Image annotation plays a significant role in computer vision, the technology that allows computers to gain a high-level understanding of digital images or videos. Annotation, or image tagging, is a primary step in the creation of image recognition algorithms and deep learning models.
The software platforms used for image annotation have greatly advanced over the past years. Key industry trends include data security and privacy. There is a growing need to standardize and integrate how companies acquire training data, annotate it, train models, and use them in applications.
In particular, this article will discuss:
- What is image annotation, and why is it needed?
- Process of annotating images: Successfully annotate image datasets
- Annotation solutions: Best software platforms for image annotation
About us: We provide the Viso Suite, the world’s only end-to-end computer vision platform. One solution with revolutionary infrastructure for image annotation, data collection, datasets, model training, application development, and deployment. Get a demo for your team.
What is Image Annotation?
Image annotation is the process of labeling images of a dataset to train a machine learning model. Therefore, image annotation is used to label the features you need your system to recognize. Training an ML model with labeled data is called supervised learning (see supervised vs. unsupervised learning).

The annotation task usually involves manual work, sometimes with computer-assisted help (see Segment Anything Model). A Machine Learning engineer predetermines the labels, known as “classes”, and provides the image-specific information to the computer vision model. After the model is trained and deployed, it will predict and recognize those predetermined features in new images that have not been annotated yet.
Popular annotated image datasets are the Microsoft COCO Dataset (Common Objects in Context), with 2.5 million labeled instances in 328k images, and Google’s OID (Open Images Database) dataset, with approximately 9 million pre-annotated images.
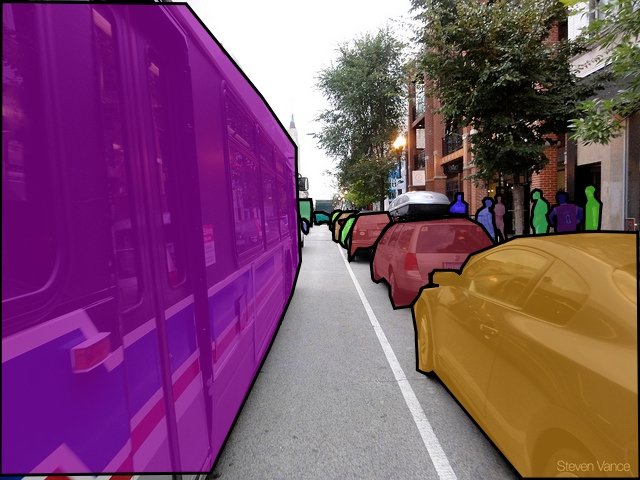
Why is Image Annotation needed?
Labeling images is necessary for functional datasets because it lets the training model know what the important parts of the image are (classes) so that it can later use those notes to identify those classes in new, never-before-seen images.
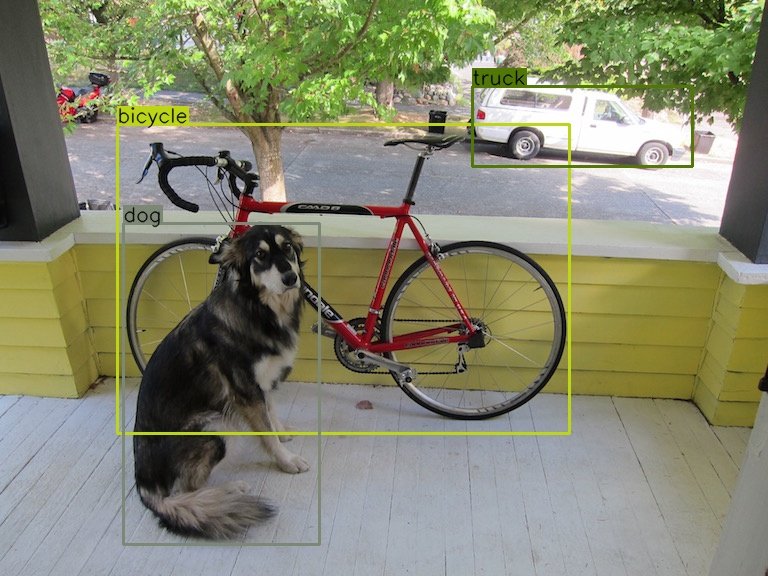
Video Annotation Tools
Video annotation is based on the concept of image annotation. For video annotation, features are manually labeled on every video frame (image) to train a machine-learning model for video detection. Hence, the dataset for a video detection model is comprised of images for the individual video frames.
The video below shows video-based real-time object detection and tracking with deep learning. The application was built on the Computer Vision Platform Viso Suite.
Some of the video annotation tools are listed below.
VGG Image Annotator (VIA): This is an open-source image and video annotation tool that supports annotation types such as bounding boxes, polygons, lines, etc. It also allows for customization of the annotation interface and provides support for various image and video formats.
Labelbox: This is one of the popular video annotation tools that offers a friendly user interface along with a wide range of annotation types. It also offers automation features such as pre-built models and workflows.
Dataloop: This is a cloud-based video annotation tool that offers a range of annotation types, automation features as well as real-time collaboration for team members.
When do I Need to Annotate Images for Computer Vision?
To train and develop computer vision algorithms based on deep neural networks (DNN), data annotation is needed in cases where pre-trained models are not specific or accurate enough.
As mentioned before, there are enormous public image datasets available, with millions of image annotations (COCO, OID, etc.). For common and standardized object detection problems (e.g. person detection), an algorithm that is trained on a massive public dataset ( pre-trained algorithm) provides very good results and the benefits of additional labeling do not justify the high additional costs in those situations.
However, in some situations, image annotation is essential:
- New Tasks: Hence, image annotation is important when AI is applied to new AI tasks without appropriate annotated data available. For example, in industrial automation, computer vision is frequently applied to detect specific items and their condition.
- Restricted Data: While there is plenty of data available on the internet, some image data requires a license agreement and its use may be restricted for the development of commercial computer vision products. In some areas such as medical imaging, manual data annotation generally comes with privacy concerns, when sensitive visuals (faces, identifiable attributes, etc.) are involved. Another challenge is the use of images that contain a company’s intellectual property.
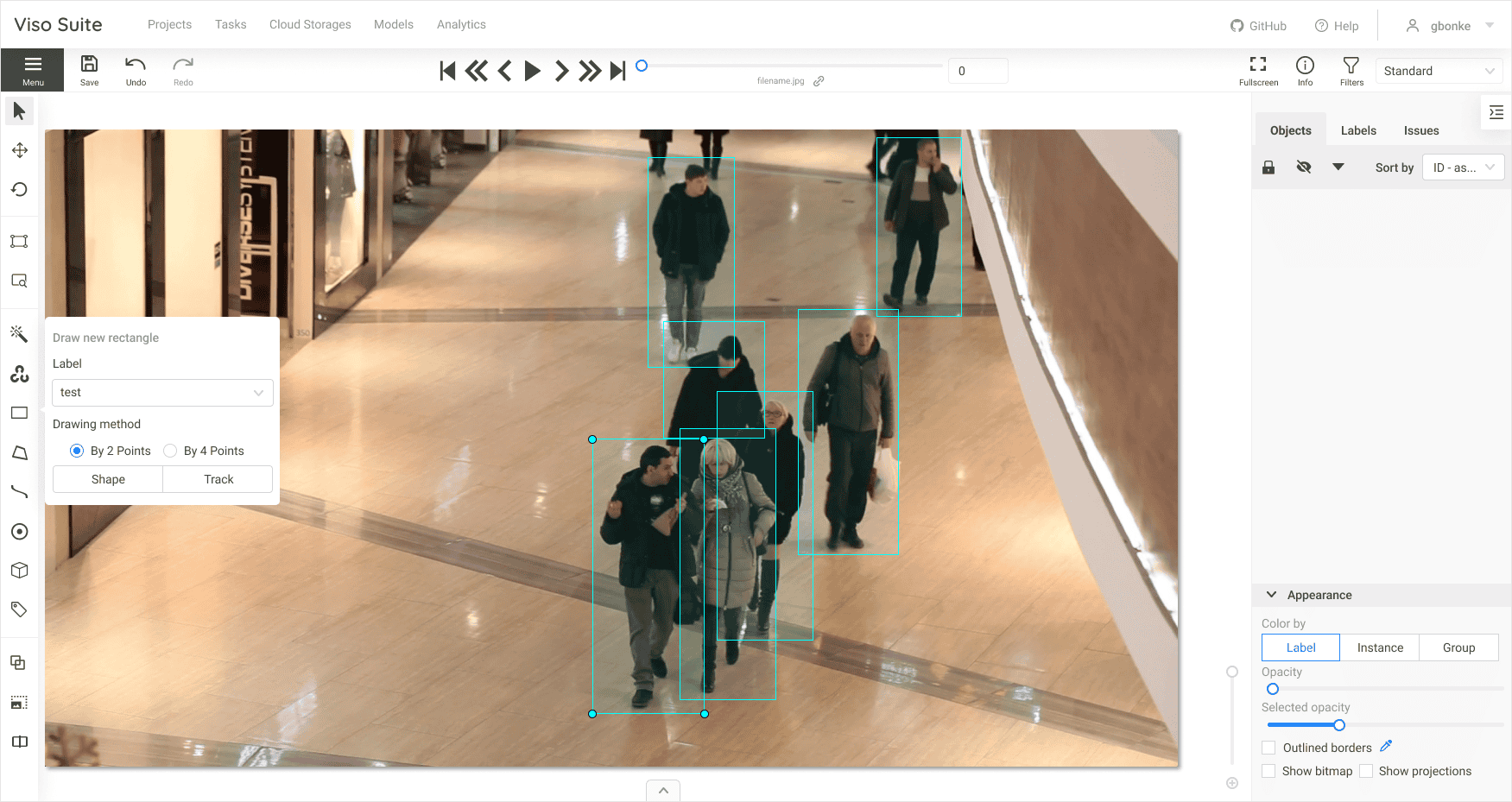
How does Image Annotation Work?
To annotate images, you can use any open-source or freeware data annotation tool. The Computer Vision Annotation Tool (CVAT) is probably the most popular open-source image annotation tool.
While dealing with a large amount of data, a trained workforce will be required to annotate the images. Companies use their own data scientists to label images, but more complex, real-world projects often require hiring an AI video annotation service provider.
The annotation tools offer different sets of features to annotate single or multiple frames efficiently. Labels are applied to the objects using any of the techniques explained below within an image; the number of labels on each image may vary, depending upon the use case.
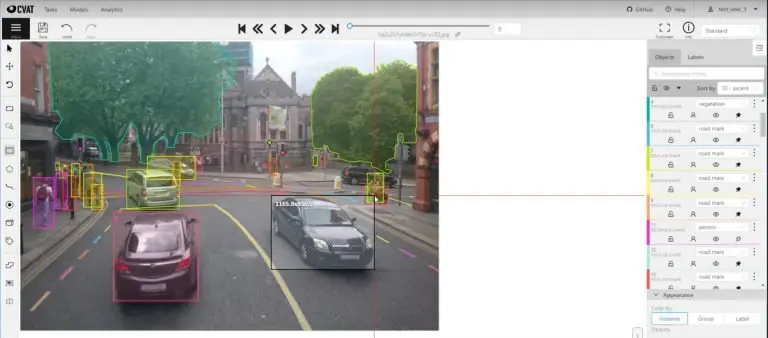
How to Annotate Images?
In general, this is how image annotation works:
- Step #1: Prepare your image dataset.
- Step #2: Specify the class labels of objects to detect.
- Step #3: In every image, draw a box around the object you want to detect.
- Step #4: Select the class label for every box you drew.
- Step #5: Export the annotations in the required format (COCO JSON, YOLO, etc.)
Free Image Annotation Tools
We tested the top free AI software tools for image annotation tasks. If you are looking for professional and enterprise image annotation solutions, we listed them further below.
Here is which free image annotation tool is the best for you:
MakeSense.AI
Makesense.ai is a free online tool for labeling photos that does not require any software installation. You can use it with a browser, and it doesn’t need any complicated installations. Makes sense ai is built on the TensorFlow.js engine, one of the most popular frameworks for training neural networks.
While the tool provides basic functionality that is easily accessible, it provides a good alternative for fast image annotation testing and small projects. The web-based image annotation tool MakeSense.AI is free to use under the GPLv3 license. GitHub Stars: 1.8k
- No installation is required; the tool is fully online.
- Makesense.ai supports multiple annotation shapes.
- Fast way to annotate a picture or a set of photos without installing software.
- A good option for beginners, this annotation tool walks the user through the annotation process.
- The annotation tool features a modern interface and new, time-saving add-ons that are appealing for large datasets.
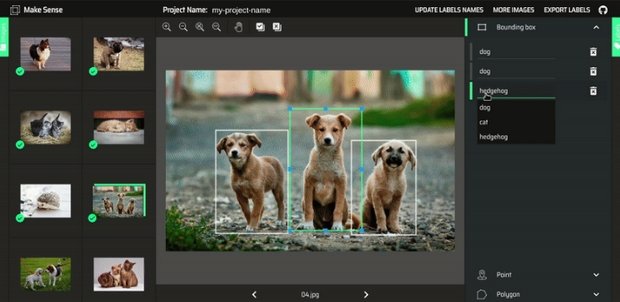
CVAT – Computer Vision Annotation Tool
Developed by Intel researchers, CVAT is a popular open-source tool for image annotation. GitHub Stars: 5.7k
- This annotation tool requires some manual installation as it is based on GitHub.
- Once it is set up, it provides more tools and features than others, for example, shortcuts and a label shape creator.
- CVAT supports add-ons like TensorFlow Object Detection and Deep Learning Deployment Toolkit.
- The computer vision application platform Viso Suite includes CVAT for businesses.
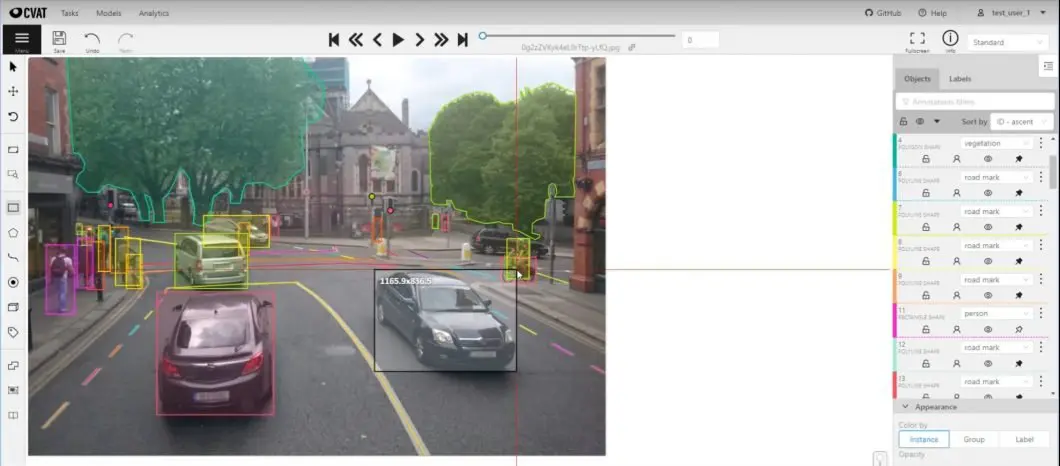
LabelImg
Written in Python, LabelImg is a popular barebones graphical image annotation tool. GitHub Stars: 14.7k
- The installation is relatively simple and is generally done through a command prompt/terminal.
- The image annotation tool is great for datasets under 10,000 images, as it requires a lot of manual interaction and is made to help annotate datasets for object detection models.
- The simple interface makes it easy to use which makes it a good tool for beginner ML programmers with many well-documented tutorials out there.
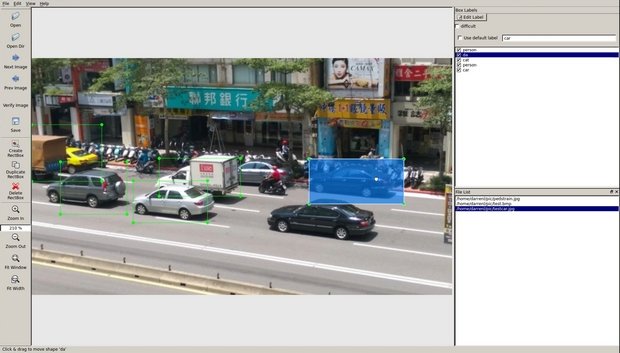
Business Image Annotation Solution
The computer vision platform Viso Suite includes a built-in image annotation environment based on CVAT. The entire Suite is cloud-native and accessible via any browser. Viso Suite provides an integrated image and video annotation solution for professional teams.
Users can collaboratively and seamlessly collect video data, annotate images, train and manage AI models, develop applications without coding, and operate large-scale computer vision systems.
Viso accelerates the entire application lifecycle end-to-end with automated features to automate and accelerate tedious integration tasks.
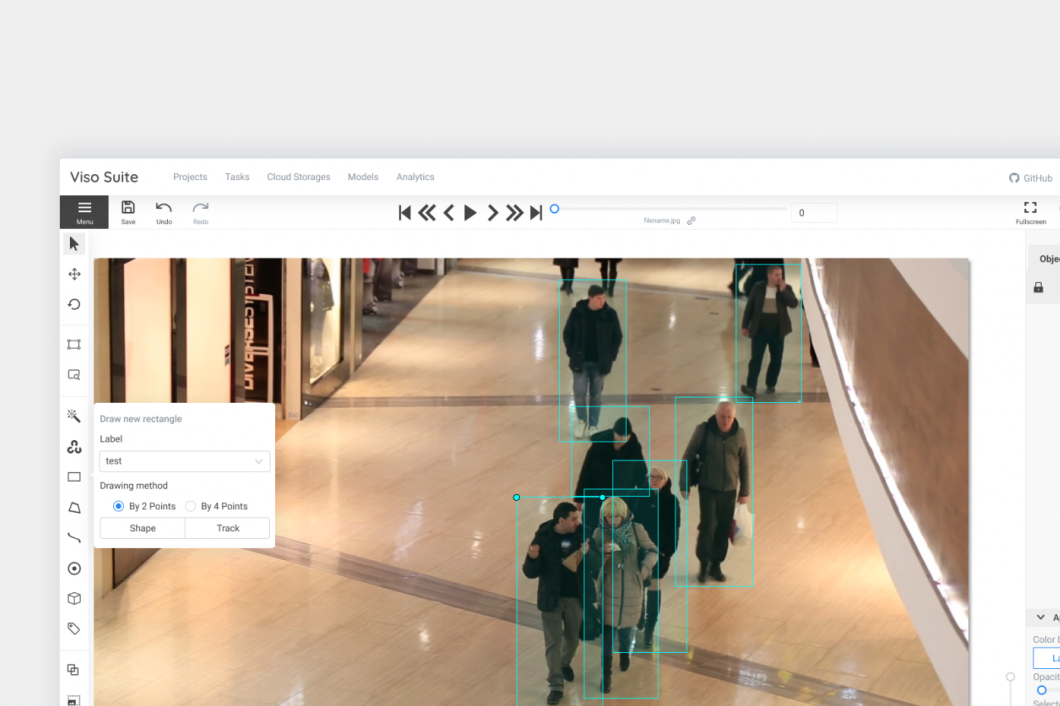
How Long Does Image Annotation Take?
The time needed to annotate images greatly depends on the complexity of the images, the number of objects, the complexity of the annotations (polygon vs. boxes), and the required accuracy and level of detail.
Usually, even image annotation companies have a hard time telling how long image annotation takes before some samples have to be labeled to make an estimation based on the results. But even then, there is no guarantee that the annotation quality and consistency allow precise estimations. While automated image annotation and semi-automated tools help to speed up the process, there is still a human element required to ensure a consistent quality level (hence “supervised”).
In general, simple objects with fewer control points (window, door, sign, lamp) require far less time to annotate compared to region-based objects with more control points (fork, wineglass, sky). Tools with semi-automatic image annotation and preliminary annotation creation with a deep learning model help to speed up both the annotation quality and speed.
Read our article about CVAT, a tool that provides semi-automatic image annotation features.
Types of Image Annotation
Image annotation is frequently used for image recognition, pose estimation, keypoint detection, image classification, object detection, object recognition, image segmentation, machine learning, and computer vision models. It is the technique used to create reliable datasets for the models to train on and thus is useful for supervised and semi-supervised machine learning models.
For more information on the distinction between supervised and unsupervised machine learning models, we recommend Introduction to Semi-Supervised Machine Learning Models and Self-Supervised Learning: What It Is, Examples and Methods for Computer Vision. In those articles, we discuss their differences and why some models require annotated datasets while others don’t.
The purposes of image annotation (image classification, object detection, etc.) require different techniques of image annotation to develop effective data sets.
1. Image Classification
Image classification is a type of machine learning model that requires images to have a single label to identify the entire image. The image annotation process for image classification models aims at recognizing the presence of similar objects in images of the dataset.
It is used to train an AI model to identify an object in an unlabeled image that looks similar to classes in annotated images that were used to train the model. Training images for image classification is also referred to as tagging. Thus, image classification aims to simply identify the presence of a particular object and name its predefined class.
An example of an image classification model is where different animals are “detected” within input images. In this example, the annotator would be provided with a set of images of different animals and asked to classify each image with a label based on the specific animal species. The animal species, in this case, would be the class, and the image is the input.
Providing the annotated images as data to a computer vision model trains the model for the unique visual characteristics of each type of animal. Thereby, the model would be able to classify new unannotated animal images into the relevant species.
2. Object Detection and Object Recognition
Object detection or recognition models take image classification one step further to find the presence, location, and number of objects in an image. For this type of model, the image annotation process required boundaries to be drawn around every detected object in each image, allowing us to locate the exact position and number of objects present in an image. Therefore, the main difference is that classes are detected within an image rather than the entire image being classified as one class (Image Classification).
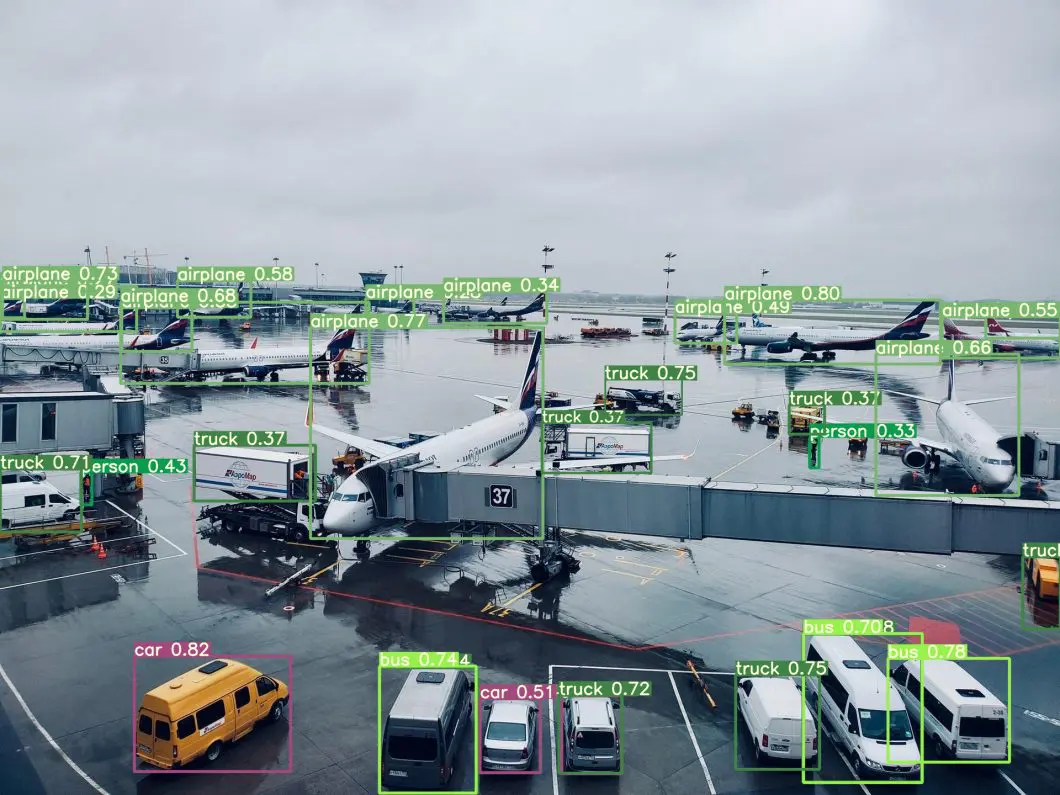
The class location is a parameter in addition to the class, whereas, in image classification, the class location within the image is irrelevant because the entire image is identified as one class. Objects can be annotated within an image using labels such as bounding boxes or polygons.
One of the most common examples of object detection is people detection. It requires the computing device to continuously analyze frames to identify specific object features and recognize present objects as persons. Object detection can also be used to detect any anomaly by tracking the change in the features over a certain period.
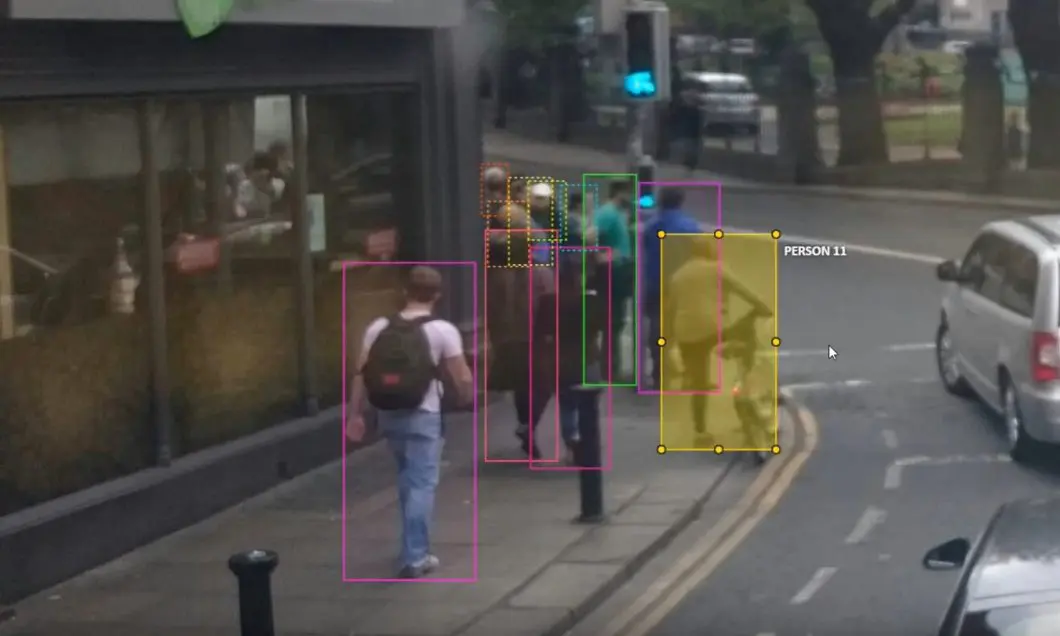
3. Image Segmentation
Image segmentation is a type of image annotation that involves partitioning an image into multiple segments. It is used to locate objects and boundaries (lines, curves, etc.) in images. This is performed at the pixel level, allocating each pixel within an image to a specific object or class. It is used for projects requiring higher accuracy in classifying inputs.
Image segmentation is further divided into the following three classes: semantic, instance, and panoptic.
- Semantic segmentation depicts boundaries between similar objects. This method is used when great precision regarding the presence, location, and size or shape of the objects within an image is needed.
- Instance segmentation identifies the presence, location, number, and size or shape of the objects within an image. Therefore, instance segmentation helps to label every single object’s presence within an image.
- Panoptic segmentation combines both semantic and instance segmentation. Accordingly, panoptic segmentation provides data labeled for background (semantic) and object (instance) within an image.
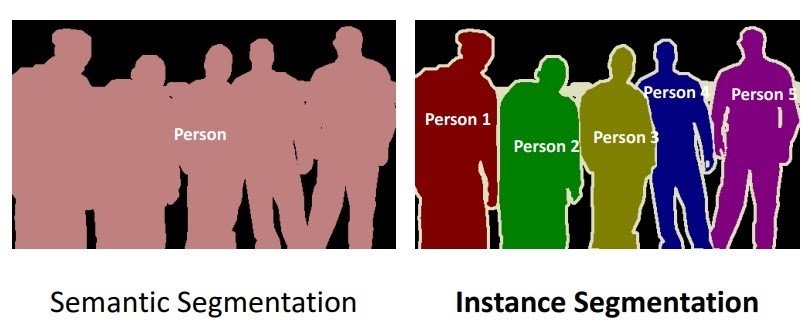
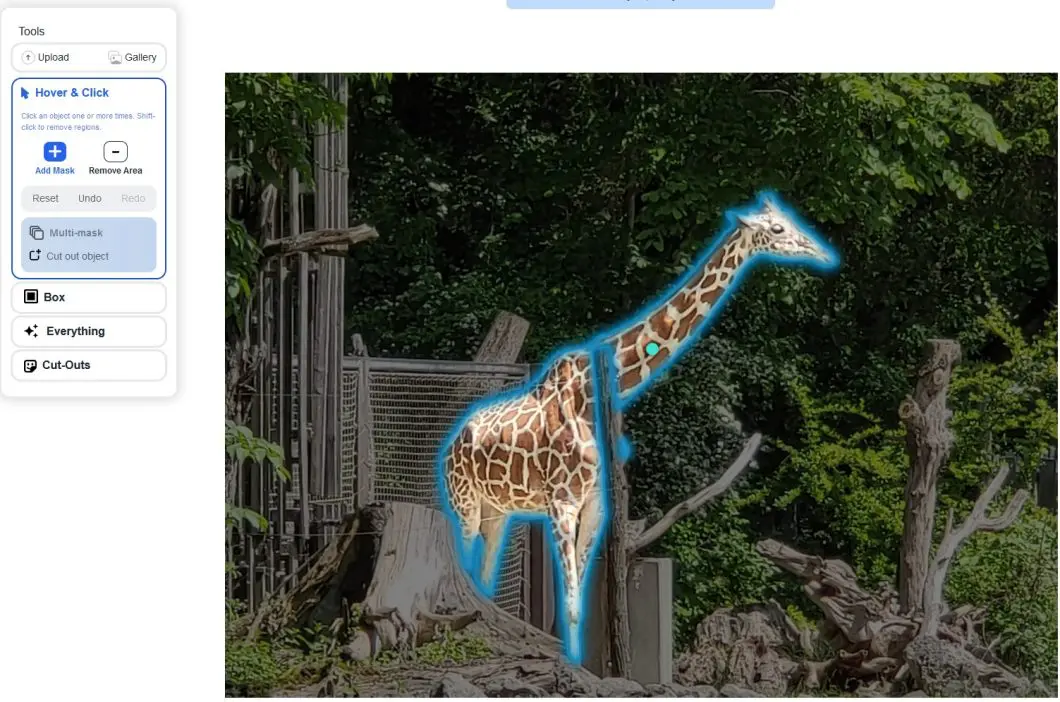
4. Boundary Recognition
This type of image annotation identifies lines or boundaries of objects within an image. Boundaries may include the edges of a particular object or regions of topography present in the image.
Once an image is properly annotated, it can be used to identify similar patterns in unannotated images. Boundary recognition plays a significant role in the safe operation of autonomous driving cars.
Image Annotation Shapes
Different types of annotations are used to annotate an image based on the selected technique. In addition to shapes, annotation techniques like lines, splines, and landmarking can also be used for image annotation.
The following are popular image annotation techniques that are used based on the use case.
1. Bounding Boxes
The bounding box is the most commonly used annotation shape in computer vision. Bounding boxes are rectangular boxes used to define the location of the object within an image. They can be either two-dimensional (2D) or three-dimensional (3D).
2. Polygons
Polygons are used to annotate irregular objects within an image. These are used to mark each of the vertices of the intended object and annotate its edges.
3. Landmarking
This is used to identify fundamental points of interest within an image. Such points are referred to as landmarks or key points. Landmarking is significant in face recognition.
4. Lines and Splines
Lines and splines annotate the image with straight or curved lines. This is significant for boundary recognition to annotate sidewalks, road marks, and other boundary indicators.
Get Started with Image Annotation
Image annotation is the task of annotating an image with data labels. The annotation task usually involves manual work with some computer assistance. Image annotation AI software such as the popular Computer Vision Annotation Tool CVAT helps to provide information about an image that can be used to train computer vision models.
If you need a professional image annotation solution that provides enterprise capabilities and automated infrastructure, have a look at Viso Suite. The end-to-end computer vision platform covers not only image annotation but also the related upstream and downstream tasks. Those cover data collection, model management, application development, DevOps, and Edge AI capabilities. Get a demo for your organization.
If you want to learn more about Computer Vision, I recommend reading the following articles:
- What is Computer Vision? Everything you need to know
- Explore the most popular Computer Vision tools
- Read the full guide about Video Analytics
- A Beginner Guide to Generative Adversarial Networks (GANs)
- Text Annotation: The Complete Guide
- LabelImg for Image Annotation