YOLOv8 is the newest model in the YOLO algorithm series – the most well-known family of object detection and classification models in the Computer Vision (CV) field. With the latest version, the YOLO legacy lives on by providing state-of-the-art results for image or video analytics, with an easy-to-implement framework.
In this article, we’ll discuss:
- The evolution of the YOLO algorithms
- Improvements and enhancements in YOLOv8
- Implementation details and tips
- Applications
About us: Viso.ai offers the world’s leading end-to-end Computer Vision Platform Viso Suite. Our solution helps several leading organizations start with computer vision and implement state-of-the-art models quickly and cheaply for various industrial applications. Get a demo.

What is YOLO
You Only Look Once (YOLO) is an object-detection algorithm introduced in 2015 in a research paper by Joseph Redmon, Santosh Divvala, Ross Girshick, and Ali Farhadi. YOLO’s architecture was a significant revolution in the real-time object detection space, surpassing its predecessor – the Region-based Convolutional Neural Network (R-CNN).
YOLO is a single-shot algorithm that directly classifies an object in a single pass by having only one neural network predict bounding boxes and class probabilities using a full image as input.
The family YOLO model is continuously evolving. Several research teams have since released different YOLO versions, with YOLOv8 being the latest iteration. The following section briefly overviews all the historical versions and their improvements.
A Brief History of YOLO
Before discussing YOLO’s evolution, let’s look at some basics of how a typical object detection algorithm works.
The diagram below illustrates the essential mechanics of an object detection model.
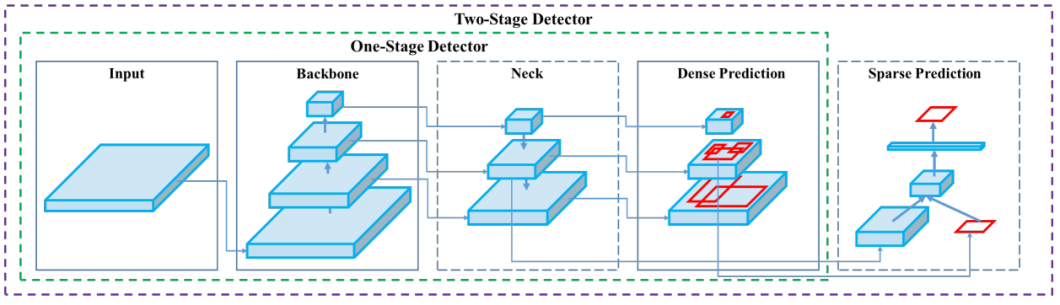
The architecture consists of a backbone, neck, and head. The backbone is a pre-trained Convolutional Neural Network (CNN) that extracts low, medium, and high-level feature maps from an input image. The neck merges these feature maps using path aggregation blocks like the Feature Pyramid Network (FPN). It passes them onto the head, classifying objects and predicting bounding boxes.
The head can consist of one-stage or dense prediction models, such as YOLO or Single-shot Detector (SSD). Alternatively, it can feature two-stage or sparse prediction algorithms like the R-CNN series.
Release | Authors | Tasks | Paper | |
---|---|---|---|---|
YOLO | 2015 | Joseph Redmon, Santosh Divvala, Ross Girshick, and Ali Farhadi | Object Detection, Basic Classification | You Only Look Once: Unified, Real-Time Object Detection |
YOLOv2 | 2016 | Joseph Redmon, Ali Farhadi | Object Detection, Improved Classification | YOLO9000: Better, Faster, Stronger |
YOLOv3 | 2018 | Joseph Redmon, Ali Farhadi | Object Detection, Multi-scale Detection | YOLOv3: An Incremental Improvement |
YOLOv4 | 2020 | Alexey Bochkovskiy, Chien-Yao Wang, Hong-Yuan Mark Liao | Object Detection, Basic Object Tracking | YOLOv4: Optimal Speed and Accuracy of Object Detection |
YOLOv5 | 2020 | Ultralytics | Object Detection, Basic Instance Segmentation (via custom modifications) | no |
YOLOv6 | 2022 | Chuyi Li, et al. | Object Detection, Instance Segmentation | YOLOv6: A Single-Stage Object Detection Framework for Industrial Applications |
YOLOv7 | 2022 | Chien-Yao Wang, Alexey Bochkovskiy, Hong-Yuan Mark Liao | Object Detection, Object Tracking, Instance Segmentation | YOLOv7: Trainable bag-of-freebies sets new state-of-the-art for real-time object detectors |
YOLOv9 | 2024 | Chien-Yao Wang, I-Hau Yeh, Hong-Yuan Mark Liao | Object Detection, Instance Segmentation | YOLOv9: Learning What You Want to Learn Using Programmable Gradient Information |
YOLOv10 | 2024 | Ao Wang, Hui Chen, Lihao Liu, Kai Chen, Zijia Lin, Jungong Han, Guiguang Ding | Object Detection | YOLOv10: Real-Time End-to-End Object Detection |
YOLOv11 | 2024 | Ultralytics | Object Detection, Instance Segmentation, Keypoint Estimation, Oriented Detection, Classification | no |
YOLOv1
As mentioned, YOLO is a single-shot detection model that improved upon the standard R-CNN detection mechanism with faster and better generalization performance.
The real change was how YOLOv1 framed the detection problem as a regression task to predict bounding boxes and class probabilities from a single pass of an image. The diagram below illustrates this point:
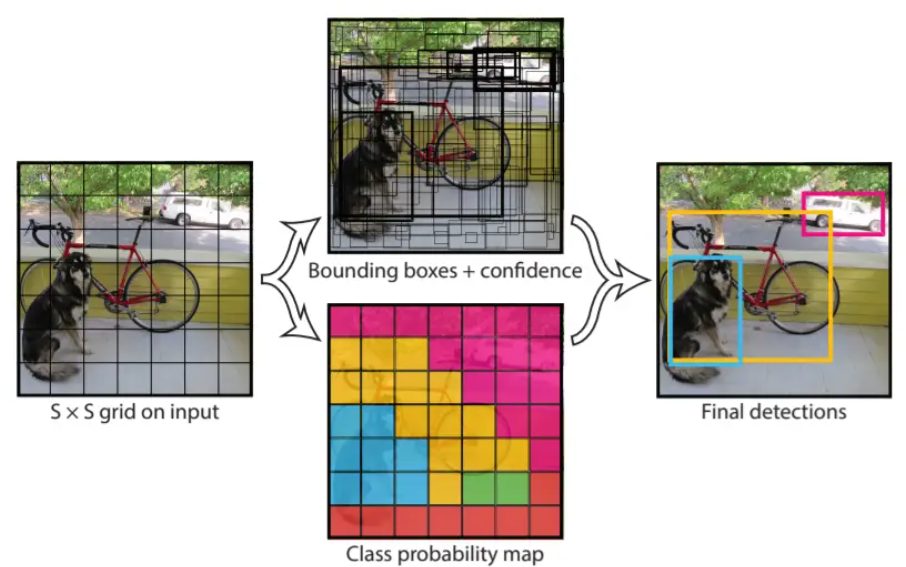
YOLO divides an image into multiple grids and computes confidence scores and bounding boxes for each grid cell that reflect the probability of an object located within a particular grid cell.
Next, given the probability of an object being greater than zero, the algorithm computes respective class probabilities and multiplies them with the object probabilities to generate an overall probability score and bounding box.
With this architecture, YOLOv1 surpassed R-CNN with a mean average precision (mAP) of 63.4 and an inference speed of 45 frames per second (FPS) on the open source Pascal Visual Object Classes 2007 dataset.
YOLOv2
In 2016, Joseph Redmon and Ali Farhadi released YOLOv2, which could detect over 9000 object categories. YOLOv2 introduced anchor boxes – predefined bounding boxes called priors that the model uses to pin down the ideal position of an object.
The algorithm computes the Intersection over Union (IoU) scores for a predicted bounding box against an anchor box. If the IOU reaches a threshold, the model generates a prediction.
YOLOv2 achieved 76.8 mAP at 67 FPS on the VOC 2007 dataset.
YOLOv3
Joseph Redmon and Ali Farhadi published another paper in 2018 to release YOLOv3 that boasted higher accuracy than previous versions, with an mAP of 28.2 at 22 milliseconds.
To predict classes, the YOLOv3 model uses Darknet-53 as the backbone with logistic classifiers instead of softmax and Binary Cross-entropy (BCE) loss.
YOLOv4
In 2020, Alexey Bochkovskiy and other researchers released YOLOv4, which introduced the concept of a Bag of Freebies (BoF) and a Bag of Specials (BoS).
BoF is a group of techniques that increase accuracy at no additional inference cost. In contrast, BoS methods enhance accuracy significantly for a slight increase in inference cost.
BoF included CutMix, CutOut, Mixup data augmentation techniques, and a new Mosaic method. Mosaic augmentation mixes four different training images to provide the model with better context information.
BoS methods have features like non-linear activations and skip connections.
The model achieved 43.5 mAP at approximately 65 FPS on the MS COCO dataset.
YOLOv5
Without an official research paper, Ultralytics released YOLOv5 in June 2020, two months after the launch of YOLOv4. The model is easy to train and use since it is a PyTorch implementation.
The architecture uses a Cross-stage Partial (CSP) Connection block as the backbone for a better gradient flow to reduce computational cost.
Also, YOLOv5 uses the Yet Another Markup Language (YAML) files instead of the CFG file that includes model configurations.
Since YOLOv5 lacks an official research paper, no authentic results exist to compare its performance with previous versions and other object detection models.
YOLOv6
YOLOv6 is another unofficial version of the YOLO series introduced in 2022 by Meituan – a Chinese shopping platform. The company targeted the model for industrial applications with better performance than its predecessor.
The significant differences include anchor-free detection and a decoupled head, which means one head performs classification. In contrast, the other conducts regression to predict bounding box coordinates.
The changes resulted in YOLOv6(nano) achieving an mAP of 37.5 at 1187 FPS on the COCO dataset and YOLOv6(small) achieving 45 mAP at 484 FPS.
YOLOv7
In July 2022, a group of researchers released the open-source model YOLOv7, the fastest and the most accurate object detector with an mAP of 56.8% at FPS ranging from 5 to 160.
Extended Efficient Layer Aggregation Network (E-ELAN) forms the backbone of YOLOv7, which improves training by letting the model learn diverse features with efficient computation.
Also, the model uses compound scaling for concatenation-based models to address the need for different inference speeds.
YOLOv8
We finally come to Ultralytics YOLOv8, released in January 2023. Like v5 and v6, YOLOv8 has no official paper but boasts higher accuracy and faster speed.
For instance, the YOLOv8(medium) has a 50.2 mAP score at 1.83 milliseconds on the COCO dataset and A100 TensorRT.
YOLO v8 also features a Python package and CLI-based implementation, making it easy to use and develop.
Let’s look closely at what the YOLOv8 can do and explore a few of its significant developments.

Since YOLOv8’s release, two different teams of researchers have released YOLOv9 (February 2024) and YOLOv10 (May 2024).
YOLOv8 Tasks
YOLOv8 comes in five variants based on the number of parameters – nano(n), small(s), medium(m), large(l), and extra large(x). You can use all the variants for classification, object detection, and segmentation.
Image Classification
Classification involves categorizing an entire image without localizing the object present within the image.
You can implement classification with YOLOv8 by adding the -cls
suffix to the YOLOv8 version. For example, you can use yolov8n-cls.pt
for classification if you wish to use the nano version.
Object Detection
Object detection localizes an object within an image by drawing bounding boxes. You don’t have to add any suffix to use YOLOv8 for detection.
The implementation only requires you to define the model as yolov8n.pt
for object detection with the nano variant.
Image Segmentation
Image segmentation goes a step further and identifies each pixel belonging to an object. Unlike object detection, segmentation is more precise in locating different objects within a single image.
You can add the -seg
suffix as yolov8n-seg.pt
to implement segmentation with the YOLOv8 nano variant.
YOLOv8 Major Developments
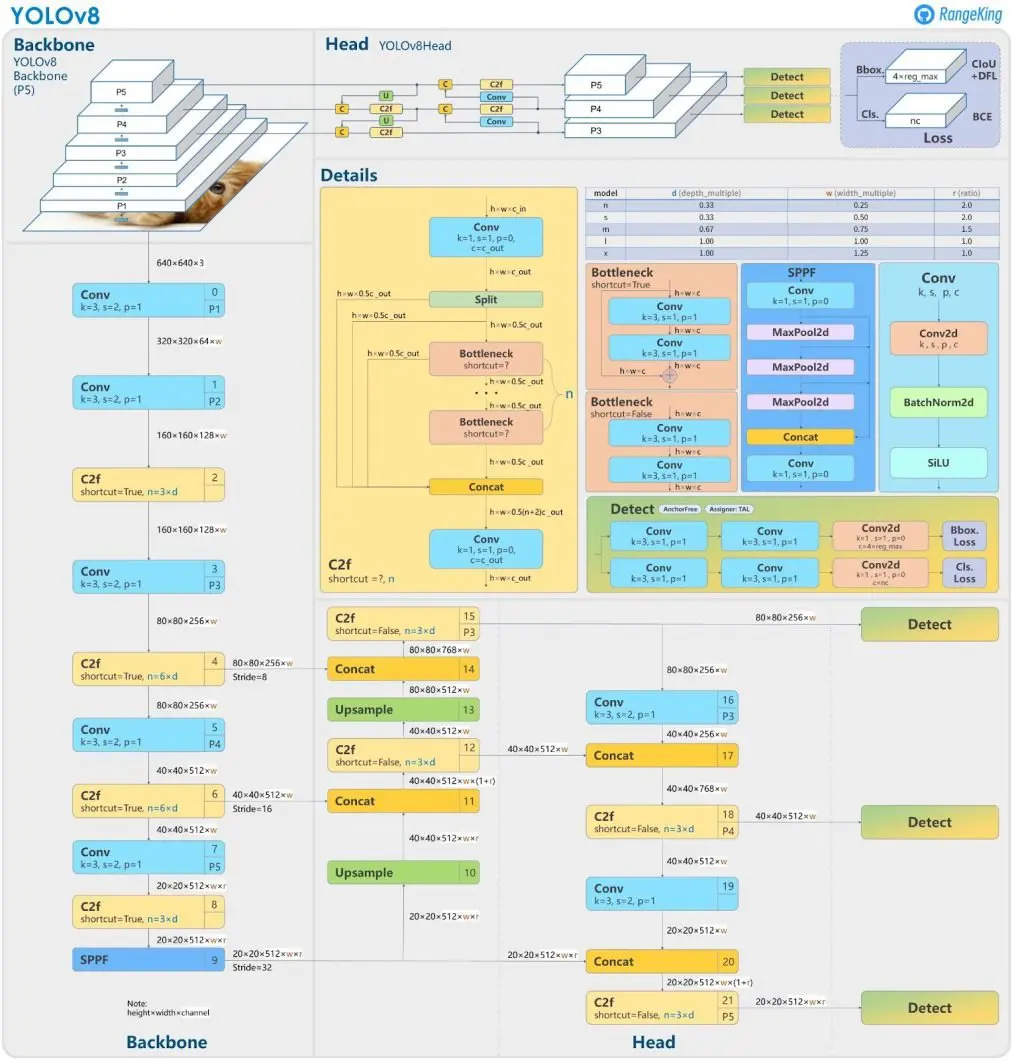
The main features of YOLOv8 include mosaic data augmentation, anchor-free detection, a C2f module, a decoupled head, and a modified loss function.
Let’s discuss each change in more detail.
Mosaic Data Augmentation
Like YOLOv4, YOLOv8 uses mosaic data augmentation that mixes four images to provide the model with better context information. The change in YOLOv8 is that the augmentation stops in the last ten training epochs to improve performance.
Anchor-Free Detection
YOLOv8 switched to anchor-free detection to improve generalization. The problem with anchor-based detection is that predefined anchor boxes reduce the learning speed for custom datasets.
With anchor-free detection, the model directly predicts an object’s mid-point and reduces the number of bounding box predictions. This helps speed up Non-max Suppression (NMS) – a pre-processing step that discards incorrect predictions.
C2f Module
The model’s backbone now consists of a C2f module instead of a C3 one. The difference between the two is that in C2f, the model concatenates the output of all bottleneck modules. In contrast, in C3, the model uses the output of the last bottleneck module.
A bottleneck module consists of bottleneck residual blocks that reduce computational costs in deep learning networks.
This speeds up the training process and improves gradient flow.
Decoupled Head
The diagram above illustrates that the head no longer performs classification and regression together. Instead, it performs the tasks separately, which increases model performance.
Loss
Misalignment is possible since the decoupled head separates the classification and regression tasks. It means the model may localize one object while classifying another.
The solution is to include a task alignment score based on which the model knows a positive and negative sample. The task alignment score multiplies the classification score with the Intersection over Union (IoU) score. The IoU score corresponds to the accuracy of a bounding box prediction.
Based on the alignment score, the model selects the top-k positive samples and computes a classification loss using BCE and regression loss using Complete IoU (CIoU) and Distributional Focal Loss (DFL).
The BCE loss simply measures the difference between the actual and predicted labels.
The CIoU loss considers how the predicted bounding box is relative to the ground truth in terms of the center point and aspect ratio. In contrast, the distributional focal loss optimizes the distribution of bounding box boundaries by focusing more on samples that the model misclassifies as false negatives.
YOLOv8 Implementation
Let’s see how you can implement YOLOv8 on your local machine for object detection. The benefit of YOLOv8 is that Ultralytics allows you to apply the model directly through the CLI and as a Python package.
CLI Implementation
You can start using the model by running pip install ultralytics
in the Anaconda command prompt.
After installation, you can run the following command, which trains the YOLOv8 nano model on the COCO dataset with ten training epochs and a learning rate of 0.01.
yolo train data=coco128.yaml model=yolov8n.pt epochs=10 lr0=0.01
You can view the CLI syntax for other operations on the Ultralytics CLI guide.
Python Implementation
The example below shows how you can quickly fine-tune the YOLOv8 nano model on a custom dataset for object detection.
The data used comes from the Open Images Dataset v7 for object detection. The images consist of ducks with bounding box labels.
The publicly available sample for fine-tuning is on Kaggle, which contains 400 training and 50 validation images. The bounding box labels consist of x-y coordinates.
You can follow along the steps using the Google Colab notebook.
Step 1
The first step is to install the Ultralytics package.
!pip install ultralytics
Step 2
Next, we will import the relevant packages.
from ultralytics import YOLO
from google.colab import files
Step 3
Then, we will import our dataset using the Kaggle API. You must create an account on Kaggle to get your unique API key and download the related Kaggle JSON file.
Once the JSON file is on your local machine, you can upload it on Colab using the following:
files.upload()
A prompt will ask you to upload the file from your local machine.
You can run the following commands to mount the data on your Google Drive.
!rm -r ~/.kaggle
!mkdir ~/.kaggle
!mv ./kaggle.json ~/.kaggle/
!chmod 600 ~/.kaggle/kaggle.json
!kaggle datasets download -d haziqasajid5122/yolov8-finetuning-dataset-ducks
!unzip yolov8-finetuning-dataset-ducks -d /content/Data
!cp /content/Data/config.yaml /content/config.yaml
YAML is the standard YOLO dataset format. In our case, it’s the file: config.yaml
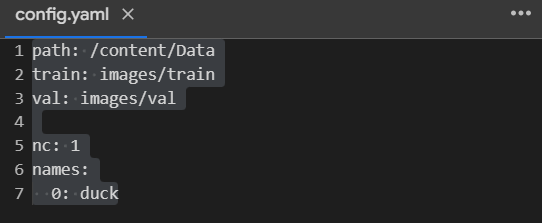
In our case, there’s just one class, “duck.”
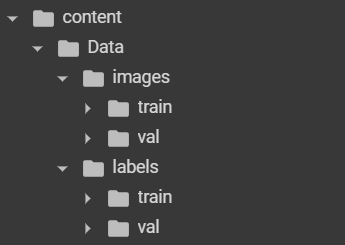
There can be an additional folder for the test set.
Step 4
Load the YOLOv8 nano model as follows:
model = YOLO("yolov8n.pt")
Step 5
Fine-tune the model with the following command:
results = model.train(data="/content/config.yaml", epochs=20)
This will train the YOLOv8 model on 20 training epochs. You can define further hyperparameters based on your requirements.
You must ensure you select a T4 GPU for faster training.
Step 6
You can load the best model and run your predictions on an image.
infer = YOLO("/content/runs/detect/train32/weights/best.pt")
results = infer.predict("/content/Data/images/val/0f5e9d02e8b110a5.png", save=True)
Step 7
You can view the image’s predicted bounding box and classification score by going to “content/runs/detect/predict” from the left menu bar. It gives the following result:
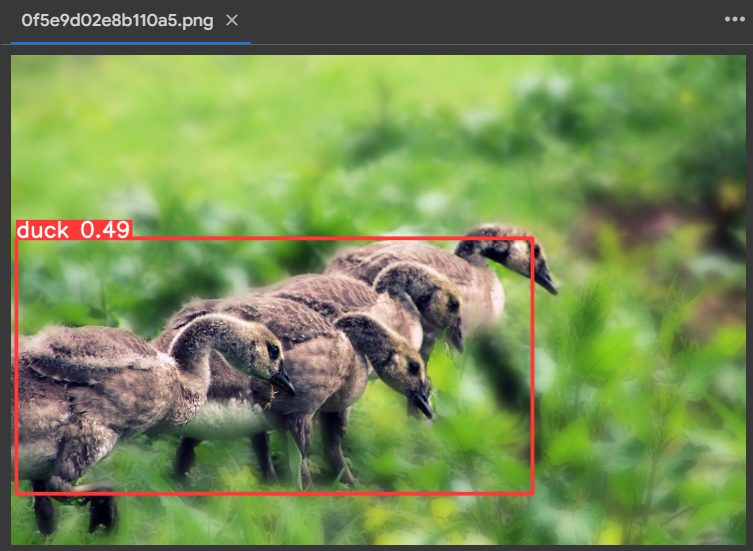
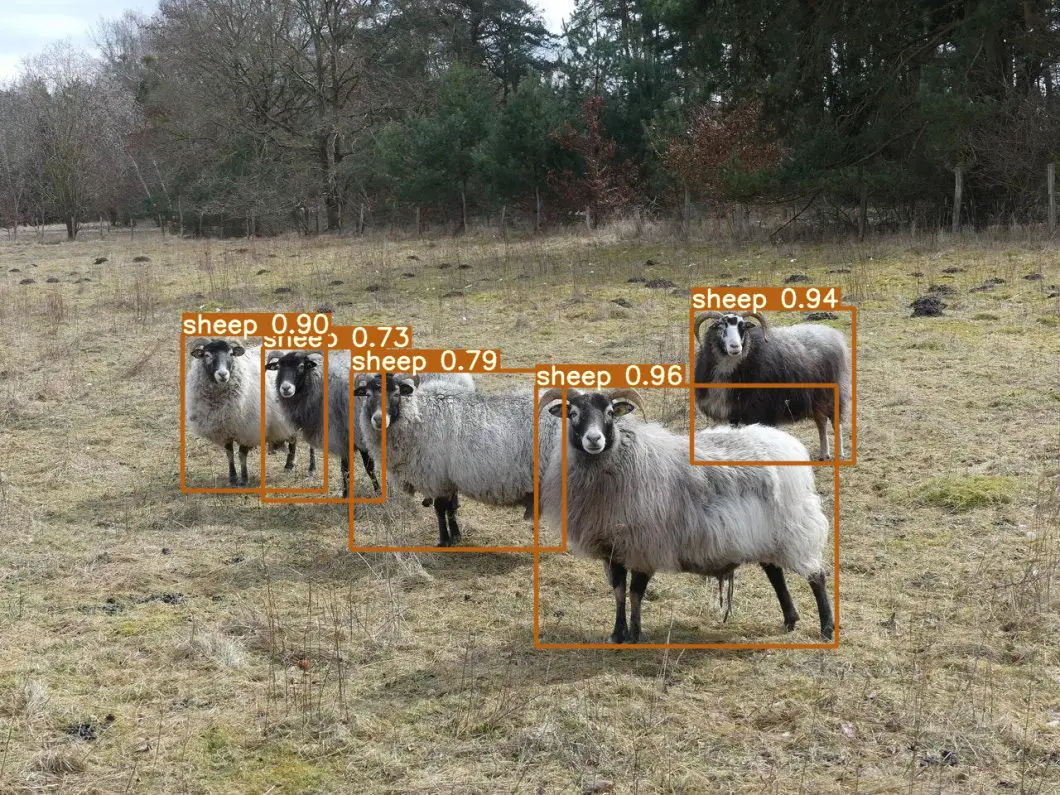
YOLOv8 Applications
YOLOv8 is a versatile model that you can use in several real-world applications. Below are a few popular use cases.
- People counting: Retailers can train the model to detect real-time foot traffic in their shops, detect queue length, and more.
- Sports analytics: Analysts can use the model to track player movements in a sports field to gather relevant insights regarding team dynamics (See AI in sports).
- Inventory management: The object detection model can help detect product inventory levels to ensure sufficient stock levels and provide information regarding consumer behavior.
- Autonomous vehicles: Autonomous driving uses object detection models to help navigate self-driving cars safely through the road.
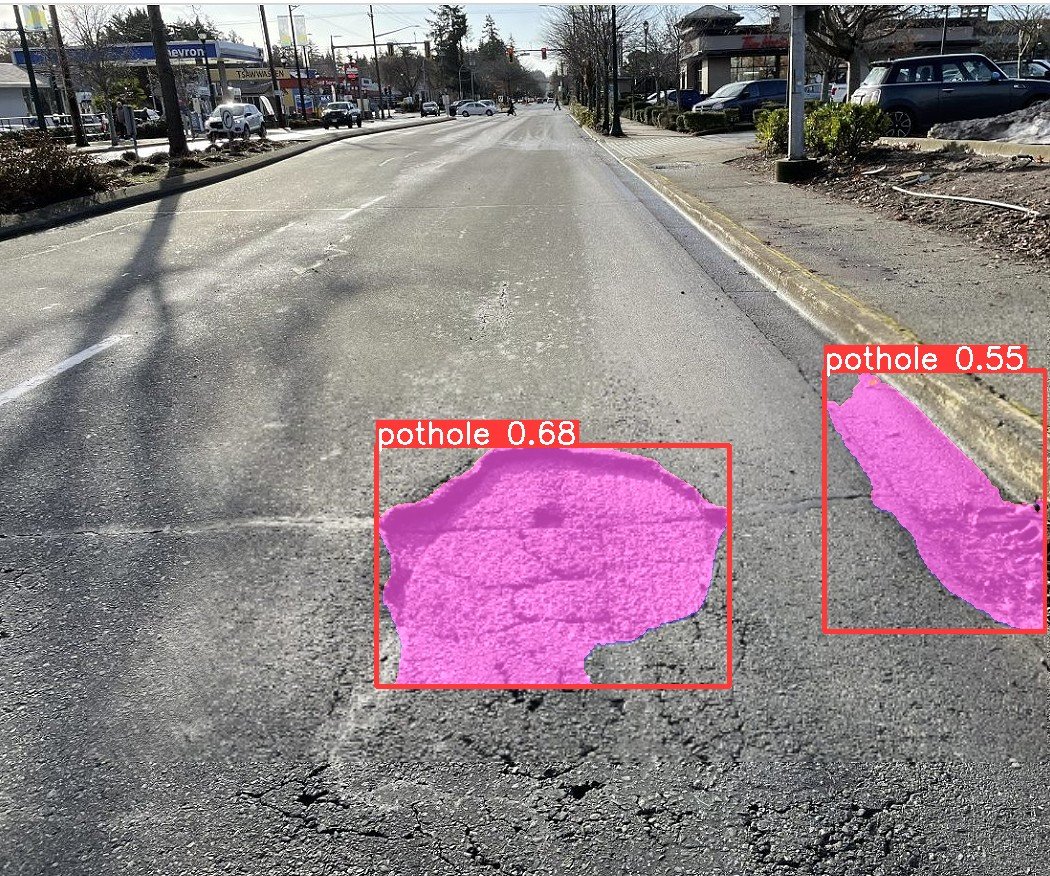
YOLOv8: Key Takeaways
The YOLO series is the standard in the object detection space with its exemplary performance and broad applicability. Here are a few things you should remember about YOLOv8.
- YOLOv8 improvements: YOLOv8’s primary improvements include a decoupled head with anchor-free detection and mosaic data augmentation that turns off in the last ten training epochs.
- YOLOv8 tasks: Besides real-time object detection with cutting-edge speed and accuracy, YOLOv8 is efficient for classification and segmentation tasks.
- Ease-of-use: With an easy-to-use package, users can implement YOLOv8 quickly through the CLI and Python IDE.
You can read related topics in the following articles:
- What is computer vision?
- Latest computer vision trends, including the Segment Anything Model (SAM)
- Data augmentation techniques and new tools for image annotation
- A guide to why computer vision projects fail
Real-Life Computer Vision for Business
While implementing YOLOv8 in isolation is quick and easy for high-performance object detection tasks. However, using it in a full-fledged computer vision system and business-critical applications is a huge challenge.
Viso.ai can help you implement computer vision models in an end-to-end computer vision system through the Viso Suite that integrates simply with the model frameworks.
The Viso computer vision platform is also useful in helping you annotate data in the required format for use in YOLO models, train custom YOLO models, and deploy them at scale.
Request a demo to see how your team can solve complex business problems with computer vision.