Computer vision is an application of artificial intelligence that enables machines to interpret and understand digital images. The aviation industry has been quick to adopt computer vision technology to improve air travel safety and efficiency.
The fields of use are very broad and include recognition and detection of airplanes, airport security and management, plane identification, autonomous flying of drones, and even aerial robotics. In this article, we share some of the top data-driven aviation solutions with computer vision.
About us: We power the Viso Suite, the world’s only end-to-end computer vision platform, to build, deploy and scale real-world applications. Leading organizations use our software infrastructure to deliver and scale their AI vision applications. Book a personal demo today.
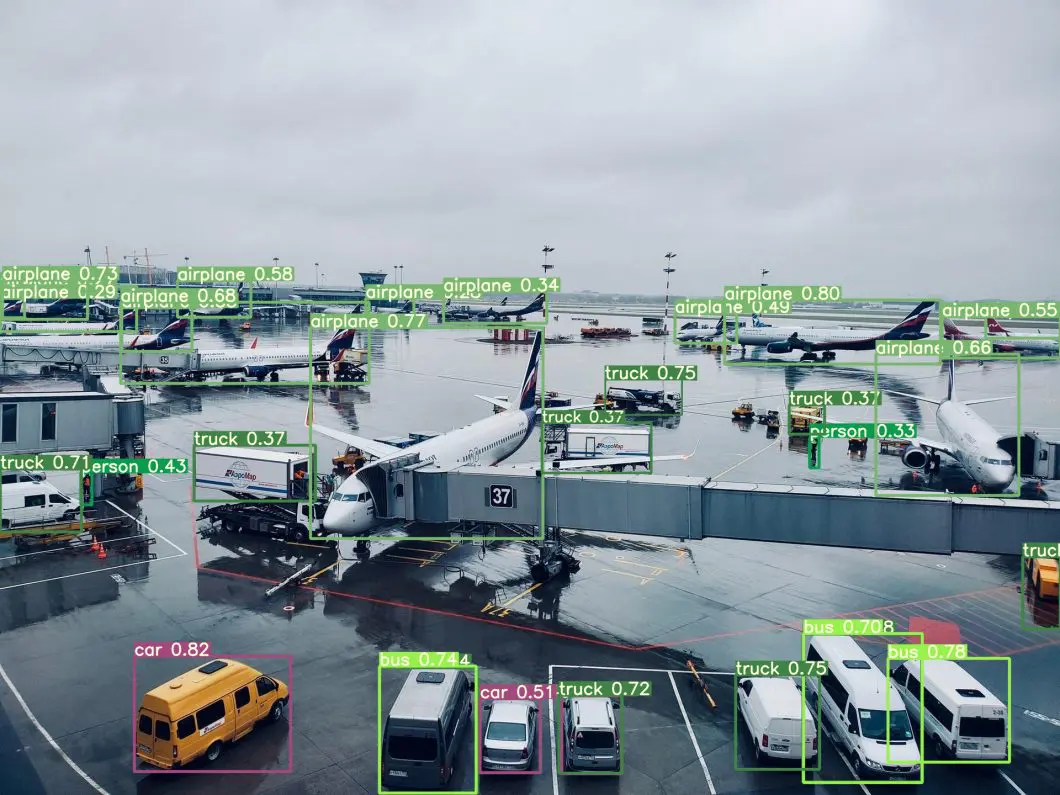
Aircraft Inspection and Maintenance
Computer vision is also used in aviation for tasks such as aircraft inspection and maintenance. By using images of the aircraft, technicians use machine learning to detect problems and recognize damage patterns that may not be visible to the naked eye. AI vision inspection helps to improve safety and avoid potential accidents or downtime.
In addition, computer vision can be used for the automatic examination of aircraft components to reduce the amount of time and labor required for manual inspection. It further helps to increase the objectivity and consistency of the assessment.
Examples of AI vision inspection applications include:
- Inspecting the body of the airplane for damages or problems
- Checking the engine for fluid leaks or other damage
- Looking for cracks or other damage on the wings or fuselage
- Inspecting the landing gear for wear or damage
- Analyzing the brakes and tires for wear or damage
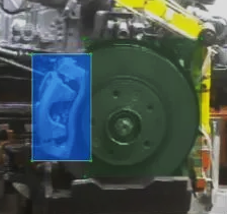
Intelligent Baggage Handling
Computer vision is being used more and more in airports for baggage handling. Deep learning systems can automatically read labels with machine vision (Optical Character Recognition) to identify trolleys and their locations. This helps to improve the efficiency of the baggage handling process and reduces the chance of lost luggage. It further reduces the number of errors and makes it easier to find misplaced luggage.
Computer vision systems use cameras to scan the tags on luggage and match them with the information in the airline’s database. This allows airport workers to quickly identify which bag belongs to which passenger. One of the first airports to implement a machine vision system was London Heathrow in 2014. Since then, many other airports have followed suit.
Another visual deep learning application helps to identify and localize ground vehicles and baggage tugs and carts at airports. This is important to increase the efficiency of managing and distributing baggage carts. In addition, vehicle identification and localization help to avoid ground vehicle collisions, which are a major cause of accidents at airports.
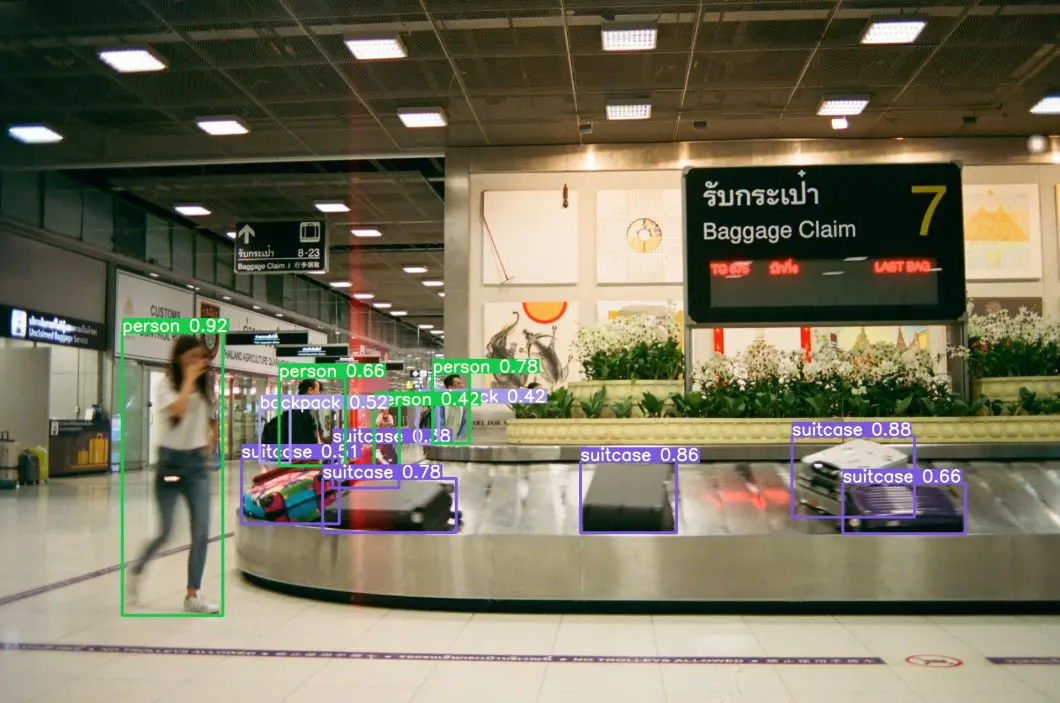
AI Vision Security at Airports
Computer vision can also be used for security applications. By installing cameras at strategic locations, it is possible to track the movement of people and objects at airports. Real-time information and reports help to identify potential security threats and improve operational security.
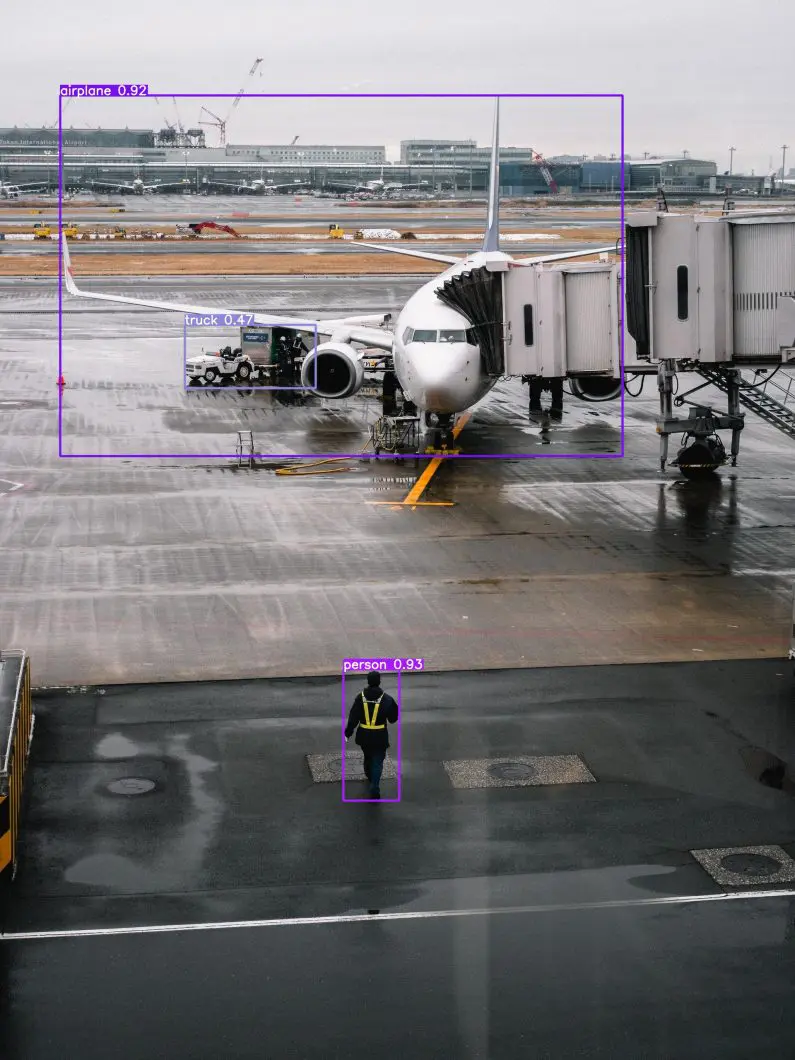
Surveillance use cases include fence-climbing detection, perimeter monitoring to detect trespassing, large-scale heat mapping, movement path analysis, and queue monitoring. Advanced applications involve emotion analysis and gaze estimation to evaluate the mood and attentiveness of people.
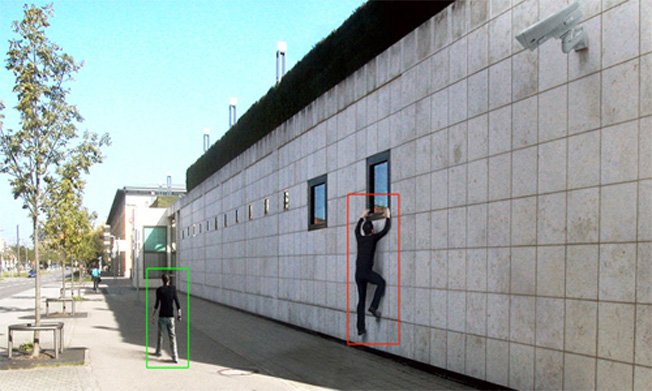
Important applications of AI vision monitoring with computer vision include:
- Tracking the movement of people and objects
- Monitoring for potential security threats, detection of abandoned objects
- Implement real-time crowd monitoring and anomaly analysis
- Reduce false positives and negatives in security checks
- Automated fire and smoke detection
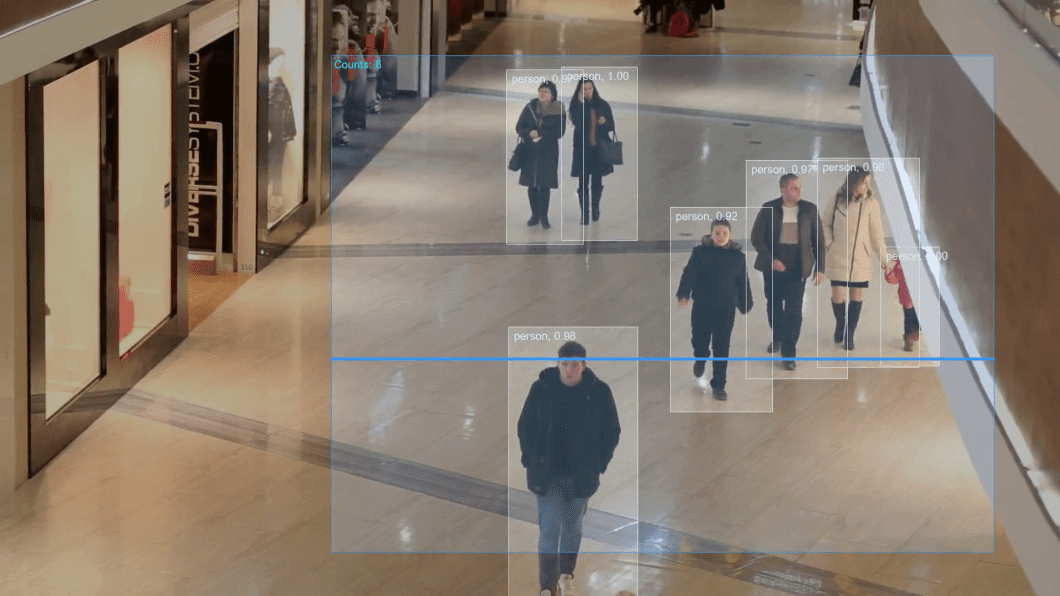
Face Recognition at Airports
Computer vision based facial recognition software is used to identify passengers at airports. Such AI software compares the passenger’s face to a database of images that have been pre-loaded into the system. The system performs face detection and face recognition with common IP cameras.
Face analysis technology is used in order to streamline the boarding process and to make sure that only passengers who are supposed to be on a particular flight are able to board. In some cases, facial recognition software is also used in order to identify suspected criminals or individuals that are on no-fly lists.
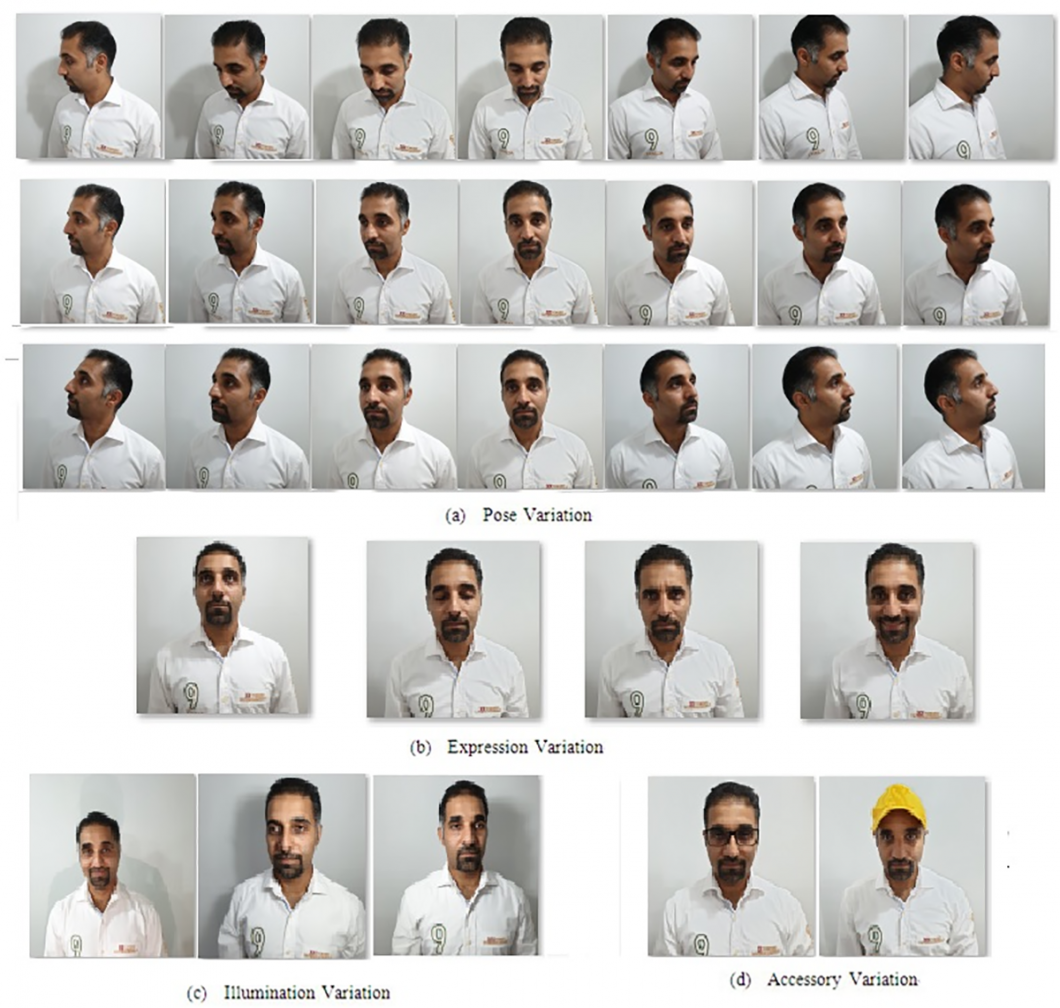
Runway Inspection with AI
Computer vision is being used more and more in the field of runway inspection. By using cameras and sensors, airport personnel can detect any potential problems with the runway surface. This is a critical task since small cracks or potholes in a runway may have dramatic consequences.
Such systems use a variety of sensors, including infrared and thermal imaging, to create a detailed map of the runway surface. This information can be used to identify any defects or damage that may need repair.
Runway inspection with AI vision is also used for detecting debris and foreign objects or vehicles, identifying and correcting pavement deficiencies, and managing airport safety. Automatic runway inspection also helps to ensure that the airport is compliant with Federal Aviation Administration (FAA) regulations.
Other large-scale runway inspection applications use multiple cameras with deep learning detection to identify unauthorized people or detect airplanes on the landing field.
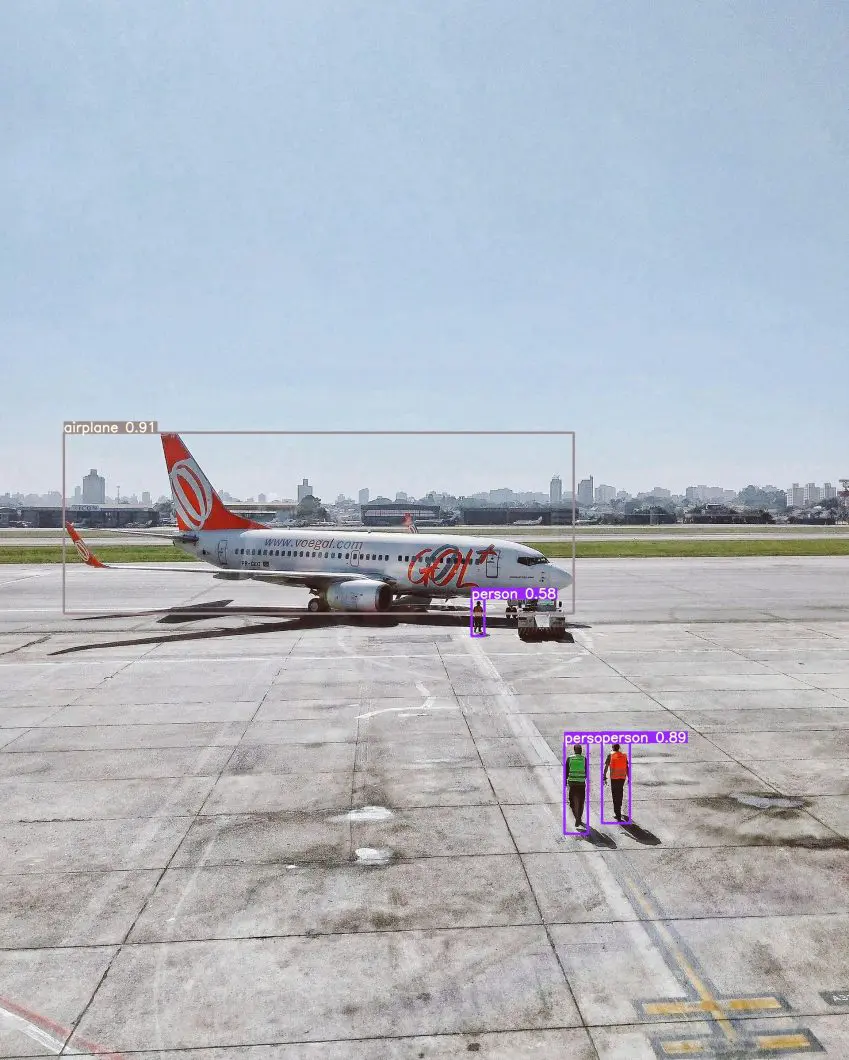
AI Vision for Cargo Inspection
Advanced computer vision algorithms help airport security personnel to inspect the cargo for any potential threats such as explosives, weapons, or narcotics. AI-assisted cargo inspection helps to speed up the process of getting cargo through security and onto the plane.
For example, a deep learning model can be used to detect explosives or narcotics in a shipment by applying pattern recognition to image data from scanners or cameras. To learn those patterns, the neural network is trained on annotated images of known explosive materials.
Once the algorithm is trained, it can then be used to scan new image data to recognize such patterns. If the algorithm finds a match, it can raise an alert to trigger a manual inspection.
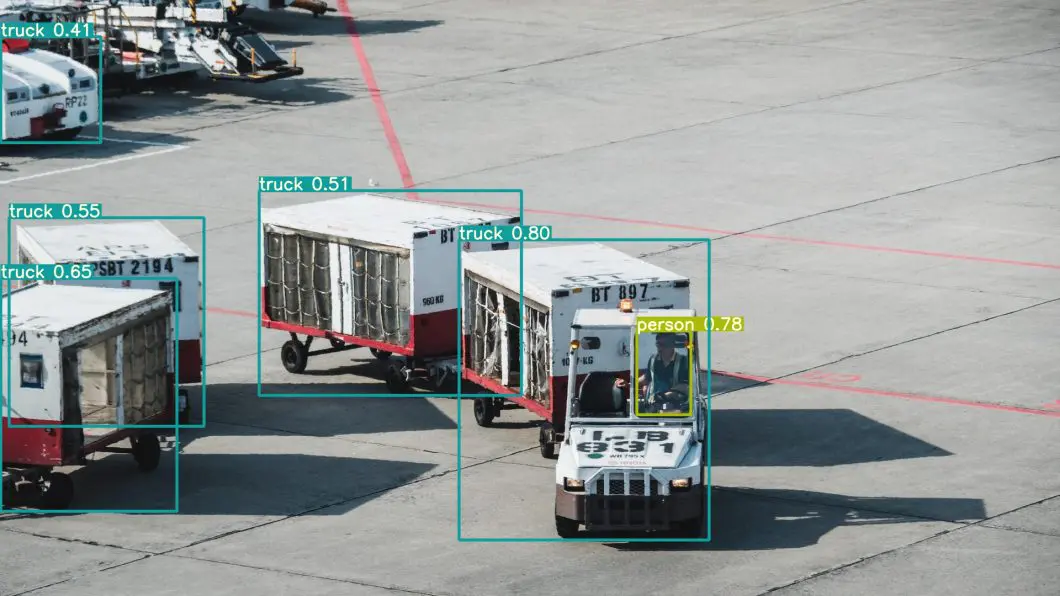
Counter-drone Sensing
As unmanned aerial systems (UAS) become more complex and widespread, they present new challenges in terms of safety, security, and privacy. One growing concern is the number of incidents involving drones near airport facilities. As drone technology continues to evolve, it is likely that such incidents will become more frequent and severe. In order to protect critical infrastructure from potential aerial attacks, it is important for airports to have effective countermeasures in place.
Counter-drone systems often involve multiple sensors, radar, PTZ, and infrared cameras to detect rogue drones in a geofenced area around the airport. Some counter-drone systems also have the ability to intercept and destroy drones that are deemed a threat. These systems use high-powered lasers or radio signals to disrupt the drone’s electronics, causing it to crash.
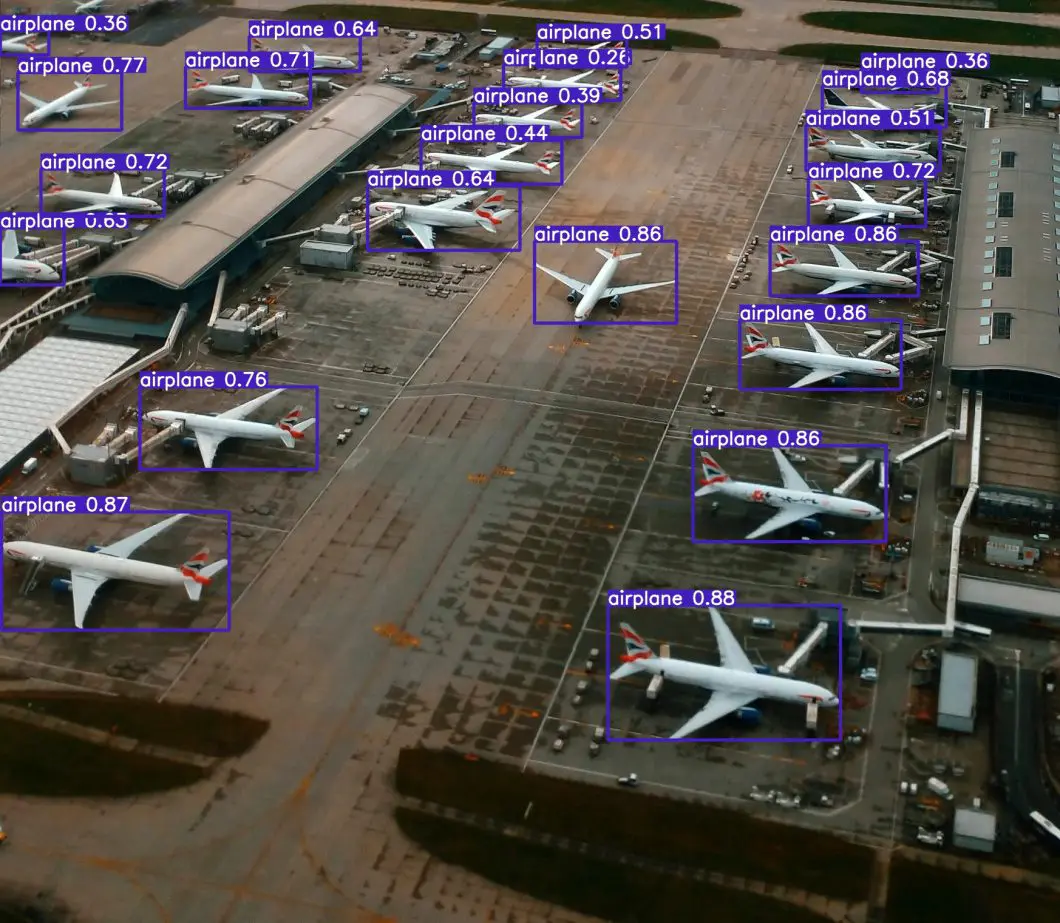
Computer Vision in Aerial Robotics
Vision-based control has been successfully used in a number of different UAV platforms, including fixed-wing aircraft, helicopters, and quadrotors. For example, computer vision is applied in UAVs to improve their autonomies both in flight control and perception of their surroundings.
Based on images and videos captured by onboard cameras, vision measures, such as stereo vision or optical flow fields, extract features that can be integrated with a flight control system to form visual serving, also known as vision-based robot control.
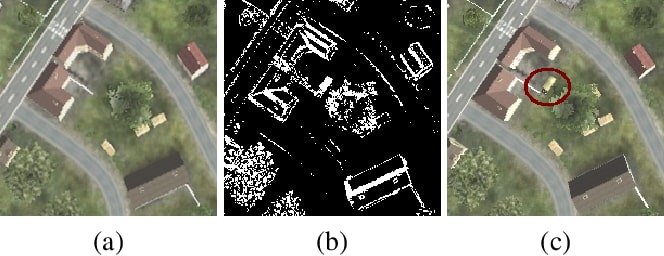
Three main interests in this field are visual navigation, aerial surveillance, and airborne visual Simultaneous Localization and Mapping (SLAM).
- Visual navigation is the ability of the vehicle to autonomously navigate its environment using only visual information. This can involve tasks such as avoiding obstacles, following paths or waypoints, or homing in on a specific target.
- Aerial surveillance uses UAVs to capture video or images of an area for later analysis. This can be useful for search and rescue missions, border patrol, or military reconnaissance.
- Airborne visual SLAM is the process of constructing a map of an area from aerial images and videos. The data from onboard sensors can be combined with imagery obtained from previous flights. This can be used for a variety of purposes, such as real-time scene reconstruction, 3D modeling, localization of detected objects, or tracking of vehicles, objects, or persons. Airborne visual SLAM systems have been used to map large areas such as city streets and buildings, forests, and agricultural fields.
Visual Aviation Aircraft Identification
In airport operations, aircraft identification is critical to various applications, including airport planning and environmental assessments. Research and commercial systems really heavily on recognizing aircraft tail numbers using text recognition.
Reduced visibility, visual obstruction, and hard-to-read tail numbers in various fonts, sizes, and orientations make this task challenging. Hence, a multi-step computer vision system is needed to provide more accurate airplane detection and identification.
To provide a more accurate identification system, computer vision systems use multiple steps. In the first step, a convolutional neural network (CNN) model identifies the aircraft type. The use of an aircraft classifier (airplane classification) is used to decrease the search space.
In the second step, the tail number is recognized within the search space, using optical character recognition (OCR) across multiple frames to provide robust text recognition in real-time video analysis.
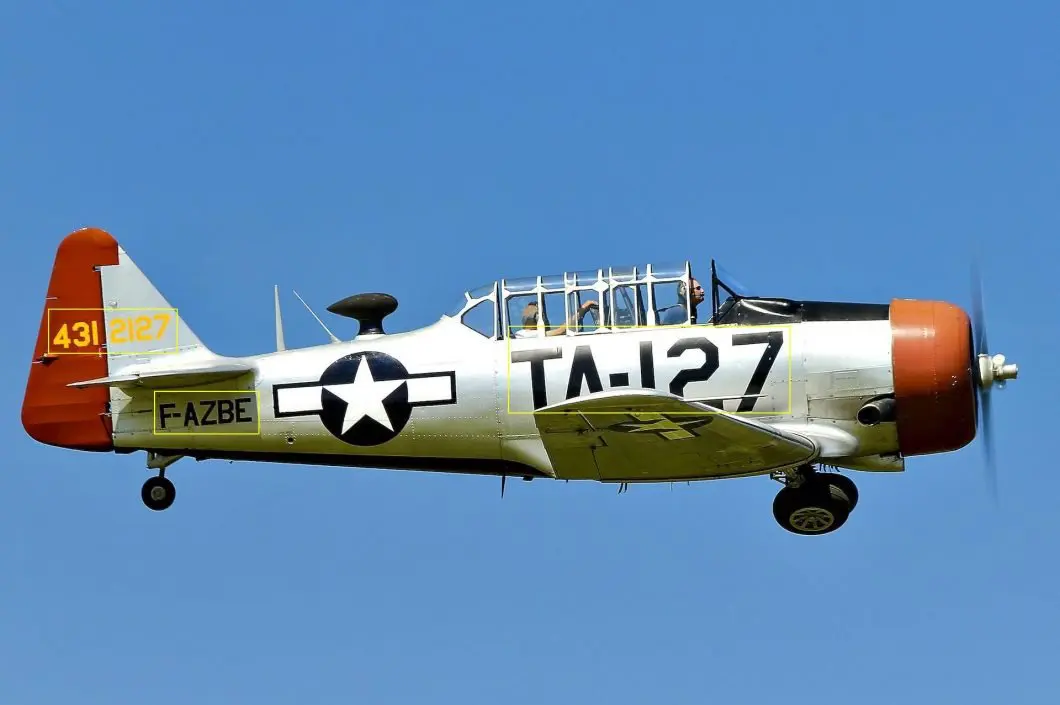
The Bottom Line
Computer vision is being used extensively in aviation for a wide range of purposes. These applications are just the beginning, and we can expect to see even more innovative uses for AI vision in the future. Recent advances in emerging technologies such as Edge AI, AIoT, and deep learning enable the development of even better and more robust machine learning solutions, while significantly improving the efficiency and cost of computer vision.
Read more about related topics and research: