Deep learning frameworks are used in the creation of machine learning and deep learning models. The frameworks offer tried and tested foundations for designing and training deep neural networks by simplifying machine learning algorithms.
These frameworks include interfaces, libraries, and tools that allow programmers to develop deep learning models more efficiently than coding them from scratch. They also provide concise ways for defining models using pre-built and optimized functions.
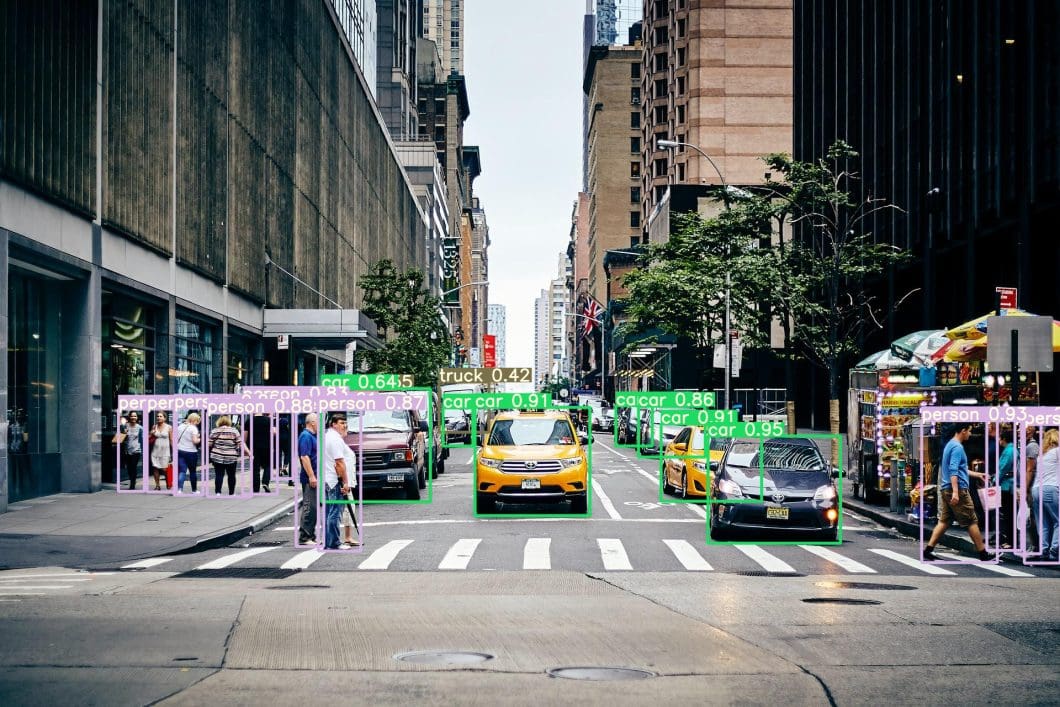
Deep Learning Frameworks
In addition to speeding up the process of creating machine or deep learning algorithms, the frameworks offer accurate and research-backed ways to do it, making the end product far more accurate than if one were to build the entirety of the model themselves.
This article will focus on the most important deep learning frameworks for data science:
TensorFlow
TensorFlow is an open-source, cost-free software library for machine learning and one of the most popular deep learning frameworks. It is coded almost entirely using Python. Developed by Google, it is specifically optimized for the inference and training of deep learning models. Deep learning inference is the process by which trained deep neural network models are used to make predictions about previously untested data.
TensorFlow allows developers to produce large-scale neural networks with many layers using data flow graphs. Hence, Tensorflow supports these large numerical computations by accepting data in the form of multidimensional arrays that host generalized vectors and matrices, called tensors.
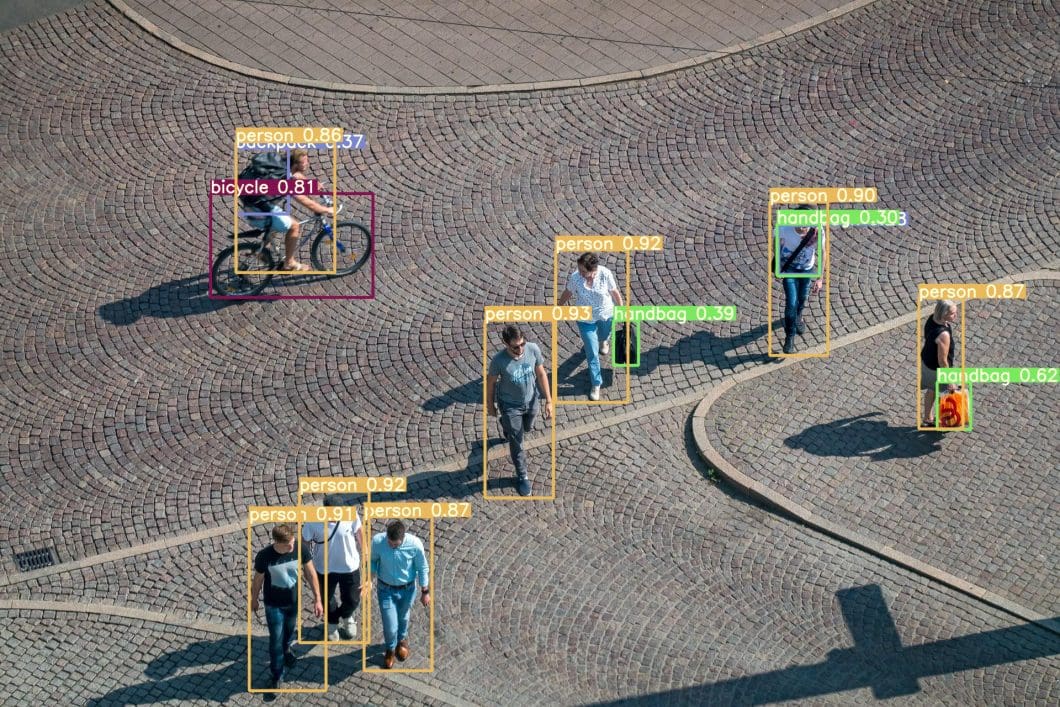
Keras
Keras is another very popular open-source deep learning library. The framework provides a Python interface for developing artificial neural networks. Keras acts as an interface for the TensorFlow library. It has been accredited as an easy-to-use, simplistic interface.
Keras is particularly useful because it can scale to large clusters of GPUs or entire TPU pods. Also, the functional API can handle models with non-linear topology, shared layers, and even multiple inputs or outputs. Keras was developed to enable fast experimentation for bulky models while prioritizing the developer experience, which is why the platform is so intuitive.
PyTorch
PyTorch is a Python library that supports building deep learning projects such as computer vision and natural language processing. It has two main features: Tensor computing (such as NumPy) with strong acceleration via GPU and deep neural networks built on top of a tape-based automatic differentiation system, which numerically evaluates the derivative of a function specified by a computer program.
PyTorch also includes the Optim and nn modules. The Optim module, torch.optim, uses different optimization algorithms used in neural networks. The nn module, or PyTorch autograd, lets you define computational graphs and also make gradients; this is useful because raw autograd can be low-level. Pytorch’s advantages over other deep learning frameworks include a short learning curve and data parallelism, where computational work is distributed among multiple CPU or GPU cores.
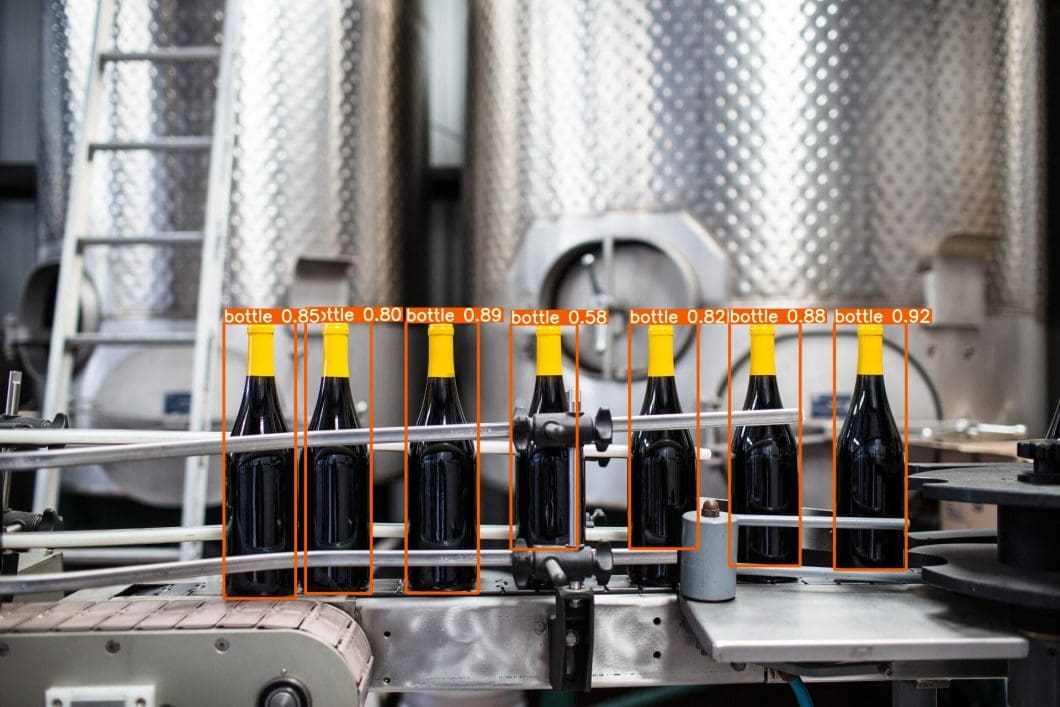
MXNet
Apache MXNet is an open-source deep learning framework designed to train and deploy deep neural networks. A distinguishing feature of MXNet, when compared to other frameworks, is its scalability (the measure of a system’s ability to increase or decrease in performance).
MXNet is also especially known for its ability to be flexible with multiple languages, unlike frameworks like Keras that only support one language. The supported languages include C++, Python, Julia, MATLAB, JavaScript, Go, R, and many more. However, it is not as widely used as other DL frameworks, which leaves it with a smaller open-source community.
Chainer
Chainer is a deep learning framework built on top of the NumPy and CuPy libraries. It is the first framework ever to implement a “define-by-run” approach, contrary to the more popular “define-and-run” approach.
The “define-and-run” scheme first defines and fixes a network, and the user continually feeds it with small batches of training data. However, the “define-by-run” means that Chainer stores the history of computation in a network rather than the actual programming logic.
Following Chainer, other frameworks such as PyTorch and TensorFlow now implement the “define-by-run” approach. Chainer also supports Nvidia CUDA computation, which has several advantages over traditional general-purpose computation on GPUs using graphics APIs.
Caffe
Caffe (Convolutional Architecture for Fast Feature Embedding) is a deep learning framework developed at the University of California, Berkeley. It is open-source software that is written in C++, with a Python interface and is licensed under the BSD license. The Caffe project was created by Yangqing Jia during his Ph.D. at UC Berkeley and is publicly available on GitHub.
Caffe is widely used for computer vision tasks, particularly for image classification and image segmentation. It supports a variety of deep learning architectures, including Convolutional Neural Networks (CNNs), Recurrent Neural Networks (RNNs), Long Short-Term Memory (LSTM) networks, and fully connected neural networks. The framework is designed for speed and efficiency, and utilizes GPU- and CPU-accelerated libraries, such as NVIDIA cuDNN and Intel MKL, to process large amounts of data quickly.
Caffe is used in a range of applications, from academic research projects to startup prototypes and large-scale industrial applications in the areas of vision, speech, and multimedia. The integration of Caffe with Apache Spark has created CaffeOnSpark, a distributed deep learning framework developed by Yahoo.
In 2017, Facebook introduced Caffe2, a deep learning framework that included new features such as support for Recurrent Neural Networks. However, in 2018, Caffe2 was merged into PyTorch, another popular deep learning framework. Despite this, Caffe continues to be widely used and remains a popular choice for computer vision tasks and other deep learning projects.
Theano
Theano is a powerful deep learning tool that allows for efficient manipulation and evaluation of mathematical expressions, especially matrix-valued ones. It is an open-source project developed by the Montreal Institute for Learning Algorithms (MILA) at the Université de Montréal and is written in Python with a NumPy-like syntax. Theano can compile computations to run efficiently on either CPU or GPU architectures. In 2017, major development of Theano ceased due to competition from other strong industrial players, but it was taken over by the PyMC development team and renamed Aesara.
Theano is used for various deep learning tasks, including image classification, natural language processing (NLP), and speech recognition. It supports a range of deep learning architectures, including Convolutional Neural Networks (CNNs), Recurrent Neural Networks (RNNs), and Autoencoders. Theano also includes tools for visualization and debugging, making it easier for users to design and validate their models.
One of the main features of Theano is its ability to optimize the computation of mathematical expressions. Theano can automatically optimize the use of available hardware resources, including CPU and GPU, to speed up computations. Theano also includes features for controlling the use of memory, making it possible to handle large datasets and models.
Deeplearning4j
Deeplearning4J is a set of projects for building JVM-based deep learning applications, supporting data preprocessing and model building/tuning. It includes high-level API (DL4J) for building MultiLayerNetworks and ComputationGraphs, a general purpose linear algebra library (ND4J), automatic differentiation/deep learning framework (SameDiff), ETL for machine learning data (DataVec), C++ library (LibND4J), and bundled cpython execution (Python4J).
The suite of tools supports multiple JVM languages and AI hardware for running deep learning, including CUDA GPUs, x86 CPU, ARM CPU, and PowerPC.
CNTK
Microsoft Cognitive Toolkit (CNTK) is an open-source deep-learning toolkit for building, training, and evaluating neural networks. It allows for the combination of popular model types, such as feed-forward DNNs, CNNs, and RNNs/LSTMs, and uses SGD learning with automatic differentiation and parallelization across multiple GPUs and servers. It was released under an open-source license in April 2015.
CNTK supports multiple languages, including C++, Python, and BrainScript, a custom language designed for building and describing neural networks. It is designed to scale efficiently in a multi-GPU and multi-machine environment, ideal for large-scale training and model deployment.
The DL tool suite has been used in several industry applications, including speech recognition, image recognition, and natural language processing (NLP). It also offers support for several popular deep learning frameworks, including TensorFlow, Keras, and PyTorch, allowing for easy integration with other deep learning tools. In addition, CNTK includes some pre-trained models and tutorials to help users get started with their deep learning projects.
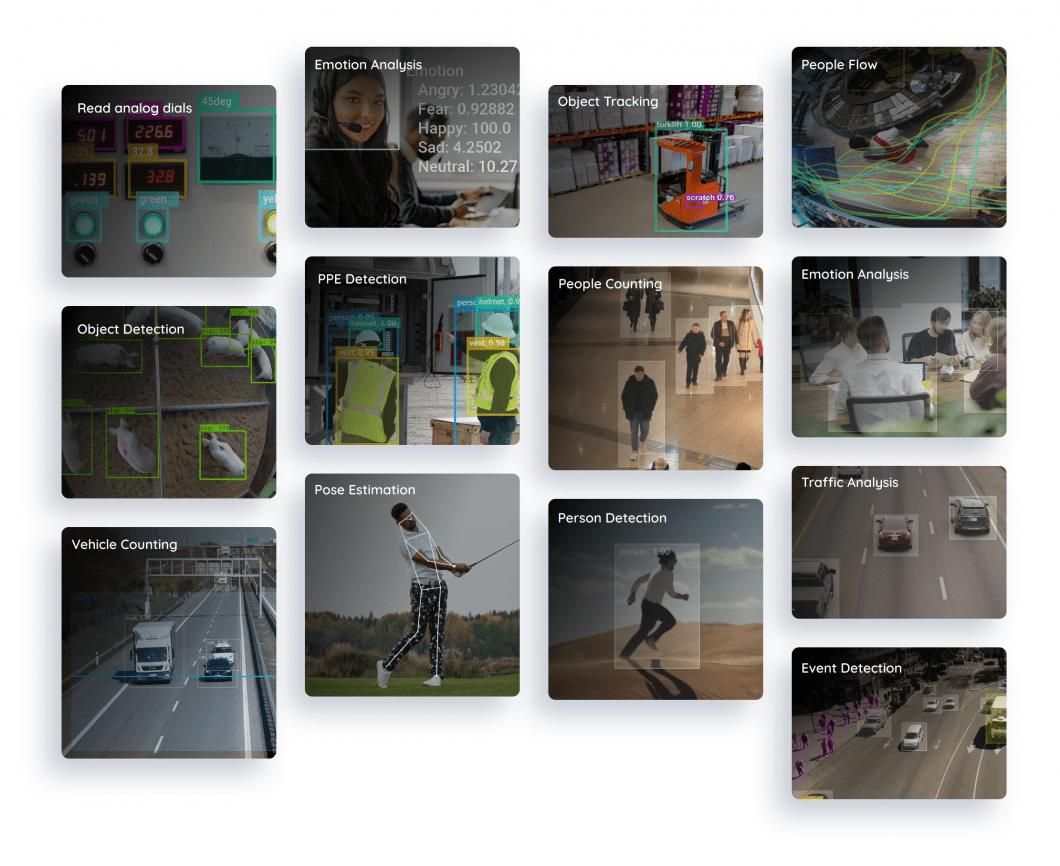
Torch
Torch is a scientific computing framework that provides a wide range of algorithms for deep learning. It is open-source and based on the Lua programming language. Torch uses the scripting language LuaJIT and has an underlying C implementation. It was developed at the IDIAP research institute at the École Polytechnique Fédérale de Lausanne (EPFL).
Torch is a powerful deep learning framework that provides a rich collection of algorithms for various applications. It is designed for research and production and has been used in various computer vision, natural language processing, and speech recognition tasks.
With its ease of use, modular design, and efficient implementation, Torch makes it simple to build complex AI models, perform dynamic computation on tensors, and deploy models to multiple platforms, including GPUs and mobile devices. It also integrates with popular tools and libraries such as CUDA, cuDNN, and Numpy, and supports popular datasets, including MNIST, CIFAR, and ImageNet.
What’s Next?
The field of deep learning is evolving fast, and there are many high-performance tools available to choose from. The top 10 deep learning tools, including TensorFlow, PyTorch, Caffe, Keras, Theano, Microsoft Cognitive Toolkit, Torch, Deeplearning4J, CNTK, and Torch, all offer unique features and capabilities.
The choice of tool ultimately depends on the specific needs of the user, such as the type of problem they are trying to solve, their preferred programming language, and the hardware they have available. Regardless of the tool chosen, deep learning is set to continue to be an important and rapidly growing field in the world of artificial intelligence.
Enterprise Deep Learning Platform
Viso Suite is designed for enterprises and organizations seeking to leverage deep learning to solve practical challenges. The computer vision platform provides an end-to-end solution with automated application development features for computer vision and integrates all necessary deep learning tools for seamless implementation.
The all-in-one solution makes it an ideal choice for organizations looking to rapidly implement scalable deep learning solutions. A recent ROI study has shown substantial benefits of delivering computer vision with an automated infrastructure of Viso Suite.
Explore related topics: