Today’s conservation efforts face several challenges that lay the foundation for the need for computer vision and Artificial Intelligence (AI). Not only are researchers faced with the enormous complexity of natural and environmental systems, but also the sheer scope and diversity of systems across the Earth. Advances in computer vision promise greater efficiency, accuracy, and lower risk for researchers and workers in this field.
Why use AI and computer vision in environmental conservation?
- Accessibility and operational efficiency: Research and monitoring efforts often focus on remote or extreme environments. This requires sending human researchers on costly and risky expeditions to gather insights. However, computer vision systems can be integrated into systems such as weather balloons, drones, or other probes to identify points of interest and collect relevant data without having any human presence.
- Accuracy and precision: Human error is a common problem that occurs when vast amounts of data must be processed. However, AI systems are impartial and use consistent metrics to analyze data, minimizing the risk. In environmental conservation applications, researchers often need to analyze large volumes of complex and disparate data, such as counting populations, identifying species, or monitoring changes in foliage. This makes it a prime use case for AI systems.
- Long-term trend analysis: AI and computer vision systems can efficiently analyze and make predictions from vast amounts of historical data. This is vital for understanding long-term trends, such as deforestation or ecosystem recovery rates. Having individual researchers continually take a look over years and years of data is extremely resource-intensive and slow.
- Data integration: Conservation and monitoring efforts often rely on collecting and collating data from many different sources. Once again, AI and computer vision systems are more efficient and accurate at this than humans. This allows analysts and conservation organizations to make informed decisions based on large-scale data collection.
Computer vision applications in many of these areas are still a nascent technology, and its potential is only now beginning to be explored for environmental conservation AI. However, many advancements in artificial intelligence in wildlife conservation applications are taking place to improve the accuracy and utility of these systems.
Application 1: Wildlife monitoring and protection
Wildlife monitoring and protection are often hampered by the diversity of animal populations and the complexity of their movement patterns. Wildlife can exist in remote, inaccessible, or dangerous locations where it’s difficult for researchers to spend prolonged hours actively monitoring them. Plus, the wildlife itself might be dangerous to observe closely.
However, constant monitoring is necessary for biologists and scientists to understand or track,
- Poaching activities
- Instinctive behaviors
- Habitats
- Recording the wildlife population.
Camera traps are a low-cost, effective, and non-intrusive form of wildlife monitoring. Combined with AI and computer vision technologies, it can also make these efforts more efficient and effective.
Researchers can monitor important events in real time by integrating object recognition technologies and alert systems.
Kaziranga National Park in India (a UNESCO World Heritage Site) is a critical habitat for endangered species, such as the Bengal Tiger. The park deployed motion-sensitive cameras with automatic sensors to count its tiger population, capture exciting animal behavior, and inform anti-poaching activities.
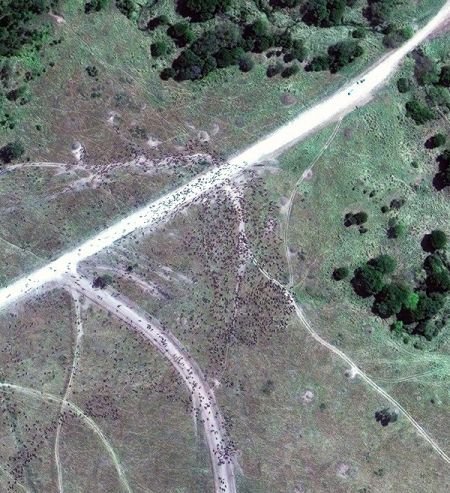
In other applications, imaging satellites combined with AI and machine learning algorithms can capture and monitor the migratory patterns of wildlife herds. This allows researchers to identify and monitor wildlife movements, patterns, species numbers, and behaviors, and to prevent poaching.
Application 2: Ocean and marine life conservation
Monitoring and conserving marine life have many of the same difficulties as land-based efforts. However, with 71% of the Earth’s surface covered in water and the difficulty of exploring deep-sea locations, the scope of the challenges is significantly higher.
Luckily, many of the same technologies, such as drones, satellite imagery, underwater camera traps, etc., can be used to monitor large and small-scale events and trends.
However, ROVs (Remotely Operated Vehicles) are particularly useful in these situations. ROVs are sometimes completely autonomous or sometimes operated by humans from a ship or the shore. Often, they are outfitted with a range of sensors, including a camera, to help identify underwater objects of interest.
However, because underwater conditions can be challenging for human sight, additional computer vision tools can help improve the accuracy and efficiency of monitoring species and environments.
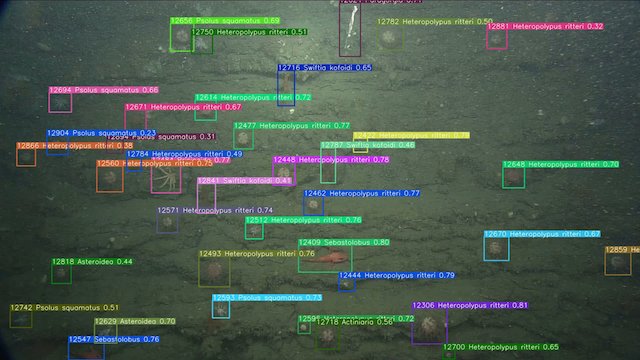
For example, The Monterey Bay Aquarium Research Institute (MBARI) operates a fleet of ROVs for oceanographic research. It was recently awarded $5 million for Ocean Vision AI/Machine Learning computer vision system for ocean video and imagery. It uses trained deep-learning models and the open-source database FathomNet to provide expert-level classifications.
Another example application is using image processing AI technologies for automatic fish counting. This is an efficient method of monitoring wildlife populations and minimizing effort for fishery operations.
Application 3: Forest conservation efforts
Researchers can attach computer vision systems to satellites to collect and process images of forest coverage. These systems can automatically detect forested areas and analyze imagery to make inferences based on visual cues.
This allows researchers to monitor changes, which may be due to:
- Illegal or industrial logging
- Livestock grazing, or
- Other forms of degradation, are due to disease, pests, etc.
By monitoring visual changes over time, these systems can establish the rate of change, its severity, and its ecological impact. With change detection algorithms and real-time alerts, computer vision systems can dramatically accelerate detection. This speeds up response times, which typically improves the outcomes of any intervention methods.
For example, the Amazon Conservation Association (ACA) has successfully used drones and satellites armed with computer vision to monitor changes in the Amazon rainforest. Through its Monitoring of the Amazon Andean Project (MAAP), it can track factors such as:
- Illegal roads
- Deforestation due to mining
- Carbon levels
- Forest fires
- Indigenous and protected areas
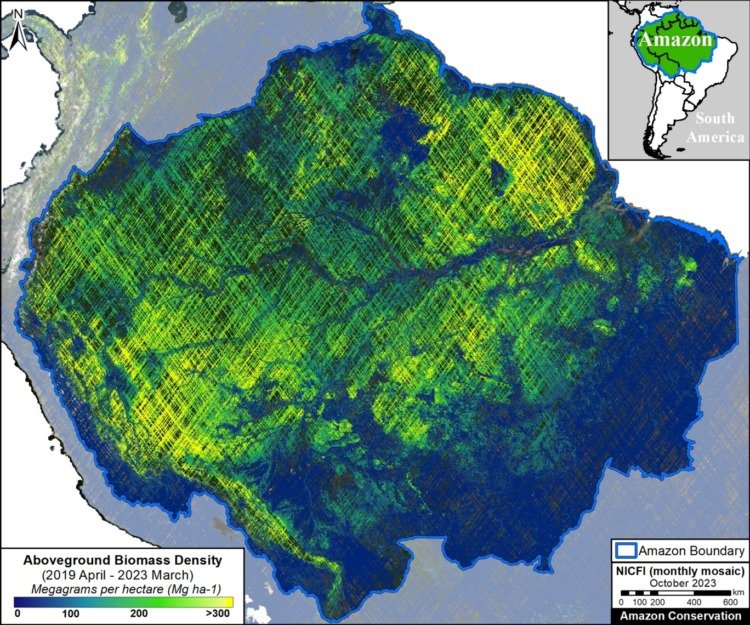
Application 4: Pollution detection and control
Pollution is a topic of immense concern today because of its impact on humans, the environment, and climate change. It’s vital that researchers can identify potential sources of pollution or “hotspots” where it’s particularly intense. Different types of pollutants can have different impacts, so it’s important to identify and differentiate them.
From the perspective of enforcement and remedial action, it’s also important that researchers can detect pollutive activities, such as illegal dumping, in real time.
A mix of drones, satellites, fixed cameras, and other systems are already being used in this regard. However, there is still no widescale adoption of AI/ML systems to help improve and streamline pollution monitoring and detection. The current researcher is focused on assessing the performance of computer vision models in accurately identifying and classifying pollution.
For example, the study “An end-to-end pollution analysis and detection system using artificial intelligence and object detection algorithms” compares the Faster R-CNN, YOLO, and EfficientNet models. It weighed their performances using common object detection metrics, like non-max suppression, precision and recall, F1 score, and intersection over union.
All models achieved a precision of over 80%, with only slightly lower recall and F1 scores. From the data, they were able to construct a heatmap that indicates pollution hotspots overlayed over a visual map. This shows the potential of these computer vision systems to help the authorities get a holistic view of the scale and impact of pollution in specific areas.
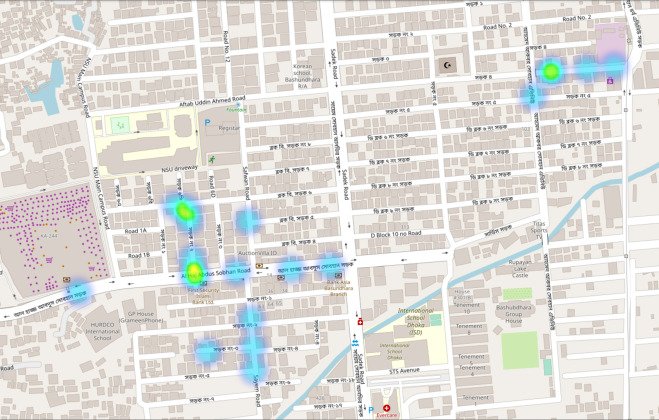
Other studies use similar techniques for spill and waste detection in coastal or port waters and trends in energy consumption.
Application 5: Agriculture operations and minimizing environmental impact
The agricultural sector continues to benefit from a wide variety of computer vision technologies. Agricultural applications optimize farming practices and minimize their impact on the surrounding environment.
In terms of smart farming, practitioners often use AI and computer vision to assist with:
- Livestock, poultry, or aquaculture monitoring
- Crop monitoring and yield estimation
- Security and compliance monitoring
- Analyzing and predicting weather and climate patterns
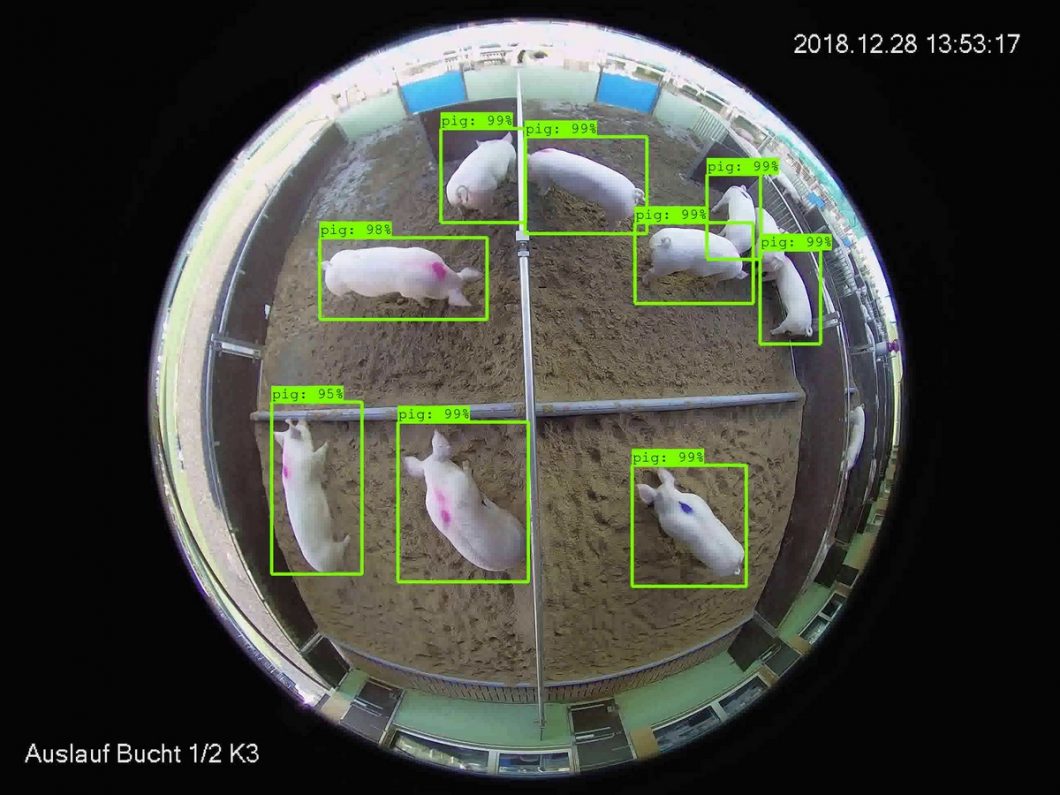
However, these same systems can help minimize agriculture’s impact on the environment. For example, farms are often located in areas designated for their natural diversity or wildlife. Pesticides, free grazing, and extensification can all negatively impact or completely destroy these natural treasures.
Many of the computer vision technologies we’ve discussed can help agricultural planners and farmers identify sensitive wildlife populations or ecological areas and inform conservation efforts.
However, with this information, steps can be taken to minimize harm. This preserves the environment and helps farmers avoid breaching protection laws, which could result in fines or civil and criminal action.
Application 6: Climate change impact assessment
Accurately analyzing the impact of climate change is challenging due to the long timescale and complexity of its manifestations. This is where computer vision systems come in handy, observing indicators across vast scales and prolonged periods.
For example, researchers already use these systems to monitor a wide spectrum of potential markers of climate change, such as:
- Melting glaciers
- Sea-level fluctuations
- Changes in snow cover on a seasonal and annual basis
- The health of coral reefs
- The occurrence of floods or other natural disasters
- The carbon footprint of specific activities
By monitoring changes in the severity and frequency of these indicators, scientists can better understand the potential impact of climate change. This helps in making accurate long-term predictions for the future and formulating more effective mitigation strategies.
Using satellite imagery to track changes in arctic ice coverage has been practiced for decades. However, modern research efforts focus on making more precise predictions using AI-powered computer vision models to measure the properties of and classify ice populations.
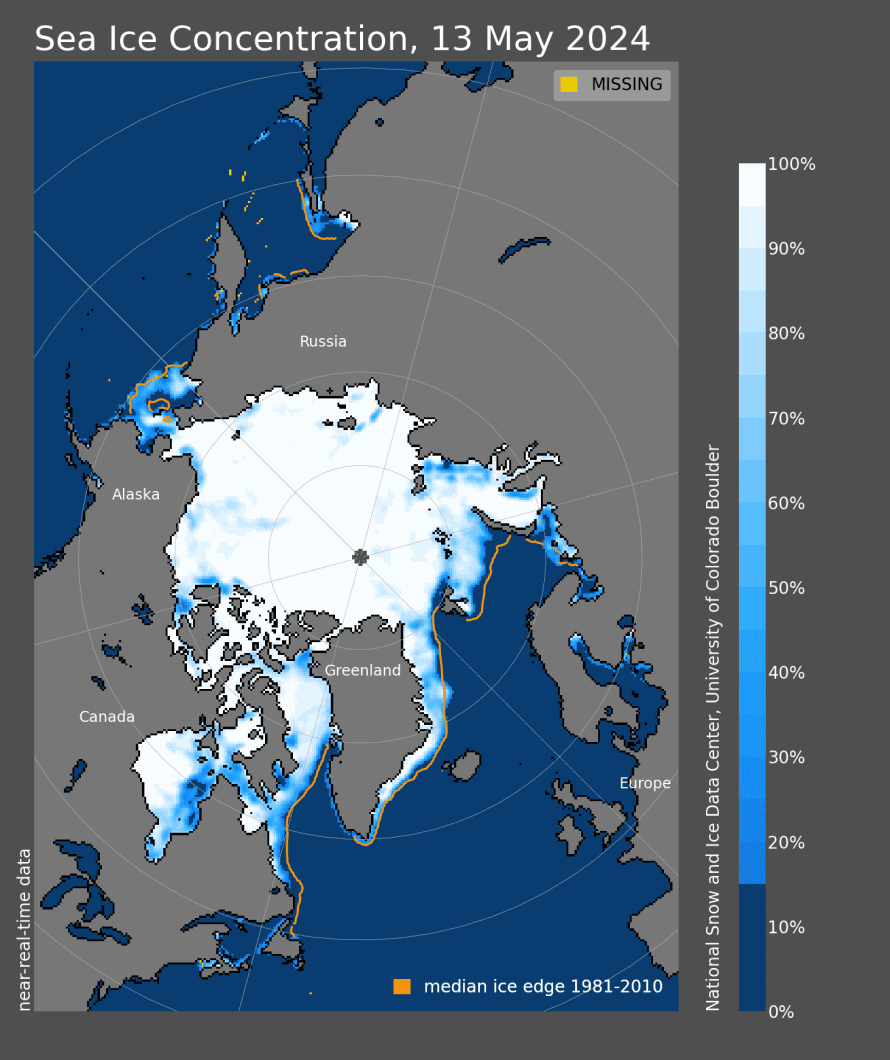
For example, this study aims to determine the accuracy of Convolutional Neural Networks (CNNs) in classifying ice objects in close-up images. It specifically examines these models’ performance in images with visual distortions and compares them to human analysts.
Another paper proposes PolarGAN, a Generative Adversarial Network (GAN) capable of creating realistic artificial images of Arctic sea ice concentrations.
What’s next?
The integration of computer vision in environmental conservation serves as a great shift in our ability to protect and save nature. Technological advancement provides a lot of precision, efficiency, and scalability addressing several challenges in today’s world.
From monitoring wildlife and marine life to assessing climate change, AI enhances our understanding and environmental exposure. The future of conservation presses on more informed and effective approaches for preserving natural resources and ecosystems. We need to embrace many innovations as we take a crucial step towards protecting the planet for generations to come.