Given the recent explosion of progress in Machine Learning (ML), most companies are trying to figure out how to implement AI within their own businesses. Computer vision in particular is a strategic advantage for businesses that know how to use it. From automated inventory tracking to employee productivity quantification, computer vision has the power to significantly improve operational efficiency and the quality of human decision-making.
In this article, we will explore several examples of how real-world companies are driving business value with specific computer vision implementations. Along the way, we will cover the key considerations, challenges, and opportunities associated with actually integrating computer vision into a business’s operations. Let’s dive in.
About us: Viso Suite is a flexible and scalable infrastructure developed for enterprises to integrate computer vision into their tech ecosystems seamlessly. Viso Suite allows enterprise ML teams to train, deploy, manage, and secure computer vision applications in a single interface. To learn more, book a demo with our team.
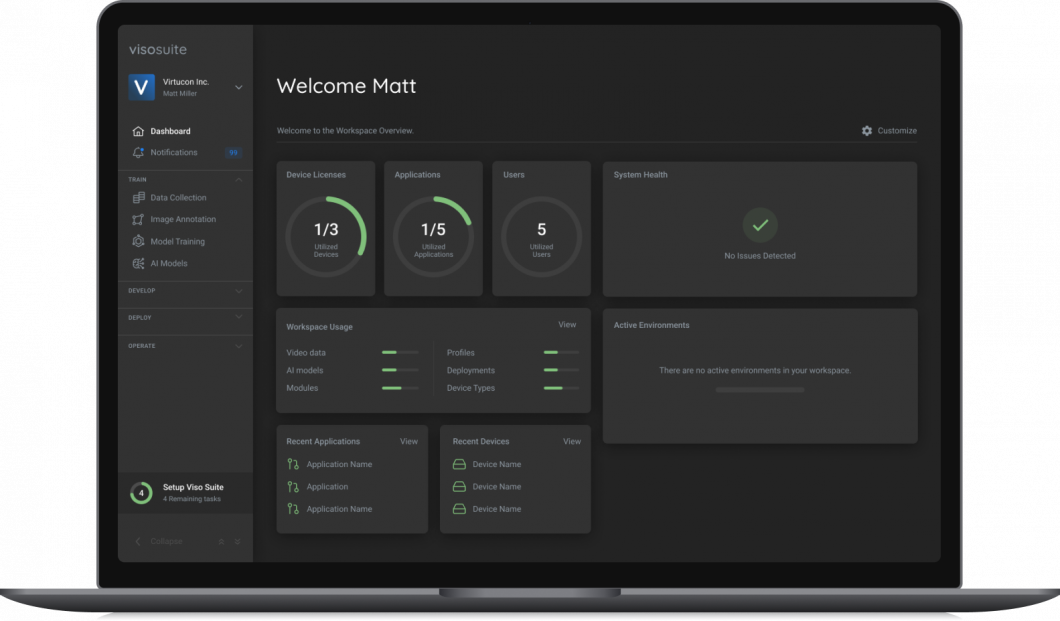
Real-World Computer Vision Implementation Examples
Example #1: Inventory Tracking
A single retail store can often carry tens of thousands of different products. Tracking which products are still stocked on the shelves is a monumental task. Most stores rely on a combination of label scanning and frequent employee inspections to track this information.
However, even with all those employee hours spent, the results are frequently inaccurate. Products sell out without anyone noticing, or products are moved around by customers. Computer vision can solve these problems by:
- Automatically detecting which products are still stocked on the shelves, and
- Detecting when a customer has returned a product to the wrong shelf.
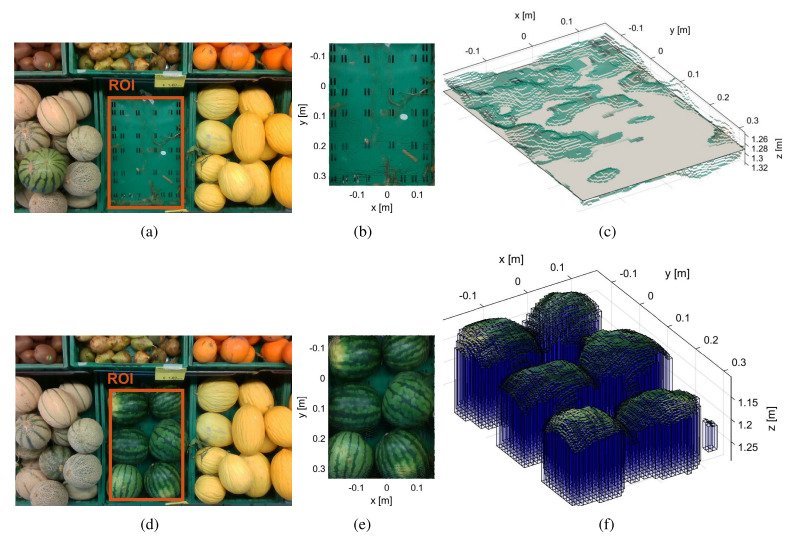
Dedicated retail stores are not the only businesses that can benefit from computer vision inventory tracking. Restaurants, salons, and mechanic shops are all businesses that need to carry an assortment of products and supplies at all times. In terms of high-level strategy, any business that either (1) sells multiple products out of a physical location, or (2) uses multiple consumable supplies or ingredients, might be able to benefit from Artificial Intelligence (AI) inventory tracking.
Example #2: Quality Control Inspections
From consumer packaged goods brands to pharmaceutical companies, every manufacturer has a quality control process. Quality control ensures that products are manufactured to the company’s standards. For example, automobile manufacturers rely on visual inspections at multiple stages of the assembly process to ensure parts are properly put together.
Traditionally, companies relied on humans to manually inspect some percentage of manufactured items. However, modern manufacturers frequently rely on computer vision techniques to ensure that every item is manufactured to a minimum standard.
Farms and grocery stores also implement quality control processes to ensure that the food they sell satisfies certain standards. Increasingly, those industries are also turning to computer vision.
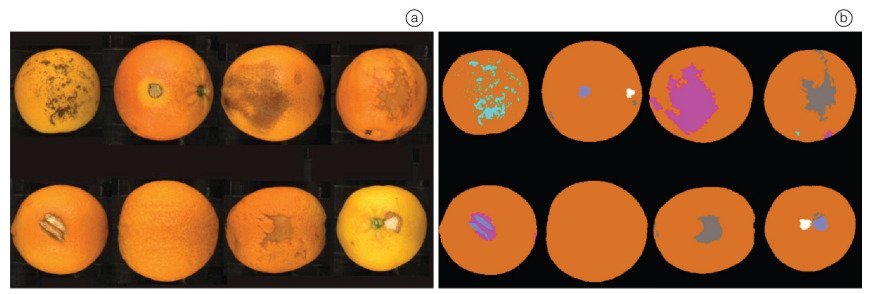
Example #3: Monitoring Customer Status & Predicting Customer Needs
A single server at a restaurant will often be responsible for dozens of customers per hour. That server will have to interact with each of those customers multiple times over that period. During the busiest times, that can often degrade the customer experience. Some customers are checked in on when they don’t need anything while others sit there waiting for several minutes to get a water refill.
Computer vision technology can significantly reduce this problem by automatically tracking customer behavior. Instead of constantly wandering around checking every table, a server can rely on an AI computer vision system to alert them when a customer needs a drink refill or has finished eating and is ready for the check.
Hospitals can benefit from a similar AI-driven system. ER patients frequently change their condition. Some of those conditions are monitored by specialized machines. For example, if a patient’s heart stops, that is detected by a heart rate monitor.
However, other conditions are not explicitly monitored by machines. Nevertheless, those conditions are frequently accompanied by visual indicators. For example, the patient might start sweating profusely or their skin may get pale. A computer vision model trained on labeled data of those conditions can give doctors and nurses an immediate warning when those visual symptoms are detected.
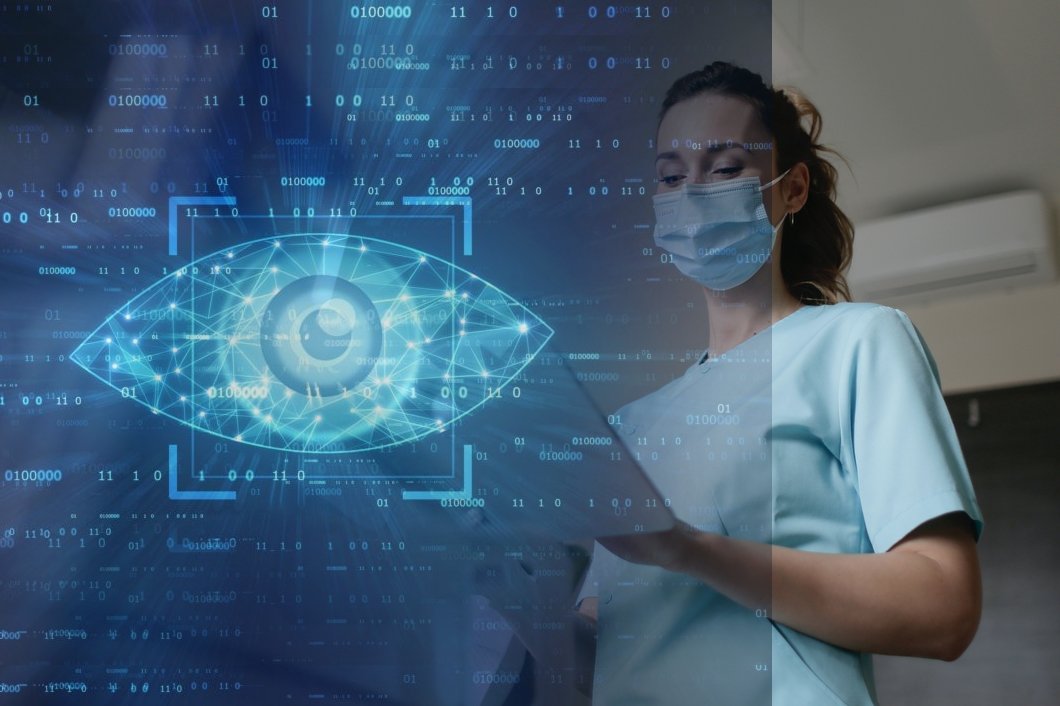
Example #4: Employee Efficiency Tracking
Restaurants, retail stores, and warehouses employ hundreds of millions of low-skill workers around the world. Identifying which of these workers are suitable for promotion can be a time-consuming task for managers. However, computer vision can substantially reduce the time required by automatically tracking employee productivity.
A computer vision system can track the average number of tables that a restaurant server serves per hour, the average number of times a retail sales worker gets on their phone during a shift, or the average square feet of floor cleaned per hour by a janitorial worker.
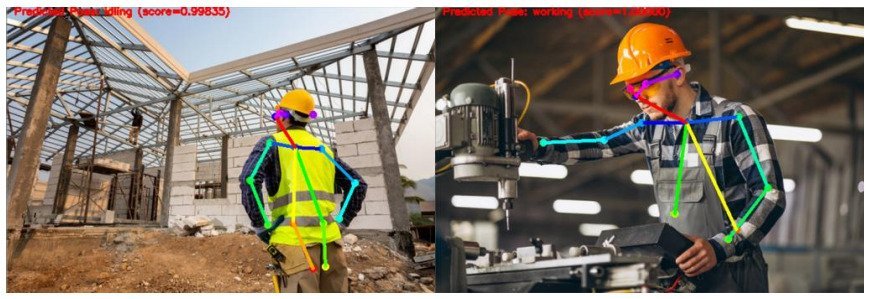
By using computer vision to quantify and track employee productivity, companies can make data-driven decisions on who to promote and who to fire. That directly helps the company by increasing the average quality of the workforce. However, it also indirectly helps the company by increasing employee morale. Employees are more motivated when they see their most productive colleagues getting promoted.
Example #5: Employee Compliance Tracking
Many businesses have standard operating procedures (SOPs) that their employees are expected to abide by. For a restaurant, those SOPs might include making a certain offer to every customer or cleaning tables in a certain way.
For a construction company, SOPs might include mandatory safety procedures. SOPs help make companies safe and efficient. However, it’s often difficult to know whether your employees are consistently following the SOPs.
Computer vision can solve that problem by automatically tracking whether employees are following certain SOPs. This works best for employees involved in physical labor. For example, construction workers, retail workers, restaurant workers, warehouse workers, and janitorial staff are all typically expected to follow SOPs that can be visually observed with computer vision AI tools. Those tools can then generate automated reports about which employees are chronically noncompliant with their company’s SOPs.
Example #6: Equipment Wear and Tear Monitoring
From a drill press at a manufacturing company to a shelf at a retail store, most businesses have physical equipment that they rely on to conduct business. Over time, that equipment wears down, requiring maintenance or replacement. In many cases, preventative maintenance would be cheaper than replacement.
However, it’s difficult to monitor thousands of pieces of equipment regularly to determine which pieces are wearing faster than expected. That’s where computer vision projects can help. A computer vision monitoring system can be layered on top of existing camera feeds to regularly check and record equipment wear. When that wear reaches a certain threshold of severity, the system can then automatically notify the relevant human decision-makers who can replace the equipment if necessary.
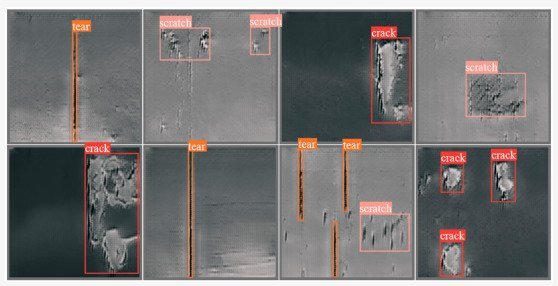
A more extreme version of this is detecting equipment that is critically damaged and poses a safety risk. For example, gyms can use computer vision to automatically detect damaged equipment that poses an unacceptable liability risk. Manufacturing companies are also well-positioned to take advantage of this type of computer vision use case.
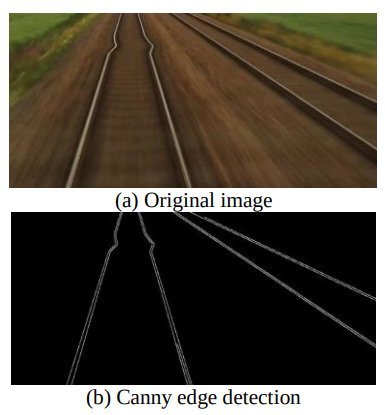
Example #7: Diagnostics
In many cases, computer vision can be used not just to detect equipment issues but also to diagnose them. For example, Amazon uses an automated vehicle inspection system to detect and diagnose common issues in its fleet of over 100,000 delivery vehicles.
Many other companies with large vehicle fleets such as Hertz also use AI solutions to help identify and diagnose malfunctioning equipment. Even auto mechanics can use computer vision to help their employees more efficiently diagnose autonomous vehicle issues. However, vehicles are not the only things computer vision can help diagnose.
Computer vision has proven incredibly effective at diagnosing various veterinary issues.
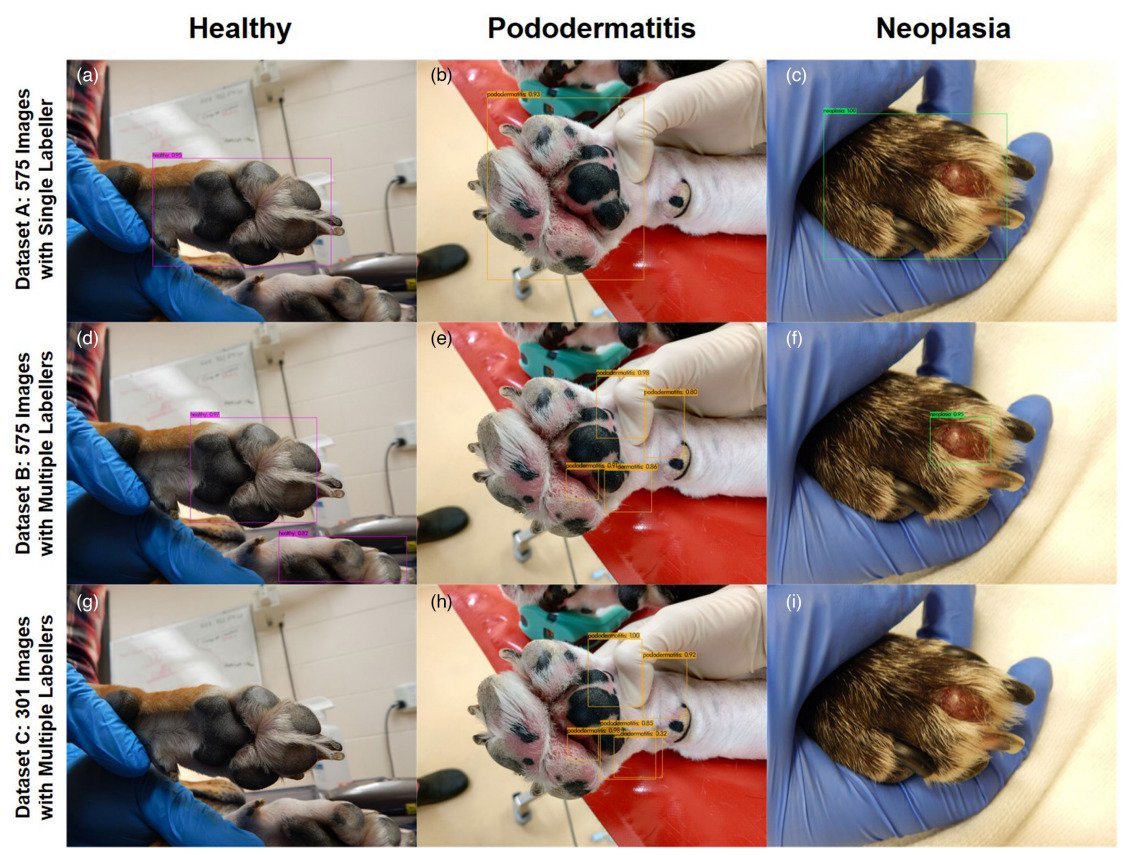
Extensive research has also shown that computer vision can be very effective at diagnosing various health issues in humans. However, regulations in many countries including the U.S. make implementing computer vision difficult for many healthcare providers.
Example #8: Risk or Threat Detection
Security is one of the oldest use cases for computer vision. A simple network of computer vision-equipped cameras can be more effective than several human guards in monitoring a facility for intruders. AI face detection can also be used to authorize entry into sensitive locations such as data centers. However, unauthorized physical access is not the only type of threat that computer vision can help mitigate.
Computer vision can also identify threats to critical infrastructure. For example, several utility companies now use drones equipped with computer vision to detect tree branches that are at risk of bringing down power lines. Additionally, many property insurance companies use drones and computer vision to assess the quality of roofs before issuing a policy.
Example #9: Cleanliness Monitoring
Another valuable use case for computer vision implementations is cleanliness monitoring. Large-footprint retailers, warehouses, restaurants, casinos, and hospitals all need to regularly monitor the conditions of their spaces. Spilled food or other cleanliness issues can degrade customer experiences, reduce employee productivity, and even pose liability risks.
Historically, companies have addressed this need by scheduling periodic inspections and cleanups by janitorial staff. However, computer vision offers a real-time alternative. Employees can be notified instantly whenever something is spilled or whenever a floor or table needs to be cleaned.
Hardware Requirements for Computer Vision Implementations
When planning an AI implementation, it’s always important to include data as a consideration. What data inputs will the model have access to? For computer vision systems in particular, the data inputs will usually come from a set of cameras in and around a business location.
In many cases, a business will already have security cameras that provide the data feeds to run an AI project application. However, in other situations, a business might need to install additional cameras to provide enough visual coverage for a particular computer vision application.
Some particularly sophisticated AI algorithms may combine traditional computer vision with additional data from Lidar systems or 3D scanners. Those applications sometimes outperform pure computer vision models. However, they require expensive specialized hardware. It’s important to estimate the incremental ROI of using such a system over a traditional computer vision model before deciding to invest in specialized hardware.
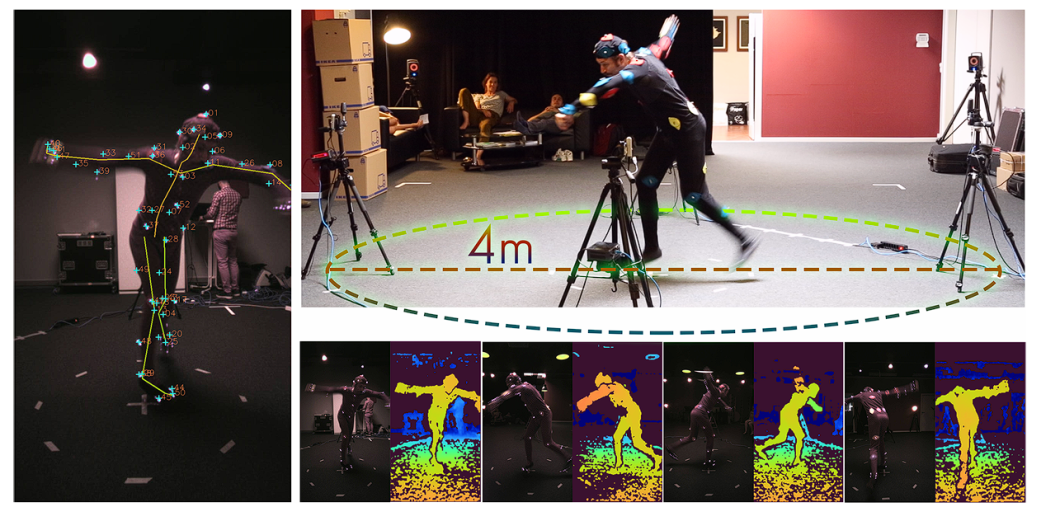
Data Requirements for Computer Vision Implementations
Every AI model has to be trained on data. Sometimes, you can bypass the need for data by using a pre-trained model. However, in many cases, you may still need to fine-tune the pre-trained model before using it. When mapping out the potential ROI of a computer vision implementation, it’s important to ask the following questions:
- Are there any pre-trained computer vision models that already provide the desired type of functionality (for example, intruder face recognition, vehicle part recognition, or customer behavior identification)?
- Suppose there are no pre-trained models that provide the necessary functionality out of the box. Do we have access to a data set of images and videos that are (or could be) labeled with the necessary information to train a custom model? In many cases, there are open-source datasets that can be used.
Future Trends for Computer Vision in Business
The manufacturing industry continues to be a leader in real-world computer vision implementations, and the accelerating adoption of robotics will only continue that trend.
The cost of robots has been dropping exponentially, year after year. The result is that more robots are being used in both industrial and non-industrial settings. Often, those robots rely on computer vision to perform tasks. Additionally, those robots must be regularly monitored for excessive wear and tear, and that will drive further demand for computer vision.
In the restaurant industry, robotics have also been making rapid progress. From food preparation to cleaning, large restaurant chains have increasingly turned to robotics to help them deal with labor costs and shortages. Those use cases will drive an increased need for computer vision systems to operate, maintain, and repair those robots.
In the finance industry, biometrics will continue to drive demand for computer vision systems. Biometric systems that combine facial recognition with “liveness” detection help banks and other financial institutions to reduce fraud.