Self-supervised learning is a machine learning approach known for its efficiency and ability to generalize. In this article, we’ll dive into the techniques, latest research, and advantages of self-supervised learning, and explore how it is being used in computer vision.
- Background and definition of self-supervised learning
- The differences between supervised and unsupervised learning.
- Challenges and advantages of self-supervised learning
- The learning process and popular methods
- Recent research and applications of self-supervised learning.
About us: Viso Suite is the leading Computer Vision Platform used by enterprises to build and deliver real-world AI applications. Request a demo for your organization!
What Is Self-Supervised Learning?
Self-supervised learning has drawn massive attention for its excellent data efficiency and generalization ability. This approach allows neural networks to learn more with fewer labels, smaller samples, or fewer trials.
Recent self-supervised learning models include frameworks such as Pre-trained Language Models (PTM), Generative Adversarial Networks (GAN), Autoencoder and its extensions, Deep Infomax, and Contrastive Coding. We will cover those later in more detail.
Background of Self-Supervised Learning
The term “self-supervised learning” was first introduced in robotics, where the training data is automatically labeled by finding and exploiting the relations between different input signals from sensors. The term was then borrowed from the field of machine learning.
The self-supervised learning approach can be described as “the machine predicts any parts of its input for any observed part.” The learning includes obtaining “labels” from the data itself by using a “semiautomatic” process. Also, it is about predicting parts of data from other parts.
Here, the “other parts” could be incomplete, transformed, distorted, or corrupted fragments. In other words, the machine learns to “recover” whole, parts of, or merely some features of its original input.
To learn more about these types of machine learning concepts, check out our article about supervised vs. unsupervised learning.
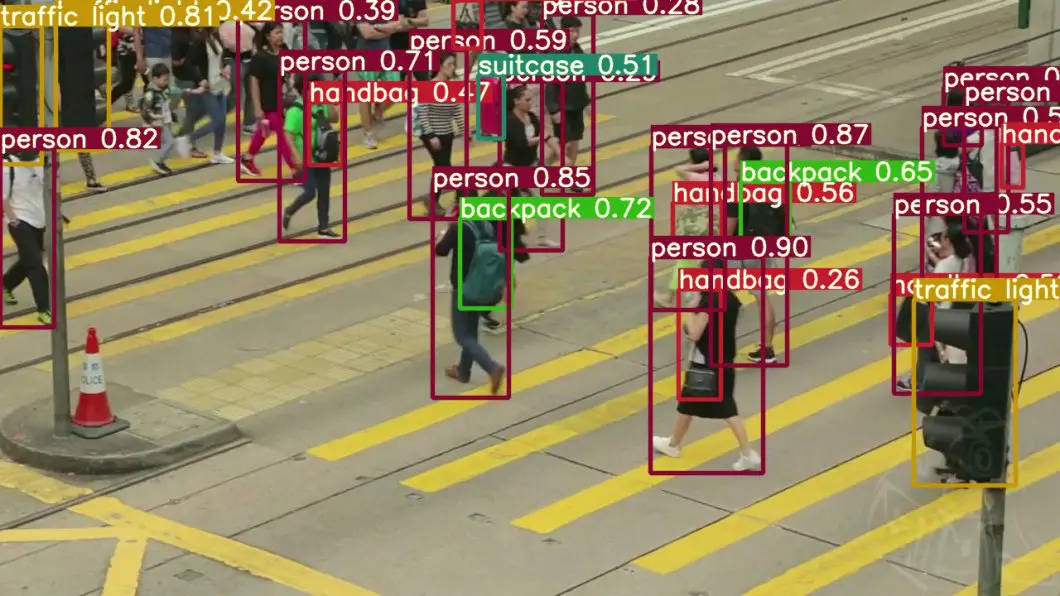
How it works: Self-Supervised Learning Is “Filling in the Blanks”
People often tend to confuse the terms Unsupervised Learning (UL) and Self-Supervised Learning (SSL). Self-supervised learning can be considered as a branch of unsupervised learning since there is no manual labeling involved. More precisely, unsupervised learning focuses on detecting specific data patterns (such as clustering, community discovery, or anomaly detection), while self-supervised learning aims at recovering missing parts, which is still in the paradigm of supervised settings.
Self-Supervised Learning Examples
Here are some practical examples of self-supervised learning:
- Example #1: Contrastive Predictive Coding (CPC): a self-supervised learning technique used in natural language processing and computer vision, where the model is trained to predict the next sequence of input tokens.
- Example #2: Image Colorization: a self-supervised learning technique where a black-and-white image is used to predict the corresponding colored image. The technique uses GANs to train computer vision models for tasks such as image recognition, image classification, image segmentation, and object detection.
- Example #3: Motion and Depth Estimation: a self-supervised learning technique used to predict motion and depth from video frames. This is an example of how self-supervised learning is used for training autonomous vehicles to navigate and avoid obstacles based on real-time video.
- Example #4: Audio Recognition: a self-supervised learning technique where the model is trained to recognize spoken words or musical notes. This technique is useful for training speech recognition and music recommendation systems.
- Example #5: Cross-modal Retrieval: a self-supervised learning technique where the model is trained to retrieve semantically similar objects across different modalities, such as images and text. This technique is useful for training recommender systems and search engines.
These are just a few self-supervised learning examples and use cases, there are many other applications in various fields, such as medicine, finance, and social media analysis.
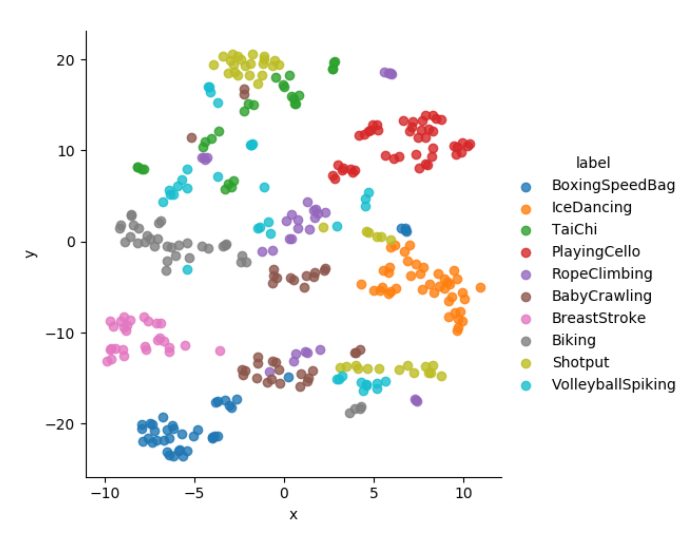
The Bottlenecks of Self-Supervised Learning
Deep neural networks have shown excellent performance on various machine learning tasks, especially on supervised learning in computer vision. Modern computer vision systems achieve outstanding results by performing a wide range of challenging vision tasks, such as object detection, image recognition, or semantic image segmentation.
However, supervised learning is trained over a specific task with a large manually labeled dataset which is randomly divided into training, validation, and test sets. Therefore, the success of deep learning-based computer vision relies on the availability of a large amount of annotated data which is time-consuming and expensive to acquire.
Besides the expensive manual labeling, supervised learning also suffers from generalization errors, spurious correlations, and adversarial machine learning attacks.
Disadvantages and Advantages of Self-Supervised Learning
For some scenarios, building large labeled datasets to develop computer vision models is not practically feasible:
- Most real-world computer vision applications involve visual categories that are not part of a standard benchmark dataset.
- Also, some applications underlay a dynamic nature where visual categories or their appearance change over time.
Hence, self-supervised learning could be developed that can successfully learn to recognize new concepts by leveraging only a small amount of labeled examples.
The ultimate goal is enabling machines to understand new concepts quickly after seeing only a few examples that are labeled, similar to how fast humans can learn.
Advantages of self-supervised learning | Disadvantages of self-supervised learning |
---|---|
Requires less labeled data than supervised learning | Can require more computation and resources |
Enables learning from unlabeled data, which is more abundant and easier to acquire in some cases | Pretext tasks can be challenging to formulate and may require expert knowledge |
Can recognize new concepts after seeing only a few labeled examples | May not perform as well as supervised learning on some tasks |
Resistant to adversarial machine learning attacks | May suffer from overfitting and generalization errors on some tasks |
Can be used in a wide range of applications, including computer vision, natural language processing, and speech recognition | Some applications may still require large labeled datasets |
Enables the development of more efficient and generalizable models |
Note that this table is not exhaustive, and the advantages and disadvantages depend on the specific implementation and applications of self-supervised learning.
Self-Supervised Visual Representation Learning
Learning from unlabeled data that is much easier to acquire in real-world applications is part of a large research effort. Recently, the field of self-supervised visual representation learning has demonstrated the most promising results.
Self-supervised learning techniques define pretext tasks that can be formulated using only unlabeled data but do require higher-level semantic understanding to be solved. Therefore, models trained for solving these pretext tasks learn representations that can be used for solving other downstream tasks of interest, such as image recognition.
In the computer vision community, multiple self-supervised methods have been introduced.
- Learning representation methods were able to linearly separate between the 1000 ImageNet categories.
- Diverse self-supervision techniques were used for predicting the spatial context, colorization, and equivariance to transformations alongside unsupervised techniques such as clustering, generative modeling, and exemplar learning.
Recent research about self-supervised learning of image representations from videos:
- Methods were used to analyze the temporal context of frames in video data.
- Temporal coherence was exploited in a co-training setting by early work on learning convolutional neural networks (CNNs) for visual object detection and face detection.
- Self-supervised models perform well on tasks such as surface normal estimation, detection, and navigation.
Self Supervised Learning Algorithms
In the following, we list the most important self-supervised learning algorithms:
Autoencoders
Autoencoding is a self-supervised learning technique that involves training a neural network to reconstruct its input data. The autoencoder model is trained to encode the input data into a low-dimensional representation and then decode it back to the original input.
The objective is to minimize the difference between the input and the reconstructed output. In general, autoencoders are widely used for image and text data. An example of autoencoding is the denoising autoencoder, where a model is trained to reconstruct clean images from noisy inputs.
Simple Contrastive Learning (SimCLR)
SimCLR is a simple framework for contrastive learning of visual representations, the model maximizes the agreement between different augmentations of the same image. A SimCLR model is trained to recognize the same image under different transformations, such as rotation, cropping, or color changes. For example, SimCLR can be used to learn representations for image classification or object detection.
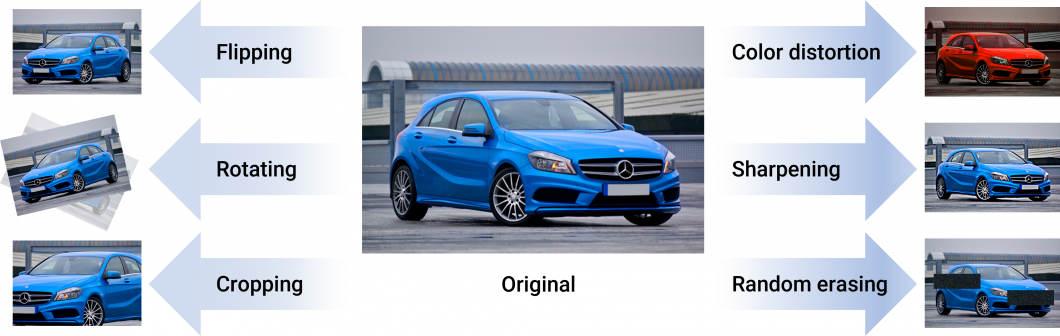
Pre-trained Language Models (PTM)
Pre-Trained neural language Models (PTM) are self-supervised learning algorithms used for natural language processing (NLP), where the machine learning model is trained on large amounts of text data to predict missing words or masked tokens. PTMs are often used for language modeling, text classification, and question-answering systems.
Deep InfoMax
Deep InfoMax is a deep neural network architecture used for learning high-level representations of data. The model is trained to learn the underlying structure and dependencies between the input features. In image recognition, for example, a model may be trained to predict the orientation of an image patch based on the surrounding patches.
Contrastive Learning
A contrastive learning approach trains a model to distinguish between similar and dissimilar pairs of data points. The goal is to learn a representation where similar data points are mapped close together, and dissimilar points are far apart.
A popular algorithm in this category is Contrastive Predictive Coding (CPC), which learns representations by predicting future data given the current context. For example, given a sequence of images, CPC learns to predict the next image, contributing to tasks like logistic regression.
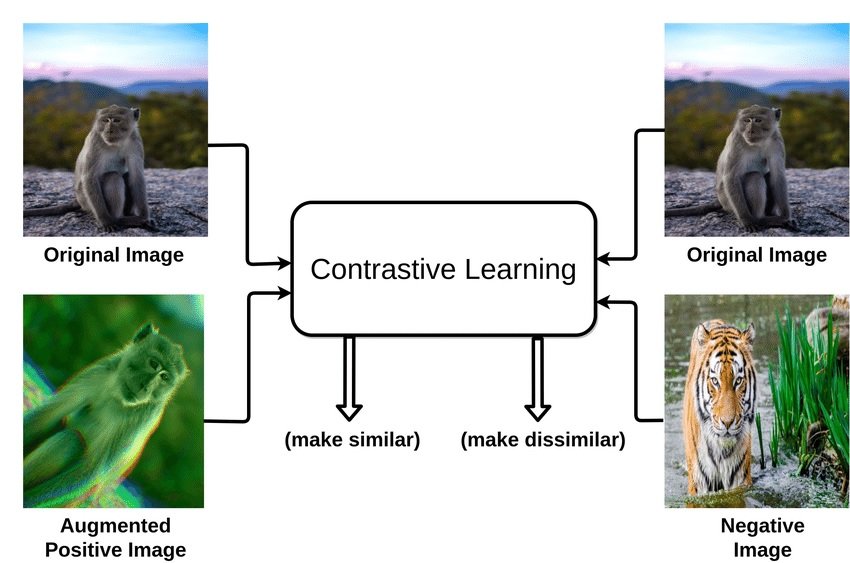
Generative Models
Generative models learn to generate new data points that are similar to the training data. One popular example is Generative Adversarial Networks (GANs). GANs consist of a generator producing synthetic data points and a discriminator distinguishing between synthetic and real data points.
The generator is trained to generate data that can fool the discriminator into thinking it is real. For instance, GANs can be used to generate realistic images of animals, landscapes, or even faces.
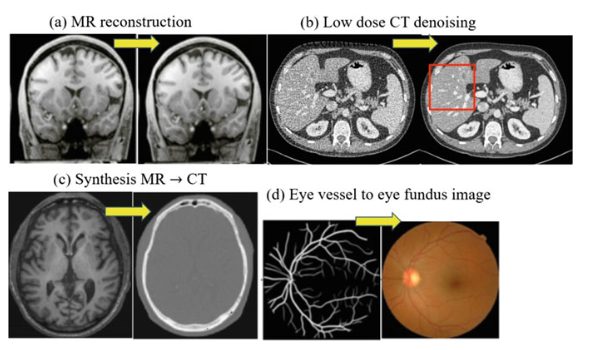
Pretext Tasks
These auxiliary tasks can be used to train a model to learn useful representations of the input data. For example, a model can be trained to predict the missing word in a sentence, to predict the next word given the previous ones, or to classify the rotation angle of an image.
By solving these tasks, the model learns to extract relevant features from the input data that can be used for downstream tasks. This includes linear regression and various linguistic applications, predicting the target variable in different scenarios.
Clustering
Clustering is a method for grouping similar data points. The clustering method trains a model to predict the cluster assignments of data points. It is trained to minimize the clustering loss, measuring how well the predicted clusters match the actual ones. For example, a model can be trained to cluster images of cars based on their make and model, without any explicit labels for the car make and model.
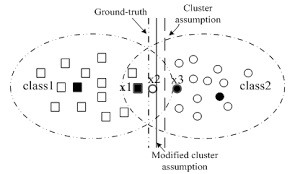
Self-Supervised Learning in Computer Vision
Self-supervised learning is popular due to the availability of large amounts of unlabeled image data. The objective is to learn meaningful representations of images without explicit supervision, such as image annotation.
In computer vision, self-supervised learning algorithms can learn representations by solving tasks such as image reconstruction, colorization, and video frame prediction, among others. In particular, models such as contrastive learning and autoencoding have shown promising results in learning representations. These can be used for downstream tasks such as image classification, object detection, and semantic segmentation.
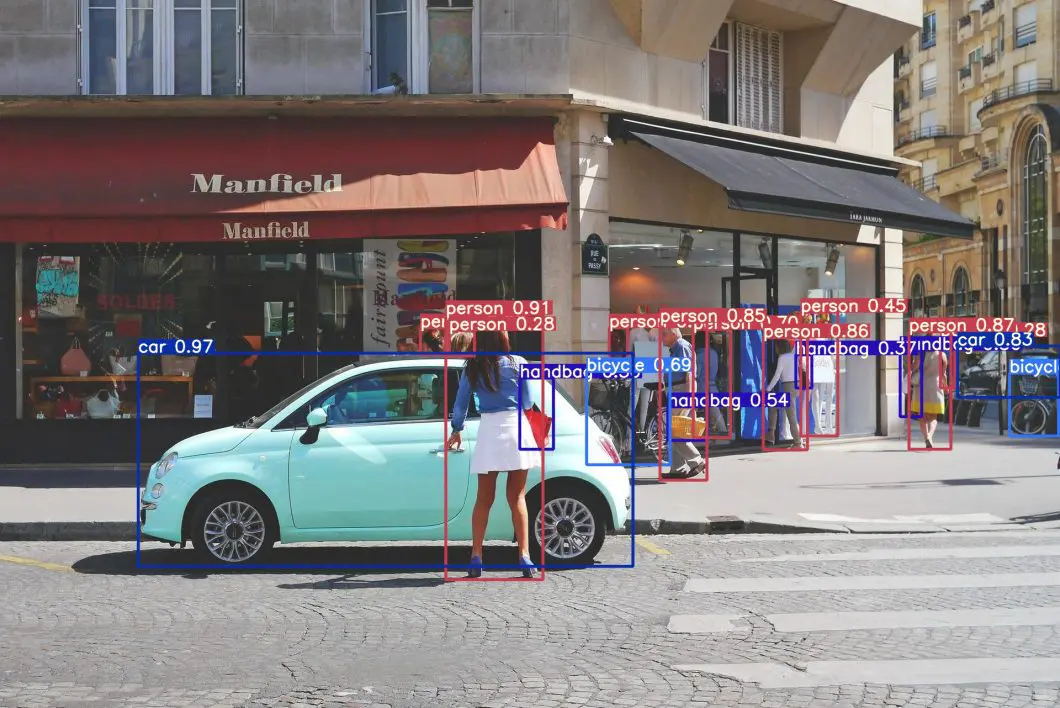
Additionally, self-supervised machine learning can also be used to improve the performance of supervised learning models by pretraining on large amounts of unlabeled data. Hence, self-supervised learning has been shown to improve the robustness and performance of supervised learning models.
This is especially valuable in scenarios where labeled data is scarce or expensive to obtain. For example, in medical applications and medical imaging with novel diseases or rare conditions.
What’s Next in Self-Supervised Learning?
In summary, supervised learning works well but requires many labeled samples and a significant amount of data. It is about training a machine by showing examples instead of programming it. This field is considered to be key to the future of deep learning-based systems. If you enjoyed reading this article, we recommend:
- How does Deep Reinforcement Learning work?
- Read an Introduction to Semi-Supervised Machine Learning Models
- A complete list of 100 computer vision applications