The charm of AI fades within a short while when budgets get exhausted, deadlines are delayed, or ROI metrics are not met. The good news? Understanding why computer vision is difficult to implement helps to cut through the complexity. Here’s why:
Mission-critical Computer Vision Use Cases Depend on Edge Computing
Artificial Intelligence is present in many areas of our lives, providing visible improvements to the way we discover information, communicate, or move from point A to point B. AI adoption is rapidly increasing not only in consumer areas such as digital assistants and self-driving vehicles but across all industries, disrupting whole business models and creating new opportunities to generate new sources of customer value. Computer vision in Smart City Applications is used for intrusion detection, vehicle counting, crowd analytics, self-harm prevention, compliance control, or remote visual inspection solutions.
Focusing on computer vision, the number of AI applications that perform at the human level or better is increasing exponentially, given the fast-paced advances in Machine Learning.
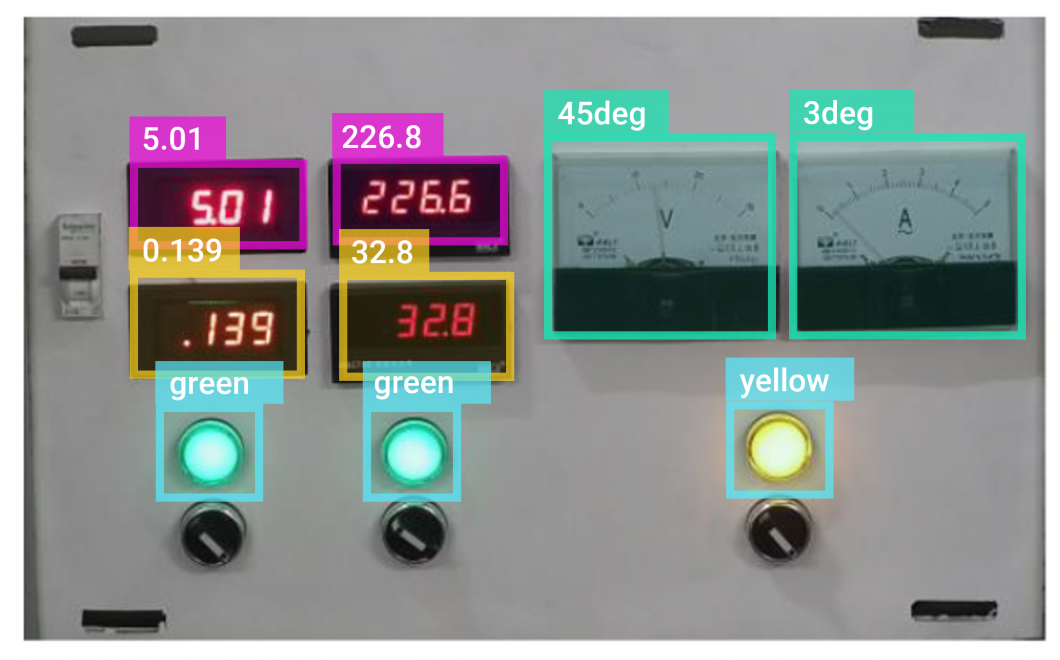
AI vision encompasses techniques used in the image processing industry to solve a wide range of previously intractable problems by using Computer Vision and Deep Learning. However, high innovation potential does not come without challenges.
AI inference requires a considerable amount of processing power, especially for real-time data-intensive applications. Also, AI solutions can be deployed in cloud environments (Amazon AWS, Google GCP, Microsoft Azure) to take advantage of simplified management and scalable computing assets.
However, running computer vision in the cloud is heavily limiting real-time computer vision applications. Collecting video streams in the cloud (data-offloading) means that every image of a video (30 per second in regular cameras) is recorded and transferred to the cloud before processing is possible. While a 24/7 internet connection is requisite, it is expensive. This is because AI computer vision models are extremely computationally intensive, leading to heightened costs.
Cloud Limitations
- What if your solution needs to run in real-time and requires fast response times?
- How can I operate a system that is mission-critical and runs off-grid?
- How can I handle the high operating costs of analyzing massive data in the cloud?
- What about data privacy if sending and storing video material in the cloud?
The solution is a technology called Edge AI that moves machine-learning visual tasks from the cloud to high-performance computers that are connected to cameras and therefore close to the source of data. Edge devices are connected over the cloud and therefore provide a truly scalable way to power Computer Vision in real-world applications effectively.
Therefore, computer vision solutions will need to be deployed on edge endpoints for most use cases. This allows on-device machine learning and processing of the data where it is captured while only the results are sent back to the cloud for further analysis (not the sensitive, data-intensive video feeds).
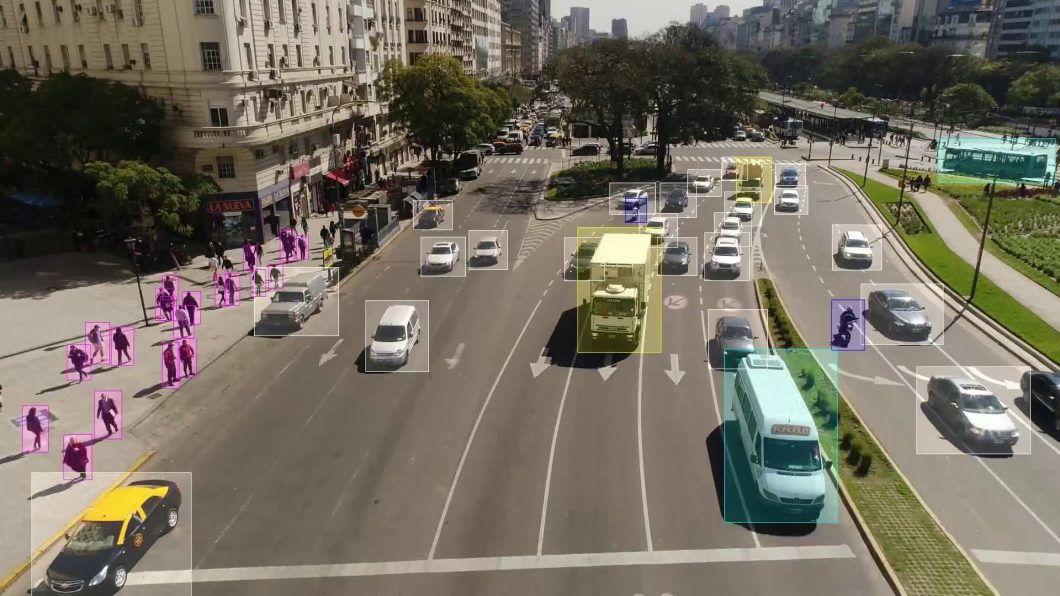
Computer Vision Can Difficult Due to Hardware Limitations
Real-world computer vision use cases require hardware to run, cameras for visual input, and computing hardware for AI inference.
Especially for mission-critical AI vision use cases that depend on near real-time video analytics, deploying AI solutions to edge computing devices (Edge AI) is the only way to overcome the latency limitations of centralized cloud computing (see Edge Intelligence).
A fine example is a farming analytics system that is used for animal monitoring. Such an AI vision system is considered mission-critical because timeouts may severely impact livestock. Also, the data load is immense as the system is meant to capture and perform inference for 30 images/second/camera feed. For an average setup of 100 cameras, we get a volume of 259.2 million images per day. Without edge computing, all this data would need to be sent to the cloud. This can lead to bottleneck problems that drive costs (unexpected cloud cost spikes after timeouts).
The best option for this use case is to run AI inference in real-time at the Edge: Analyze the data where it is being generated! And only communicate key data points to the cloud backend for data aggregation and further analysis.
Hence, the most powerful way to deliver scalable AI vision applications is with the latest Edge AI hardware and accelerators optimized for on-device AI inferencing. Edge or on-device AI is based on analyzing video streams in real time with pre-trained models deployed to edge devices connected to a camera.
Considering the rapid growth of AI inference capabilities in Edge AI hardware platforms (Intel NUC, Intel NCS, Nvidia Jetson, ARM Ethos), transferring the processing requirements from Cloud to Edge becomes a desirable option for a wide range of businesses.
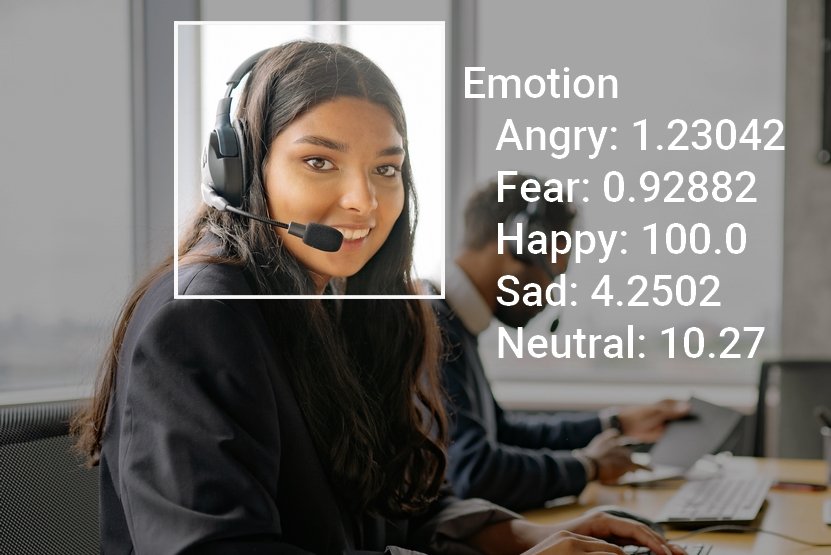
Scaling Computer Vision Systems Can be Complex
Even with the promise of great hardware support for Edge deployments, developing a visual AI solution remains a complex process.
In a traditional approach, several of the following building blocks may be necessary for developing your solution at scale. Those are the seven most important drivers of complexity that make computer vision difficult:
- Collecting input data specific to the problem
- Expertise with popular Deep Learning frameworks like Tensorflow, PyTorch, Keras, Caffe, and MXnet for training data and evaluating Deep Learning models
- Selecting the appropriate hardware (e.g., Intel, NVIDIA, ARM) and software platforms (e.g., Linux, Windows, Docker, Kubernetes), and optimizing Deep Learning models for the deployment environment
- Managing deployments to thousands of distributed Edge devices from the Cloud (Device Cloud)
- Organizing and rolling out updates across the fleet of Edge endpoints that may be offline or experiencing connectivity issues.
- Monitoring metrics from all endpoints and data analysis in real-time. Regular inspection helps to make sure the system is running as intended.
- Knowledge about data privacy and security best practices. Data encryption at rest and in transit and secure access management is an absolute necessity in computer vision.
We associate a high level of development risk with this approach. Especially when considering development time, required domain experts, and difficulties in developing a scalable infrastructure.
5 Ways To Overcome the Complexity of Computer Vision
Viso Suite is an end-to-end cloud platform for Computer Vision applications, focusing on ease-of-use, high performance, and scalability. The viso.ai platform is industry agnostic. It provides Deep Learning and Computer Vision tools to build, deploy, and operate deep learning applications in a low-code environment. This includes everything you need to get to market 10x faster and with minimal risk.
Viso Suite Features
- Visual Programming: Use a visual approach to build complex computer vision and deep learning solutions on the fly. The visual programming approach can reduce development time by over 90%. In addition, it greatly reduces the effort to write code from scratch and gives visibility into how the AI vision application works. Use over 60 of the latest AI models with one click; Viso Suite already integrates and optimizes them for different computing architectures.
- Integrated Device Management: Add and manage thousands of edge devices and AI hardware easily, regardless of device type and architecture (amd64, aarch64, …). Create a device image and flash it to your device to make it appear in your workspace. Check device health metrics and online or deployment statuses without writing a single line of code. Use the latest AI accelerators and chips optimized for computer vision AI inference: Google Coral TPU, Intel Neural Compute Stick 2, Nvidia Jetson, and more.
- Deployment Management: Managing and deploying to remote edge devices that can be offline or experience network disruptions is a big challenge and can break the entire system. Viso Suite, therefore, offers fully integrated deployment management to enroll and manage endpoint devices. Deploy AI applications to numerous edge devices at the click of a button. Save time on doing everything from scratch, so you have more time to build and update your computer vision application. Use scalable, robust, and brick-safe deployment management that works out of the box.
- Agility and Modularity: Benefit from many pre-existing software modules to build your use case. Viso Suite provides the most popular deep learning frameworks for Object Detection, Image Classification, Image Segmentation, and Pose Estimation. Select the suitable model and create your application with thousands of ready-to-use logic modules.
- Extendability and Integration: Add your algorithms and AI models for use and scaling in Computer Vision Applications. Add your code, and integrate with any third-party system using MQTT and APIs.
What’s Next?
- Read an easy-to-understand guide to Edge Intelligence
- How Viso Suite enables businesses to build and power Visual AI Applications
- Find a guide on how to structure Computer Vision projects
- Read about the most powerful AI hardware in 2021