Recently, the fields of computer vision and machine learning have been gaining traction in agriculture. Computer Vision (CV) technology is changing the way agriculture operates by allowing for non-contact and scalable sensing solutions. The use of computer vision techniques in conjunction with image acquisition through remote cameras has opened up a range of new applications in the agricultural sector, from saving production costs with intelligent automation to boosting productivity.
This article provides an overview of computer vision for agriculture and smart farming applications. In particular, we will be looking into:
- Livestock, Poultry or Aquaculture Monitoring
- Crop Monitoring and Yield Estimation
- Security and Compliance Monitoring
About us: Viso.ai provides the leading end-to-end Computer Vision Platform Viso Suite. The technology enables organizations to build, deliver, and scale their computer vision applications. Get a demo for your company.
Computer Vision in Agriculture
The agricultural sector has witnessed a lot of contributions when it comes to artificial intelligence (AI) and computer vision in areas like plant health detection and monitoring, planting, weeding, harvesting, and advanced analysis of weather conditions.
Numerous smart farming use cases impact the complete food supply chain by providing useful insights about the entire farming process, facilitating real-time operational decision-making, and enhancing farming practices by introducing on-field smart sensors and devices.
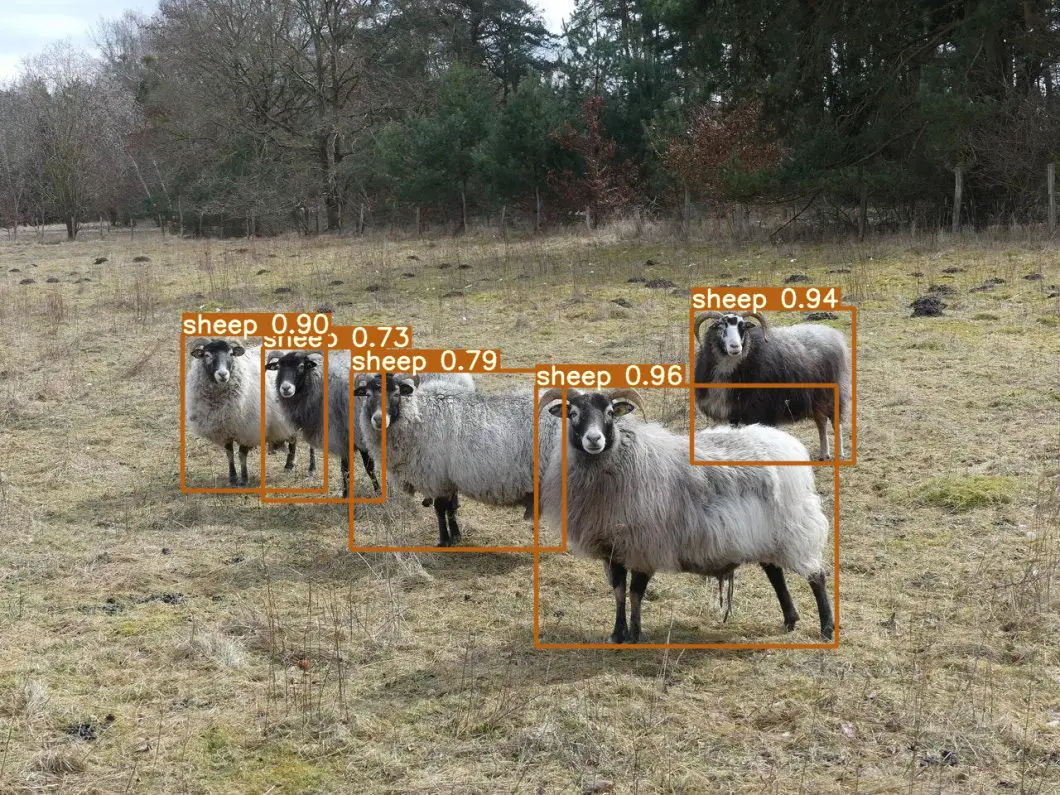
How is Computer Vision Helpful in Agriculture?
Computer Vision, a subfield of Artificial Intelligence, enables machines to perceive and interpret the visual world like humans. By combining computer vision techniques with remote cameras for image acquisition, non-contact and scalable sensing solutions are made possible in agriculture.
Some of the applications in agriculture include AI-powered animal monitoring, visual quality control, automated inspections for quality standards, and infrastructure monitoring. Additionally, computer vision has the potential to significantly improve crop monitoring and yield prediction by using image analysis to detect plant health, growth patterns, and potential stress factors.
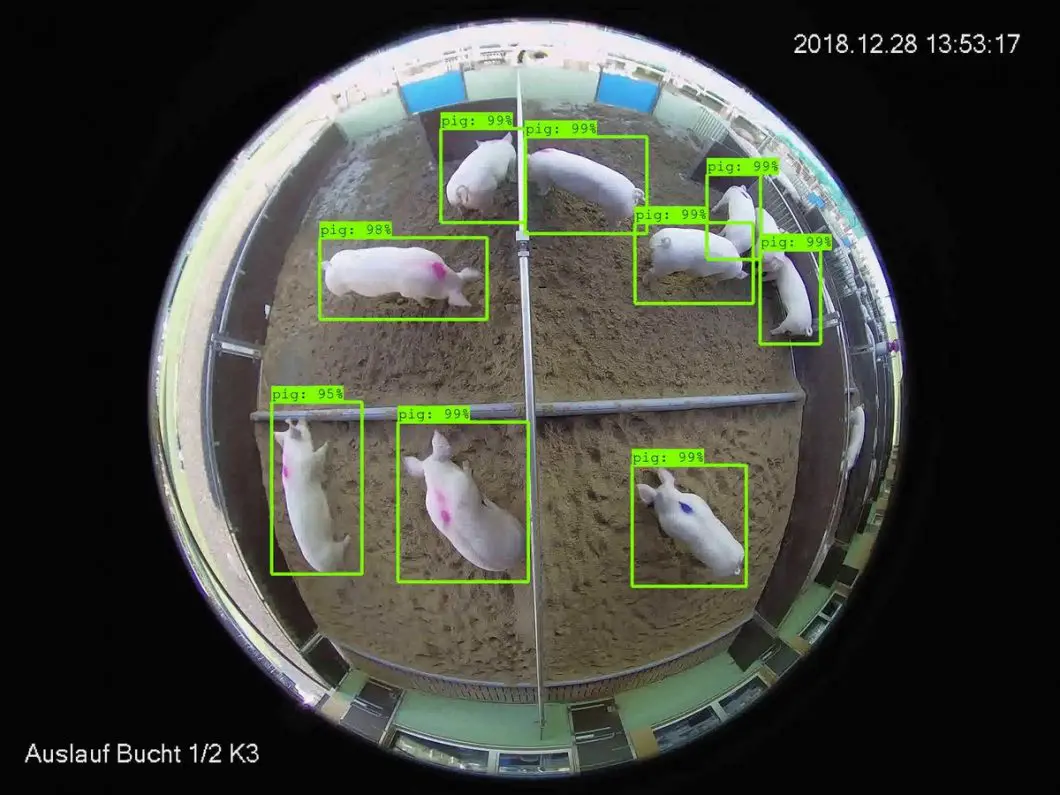
AI Technology Trends of Computer Vision Agriculture Applications
Generally, computer vision works in three basic steps:
- (1) acquiring the image/video from a camera,
- (2) processing the image, and
- (3) understanding the image.
Recently, new deep learning technologies achieved great breakthroughs in the field of image recognition. Compared to traditional computer vision, modern deep learning algorithms are much more robust and allow highly accurate real-time image recognition. Hence, deep learning methods can be used to perform video analytics with the video of common surveillance cameras or webcams.
The latest trends combine edge computing with on-device Machine Learning; a method also called Edge AI. Moving AI processing from the cloud to edge devices makes it possible to run machine learning everywhere, combining IoT and AI to create scalable computer vision applications.
Viso Suite Infrastructure for Developing and Operation Computer Vision Systems
To build and deploy such applications effectively, we’ve built a computer vision platform Viso Suite that helps industry leaders across industries to deliver all their AI vision applications 10x faster and more agile. Get started and use the best computer vision capabilities out-of-the-box, and on enterprise-grade infrastructure.
In the following, we will list some of the most significant AI vision applications in agriculture. Given the recent technological advances, we expect to see many more use cases and large-scale computer vision applications soon.
Best Applications of Computer Vision in Agriculture
- Application #1: Computer Vision Systems in Livestock Farming
- Application #2: Computer Vision Systems in Poultry Farming
- Application #3: Fish Farming With Computer Vision
- Application #4: Yield Estimation With Fruit or Vegetable Counting
- Application #5: Security Monitoring for Remote Farms
- Application #6: Achieve Compliance With Animal Welfare Law
- Application #7: Drone-Based Crop Monitoring with AI
- Application #8: Weed Detection and Management
- Application #9: Soil Health Assessment
1. Computer Vision Agriculture Systems in Livestock Farming
Food security is one of the world’s biggest challenges. Livestock and poultry contribute to a large proportion (30%) of the daily protein intake through products like meat, milk, egg, and offal. Animal production is expected to increase accordingly to feed the growing human population.
As production is intensified to meet the increased demands, producers are confronted with increasing pressure to provide quality care for an increasing number of animals per management unit. This becomes even more challenging given the expected labor shortages for farm jobs in the future.
Computer Vision systems monitor animals such as cattle, sheep, pigs, or others with cameras. Neural networks are used to analyze video feeds in real time. The advantages of computer vision systems root in their automatic, non-invasive, and low-cost animal monitoring capabilities.
AI vision systems allow information extraction with minimal external inferences (human adjustment of sensors, maintenance) at an affordable cost.
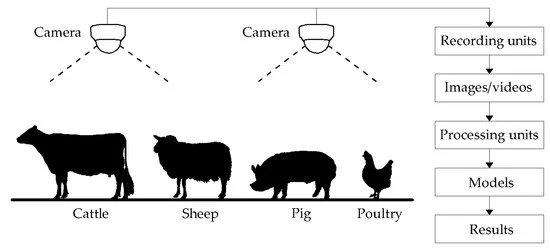
Computer vision is therefore needed for data collection, analysis, and decision-making in livestock farming. The insights help to improve the welfare, environment, engineering, genetics, and management of farm animals through evidence-based facility design and farm management.
Animal monitoring systems provide continuous real-time monitoring and assist producers in management decisions. They also provide early detection and prevention of disease and production inefficiencies. AI vision is able to provide objective measures of animal behaviors and phenotypes as opposed to subjective manual observation.
2. Computer Vision in Agriculture Systems in Poultry Farming
Advanced deep learning algorithms are robust enough to be applied in poultry farming. The term “poultry” includes a range of domesticated species, including chickens, turkeys, ducks, geese, game birds, and ratites (e.g., emus and ostriches).
In poultry farms, computer vision technology aims to prevent diseases and ensure food security while enhancing overall productivity by lowering costs and providing information to increase product quality.
Today, computer vision is widely used in poultry production systems. It includes house management automation, behavior analysis, animal welfare, disease detection, weight measurement, egg examination, and more.
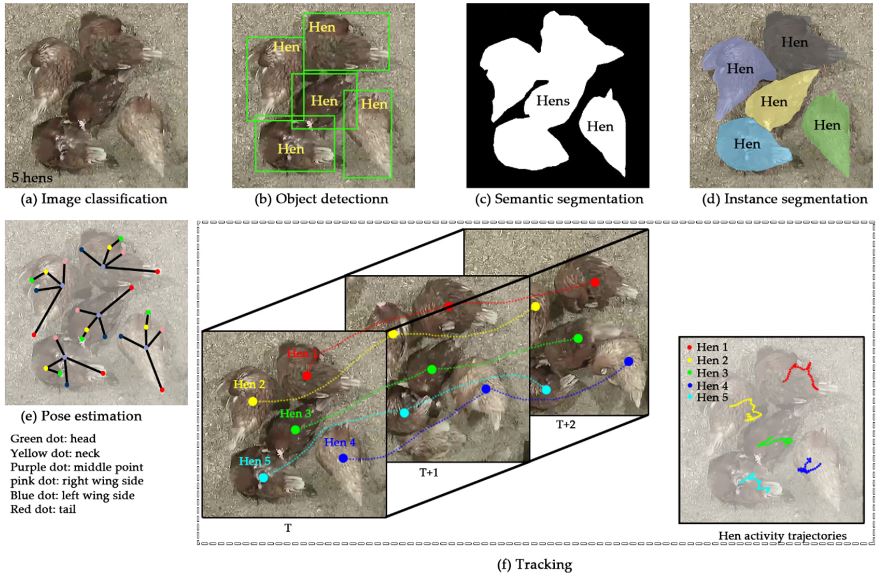
3. Fish Farming With Computer Vision
Automatic fish detection with computer vision is an important tool in precision farming for achieving automatic fish detection. Especially, deep learning methods have shown great potential in fish species identification, counting, and behavior analysis.
Also, computer vision is rapidly developing to be used in effective intelligent feeding systems. Such systems are based on underwater image preprocessing, fish detection, fish weight and length estimation, and fish behavior analysis.
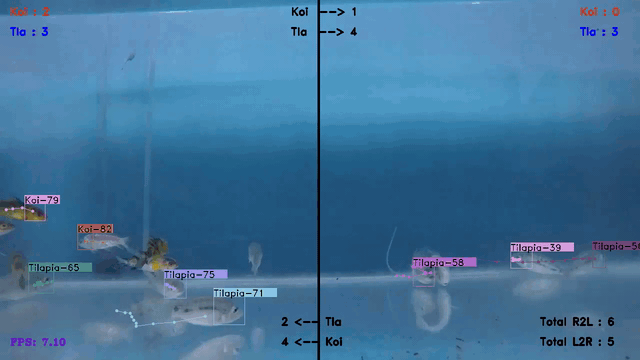
Fish counting is still a rudimentary process in many fisheries. Computer vision-based systems provide a cost-efficient method for counting fish with deep learning. Automatic fish counting reduces costs, helps to boost production, and increases labor availability. For example, computer vision has been used for automatic live fingerling counting.
Related novel use cases for aquaculture enterprises involve analyzing the integrity and safety of fishing nets with deep learning machine learning technology.
4. Yield Estimation With Fruit or Vegetable Counting
Yield estimation is an essential preharvest practice among most large-scale farming companies. It supports decision-making for allocating essential logistics such as transportation means, labor force, supplies, and more. An overestimation leads to further costs that impact profitability; underestimation entails potential crop waste and additional costs. Yield prediction is also used to optimize cultivation practices and plant disease prevention.
Deep convolutional network algorithms are developed to facilitate the accurate yield prediction and automatic counting of fruits and vegetables on images. Modern deep learning methods provide good accuracy even with occlusion caused by leaves or branches, illumination, and object size.
Manual yield estimation with the counting of products such as fruits or vegetables is very time-consuming and expensive. Computer vision approaches can be used for the automatic counting of fruits or flowers. An example is the automatic on-tree counting and yield estimation of kiwifruit.
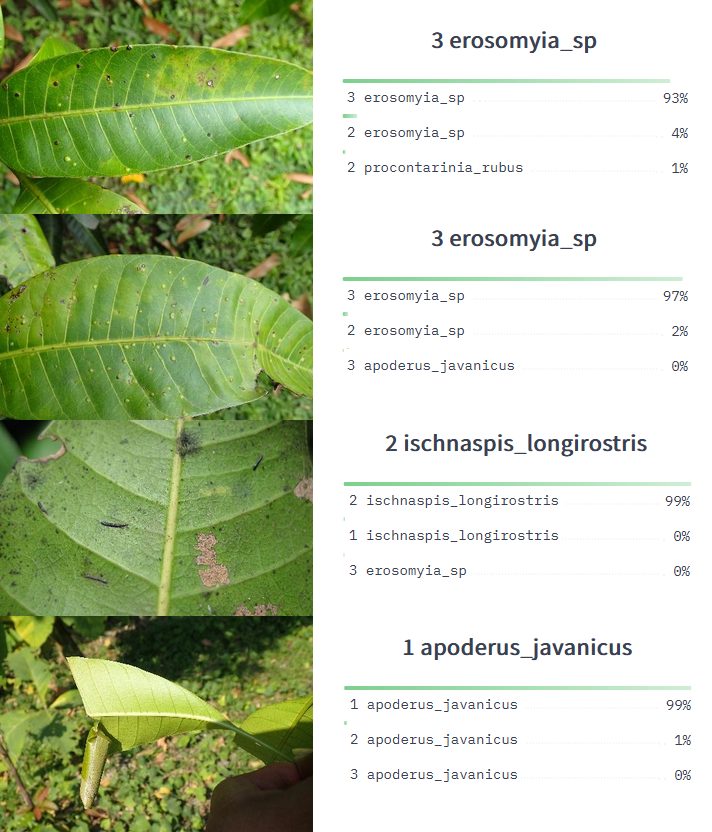
5. Security Monitoring for Remote Farms
Real-time surveillance and security monitoring for remote farms is another current application of ML in smart farming. Such monitoring and notification systems are of high importance to farms. The images detected with common surveillance systems can be processed by AI algorithms to perform intrusion detection and automatically identify anomalies.
Modern methods use deep neural networks to perform accurate face recognition that is invariant to changes in illumination. This makes it possible to implement deep face recognition in multiple remote farms.
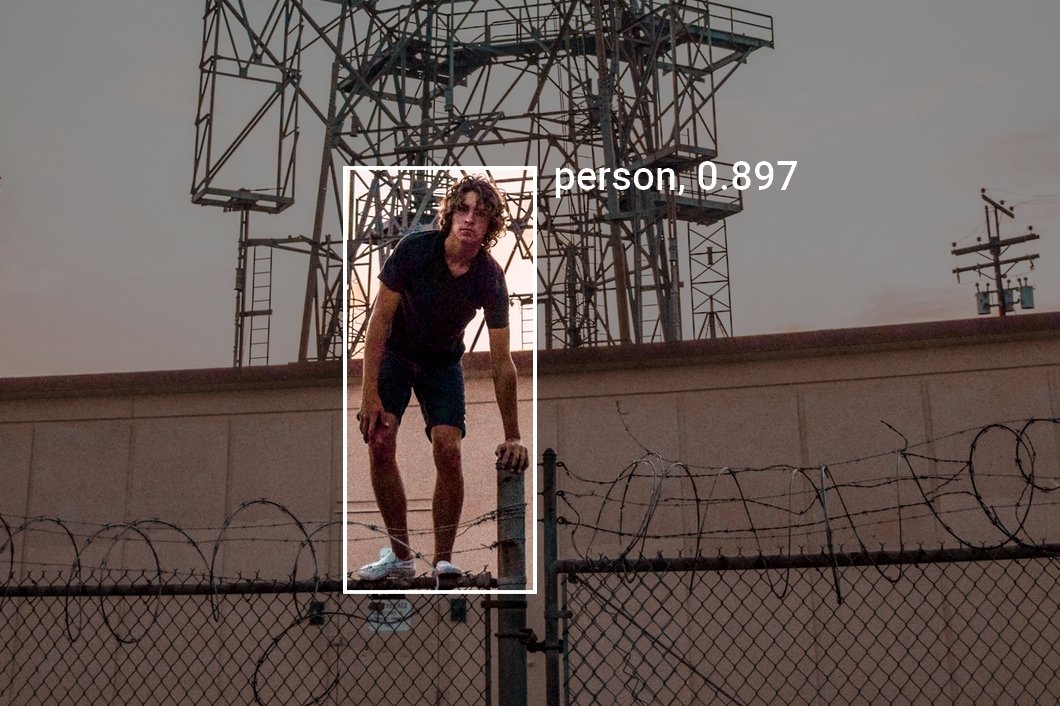
6. Achieve Compliance With Animal Welfare Law
Computer vision systems provide a way to automate regular on-farm monitoring to ensure compliance with animal welfare law. Animal monitoring with deep learning algorithms and conditional logic can trigger alarms to trigger corrective actions.
Smart vision systems use AI cameras to provide objective measurements of animal welfare under field conditions. Modern methods are capable of assessing the resources provided to the animals (space, lying substrate, drinker access) and measuring the animals themselves to detect lameness, indicators of injury or disease, and abnormal behaviors. Hence, computer vision provides quantifiable data about animal welfare that can be used to ensure compliance with on-farm animal welfare.
To learn more, check out our animal monitoring case study with Viso Suite.
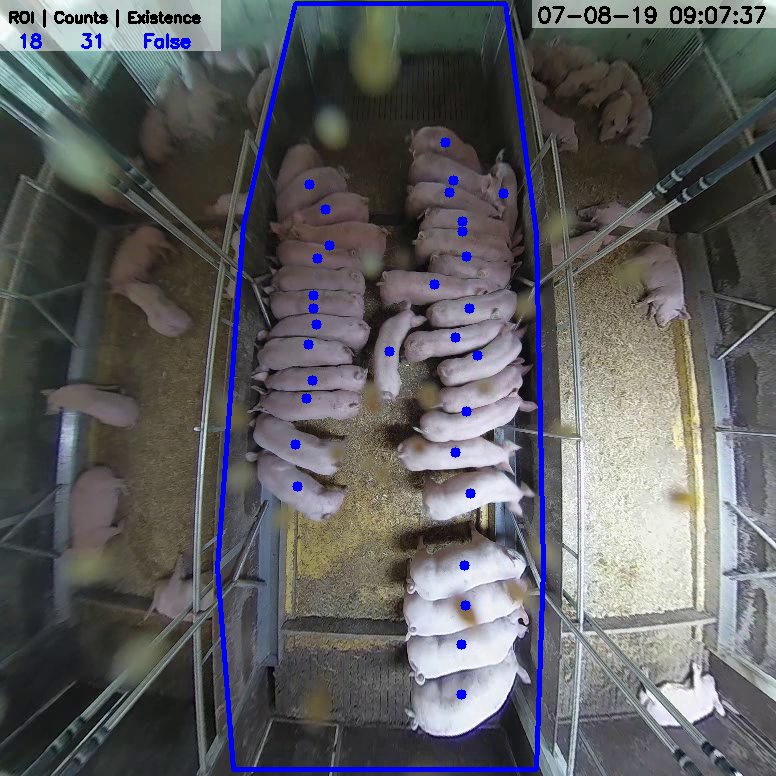
7. Drone-Based Crop Monitoring
Over the last few years, drone technology has gained huge popularity because of its autonomous flying capabilities. Drones have become a significant element in precision agriculture and farming. Because of their flying abilities and cover a large distance, drones can capture huge volumes of data with a built-in camera.
Computer vision algorithms are trained with the captured footage to assist in crop mapping and planning and environmental monitoring based on geo-sensing information. Therefore, the images are labeled with image annotation to create training data for algorithm training. The computer vision models perform object detection and semantic segmentation to recognize objects and conditions in drone footage.
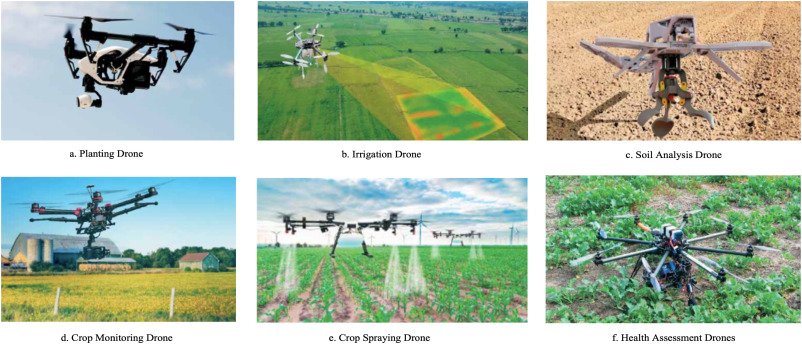
8. Weed Detection and Management
With the help of the aforementioned drone technology, farmers have been able to optimize weed detection and management. With a two-pronged approach, farmers can use drones equipped with cameras for image recognition to both distinguish crops from weeds and identify the specific species of weeds. The cameras collect visual media
By pinpointing these exact instances of weeds, farmers can precisely apply herbicides before weeds spread further. This approach allows for more efficient weed control and helps optimize agricultural productivity while minimizing the use of herbicides.
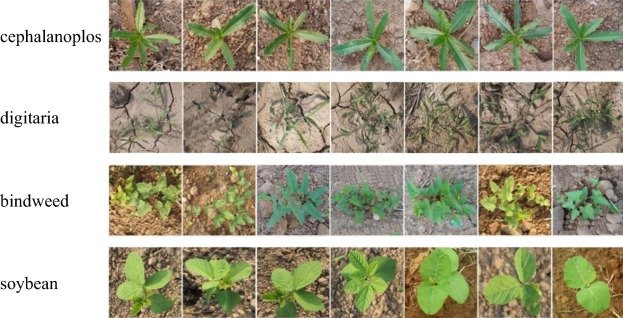
9. Soil Health Assessment
By integrating IoT systems with computer vision farmers can use ground sensors, drone cameras, and deep learning networks to monitor soil conditions. These systems can perform tests such as root health analysis, soil erosion monitoring, and pH analyses.
For example, very acidic soil, which can appear light brown and be categorized by RGB codes, is suitable for cranberries, blueberries, and broccoli. If the soil were to change in pH, it would be necessary for farmers to introduce treatments to reregulate the pH back to a desirable level.
The same can be done for moisture monitoring in the soil, helping farmers in real time determine whether crops require further irrigation.
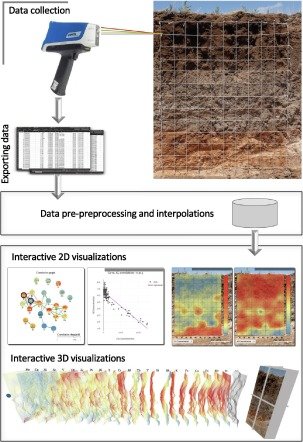
Get Started With Computer Vision in Agriculture Solutions
If you are looking for ways to rapidly build and deliver a computer vision application in smart farming and agriculture, you might want to look at our computer vision platform Viso Suite.
The integrated platform provides all the end-to-end tools, machine learning model frameworks, and software infrastructure your teams need to build, deploy, and scale your deep learning vision solutions – without coding everything from scratch. Get in touch and get a live demo.
To read more about related topics, you might be interested in the following articles: