One of the main issues for computer vision (CV) developers is to avoid overfitting. That’s a situation when your CV model works great on the training data but is inefficient in predicting the test data. The reason is that your model is overfitting. To fix this problem you need regularization, most often done by Batch Normalization (BN).
The regularization methods enable CV models to converge faster and prevent over-fitting. Therefore, the learning process becomes more efficient. There are several techniques for regularization and here, we present the concept of batch normalization.
About us: Viso Suite is the computer vision solution for enterprises. By covering the entire ML pipeline in a simple interface, teams can easily integrate computer vision into their business workflows. To learn how your team can get started with computer vision, book a demo of Viso Suite.
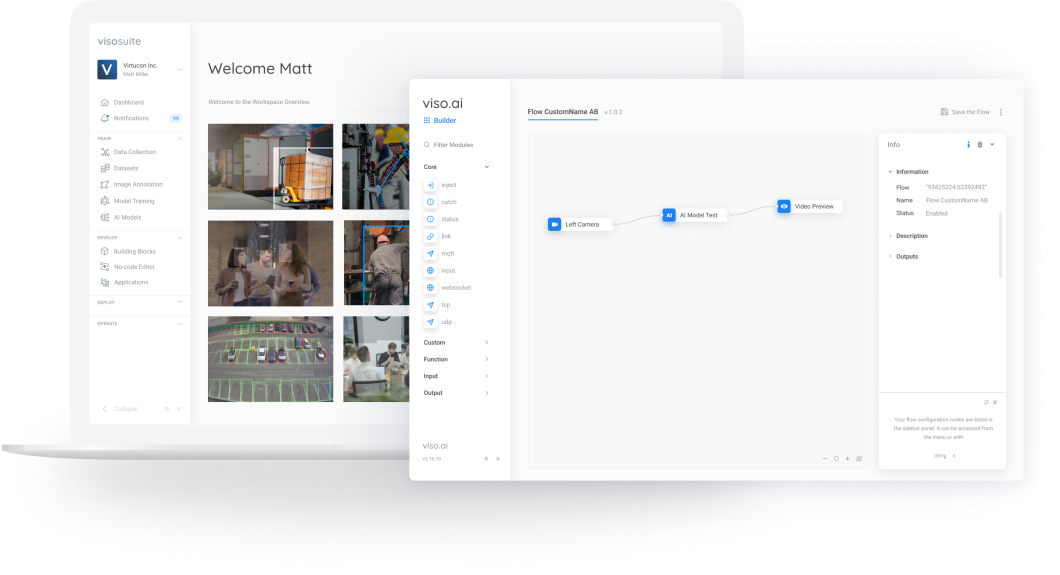
What is Batch Normalization?
Batch normalization is a method that can enhance the efficiency and reliability of deep neural network models. It is very effective in training convolutional neural networks (CNN), providing faster neural network convergence. As a supervised learning method, BN normalizes the activation of the internal layers during training. The next layer can analyze the data more effectively by resetting the output distribution from the previous layer.
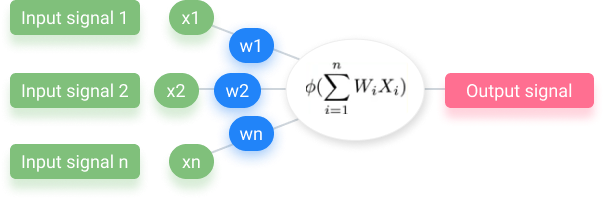
Internal covariate shift denotes the effect that parameters change in the previous layers have on the inputs of the current layer. This makes the optimization process more complex and slows down the model convergence.
In batch normalization – the activation value doesn’t matter, making each layer learn separately. This approach leads to faster learning rates. Also, the amount of information lost between processing stages may decrease. That will provide a significant increase in the precision of the network.
How Does Batch Normalization Work?
The batch normalization method enhances the efficiency of a deep neural network by discarding the batch mean and dividing it into the batch standard deviation. The gradient descent method scales the outputs by a parameter if the loss function is large. Subsequently – it updates the weights in the next layer.
Batch normalization aims to improve the training process and increase the model’s generalization capability. It reduces the need for precise initialization of the model’s weights and enables higher learning rates. That will accelerate the training process.
Batch normalization multiplies its output by a standard deviation parameter (γ). Also, it adds a mean parameter (beta) when applied to a layer. Due to the coaction between batch normalization and gradient descent, data may be disarranged when adjusting these two weights for each output. As a result, a reduction of data loss and improved network stability will be achieved by setting up the other relevant weights.
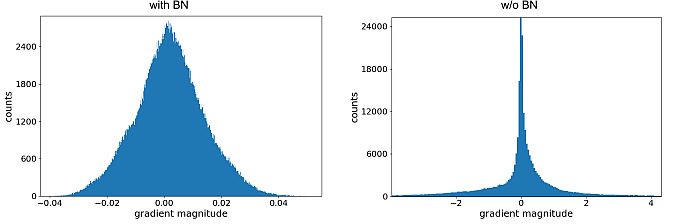
Commonly, CV experts apply batch normalization before the layer’s activation. It is often used in conjunction with other regularization functions. Also, deep learning methods, including image classification, natural language processing, and machine translation utilize batch normalization.
Batch Normalization in CNN Training
Internal Covariate Shift Reduction
Google researchers Sergey Ioffe and Christian Szegedy defined the internal covariate shift as a change in the order of network activations due to the change in network parameters during training. To improve the training, they aimed to reduce the internal covariate shift. Their goal was to increase the training speed by optimizing the distribution of layer inputs as training progressed.
Previous researchers (Lyu, Simoncelli, 2008) applied statistics over a single training example, or, in the case of image networks, over different feature maps at a given location. They wanted to preserve the information in the network. Therefore, they normalized the activations in a training sample relative to the statistics of the entire training dataset.
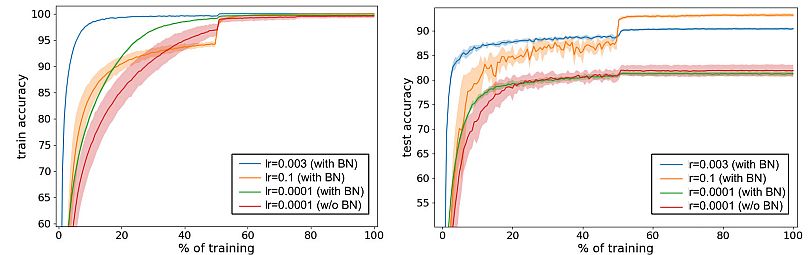
The gradient descent optimization doesn’t take into account the fact that the normalization will happen. Ioffe and Szegedy wanted to ensure that the network always produces activations for parameter values. Due to the gradient loss, they applied the normalization and calculated its dependence on the model parameters.
Training and Inference with Batch-Normalized CNNs
Training can be more efficient by normalizing activations that depend on a mini-batch, but it is not necessary during inference. (Mini-batch is a portion of the training dataset). The researchers needed the output to depend only on the input. By applying moving averages, they tracked the accuracy of a model, while it trained.
Normalization can be done by applying a linear transformation to each activation, as means and variances are fixed during inference. To batch-normalize a CNN, researchers specified a subset of activations and inserted the BN transform for each (Algorithm below).
Authors considered a mini-batch B of size m. They performed normalization to each activation independently, by focusing on a particular activation x(k) and omitting k for clarity. They got m values for each activation in the mini-batch:
B = {x1…m}
They denoted normalized values as x1…m, and their linear transformations were y1…m. Researchers have defined the transform
BN γ,β : x1…m → y1…m
to be the Batch Normalizing transform. They conducted the BN Transform algorithm given below. In the algorithm, σ is a constant added to the mini-batch variance for numerical stability.
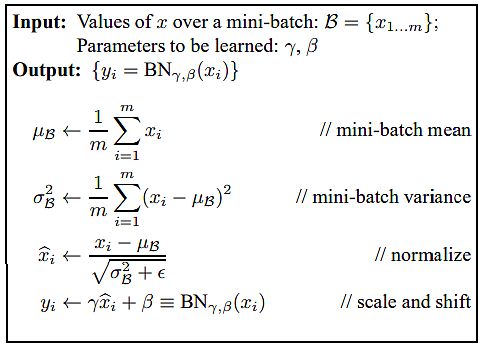
The BN transform has been added to a network to manipulate all activations. By y = BN γ,β(x), researchers indicated that the parameters γ and β should be learned. However, they noted that the BN transform does not independently process the activation in each training example.
Consequently, BN γ,β(x) depends both on the training example and the other samples in the mini-batch. They passed the scaled and shifted values y to other network layers. The normalized activations xb were internal to the transformation, but their presence was crucial. The distributions of values of all xb had the expected value of 0 and the variance of 1.
All layers that previously received x as the input – now receive BN(x). Batch normalization allows for the training of a model using batch gradient descent, or stochastic gradient descent with a mini-batch size m > 1.
Batch-Normalized Convolutional Networks
Szegedy et al., (2014) used batch normalization to create a new Inception network, trained on the ImageNet classification task. The network had a large number of convolutional and pooling layers.
- They included a SoftMax layer to predict the image class, out of 1000 possibilities. Convolutional layers include ReLU as the nonlinearity.
- The main difference between their CNN was that the 5 × 5 convolutional layers were replaced by two consecutive layers of 3 × 3 convolutions.
- By using batch normalization researchers matched the accuracy of Inception in less than half the number of training steps.
- With slight modifications, they significantly increased the training speed of the network. BN-x5 needed 14 times fewer steps than Inception to reach 72.2% accuracy.
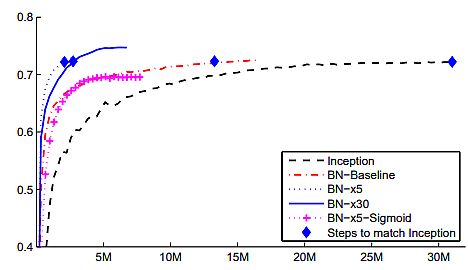
By increasing the learning rate further (BN-x30) they caused the model to train slower at the start. Still, it was able to reach a higher final accuracy. It reached 74.8% after 6·106 steps, i.e. 5 times fewer steps than required by Inception.
Benefits of Batch Normalization
Batch normalization brings multiple benefits to the learning process:
- Higher learning rates. The training process is faster since batch normalization enables higher learning rates.
- Improved generalization. BN reduces overfitting and improves the model’s generalization ability. Also, it normalizes the activations of a layer.
- Stabilized training process. Batch normalization reduces the internal covariate shift that occurs during training, improving the stability of the training process. Thus, it makes it easier to optimize the model.
- Model Regularization. Batch normalization treats the training example together with other examples in the mini-batch. Therefore, the training network no longer produces deterministic values for a given training example.
- Reduced need for careful initialization. Batch normalization decreases the model’s dependence on the initial weights, making it easier to train.
What’s Next?
Batch normalization offers a solution to address challenges with training deep neural networks for computer systems. By normalizing the activations of each layer, batch normalization allows for smoother and more stable optimization, resulting in faster convergence and improved generalization performance. Because it can mitigate issues like internal covariate shifts it enables the development of more robust and efficient neural network architectures.
For other relevant topics in computer vision, check out our other blogs:
- Deep Neural Networks: The 3 Popular Types (MLP, CNNs, ANNs)
- Representation Learning: Unlocking the Hidden Structure of Data
- OMG-Seg: 10 Segmentation Tasks in 1 Framework (2024)
- AI Emotion and Sentiment Analysis With Computer Vision in 2024
- AI Software: 17 Popular Products
Enterprise Computer Vision with Viso Suite
Viso Suite omits the need for enterprises to approach process automation with point solutions. Traditionally, computer vision solutions have only tackled a single step in the computer vision pipeline. This has led to companies dealing with disjointed computer vision initiatives.
As a result, using mixed infrastructure leads to a breakdown of the computer vision project at hand. Conversely, as a development platform, Viso Suite consolidates the entire machine learning pipeline into a single interface. With a unified initiative, enterprises have been able to realize a 695% ROI based on improved efficiency, productivity, and process automation. To learn more, check out our economic impact study.
Finally, Viso Suite was developed on the premise of being:
- Fast and accurate
- Easy to use
- Open and extensible
- Efficient and scalable
- Private and secure
To learn more about Viso Suite and the value it provides enterprise ML teams, book a demo with our team.
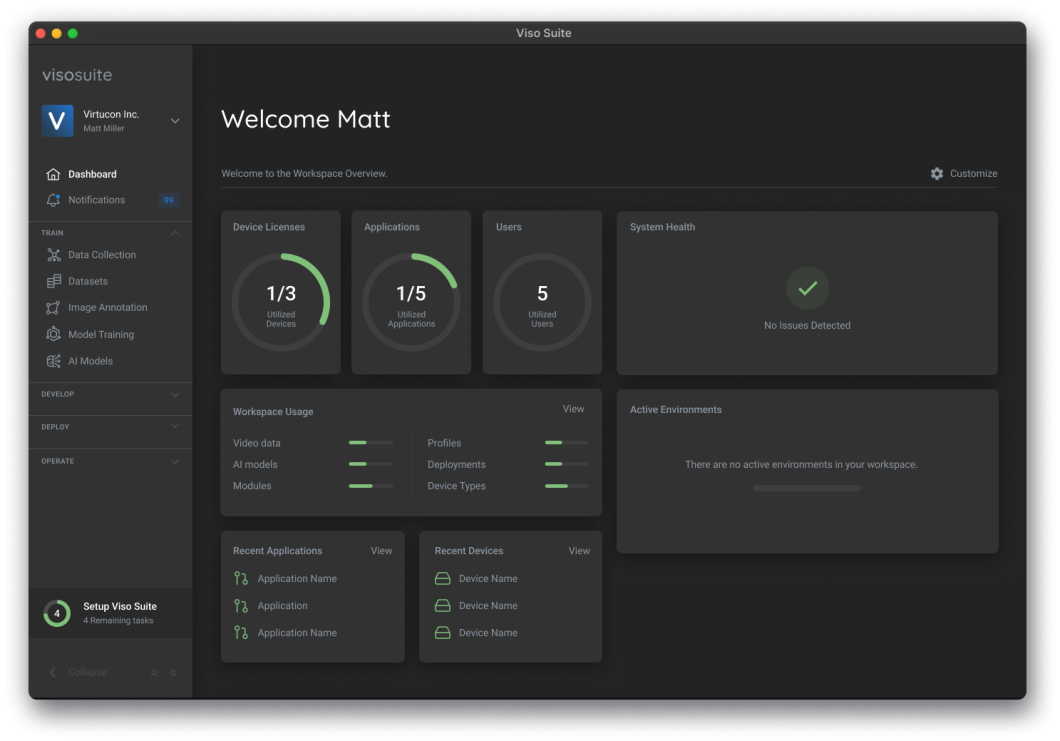