increasingly be embedded in the processes, systems, products, and services through which business and society function, all of which are within human control.”
Emerging Technologies
Autonomous Driving (2-5 years)
Autonomous vehicles, also known as robotic vehicles or self-propelled vehicles, are motor vehicles that can move independently (i.e. without driver / human assistance) so that all real-time driving functions are being transferred to the so-called Vehicle Automation System.
This type of vehicle can perform all the steering and movement functions otherwise performed by a human being and can detect, and see the traffic environment, while the “driver” only needs to choose a destination and doesn’t have to perform any operation while driving.
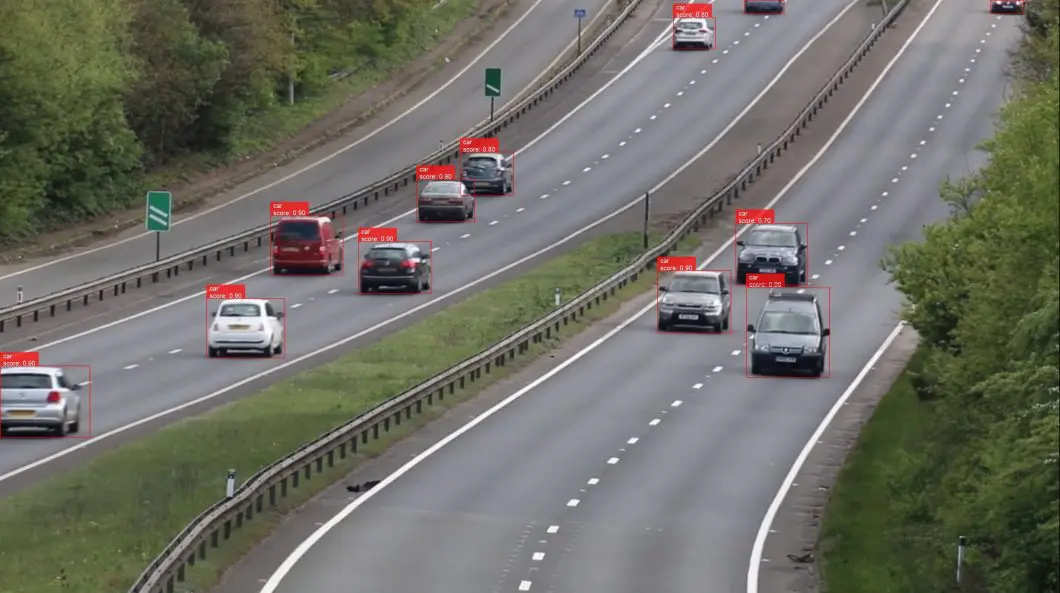
Video cameras, radar sensors, and laser range-finders can operate an autonomous vehicle independently. They can also see other road users, as well as download detailed maps. Google’s street view data allows the car to plan its route by understanding road maps and intersections.
The vehicle records the information it collects using ultrasonic sensors and cameras constantly from the environment. By processing images from video cameras, the autonomous vehicle control system detects the position of the vehicle concerning the marked lines on the road.
Humanoid Robotics (2-5 years)
The near future will bring us robots that are closely related to us, which can move, communicate, and feel as humans. In 2022 E. Musk presented the latest prototype of the Tesla bot humanoid robot. It belongs to a new class of humanoid robots that are applicable in homes and factories.
Tesla Bot is approximately the same size and weight as a human, weighing around 60 kg and height 170 cm. The robot can function for several hours without recharging. In addition, Optimus can follow verbal instructions to perform various tasks, including complex jobs such as picking up items.
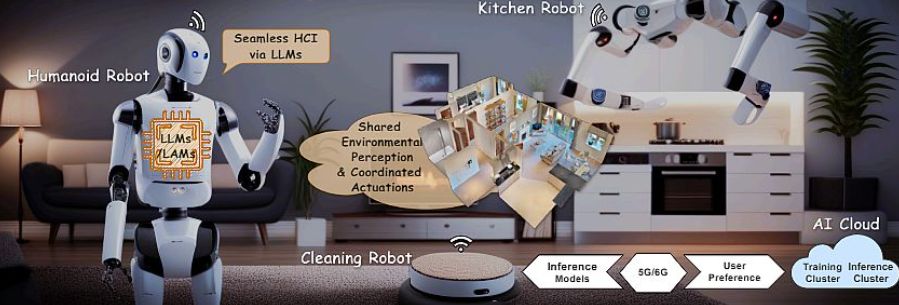
The Tesla bot has two legs and a maximum speed of 8km per hour. The Optimus incorporates 40 electromechanical actuators of which 12 are in the arms, 2 each in the neck and torso, 12 in the legs, and 12 in the hands.
Additionally, the robot has a screen on its face to present information needed in cognitive interaction. The robot contains some original Tesla features, such as a self-running computer, autopilot cameras, AI tools, neural network planning, auto-labeling for objects, etc.
Hype Cycle Dynamic
There are expert opinions that AI is a cornerstone technology that does not obey the regular innovation hype cycle.
Instead of just one strong peak of development, multiple peaks go through the peaks and drops. Though some generative AI applications might not work out and leave investors with empty hands, the development of AI technology will continue.
Although the AI hype cycle is similar to other cycles, the examination of market applicability indicates otherwise. It may be AI senility, or the weariness of novelty, but in some market areas, the indifference toward AI arises.
This opinion is not unjustified, since some firms and customers have started quick rate of AI research and have taken the developments for granted. They expect incremental improvements rather than revolutionary breakthroughs.
Additionally, the AI stakeholders are beginning to actively lower expectations, indicating that they are aware of the growing sense of weariness. Recently, Google and Amazon slowed down generative AI expectations, telling their sales teams to be less enthusiastic about the AI capabilities they are promoting.
What’s Next for Gartner Hype Cycle?
The concept of Artificial Intelligence was introduced in the 50s’ by John McCarthy and Marvin Minsky (MIT). Since then, numerous fields have emerged (e.g. neural networks), together with proper applications (speech recognition, computer vision, autonomous robotics).
However, it is booming nowadays due to the large advancement in processing power (multi-core processors), as well as the new software paradigms (deep learning, big data, Python).
Teams utilize AI to predict stock market conditions, insurance companies to predict the degree of risk, medicine for more accurate diagnoses, etc. Some expect that AI-based machines, (thanks to the ability to store and process incredibly large amounts of data), could solve major global crises, such as global warming.
Getting Started with Computer Vision
Viso Suite makes it possible for enterprise IT innovation teams to seamlessly integrate computer vision into their business processes. Our computer vision infrastructure omits the need for point solutions, placing the ability to manage the entire ML pipeline, including training, deploying, measuring, scaling, and securing. To learn more about Viso Suite, book a demo with our team of experts.
