COCO is a visual dataset that plays an important role in computer vision. In this article, we will cover everything you need to know about the popular Microsoft COCO dataset that is widely used for machine learning Projects. Learn what you can do with MS COCO and what makes it different from COCO alternatives such as Google’s OID (Open Images Dataset).
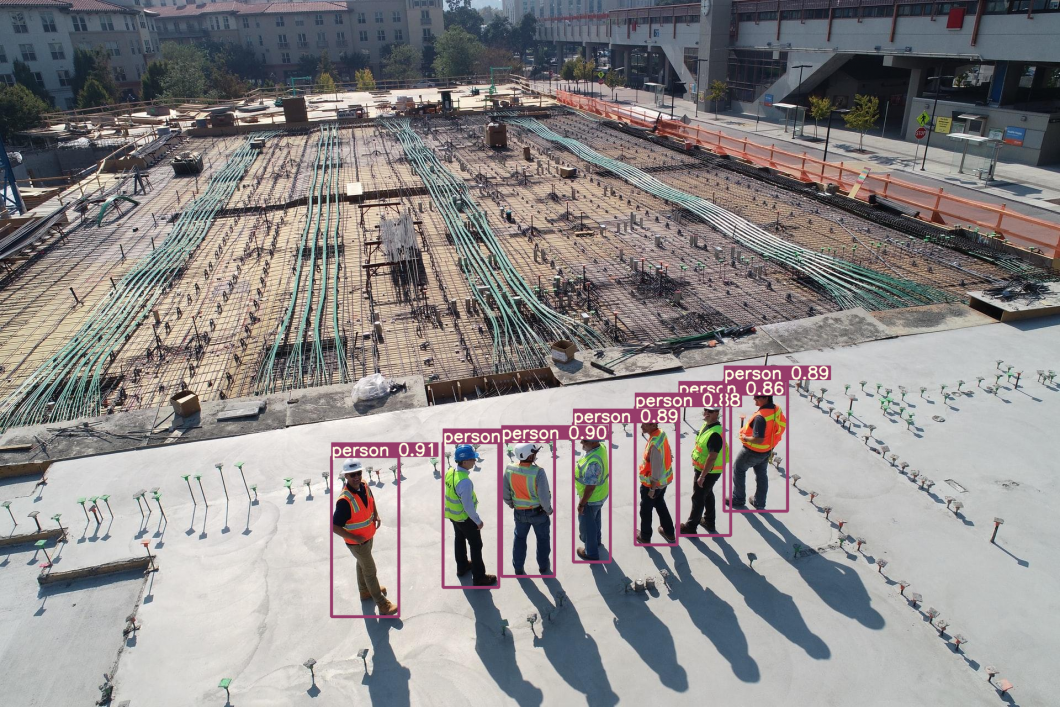
The COCO Dataset
The MS COCO dataset is a large-scale object detection, image segmentation, and captioning dataset published by Microsoft. Machine Learning and Computer Vision engineers popularly use the COCO dataset for various computer vision projects.
Understanding visual scenes is a primary goal of computer vision; it involves recognizing what objects are present, localizing the objects in 2D and 3D, determining the object’s attributes, and characterizing the relationship between objects. Therefore, algorithms for object detection and object classification can be trained using the dataset.
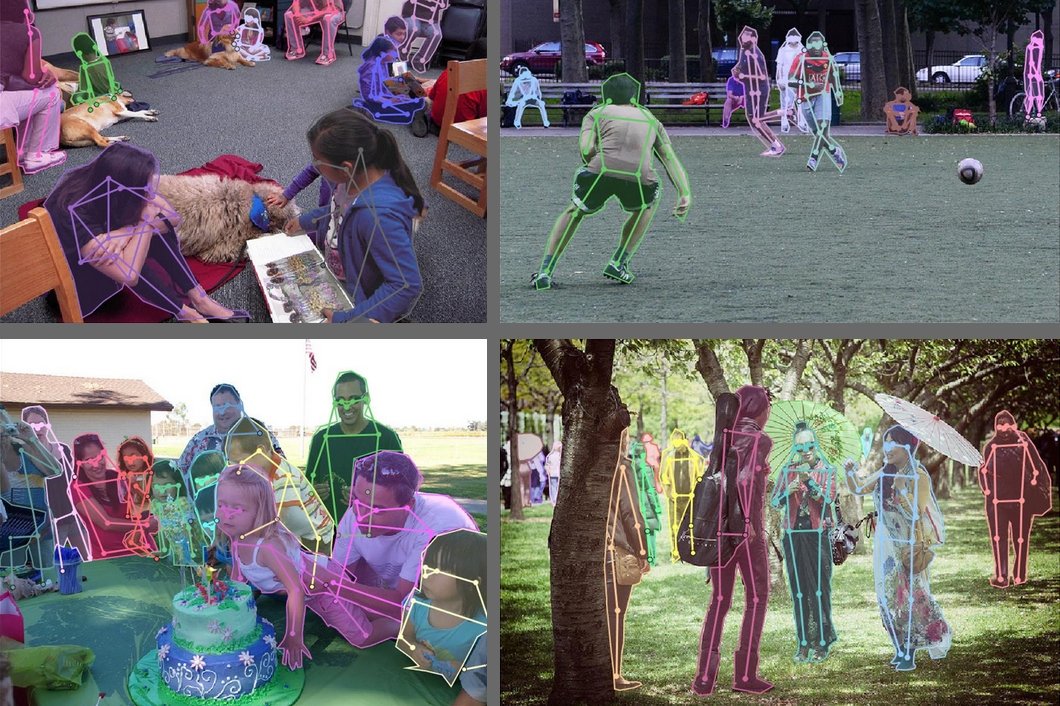
What is COCO?
COCO stands for Common Objects in Context dataset, as the image dataset was created to advance image recognition. The COCO dataset contains challenging, high-quality visual datasets for computer vision, mostly state-of-the-art neural networks.
For example, COCO is often used to benchmark algorithms to compare the performance of real-time object detection. The format of the COCO dataset is automatically interpreted by advanced neural network libraries.
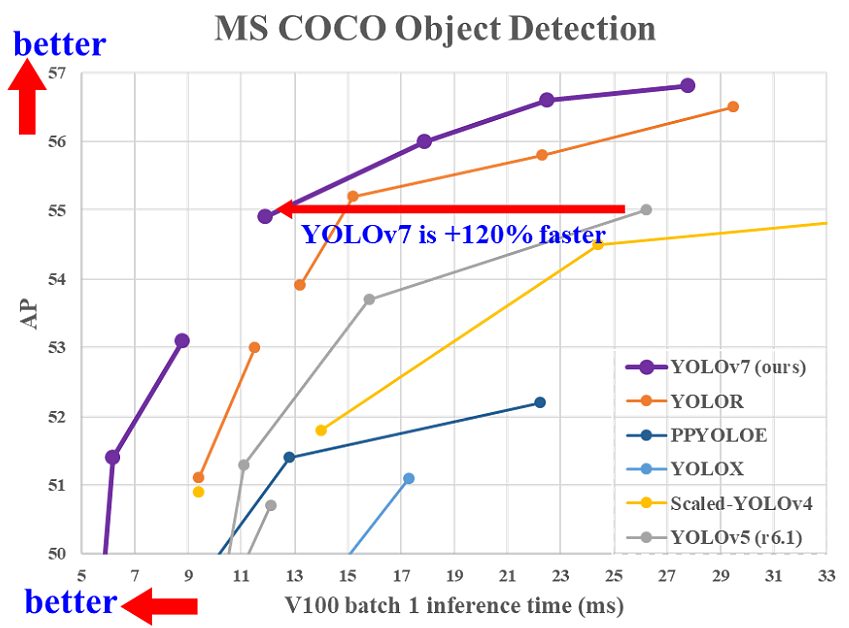
Features of the COCO dataset
- Object segmentation with detailed instance annotations
- Recognition in context
- Superpixel stuff segmentation
- Over 200’000 images of the total 330’000 images are labeled
- 1.5 Mio object instances
- 80 object categories, the “COCO classes”, which include “things” for which individual instances may be easily labeled (person, car, chair, etc.)
- 91 stuff categories, where “COCO stuff” includes materials and objects with no clear boundaries (sky, street, grass, etc.) that provide significant contextual information.
- 5 captions per image
- 250’000 people with 17 different key points, popularly used for Pose Estimation
List of the COCO Object Classes
The COCO dataset classes for object detection and tracking include the following pre-trained 80 objects:
'person', 'bicycle', 'car', 'motorcycle', 'airplane', 'bus', 'train', 'truck', 'boat', 'traffic light', 'fire hydrant', 'stop sign', 'parking meter', 'bench', 'bird', 'cat', 'dog', 'horse', 'sheep', 'cow', 'elephant', 'bear', 'zebra', 'giraffe', 'backpack', 'umbrella', 'handbag', 'tie', 'suitcase', 'frisbee', 'skis','snowboard', 'sports ball', 'kite', 'baseball bat', 'baseball glove', 'skateboard', 'surfboard', 'tennis racket', 'bottle', 'wine glass', 'cup', 'fork', 'knife', 'spoon', 'bowl', 'banana', 'apple', 'sandwich', 'orange', 'broccoli', 'carrot', 'hot dog', 'pizza', 'donut', 'cake', 'chair', 'couch', 'potted plant', 'bed', 'dining table', 'toilet', 'tv', 'laptop', 'mouse', 'remote', 'keyboard', 'cell phone', 'microwave', 'oven', 'toaster', 'sink', 'refrigerator', 'book', 'clock', 'vase', 'scissors', 'teddy bear', 'hair drier', 'toothbrush'
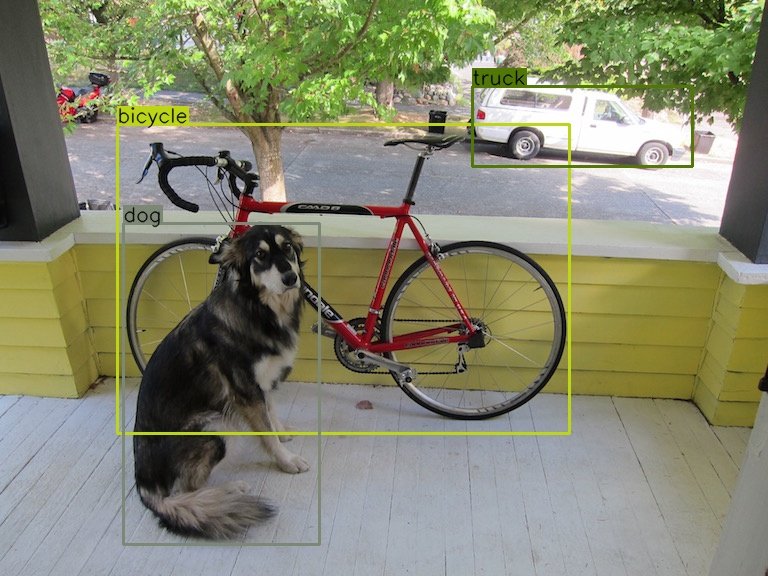
List of the COCO key points
The COCO key points include 17 different pre-trained key points (classes) that are annotated with three values (x,y,v). The x and y values mark the coordinates, and v indicates the visibility of the key point (visible, not visible).
"nose", "left_eye", "right_eye", "left_ear", "right_ear", "left_shoulder", "right_shoulder", "left_elbow", "right_elbow", "left_wrist", "right_wrist", "left_hip", "right_hip", "left_knee", "right_knee", "left_ankle", "right_ankle"
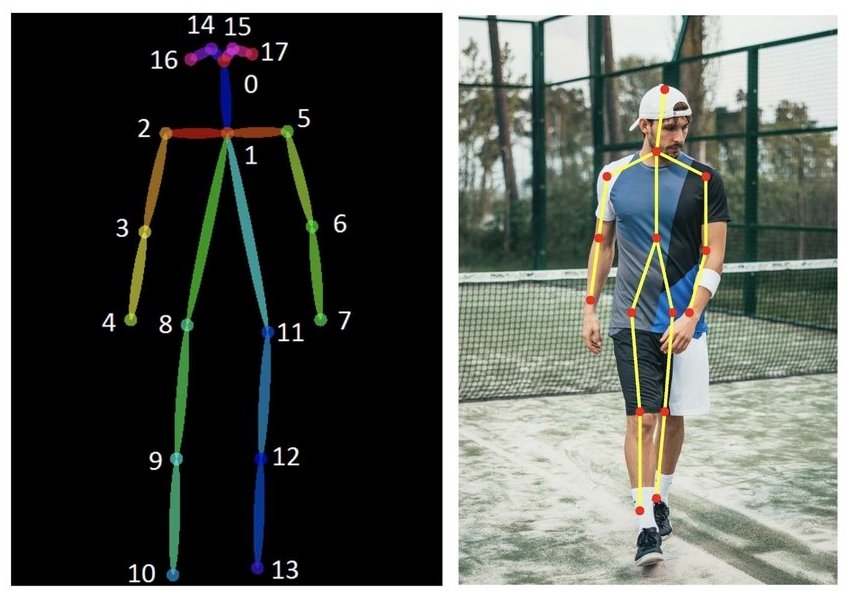
Annotated COCO images
The large dataset comprises annotated photos of everyday scenes of common objects in their natural context. Those objects are labeled using pre-defined classes such as “chair” or “banana”. The process of labeling, also named image annotation is a very popular technique in computer vision.
While other object recognition datasets have focused on 1) image classification, 2) object bounding-box localization, or 3) semantic pixel-level instance segmentation – the mscoco dataset focuses on 4) segmenting individual object instances.
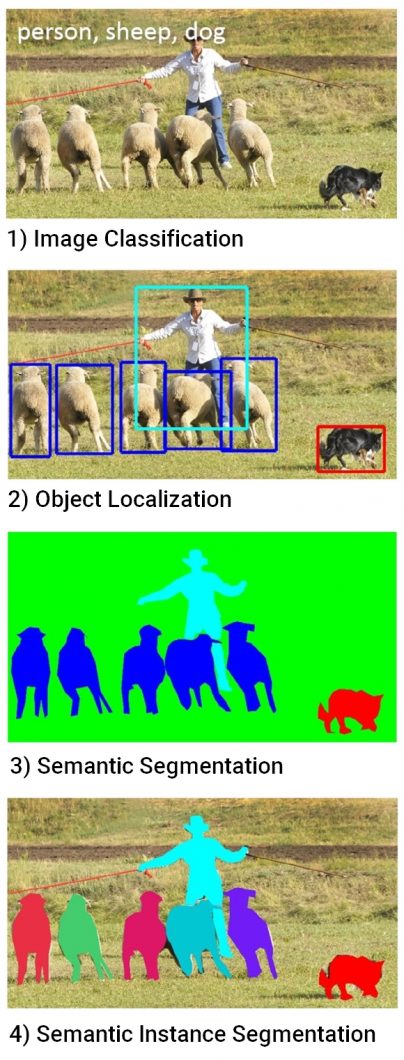
Why are common objects in a natural context?
For many categories of objects, there are iconic views available. For example, when performing a web-based image search for a specific object category (for example, “chair”), the top-ranked examples appear in profile, unobstructed, and near the center of a very organized photo. See the example images below.
While image recognition systems usually perform well on such iconic views, they struggle to recognize objects in real-life scenes that show a complex scene or partially occlude the object. Hence, it is an essential aspect of the coco images that they contain natural images that contain multiple objects.
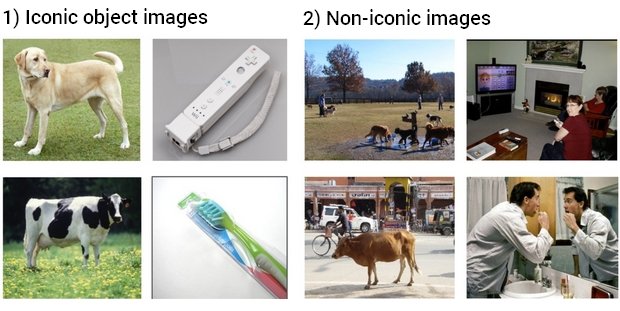
How to use the COCO Computer Vision dataset
Is the COCO dataset free to use?
Yes, the MS COCO images dataset is licensed under a Creative Commons Attribution 4.0 License. Accordingly, this license lets you distribute, remix, tweak, and build upon your work, even commercially, as long as you credit the original creator.
How to download the COCO dataset
There are different dataset splits available to download for free. Each year’s images are associated with different tasks such as Object Detection, point tracking, Image Captioning, and more.
To download them and see the most recent Microsoft COCO 2020 challenges, visit the official MS COCO website. To efficiently download the COCO images, it is recommended to use gsutil rsync to avoid the download of large zip files. You can use the COCO API to set up the downloaded COCO data.
COCO recommends using the open-source tool FiftyOne to access the MSCOCO dataset for building computer vision models.
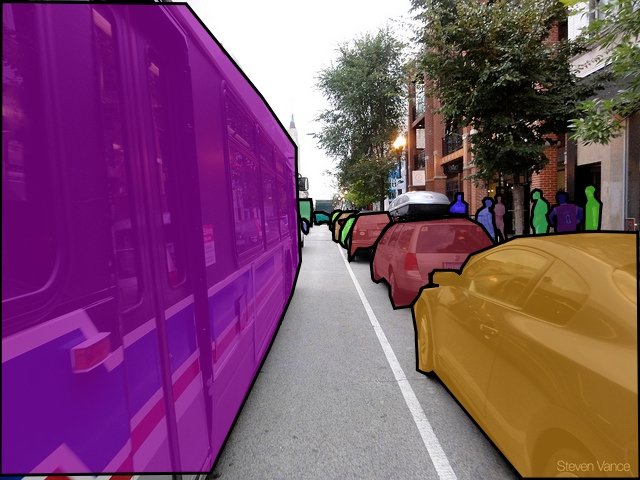
Comparison of COCO Dataset vs. Open Images Dataset (OID)
A popular alternative to the COCO Dataset is the Open Images Dataset (OID), created by Google. It is essential to understand and compare the visual datasets COCO and OID with their differences before using one for projects to optimize all available resources.
Open Images Dataset (OID)
What makes it unique? Google annotated all images in the OID dataset with image-level labels, object bounding boxes, object detection segmentation masks, visual relationships, and localized narratives. This leaves it to be used for slightly more computer vision tasks when compared to COCO because of its slightly broader annotation system. The OID home page also claims it’s the largest existing dataset with object location annotations.
Data. Open Image is a dataset of approximately 9 million pre-annotated images. Most, if not all, images of Google’s Open Images Dataset have been hand-annotated by professional image annotators. This ensures accuracy and consistency for each image and leads to higher accuracy rates for computer vision applications when in use.
Common Objects in Context (COCO)
What makes it unique? With COCO, Microsoft introduced a visual dataset that contains a massive number of photos depicting common objects in complex everyday scenes. This sets COCO apart from other object recognition datasets that may be specific sectors of artificial intelligence. Such sectors include image classification, object bounding box localization, or semantic pixel-level segmentation.
Meanwhile, the annotations of COCO are mainly focused on the segmentation of multiple, individual object instances. This broader focus allows COCO to be used in more instances than other popular datasets like CIFAR-10 and CIFAR-100. However, compared to the OID dataset, COCO does not stand out too much, and in most cases, both could be used.
Data. With 2.5 million labeled instances in 328k images, COCO is a very large and expansive dataset that allows many uses. However, this amount does not compare to Google’s OID, which contains a whopping 9 million annotated images.
Google’s 9 million annotated images were manually annotated, while OID discloses that it generated object bounding boxes and segmentation masks using automated and computerized methods. Both COCO and OID have not disclosed bounding box accuracy, so it remains up to the user whether they assume automated bounding boxes would be more precise than manually made ones.
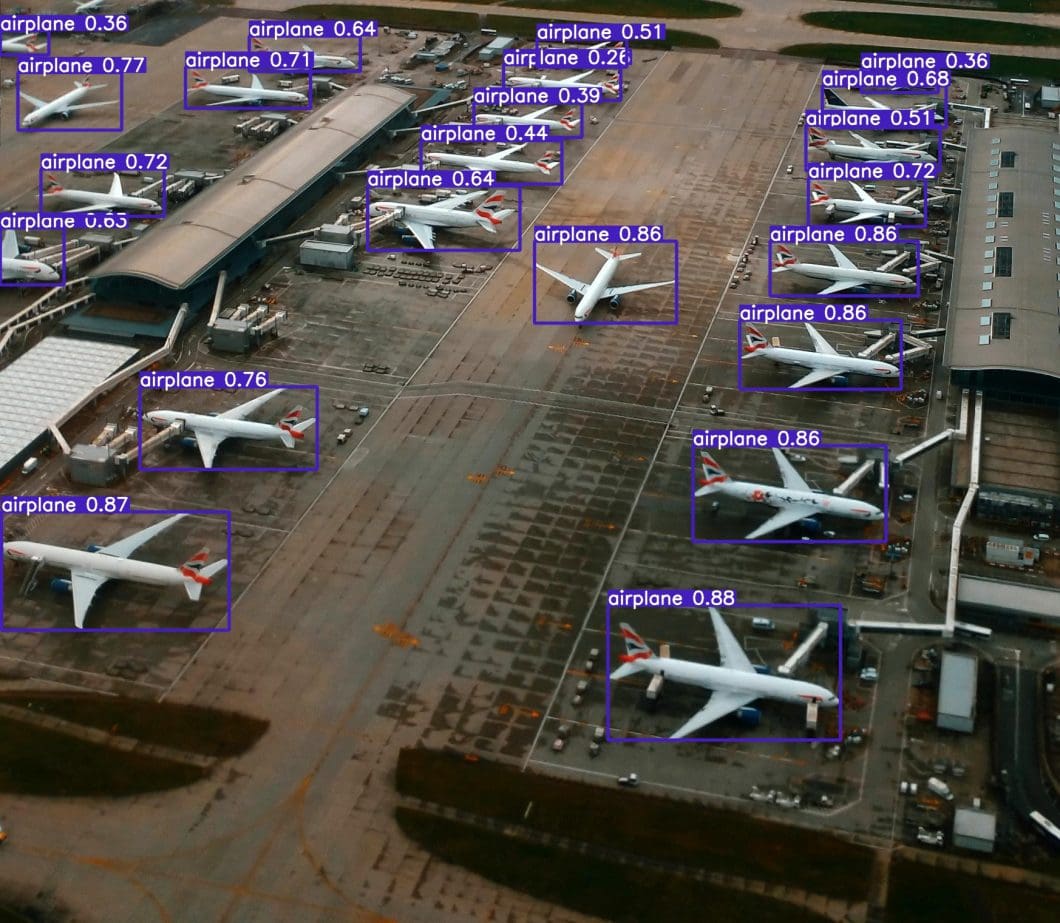
What’s Next?
The COCO dataset and benchmark are used in a wide range of AI vision tasks and disciplines. Models trained on COCO are used for object detection, people detection, face detection, pose estimation, and many more computer vision tasks.
Check out the following related articles: