Data preprocessing describes the steps needed to encode data with the purpose of transforming it into a numerical state that machines can read. Data preprocessing techniques are part of data mining, which creates end products out of raw data which is standardized/normalized, contains no null values, and more.
Data preprocessing is essential for machine and deep learning tasks, and it is the basis of algorithm development, ML model training, and computer vision or image processing systems.
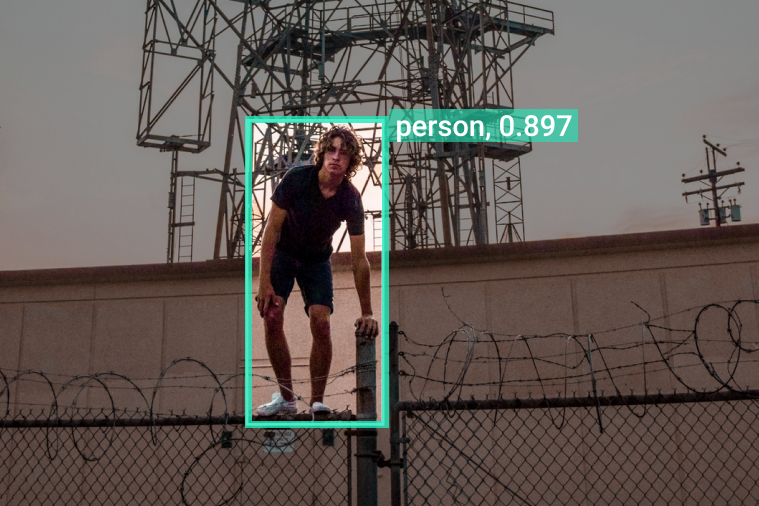
Why Is Data Preprocessing Important?
The massive growth in the scale of data in recent years is the basis of Big Data and IoT systems. Sensors and applications generate vast amounts of raw data. That data cannot be directly treated by humans or manual methods to obtain organized knowledge and useful insights. Big data’s high volume, variety, and velocity require advanced, high-performance processing to easily understand and automatically extract information from data.
The emergence of big data has led to the development of data science or data mining. This popular discipline is rapidly gaining importance in the current world of data. The implementation of emerging technologies and services, like Cloud and Edge computing or machine learning, as well as the reduction in hardware costs, accelerates the adoption rate of intelligent AI applications.
However, it is well-known that low-quality data will lead to low-quality information. Thus, data processing is a major and critical stage to obtain final data sets that can be used for further data analysis or machine learning algorithms. This is why data preprocessing is an integral part of AIoT applications and systems for image recognition with AI vision.
Python Data Preprocessing Techniques
Replacing Null Values
Replacing null values is usually the most common of data preprocessing techniques because it allows us to have a full dataset of values to work with. To execute replacing null values as part of data preprocessing, I suggest using Google Colab or opening a Jupyter notebook. For simplicity’s sake, I will be using Google Colab. Your first step will be to import, SimpleImputer
which is part of the sklearn
library. SimpleImputer
provides basic strategies for imputing, or representing missing values.
from sklearn.impute import SimpleImputer
Next, you’re going to want to specify which missing values to replace. We will be replacing those missing values with the mean of that row of the dataset, which we can do by setting the strategy variable equal to the mean.
imputer = SimpleImputer(missing_values=np.nan, strategy='mean')
The imputer fills missing values with some statistics (e.g., mean, median) of the data. To avoid data leakage during cross-validation, it computes the statistic on the training data during the fit and then stores it. It then uses that data on the test portion, done during the transform.
imputer.fit(X[:, 1:3]) #looks @ rows and columns X[:, 1:3] = imputer.transform(X[:, 1:3])
Feature Scaling
Feature scaling is a data preprocessing technique used to normalize our set of data values. The reason we use feature scaling is that some sets of data might be overtaken by others in such a way that the machine learning model disregards the overtaken data. The sets of data, in this case, represent separate features.
The next bit of code we’ll be using will scale our data features using a Python function called standardization. The function operates by subtracting each value of the feature by the mean of all the values of that feature, and then dividing that difference by the standard deviation of the feature.
Doing this will allow all the values to be within three numbers, or values, of each other. Standardization is a commonly used data preprocessing technique. Another feature scaling function we could have used is normalization, which works by subtracting each feature value from the minimum and then dividing that by the difference between the maximum and minimum.
Normalization puts all values between 0 and 1. However, normalization is a recommended data preprocessing technique when most of your features exhibit a normal distribution, which may not always be the case. Since standardization would work for both cases, we’ll be using it here.
from sklearn.preprocessing import StandardScaler sc = StandardScaler() #sc = standard scaler variable x_train = sc.fit_transform(x_train)#only apply feature scaling to numerical values x_test = sc.transform(x_test)
Above, we begin by importing the class StandardScaler
from sklearn
preprocessing module. After this, we create an object of the class in the variable sc. Since we’ll be applying it to all our values, we don’t need to pass any parameters.
Then, we take the training set X and fit our standard scalar object only on the columns containing independent values. We have to do the same process for the testing values, which is why we have x_test
.
What’s Next?
Data preprocessing techniques are important to create a final product out of data sets. The above were two common steps or methods of data preprocessing with Python.
For more information about the workings behind machine learning and data analysis, we recommend the following articles: