Artificial intelligence (AI) in retail is a valuable asset for companies with physical stores. This automation allows stores to keep up with data-driven online business models, thus improving the shopping experience. This article is an update to our previous article featuring the most popular retail applications of computer vision.
We will share practical information about the challenges and opportunities of implementing AI vision in retail businesses. We discuss the future of retail AI, technology, and use cases and applications:
- AI vision analysis and compliance inspection
- Visitor profiling and customer behavioral analysis using AI
- AI product engagement assessment
- AI customer service evaluation
- In-store and outdoor AI inspection
- AI-powered checkout experience
- Security and safety use cases
About us: Viso.ai provides the leading end-to-end Computer Vision Platform Viso Suite. Our platform enables leading organizations worldwide to build, deliver, and scale their computer vision applications for retail solutions. Get a demo for your company.
Before we get started, check out this people counting application for retail artificial intelligence. The entire application was built and delivered with Viso Suite.
Computer Vision in Retail
Web-based retailing companies have gained market share from traditional brick-and-mortar retailers with multiple store locations. The defining feature of e-commerce is the facilitation of accessing the Point-of-Sale through the Internet. On the other hand, physical retail’s strength is its ability to shape the customer experience and provide personalized services.
However, one of the advantages leveraged by online retail is the ability to personalize the customer journey. Therefore, massive amounts of data can be acquired easily in a digital environment. This data can then be used to generate individual recommendations based on prior “click behavior.”
Physical retailers must transform their business into the digital world to keep up and play to their actual strengths. Therefore, new data acquisition methods are needed that are practical, compatible, and viable in an offline setting.
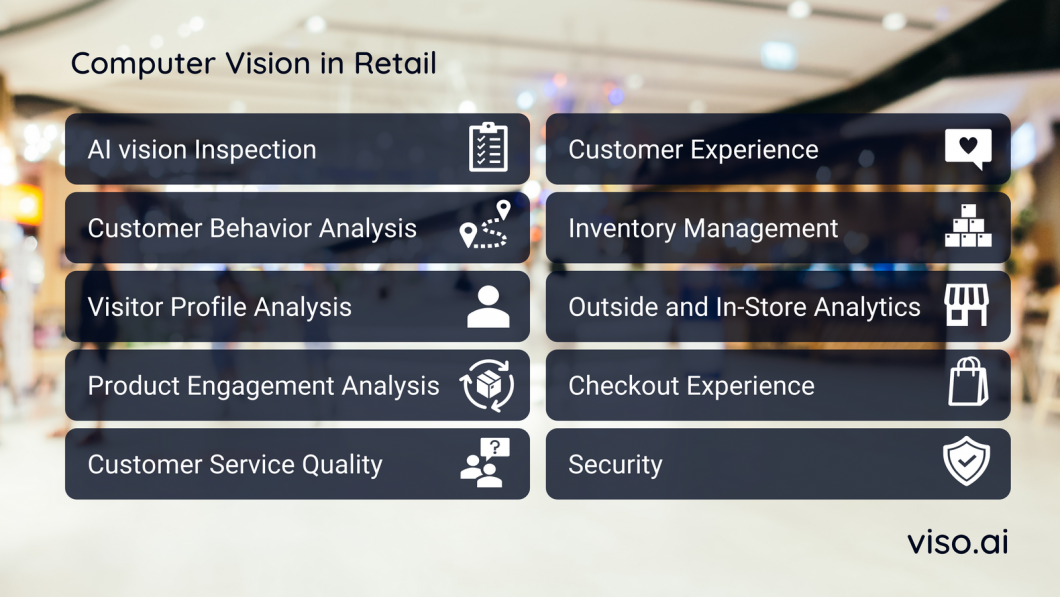
Data Acquisition Bottleneck
Most data acquisition systems are basic and differ greatly from the fancy, expensive showcases of large technology companies. It’s challenging to digitize the offline world. Retailers find it hard to scale sensing systems to collect valuable information. It is difficult to gather data in offline stores as layout changes can impact the customer experience.
Often, retailers experience limitations regarding equipment installations or layout changes when properties are rented, franchised, or externally managed. While sensors become more cost-effective, the costs of software, implementation, and maintenance can quickly exceed business value.
In-store Retail Analytics Technologies
A variety of sensors have been introduced to detect customers, count people, analyze footfall, and measure dwell times. Some popular sensors on the market are Xovis, SensMax, ShopperTrak, V-Count, and FLIR Brickstream. These types of sensors include:
- 3D sensors
- Cameras
- Lidar
- Time-of-Flight sensors
However, sensors are usually limited to specific tasks and require new hardware purchases and certain setups (height, distance, etc.).
Modern deep learning technology allows ML algorithm application to almost any video stream. The application software uses a camera video feed and applies AI video analytics in a multi-step process. This process is otherwise known as computer vision pipeline or flow.
Any network camera or even existing CCTV or surveillance cameras can be integrated with such computer vision systems. Thus, reducing the risk of sunk costs and hardware lock-in. Some cameras already provide some intelligence features, but those are often limited and not flexible or powerful enough.
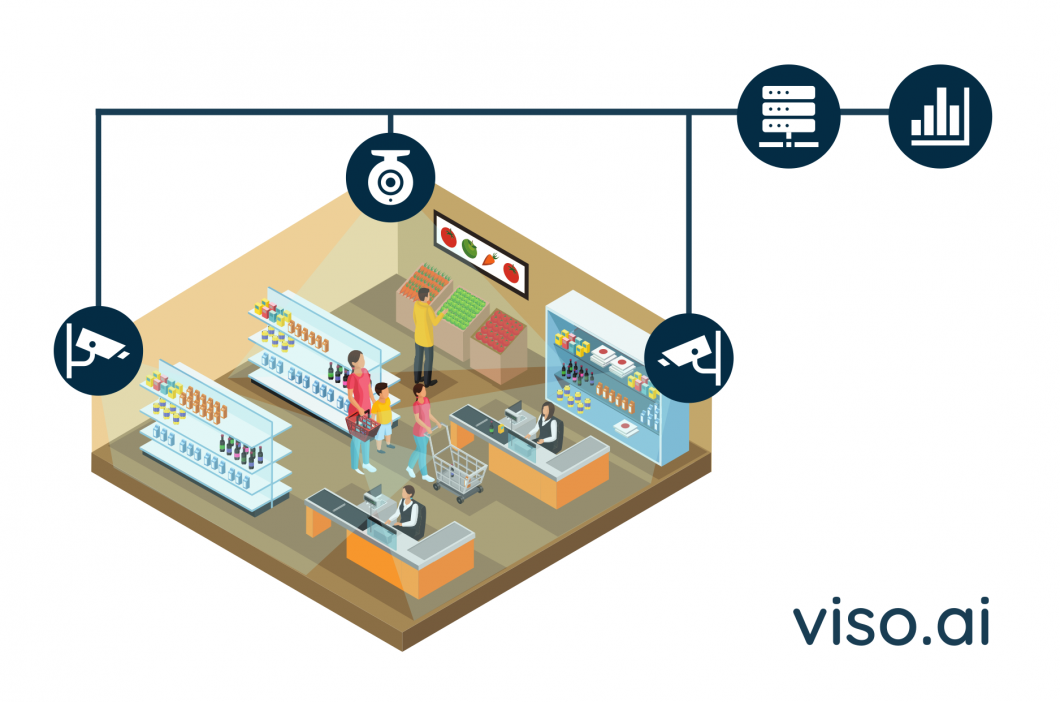
What is Computer Vision?
AI vision analyzes camera video with algorithms. This analysis provides traditional retailers with data-driven insights to improve the customer experience and strengthen their market position.
Computer Vision is a powerful technology to overcome the data acquisition bottleneck. Compared to other sensors, camera analysis requires much less installation equipment, is contactless, and can operate automatically.
Navigating the Sea of Sensors and Applications
In offline store digitization attempts, retailers tend to create massive lists of data they aim to gather with cameras. Ambitious digital strategies require the development and maintenance of a portfolio of applications. One computer vision application or system is not enough, and serious investment is required.
Proof of concepts (POC) or smaller implementations can often work well. However, issues usually appear in application scaling and operation in production outside of the optimized lab setting. In this phase, companies must have full data control and be able to tailor applications to specific business needs.
Hence, the implementation of a computer vision application platform helps build up internal capabilities, leverage synergies, standardize privacy and security, and prevent data silos.
Data Privacy for Computer Vision
Businesses must ensure complete data privacy per privacy protection laws at the state and federal levels. Therefore, privacy-preserving computer vision is a must-have for all vision intelligence systems in retail.
Any information that can be used to distinguish or trace an individual’s identity (Personally Identifiable Information, PII) is highly sensitive. Compared to human operators watching video streams, visual AI provides a fully automated method. In addition, there is a wide range of visual obfuscation methods, for example, face blurring. However, this may not be enough to comply with data privacy regulations.
Easy-to-use computer vision APIs are often used to develop prototype applications. These can include Google Vision API or AWS Rekognition. However, cloud-based AI processing sends data to the cloud and analyzes using remote resources of a data center. Most companies’ privacy policies do not allow this form of cloud-based computer vision.
New computer vision technologies leverage Edge AI concepts to process data on-device and in real time. This data processing is done without storing or transferring video to the cloud. Edge Computer Vision does not require a constant internet connection, allowing for much higher performance and cost-efficiency.
Hence, companies must have full control over the data flows and application design. This is to implement mechanisms that allow complete anonymization of videos analyzed by AI algorithms. Deep learning models can analyze video streams and convert them into an anonymous stream of metadata. This stream will not contain sensitive visuals without losing any valuable information the business is looking for.
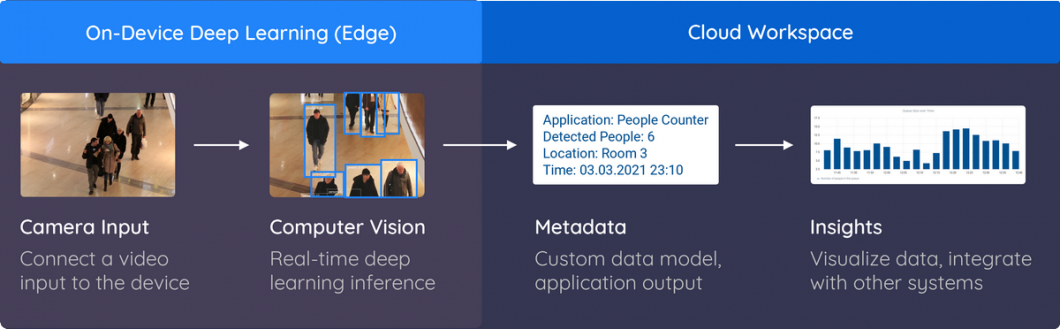
AI Vision Inspection and Compliance
AI technology with computer vision has been widely used to automate visual inspection tasks of all kinds.
- SOP Monitoring: Computer vision can be used to continuously track compliance with standard operating procedures (SOP). This aids in meeting safety and operational guidelines. Compared to human operators, AI vision analysis is continuous, subjective, and consistent.
- Following Branding Policies: Cameras can ensure adherence to branding policies. I.e., to analyze the presence or distribution of promotional material.
- Temperature and Control Monitoring: Machine learning algorithms can be used to read analog controls and temperature displays. Such an AI application can send alerts if thresholds are met.
- Staff Uniform Detection as per Policy: Detection of uniform clothing of staff to identify adherence to internal policies.
- Store Opening and Closing Time Analysis: Video analysis can detect retail store openings and closings to identify irregularities.
- Safety Compliance: Automatically detect dangerous events and situations in the store, such as unstable stacks or narrow aisles. AI vision is also widely used to monitor adherence to COVID-19 policies such as social distancing and mask detection.
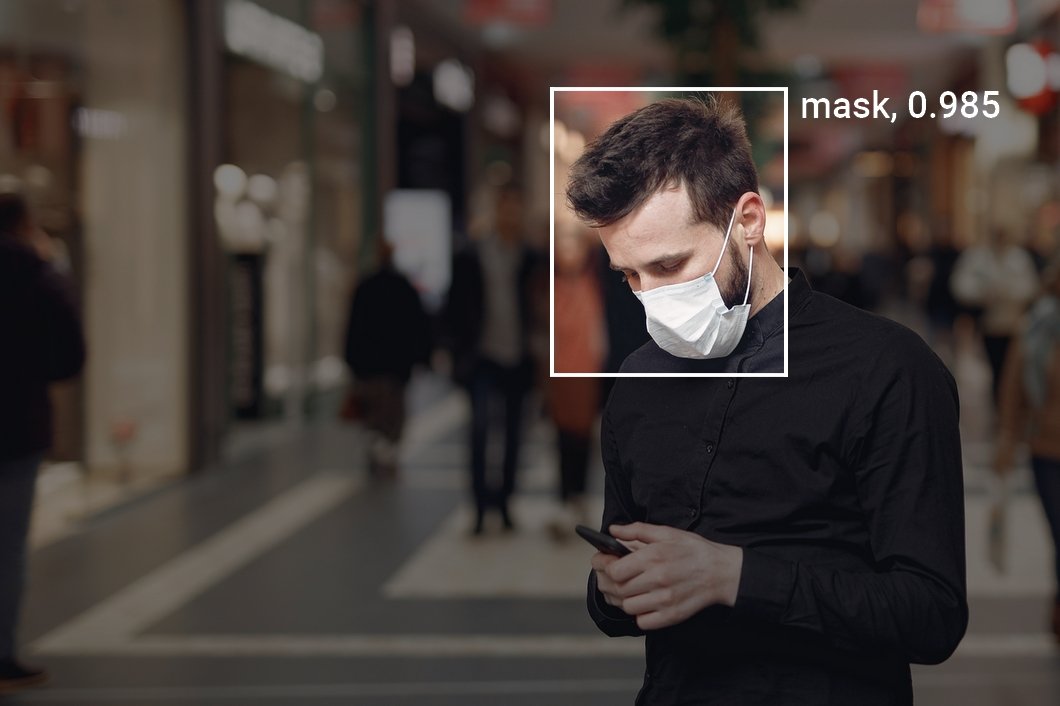
Customer Behavior Analysis
Deep learning for people detection and movement analysis is used to gain an understanding of customer behavior. Pose estimation and real-time object detection are technologies used to provide data and metrics about the shopper journey. In-store consumer analytics are often modeled after online retail analytics, people’s paths vs. e-commerce page visits.
- Average Time Spent (Dwell Time): AI algorithms can estimate the average time a customer spends in the shop. The dwell time provides valuable insights for marketing and operations.
- Customer Visit Time Distribution: Identifies the number of visitors per hour to optimize staffing and planning decisions.
- People Counting and Flow Analytics: Customer footfall analysis tracks customer paths inside the retail store. Information about customer paths is often visualized as a heat map or spaghetti diagram.
- Shopper Behavior Analysis: Image classification within certain regions of interest detects important shopper behavior. I.e., to analyze the use of self-checkouts.
- Trolley Against Baskets: Generate data about whether customers use trolleys or baskets while shopping.
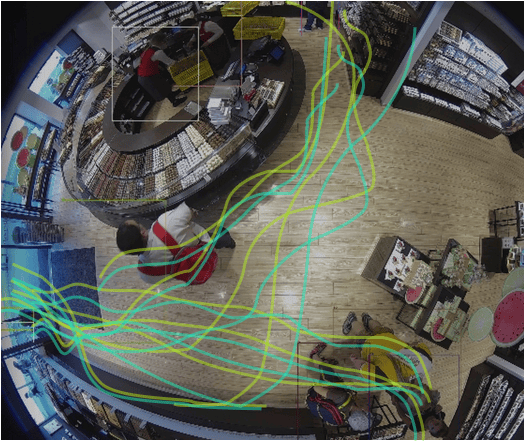
Visitor Profile Analysis
In-store analytics often involves the classification of visitors into groups or customer types. Deep-learning algorithms can classify visitors by gender, mood, activity, or other characteristics. Such customer analytics can be combined with other data in business intelligence tools for valuable retail solutions.
- Gender Distribution of Visitors: Machine learning algorithms with facial analysis can detect the gender profile of customer groups.
- Demographics Analytics: Use deep learning models to estimate the age and gender or cultural background of customers automatically.
- Shopper Profile Against Shelf Visits: AI-based classification algorithms categorize customer characteristics and link them to specific store shelves.
- Basket Size: Estimate basket fill and shopping cart fill levels across different hours and customer groups.
- Emotion Analysis: Emotion recognition aims to classify the facial expressions of customers. This is to analyze the sentiments of customer groups in specific areas (“regions of interest”).
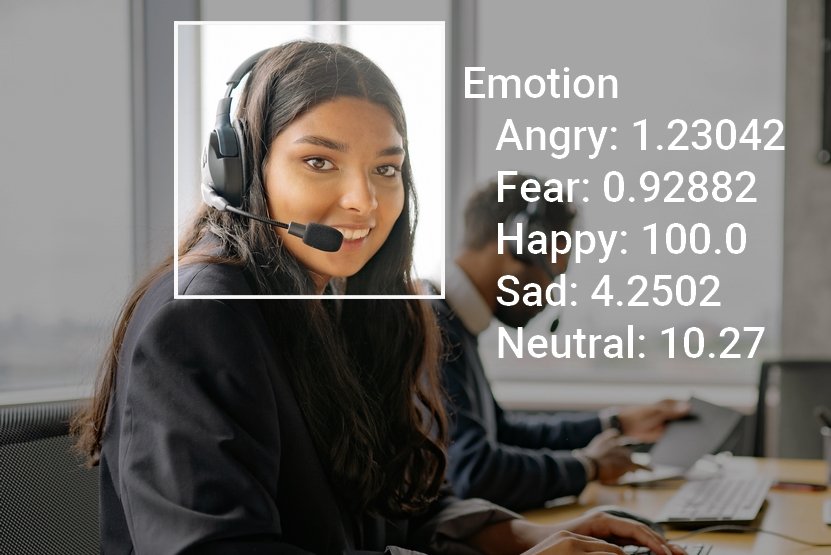
Product Engagement Analysis With Computer Vision for Retail
Image recognition with one or multiple cameras can determine what products customers are interacting with, considering, or abandoning. Digitizing product engagement and the in-shop buying process can provide valuable insights to retail store managers.
This type of in-store analytics is essential for understanding and optimizing the on-site buying process. Cashierless stores combine multiple methods, with a high number of different cameras and multiple sensors.
- Hot Zones and Hot Shelves (Attention): AI camera analysis identifies popular shelves or locations inside the store. The information can be used to optimize promotions and product placements.
- Count the Visits per Shelf or Area (Aisle Usage): the number of interested customers stopping at shelves or aisles. The data shows changes or irregularities over time.
- Shelf-level Offtakes: Object detection can analyze the offtakes of products from shelves at different heights. This can be used to analyze the low-level shelf offtake.
- Product Oon-shelf Interaction: Movement analysis can detect situations where a product is picked up but placed back.
- Zero Purchase Shoppers: Detect and count the number of visitors who leave the store without making a purchase.
- Average Time Spent on Key Aisles or Areas: Gather the customer dwell time at regions of interest.
- Store-in-store Analysis: Analyze product categories and special shelves. This helps measure customer engagement in shop-in-shop areas.
- Logo Recognition: Automatically recognize logos and detect logo placements to analyze brand exposure.
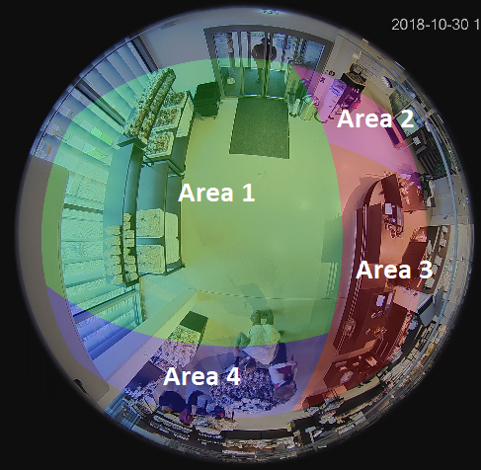
Customer Service Quality
Video AI can identify factors that determine in-store interactions. Hence, retailers can generate data about the retail service quality at the point of sale (POS).
- Occupancy Detection: Detect if the personnel is present at assigned points. These can include aisles, service desks, checkout, cash registers, self-checkout, or entries (occupancy analysis).
- Staff Engagement Levels: Recognize customer-staff interactions to measure the engagement levels across different stores.
- Queue Detection (Line Counting): AI camera analysis detects queues waiting at service desks or during the retail checkout process.
- Missed Rings: Detect and track the number of times when customer service staff disregards customer requests.
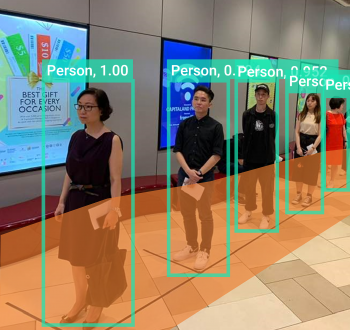
Customer Experience
AI vision can identify and track parameters that directly impact the customer experience in a retail location.
- Shopping Cart and Basket Availability: Real-time object detection for basket or trolley supply monitoring and distribution. Allowing stores to localize carts automatically.
- Customer Experience Factors: Detect metrics for waiting times, crowd density, or queue length.
- Empty Shelves Visited: Identify and count occurrences where people did not find what they were looking for.
- Staff Attentiveness: Spot situations and events where employees use their mobile phones while customers are present or waiting.
- Kiosk Activation: Enable kiosks or interactive screens automatically when customers are present. This can verify that sales displays are working as intended.
Backroom and Inventory Management
- Real-time Object Detection: Security cameras recognize specific products, boxes, or pallets delivered or stored in specific areas.
- Out-of-stock Alerts: Image recognition can detect longer out-of-stock situations to improve inventory levels and accuracy.
- Loading Dock Theft: Retail video analytics detects and prevents loading dock theft where goods are permanently or temporarily stored.
- Logistics and Warehousing: Detect misplaced objects in warehouses, useful for both brick-and-mortar and online stores. Explore our article with 25+ Applications of Computer Vision in Logistics.
- Label and Barcode Detection: Optical Character Recognition (OCR) reads labels, barcodes, QR codes, or text on containers and packages.
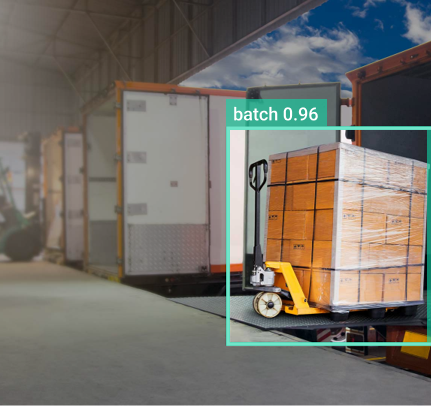
In-store and Outside AI inspection
Regular security cameras in stores can be used to implement continuous store inspections with AI vision. Here, visual sensing can automate manual inspection tasks effectively.
- Cleanliness: Use AI vision to detect if the shop floor is clean and clear. Use cleanliness analysis to determine whether shop shelves, billboards, and columns are intact, clean, and complete.
- Store Accessibility: Analyze the pavement and entrance area outside of the store to identify and optimize people flows.
- Vehicles Parked in Front of the Store: Vehicle detection to recognize parked vehicles. Businesses can then take action to ensure vehicles do not block the shop entrance for an extended time.
- Parking Lot Occupancy Analysis: Recognize if parking spaces are occupied or available (Parking lot analysis).
- Door Operation: People detection to analyze if automatic doors operate and open efficiently.
- Shop Floor Obstacles: Identify obstacles hindering visitor flow inside the store. I.e., misplaced boxes or promotional material.
- Event Detection: Camera-based spill and fall detection or crash alerts help identify events and measure responding time.
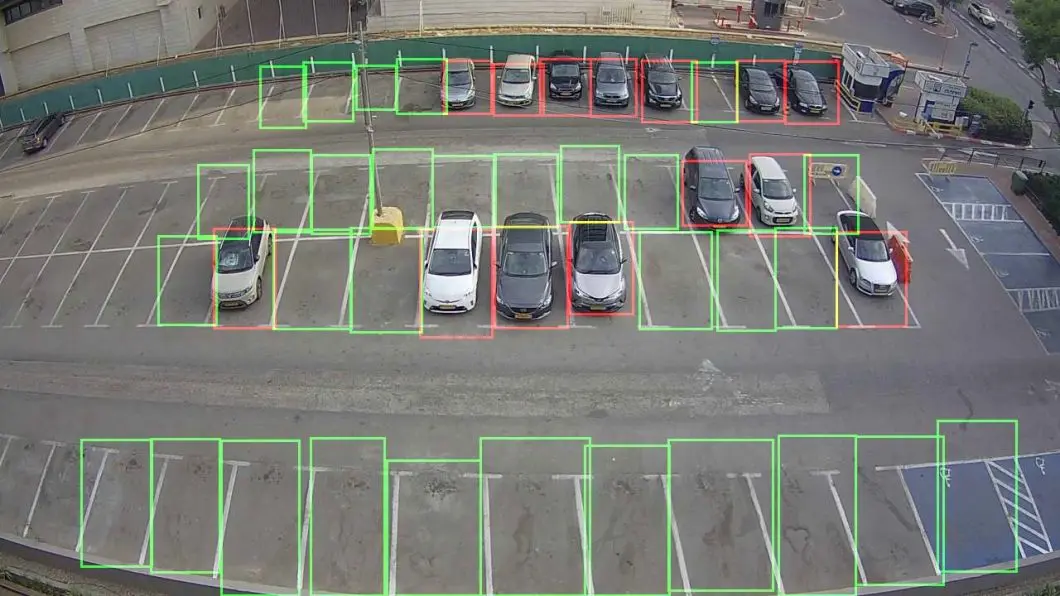
Checkout Experience
- Cash Register Occupancy: Recognize if checkout systems are occupied whenever there are customers.
- Counter Cleanliness: Implement ways to organize counters well and ensure that there is enough room for customer service.
- Customer Checkout Time: Measure the average customer checkout times across different counters and identify unusual waiting times.
- Self Checkout: Analyze self-checkout machines occupancy to identify if more staff assistance or checkout desks are needed.
Security and Safety
- Presence of Fire Extinguishers: Implement an automated inspection to ensure fire extinguishers and other safety gear are constantly available.
- Accessibility of Emergency Exits: Use intelligent security and surveillance camera systems to constantly monitor the emergency exits and detect and remove obstacles early.
- Detect Intrusion: Detect intrusion events with automated people detection during times when the stores are closed. Automatically identify and report suspicious activities.
- Detection of Shoplifting: Deep learning algorithms have been used for loss prevention to detect shoplifting or unattended registers. This task is rather difficult because people may pay for the goods later.
- Self-Checkout Monitoring: Detect pass-around and empty carts, the bottom of the baskets, and ticket switching automatically.
- Unrestricted Access Alert: Recognize unauthorized access to restricted areas, for example, behind the counter or in staff-only areas.
- Authentication: Face recognition to identify staff members in restricted areas and unauthorized activities by those with temporary access. I.e., suppliers or visitors entering the store.
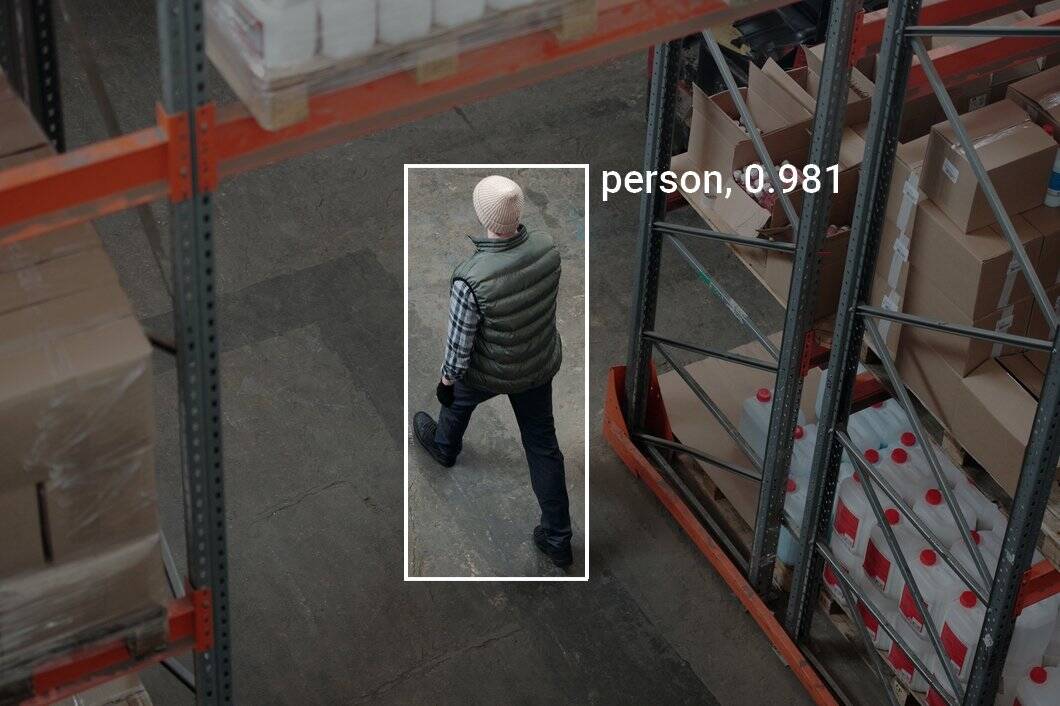
How to Get Started With Computer Vision in Retail
Visual AI retail has enormous potential and the list of use cases continues to grow. Specifically, multi-modal retail solutions grow this potential tenfold, namely generative AI and natural language processing.
For organizations looking to implement computer vision systems, it’s important to realize that each application may be difficult and require a significant financial commitment. Check out our business guide “What Does Computer Vision Cost?” to learn more about the costs and pricing of AI software and services.
Organizations using visual AI for business must have:
- Full understanding of the data at play
- Complete control over the application pipeline
- High agility to adjust and change applications for their needs due to continuously evolving regulations and business requirements.
Hence, companies establish Proof-of-Concept (PoC) and Pilot projects to gather operational experience with applying AI vision.
Novelty and technical difficulty usually mean that organizations must start adopting AI as early as possible. Due to the highly disruptive nature of AI technology, catching up with competitors later will be difficult and expensive.
Compared to web applications or standard software, AI systems are considerably more sophisticated. Especially real-world applications require connected sensors, advanced computing algorithms, and compute-intensive deep learning workloads. A computer vision application is far more than only the ML model. It includes image acquisition, business logic, data formatting, data aggregation, and integration into other systems.
Initially, companies often start with various PoC or Pilot projects to identify and validate high-value strategies. In later stages, it does not only involve technical feasibility. Teams must consider other factors such as security, scalability, cost-efficiency, and flexibility of applications that determine the economic potential of visual AI applications.
Using the Viso Suite Platform
Most organizations will need multiple AI vision applications. However, it can be challenging to develop and maintain such a portfolio efficiently. This is why companies use our computer vision application platform Viso Suite™.
The end-to-end platform enables their teams to build, deploy, operate, and scale all applications in one software suite. Viso provides end-to-end automated development features for professionals to accelerate every step of the lifecycle. From image annotation and training to application development, deployment, device management, remote configuration, and monitoring.
Fortune 500 and Governmental Organizations worldwide deliver their AI vision applications using Viso Suite™ technology. The model-driven architecture and automated infrastructure are fully optimized for Edge AI and real-time computer vision. Read the solution brief by Intel.
These qualities make the computer vision platform completely extensible. Thus, allowing for the integration into Business Intelligence tools or the use of existing ML models and software containers.
If you are looking for enterprise-grade computer vision, request a personalized demo.
Check out other articles from the Viso Blog: