Object detection, a fundamental task in computer vision, focuses on recognizing and locating various objects within visual data, enabling machines to interpret and understand their surroundings. While there are a handful of different object detection algorithms, we’ll examine YOLOv3 (You Only Look Once).
YOLOv3’s ability to provide accurate and rapid object detection has positioned it as a prominent algorithm in computer vision applications. Particularly, in scenarios where real-time processing is crucial, such as in autonomous vehicles, surveillance systems, and robotics.
What is YOLOv3?
YOLOv3 (You Only Look Once, Version 3) is a real-time object detection algorithm that identifies specific objects in videos, live feeds, or images. The YOLO machine learning algorithm uses features learned by a Deep Convolutional Neural Network to detect objects located in an image. Joseph Redmon and Ali Farhadi are the creators of YOLO versions 1-3, with the third version of the YOLO Machine Learning (ML) algorithm as the most accurate version of the original ML algorithm.
Joseph Redmon and Ali Farhadi created the first version of YOLO algorithms in 2016. The two later released Version 3 two years later, in 2018. YOLOv3 is an improved version of YOLO and YOLOv2. YOLO is implemented using the Keras or OpenCV deep learning libraries.
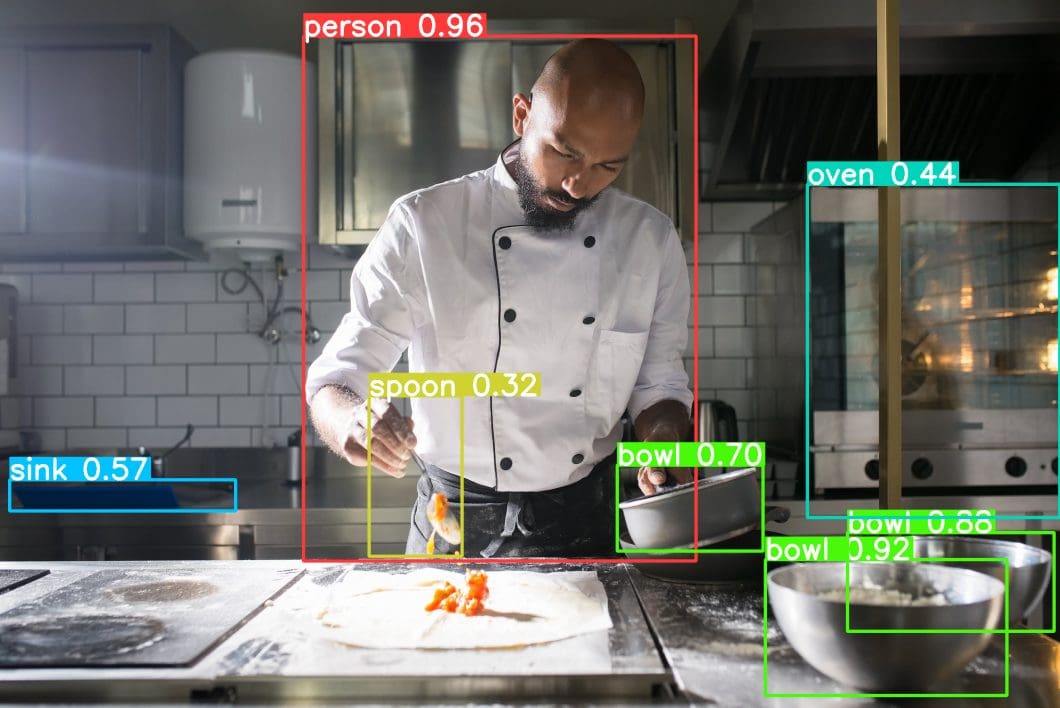
Object classification systems, utilized by Artificial Intelligence (AI) programs, aim to perceive specific objects in a class as subjects of interest. These systems sort objects in images into groups that place objects with similar characteristics together while neglecting others unless programmed to do otherwise. The resulting groups help identify and categorize objects based on their features, contributing to a more nuanced understanding of the predicted class to which each object belongs.
Why the Name “You Only Look Once”?
As is typical for object detectors, the features learned by the convolutional layers are passed onto a classifier, which makes the detection prediction. In YOLO, the prediction is based on a convolutional layer that uses 1×1 convolutions.
YOLO stands for “you only look once” because its prediction uses 1×1 convolutions. This means that the size of the prediction map is exactly the size of the feature map before it. This efficient use of 1×1 convolutions contributes to streamlining the prediction process, allowing the fully connected layer to leverage the compact representation of features before making detection predictions.
How Does YOLOv3 Work?
YOLO is a Convolutional Neural Network (CNN), a type of deep neural network, for performing object detection in real-time. CNNs are classifier-based systems that process input images as structured arrays of data and recognize patterns between them. YOLO has the advantage of being much faster than other networks and still maintains accuracy.
It allows the object detection model to look at the whole image at test time. This means that the global context in the image informs the predictions. YOLO and other CNN algorithms “score” regions based on their similarities to predefined classes.
High-scoring regions are noted as positive detections of whatever class they most closely identify with. For example, in self-driving car footage, YOLO can be used to detect different kinds of vehicles depending on which regions of the video score highly in comparison to pre-defined classes of vehicles. This scoring mechanism, involving regional proposals, enables precise and efficient object detection across various scenes.
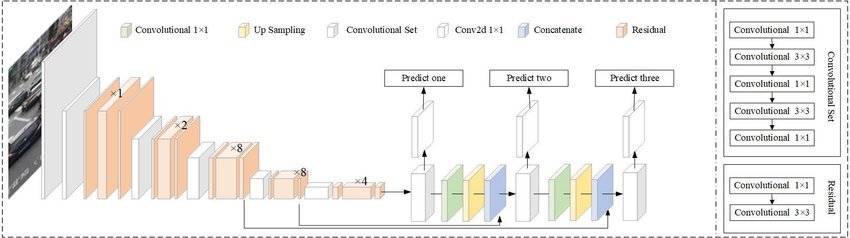
The YOLOv3 Architecture at a Glance
The YOLOv3 algorithm first separates an image into a grid. Each grid cell predicts some number of bounding boxes (sometimes referred to as anchor boxes) around objects that score highly with the aforementioned predefined classes.
Each bounding box has a respective confidence score of how accurate it assumes that prediction should be. Only one object is identified per bounding box. The bounding boxes are generated by clustering the dimensions of the ground truth boxes from the original dataset to find the most common shapes and sizes.
Other comparable algorithms that can carry out the same objective are R-CNN (Region-based Convolutional Neural Networks made in 2015), Fast R-CNN (R-CNN improvement developed in 2017), and Mask R-CNN. However, unlike systems like R-CNN and Fast R-CNN, YOLO can perform classification and bounding box regression at the same time. Thus, ensuring efficient and accurate predictions of the predicted bounding boxes.
Update: Check out our article about the new YOLOv7 model. YOLOv7 is widely expected to become the new industry standard for object detection.
What’s New in YOLO v3?
There are major differences between YOLOv3 and older versions that occur in terms of speed, precision, and specificity of classes. YOLOv2 and YOLOv3 are worlds apart regarding accuracy, speed, and network architecture. YOLOv2 came out in 2016, two years before YOLO v3.
The following sections will give you an overview of what’s new in YOLOv3.
Speed
YOLOv2 was using Darknet-19 as its backbone feature extractor, while YOLOv3 now uses Darknet-53. YOLO creators Joseph Redmon and Ali Farhadi are also the creators of the Darknet-53 backbone.
Darknet-53 has 53 convolutional layers instead of the previous 19. This makes it more powerful than Darknet-19 and more efficient than competing backbones (ResNet-101 or ResNet-152).
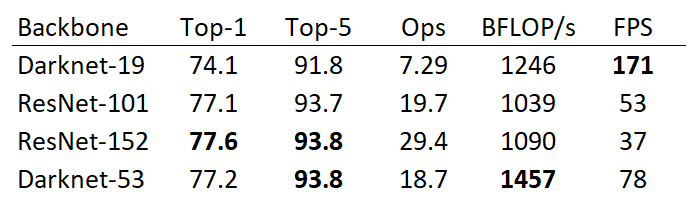
Using the chart in Redmon and Farhadi’s YOLOv3 paper, we can see that Darknet-52 is 1.5 times faster than ResNet101. The depicted accuracy doesn’t entail any trade-off between accuracy and speed between Darknet backbones either. This is because it is still as accurate as ResNet-152, yet two times faster.
YOLOv3 is fast and accurate in terms of mean average precision (mAP) and intersection over union (IOU) values. It runs significantly faster than other detection methods with comparable performance (hence the name, You Only Look Once).
Moreover, you can easily trade off between speed and accuracy simply by changing the model’s size, without the need for model retraining. Thus, showcasing the versatility of feature extraction within the YOLOv3 architecture.
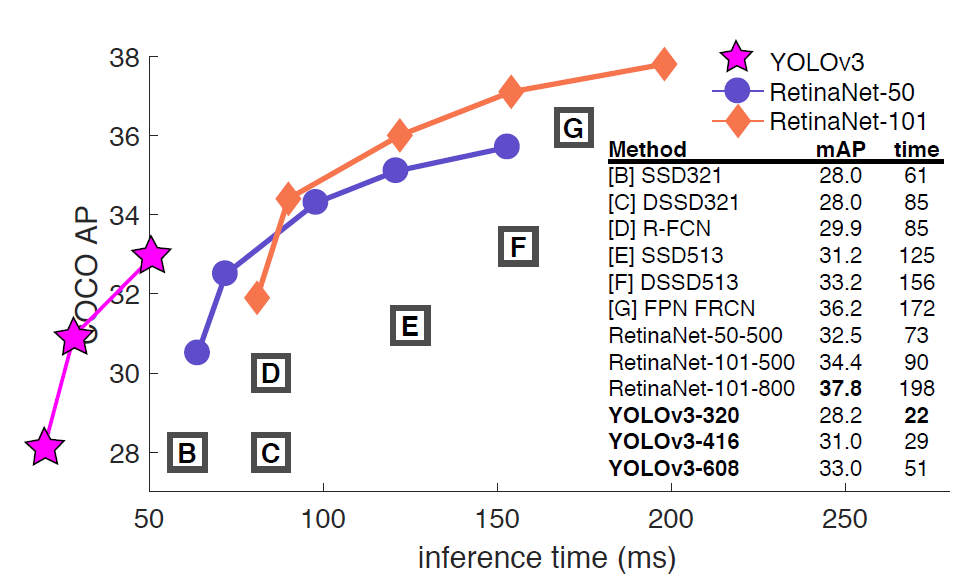
Precision for Small Objects
The chart below (taken and modified from the YOLOv3 paper) shows the average precision (AP) of detecting small, medium, and large images with various algorithms and backbones. The higher the AP, the more accurate it is for that variable.
The precision for small objects in YOLOv2 was incomparable to other algorithms. This was because of how inaccurate YOLO was at detecting small objects. With an AP of 5.0, it paled compared to other algorithms like RetinaNet (21.8) or SSD513 (10.2), which had the second-lowest AP for small objects.
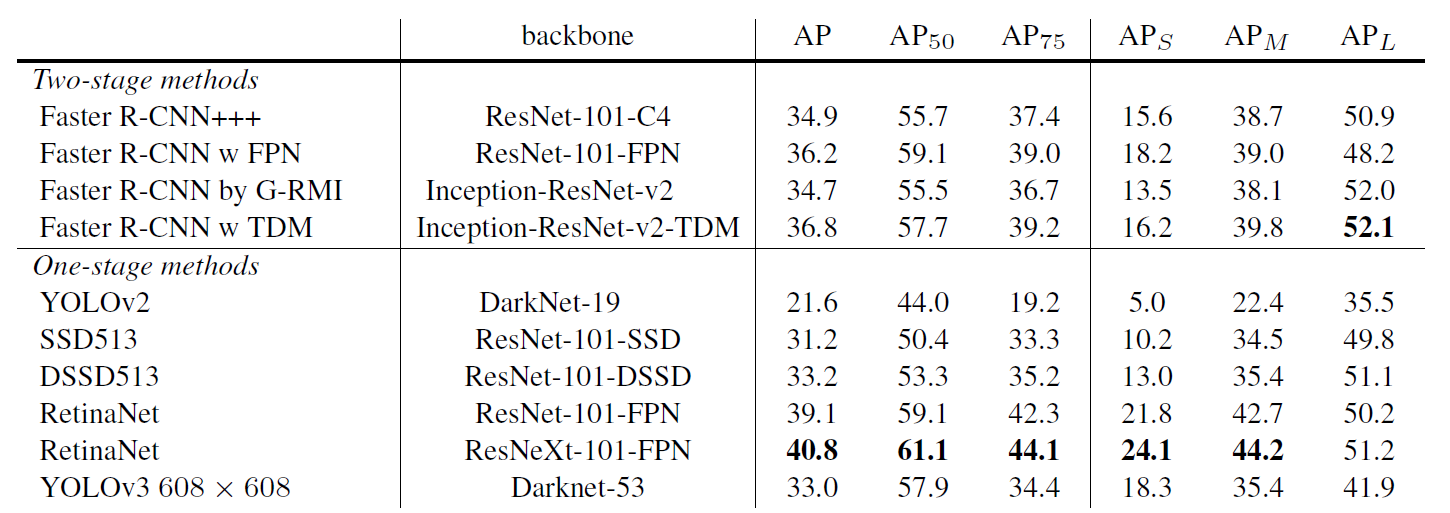
YOLOv3 increased the AP for small objects by 13.3, which is a massive advance from YOLOv2. However, the average precision (AP) for all objects (small, medium, large) is still less than RetinaNet.
Specificity of Classes
The new YOLOv3 uses independent logistic classifiers and binary cross-entropy loss for the class predictions during training. These edits make it possible to use complex datasets such as Microsoft’s Open Images Dataset (OID) for YOLOv3 model training. OID contains dozens of overlapping labels, such as “man” and “person” for images in the dataset.
YOLO v3 uses a multilabel approach which allows classes to be more specific and be multiple for individual bounding boxes. Meanwhile, YOLOv2 used a softmax, which is a mathematical function that converts a vector of numbers into a vector of probabilities, where the probabilities of each value are proportional to the relative scale of each value in the vector.
Using a softmax makes it so that each bounding box can only belong to one class, which is sometimes not the case, especially with datasets like OID.
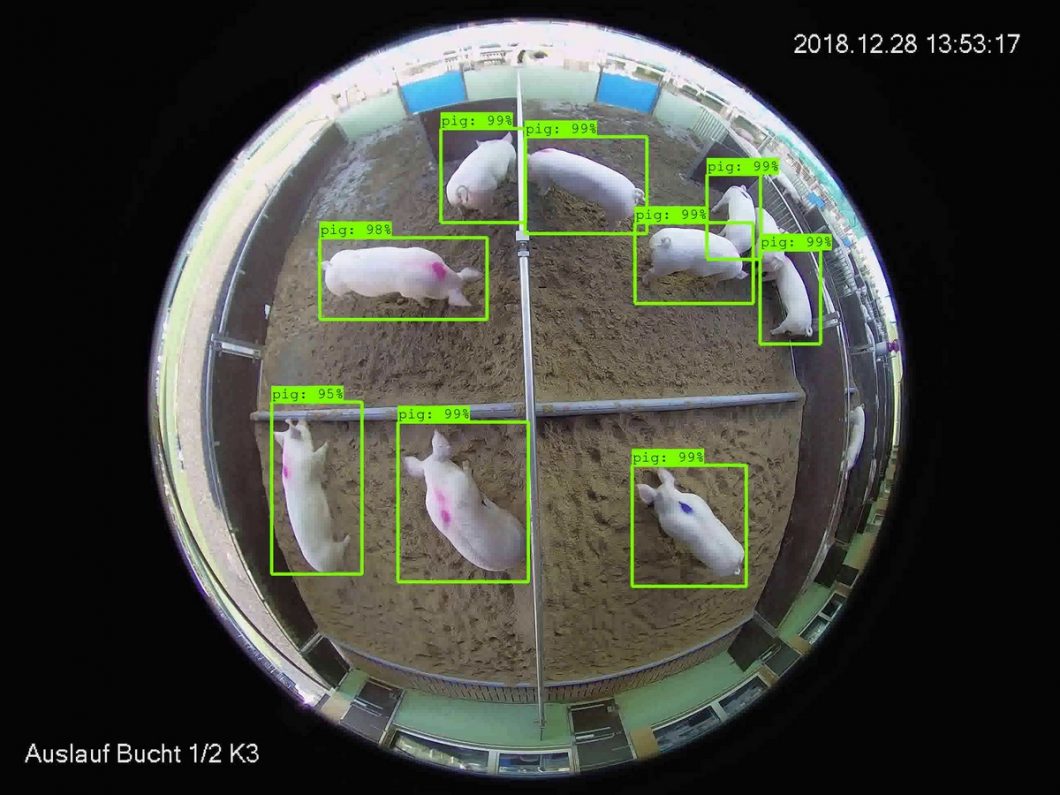
Disadvantages of YOLO v3 vs. Other Algorithms
The YOLOv3 AP does indicate a trade-off between speed and accuracy for using YOLO when compared to RetinaNet since the training time is greater than YOLOv3. However, the accuracy of detecting objects with YOLOv3 can become equal to the accuracy when using RetinaNet by having a larger dataset. Thus, an ideal option for models trained with large datasets.
For example, in common object detection models like traffic detection, there is plenty of data available for model training. This is because there is a large number of organic data and vehicle images readily available. However, YOLOv3 may not be ideal for using niche models where large datasets can be hard to obtain.
Installing YOLOv3
The YOLOv3 installation, including pre-trained models, is relatively straightforward. First, it is necessary to install some dependencies and libraries. YOLOv3 can be installed either directly onto a computer or through a notebook (such as Google Colaboratory or Jupyter). For both implementations, the commands remain the same. Assuming all libraries are installed, the command for installing YOLOv3 is pip install YOLOv3.
We will briefly guide you through installing YOLOv3 with the required libraries.
- Before installing anything, we advise that you make sure the pip version is at least 3.0. You can check the version with the command pip -V.
If for any reason, you are unable to uninstall older versions of pip or can’t directly use pip version 3, you can use the command “pip3 install ___” rather than just “pip.” - Next, we install the required libraries one by one. Starting with OpenCV (Version 3.4 or more recent): pip install opencv-python
- Python (Version 3.6 or more recent): Check if you already have Python: python– version
- Install Python for the first time on Mac or Linux: brew install python (will need Homebrew first if you don’t already have it: /bin/bash -c “$(curl -fsSL https://raw.githubusercontent.com/Homebrew/install/HEAD/install.sh)”)
Install Python for the first time on Windows: use this guide. You will need admin privileges on your computer. - Tensorflow-gpu (Version 1.5.0 or later): pip install TensorFlow
- Keras 2.1.3: pip install keras. Once you’ve downloaded all the above libraries, you can install YOLOv3 with the command pip install YOLOv3.
How to Use YOLOv3
The first step to using YOLOv3 would be to decide on a specific object detection project. YOLOv3 performs real-time detections. Thus, YOLOv3 is ideal for beginners choosing a simple project with an easy premise.
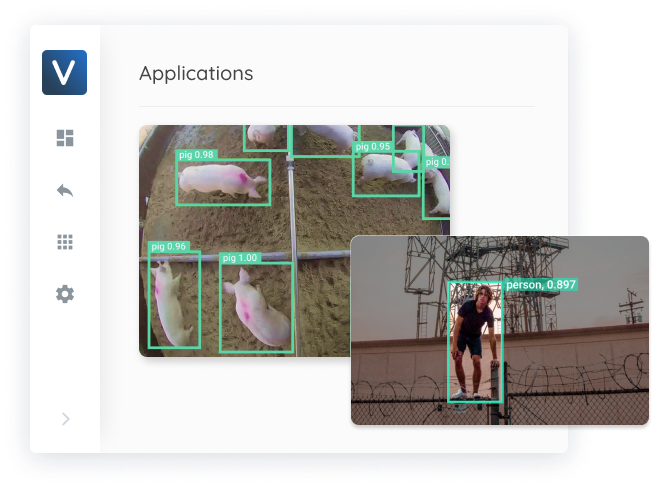
Model Weights
Weights and cfg (or configuration) files are downloadable from the website of the original creator of YOLOv3. Download the model weights and place them into your current directory with the filename “yolov3.weights.”. You can also (more easily) use YOLO’s COCO pre-trained weights by initializing the model with model = YOLOv3().
Using COCO’s pre-trained weights means that you can use YOLO for object detection with the 80 pre-trained classes that come with the COCO dataset. This is a good option for beginners because it requires the least amount of new code and customization.
The following 80 classes are available using COCO’s pre-trained weights:
'person', 'bicycle', 'car', 'motorcycle', 'airplane', 'bus', 'train', 'truck', 'boat', 'traffic light', 'fire hydrant', 'stop sign', 'parking meter', 'bench', 'bird', 'cat', 'dog', 'horse', 'sheep', 'cow', 'elephant', 'bear', 'zebra', 'giraffe', 'backpack', 'umbrella', 'handbag', 'tie', 'suitcase', 'frisbee', 'skis','snowboard', 'sports ball', 'kite', 'baseball bat', 'baseball glove', 'skateboard', 'surfboard', 'tennis racket', 'bottle', 'wine glass', 'cup', 'fork', 'knife', 'spoon', 'bowl', 'banana', 'apple', 'sandwich', 'orange', 'broccoli', 'carrot', 'hot dog', 'pizza', 'donut', 'cake', 'chair', 'couch', 'potted plant', 'bed', 'dining table', 'toilet', 'tv', 'laptop', 'mouse', 'remote', 'keyboard', 'cell phone', 'microwave', 'oven', 'toaster', 'sink', 'refrigerator', 'book', 'clock', 'vase', 'scissors', 'teddy bear', 'hair drier', 'toothbrush'
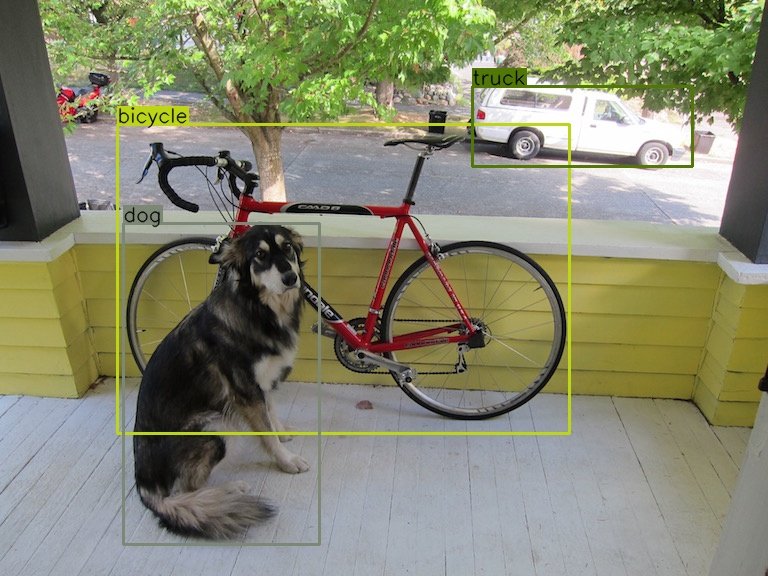
Making a Prediction With YOLO v3
The convolutional layers included in the YOLOv3 architecture produce a detection prediction after passing the features learned onto a classifier or regressor. These features include the class label, coordinates of the bounding boxes, sizes of the bounding boxes, and more.
Since the prediction with the YOLO machine-learning algorithm uses 1 x 1 convolutions (hence the name, “you only look once”), the size of the prediction map is exactly the same size as the feature map before it.
In YOLOv3, each cell interprets the prediction map by predicting a fixed number of bounding boxes. Then, whichever cell contains the center of the ground truth box of an object of interest is designated as the cell that will finally be responsible for predicting the object. There is a ton of mathematics behind the inner workings of the prediction architecture.
- Anchor Boxes
Although we previously touched on bounding boxes and classes in this article, implementation and use with YOLOv3 require more detail. Object detectors using YOLOv3 usually predict log-space transforms, which are offsets to predefined “default” bounding boxes. Those specific bounding boxes are anchors. The transforms are later applied to the anchor boxes to receive a prediction. YOLOv3, in particular, has three anchors. This results in the prediction of three bounding boxes per cell. The cell is also referred to as a neuron in more technical terms. - Non-Maximum Suppression
Objects can sometimes be detected multiple times when more than one bounding box detects the object as a positive class detection. Non-maximum suppression helps avoid this situation and only passes detections if they haven’t already been detected. Using the NMS threshold value and confidence threshold value, we implement NMS to prevent double detections. It is an imperative part of utilizing YOLOv3 effectively. Here, we briefly described some features that make the predictions possible, such as anchor boxes and non-maximum suppression (NMS) values. This is, however, not a complete representation of all the features that go into creating a successful prediction with YOLOv3. For full descriptions of YOLOv3’s mathematical background, I suggest reading the official YOLOv3 paper linked at the end of this article.
Interpreting Results
Interpreting the results of a YOLO model prediction is just as nuanced as the actual implementation of the model. Multiple factors go into a successful interpretation and accuracy rating, such as the box confidence score and class confidence score used when creating a YOLOv3 computer vision model. There are many other ways and features used when interpreting results, but these are just a few. Other YOLOv3 prediction features include classification loss, loss function, objectness score, and more.
Class Confidence and Box Confidence Scores
Each bounding box has an x, y, w, h, and box confidence score value. The confidence score is the value of how probable a class is contained by that box, as well as how accurate that bounding box is.
The bounding box width and height (w and h) are first set to the width and height of the image given. Then, x and y are offsets of the cell in question and all 4 bounding box values are between 0 and 1. Then, each cell has 20 conditional class probabilities implemented by the YOLOv3 algorithm.
The class confidence score for each final bounding box used as a positive prediction is equal to the box confidence score multiplied by the conditional class probability. The conditional class probability in this context is the probability that the detected object is part of a certain class (the class being the object of interest’s identification). YOLOv3’s prediction, therefore, has 3 values of h, w, and depth.
The spatial dimensions of the images and tensors used to produce boundary box predictions involve some high-level math. To learn more about this stage, you may find the YOLOv3 Arxiv paper at the end of this article.
For the final step, the boundary boxes with high confidence scores (more than 0.25) are kept as final predictions.
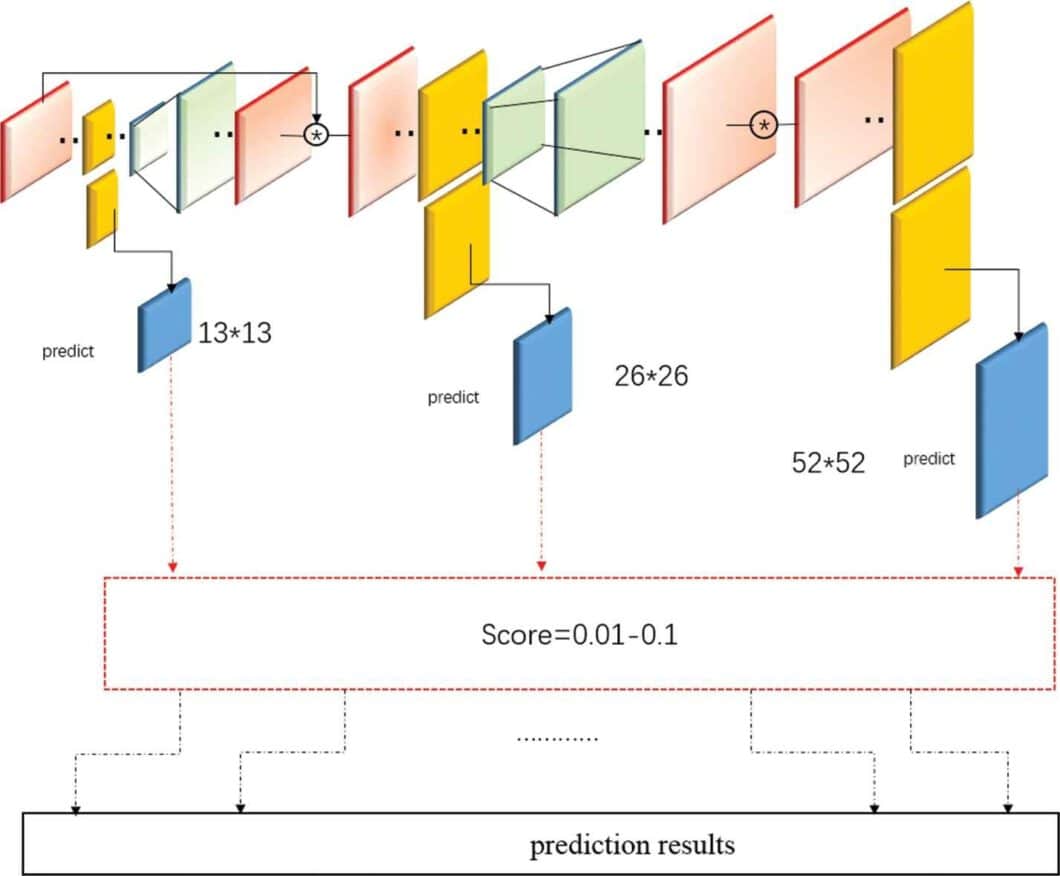
YOLOv3 Resources
The YOLOv3 algorithm has a multitude of credible resources created by the author and makers of the algorithm itself. For any purpose, primary resources are always best for getting accurate information on the topic. However, for YOLO v3, these resources are even more important because of all the second-hand information available on its use.
In researching for this article, the most useful primary resources were:
- YOLOv1, an accredited paper on the first version of the architecture: Redmon, Joseph, Divvala, Girshick. “You Only Look Once: Unified, Real-Time Object Detection.” (2015) – Find it here.
- YOLOv3, an accredited paper on the third version of YOLO: Redmon, Joseph, and Ali Farhadi. “YOLOv3: An Incremental Improvement.” (2018) – Find it here.
- YOLOv3 source code and algorithm specifics by the original author (Joseph Redmon) – Find it here.
- Results from the Paper for YOLOv3: Paperswithcode, YOLOv3: An Incremental Improvement (uploaded by Redmon and Farhadi) – Find it here.
Why Use YOLOv3?
Since the release of YOLOv3 in April 2018, several other official and unofficial YOLO versions have been released. These include:
- YOLOv4: Alexey Bochkovskiy, Chien-Yao Wang, and Hong-Yuan Mark Liao (April 2020)
- YOLOv5: Ultralytics (May 2020)
- YOLOX: Huawei Noah’s Ark Lab (July 2021)
- YOLOv6: Meituan Technical Team (June 2022)
- YOLOv7: Chien-Yao Wang, Alexey Bochkovskiy, and Hong-Yuan Mark Liao (July 2022)
- YOLOv8: Ultralytics (January 2023)
- YOLOv9: Chien-Yao Wang, I-Hau Yeh, and Hong-Yuan Mark Liao (February 2024)
- YOLOv10: Ao Wang, Hui Chen, Lihao Liu, Kai Chen, Zijia Lin, Jungong Han, Guiguang Ding (May 2024)
- YOLO11: Ultralytics (September 2024)
- YOLOv12: Yunjie Tian, Qixiang Ye, David Doermann (February 2025)
Users may choose to stick with YOLOv3 over newer iterations like YOLOv4 or YOLOv5 due to project requirements. One significant consideration is stability and maturity. YOLOv3 has been in circulation for a more extended period, undergoing extensive testing and validation across diverse applications. This accumulated experience contributes to a perception of stability and reliability, particularly in situations where the latest features of newer versions are not critical.
Additionally, the choice may hinge on practical considerations such as model size and deployment. YOLOv3 is a comparatively lightweight model, making it a suitable option for deployment on edge devices with limited storage capacity or bandwidth constraints. Users who prioritize model compactness and ease of deployment may find YOLOv3 preferable over later versions that might demand more storage and computational resources.
Comparing YOLOv3 and YOLOv5
YOLOv5 was published by the company Ultralytics and is therefore not part of the official YOLO series. This has sparked some controversy in the computer vision community. The architecture is similar to the official YOLOv4 but is based on a different Framework, PyTorch instead of Darknet.
According to the creator of the official YOLOv4, the performance of v5 is similar to the official v4.
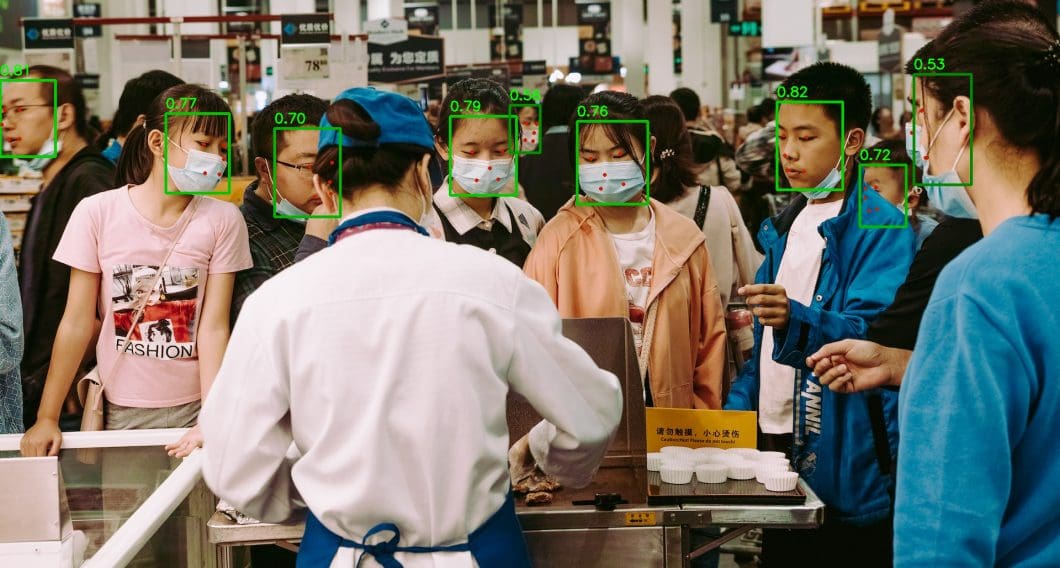
Comparing YOLOv3 and YOLOR
YOLO is just one of many algorithms used extensively in artificial intelligence. We’ve discussed the new version of YOLO, YOLOv5, and its surrounding controversy regarding the new architecture and validity. Check out our analysis to learn more about the history of YOLO and why the original author of YOLO did not make the new versions 4 and 5.
YOLOR (You Only Learn One Representation) is a different, high-performing object detection algorithm. It provides significant performance gains over YOLOv3 and performs very well on the COCO benchmark. YOLOR had been considered state-of-the-art before the release of YOLOv7 in July 2022.
Comparing YOLOv3 and YOLOv7
At its release in 2022, YOLOv7 boasted that it surpassed all known real-time object detectors in terms of accuracy and speed. Check out our article about YOLOv7 and learn how to implement it for business solutions.
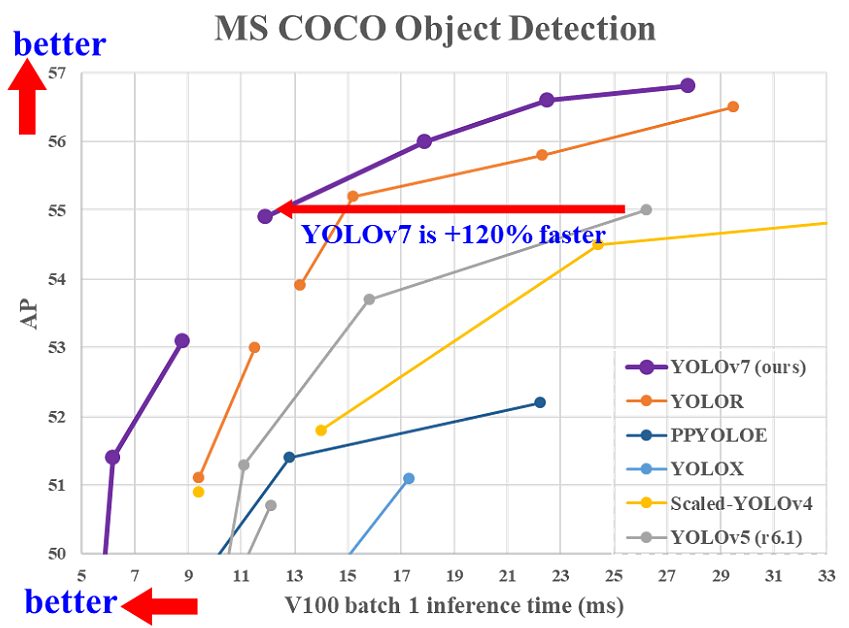
Comparing YOLOv3 and YOLOv8
Ultralytics has referred to its YOLOv8 model as state-of-the-art since its January 2023 release. Newer versions of YOLOv8 are more lightweight and prove to outperform older YOLO versions on the COCO dataset. However, the community considers YOLOv8 an “unofficial” version. Additionally, Ultralytics licenses YOLOv8 under the stringent AGP-3.0 license, which requires organizations to pay for commercial use. At this time, many organizations choose to instead use YOLOv3 for real-time object detection tasks.
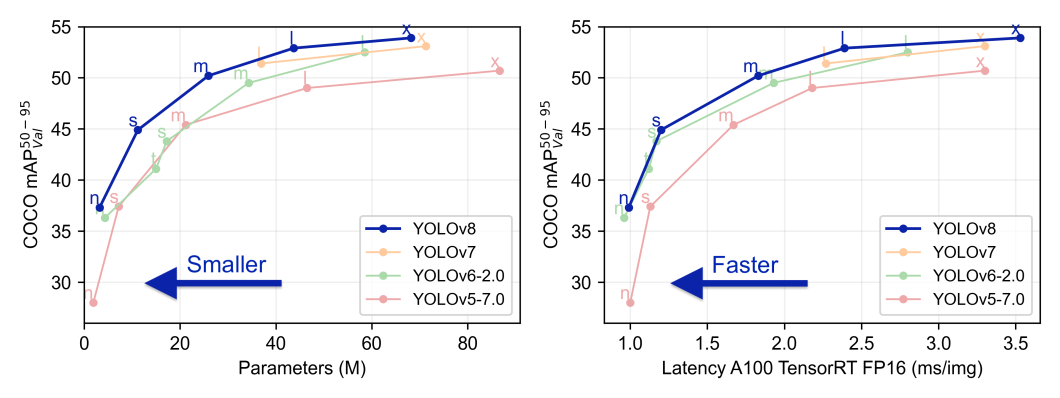
What’s Next for YOLOv3?
While developers release faster and more efficient models every day, YOLOv3 remains a popular and reliable object detection model.
If you are looking for a business solution to implement a custom computer vision application based on YOLOv3 or other AI models, check out the next-gen computer vision platform Viso Suite. The end-to-end solution covers the entire computer vision lifecycle, with image annotation, AI model training, and AI model management for YOLO v3 and all other popular deep learning models, including YOLO v7 and YOLOv8.
Viso Suite provides a powerful enterprise solution to power private on-device AI vision. It leverages Edge AI to avoid storing or sending video data to the cloud. The Suite provides the most comprehensive features and state-of-the-art automation.
Get in touch and request a demo with our team.