The accelerating convergence of artificial intelligence (AI) and the Internet of Things (IoT) has sparked a recent wave of interest in Artificial Intelligence of Things (AIoT). This article covers everything you need to know about the basics of the Artificial Intelligence of Things. We will discuss how emerging technology drives the development of disruptive applications, software, sensors, and systems.
In particular, we will cover the following:
- What is the Artificial Intelligence of Things (AIoT)
- Combination and difference of AI and IoT concepts
- Distributed computing and Edge Intelligence
- Benefits and use cases of AIoT
- Popular AIoT Applications
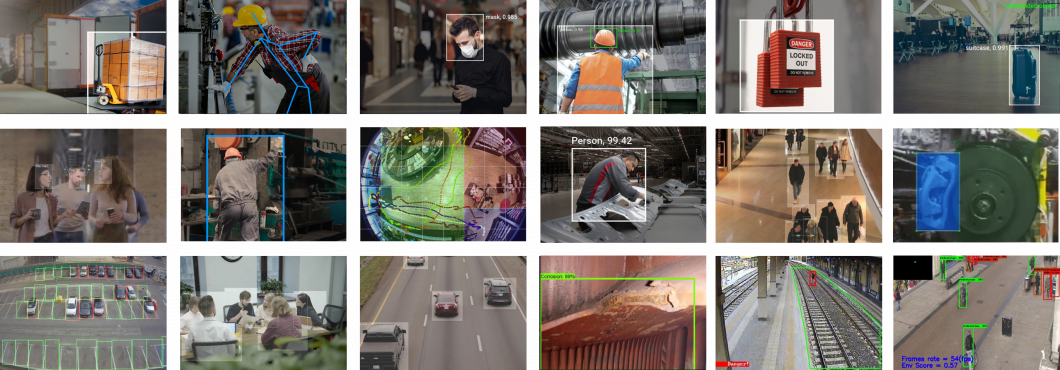
What Is AIoT?
AIoT stands for Artificial Intelligence of Things; it combines the connectivity from the Internet of Things (IoT) with the data-driven knowledge obtained from Artificial Intelligence (AI). This emerging technology is based on the integration of Artificial Intelligence in IoT infrastructure.
By combining IoT with AI, the data collected by distributed nodes can be utilized by applying AI techniques such as machine learning and deep learning. As a result, machine learning capabilities are moved closer to the data source. This concept is called Edge AI, or Edge Intelligence, and it allows higher scalability, robustness, and efficiency.
We at viso.ai specialize in the combination of Computer Vision and IoT, or visual AIoT. We power the end-to-end computer vision platform Viso Suite used by leading companies to power their AI vision applications. The platform provides AI software infrastructure with automated features to develop, deploy, and operate large-scale AIoT applications faster.
AIoT Meaning: The Combination of AI and IoT
To understand the need for combining AI and IoT, we first need to look at the advantages of the two concepts.
- Artificial Intelligence, or AI, is a field of computer science that deals with the development of smart systems that can simulate human intelligence. Simply speaking, AI aims to enable computers to reproduce human capabilities like perception, reasoning, understanding, etc. Hence, the highly disruptive AI capabilities are the foundation of intelligent systems in a wide range of industries to increase efficiency and develop new products and services.
- The Internet of Things, or IoT, on the other hand, is a system of connected objects or devices that can collect and transfer data in real-time with the help of software or sensors embedded within them. The application of IoT helps achieve a high level of automation in a wide range of tasks across industries. Through sensors or user input, IoT devices create a massive amount of data.
The combination of the two concepts (AI, IoT) focuses on using AI capabilities to process the data generated and collected by IoT systems. Therefore, machine learning models in AI systems are combined with the connectivity and data transfer capabilities of the Internet of Things. In other words, with the incorporation of AI in IoT systems, their functioning is not limited to collecting and transferring information but actually understanding and analyzing the data.
Edge Computing AIoT
In recent years, the Edge Computing paradigm has gained considerable popularity. It serves as a key enabler for many future technologies like the Internet of Things (IoT), 5G, and artificial intelligence. Hence, Hence, Edge Computing is the primary driver of AIoT; it moves data processing from the Cloud to the network edge.
Edge-Computing-Powered Artificial Intelligence of Things
The concept of Edge Computing promotes distributed system designs with on-device data processing that is highly efficient, scalable, robust, and suitable for low-latency use cases. Initially, Machine Learning and Deep Learning were limited to the Cloud, mainly because of the availability and scalability of the high computational resources required to process ML tasks.
By exploiting the novel paradigm of edge intelligence, emerging computationally intensive and resource-demanding AIoT applications can be efficiently supported at the network edge. Therefore, Edge Computing is essential to achieve the fast processing capacity and low latency required in intelligent IoT applications.
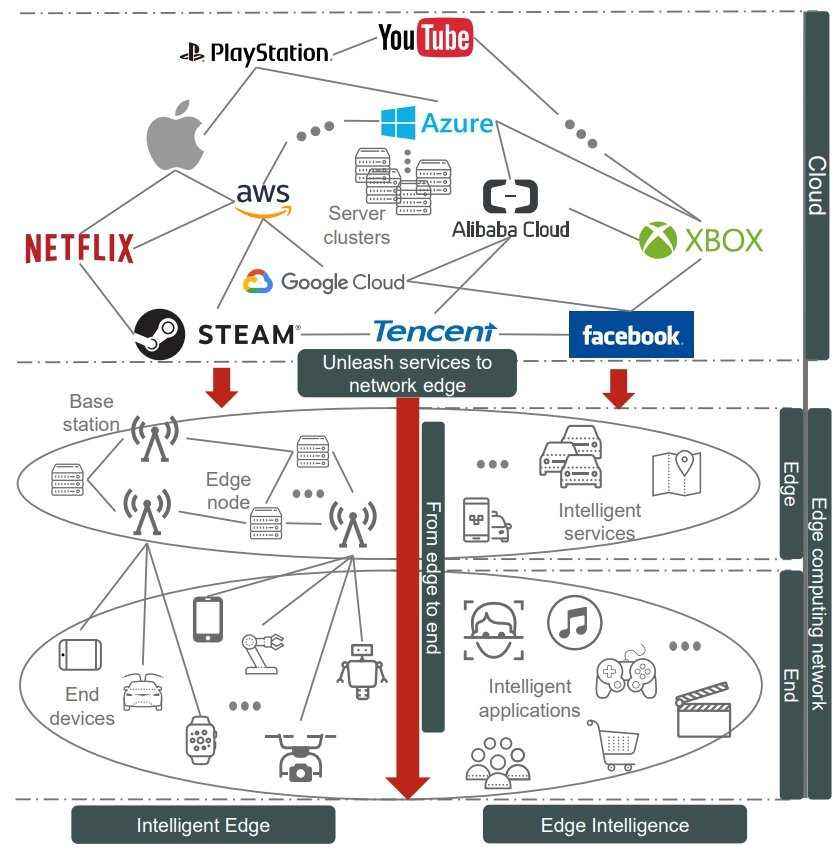
On-device Machine Learning With AIoT Devices
The recent advances in hardware and machine learning have accelerated the deployment of billions of interconnected, intelligent, and adaptive devices in critical infrastructures like health, environmental control, logistics, transportation, and agriculture. Moving the AI processing from the Cloud to distributed, connected edge devices provides a solution to overcome the bottlenecks, latency, and privacy issues of cloud-based AI applications.
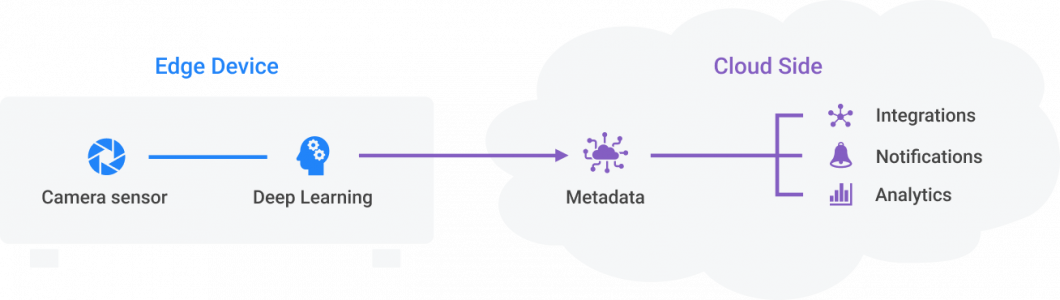
Compared to traditionally low-powered IoT devices, AIoT demands edge devices with sufficient computing resources to perform on-device machine learning tasks. However, the resource capacity and power budget of edge devices are naturally limited. As a result, AIoT applications are based on an optimization challenge to balance
- (1) hardware cost and performance with
- (2) an optimized AI model and application design.
This is the reason why recent trends focus on AI model optimization to minimize the model size and find ways to increase the model efficacy. AI model compression is used to implement low-latency and energy-efficient model inference at the edge. The much smaller and more efficient “lightweight” ML models can run on low-power devices like mobile phones, SoC, or embedded computers. Popular examples are on-device ML model versions TensorFlow Lite or Lightweight OpenPose.
Embedded machine learning on-device transforms AIoT devices into smart, intelligent systems that can process data independently. The technical advances in different fields make it possible to apply AI technology efficiently. The newly gained flexibility and scalability of AIoT systems allow for building real-world applications that have not been possible before.
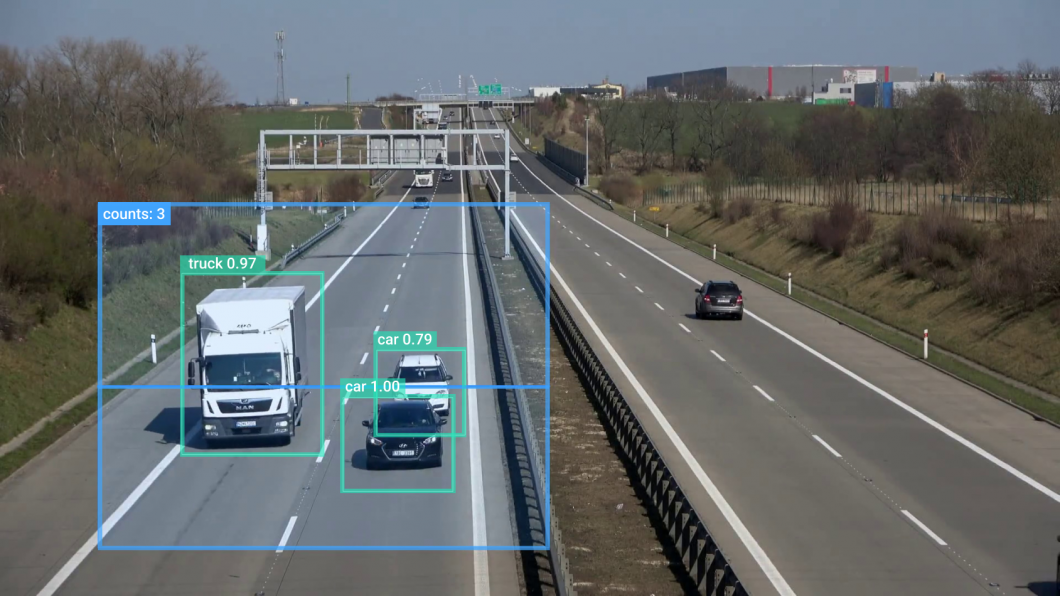
Benefits Of Combining AI With IoT
The artificial intelligence of things enables AI adoption across industries to solve real business problems more effectively than with traditional methods. There are several benefits of combining AI with IoT, the foremost being improved efficiency and reduced costs.
Boosts Operational Efficiency
AIoT enables businesses to achieve the optimum level of operational efficiency. AIoT-powered machines are capable of generating and analyzing data and recognizing patterns by applying machine learning methods. This enables it to provide operational insights quickly, detect and fix problems, as well as to increase the automation of manual processes. Hence, AI capabilities performed on repetitive tasks enable companies to provide better services with a smaller workforce.
An example is the automation of vision-based quality inspection and the use of cameras for quality control in industrial automation. Various applications aim to track and ensure adherence to guidelines and regulations (e.g., detecting personal protective equipment such as masks, helmets, vests, or gloves).
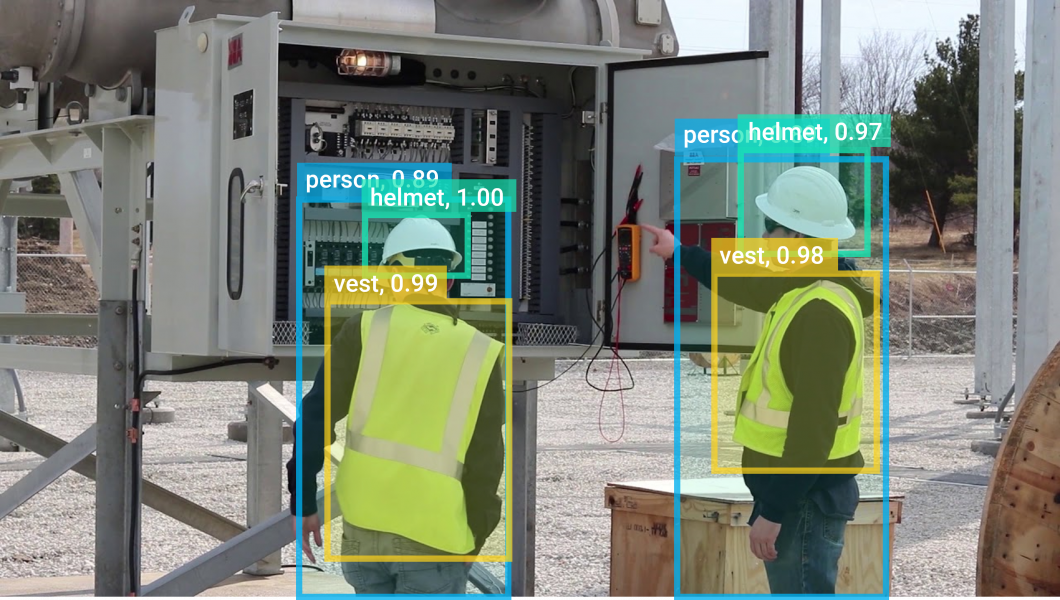
Makes Real-Time Monitoring Easier
Real-time monitoring of systems can help save time and reduce expensive business interruptions. It involves constant supervision by the system to detect anomalies and make predictions or make decisions based on the same. And that too without the need for any human intervention, achieving faster, objective results. An example is the use of industrial AI IoT in Oil and Gas, such as cameras for remote leakage detection.
Reduces Operational Costs
Intelligent AIoT devices and systems play an essential role in reducing operational costs. The development of smart systems allows for higher resource efficiency. Examples include smart building applications for adjusting light and temperature controls based on occupancy (presence of people).
AIoT devices play a crucial role in preventive maintenance and machinery analysis in smart factories. Here, sensors and cameras identify and monitor the condition of machine parts to avoid failure and expensive business interruptions (Smart Factory Applications).
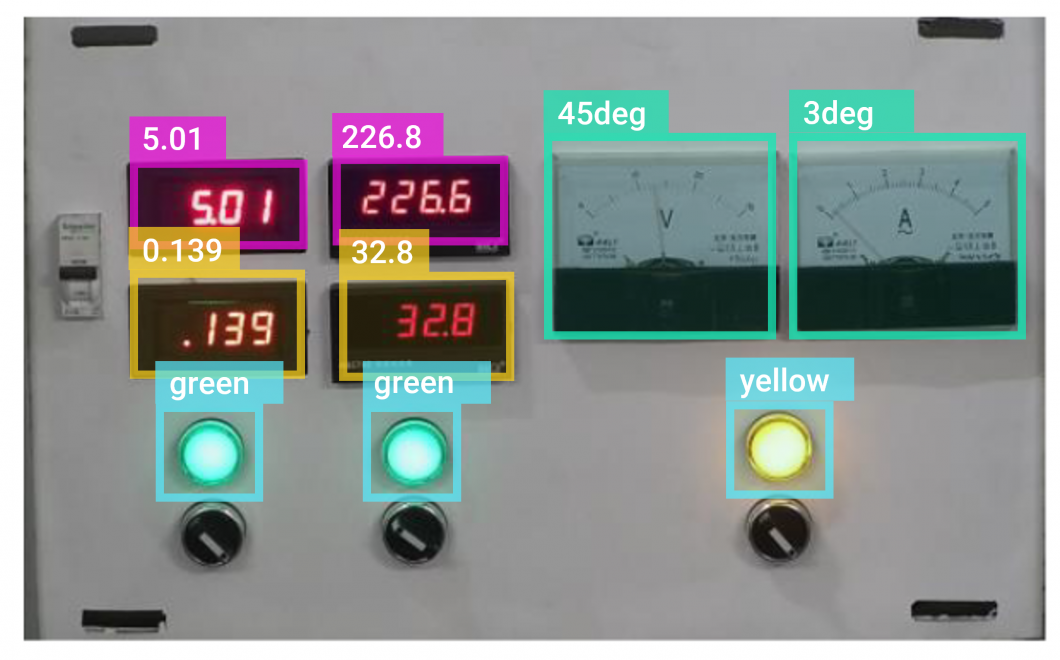
Helps In Risk Management
Risk management is important for organizations across industries. Distributed, intelligent systems are capable of predicting future risks and even taking measures for their prevention. Examples include the analysis of water levels, employee safety analysis, or crowd analysis in public places (Smarty City).
With the help of AIoT systems, organizations can stay a step ahead in preparing for and handling the probable risks in the future. Recently, insurers started to use such applications to manage insurance risks for machines and entire factories.
AIoT Applications
Integrating AI with IoT is the basis of highly scalable and efficient intelligent systems that combine software and hardware. Hence, AIoT makes it possible to develop and maintain large-scale deep learning systems.
The adoption of AIoT is an emerging technology trend across a wide range of industries, such as Logistics, Agriculture, Healthcare, Manufacturing, Oil and Gas, Retail, and Services. Specific applications in Industrial IoT (IIoT) leverage AI capabilities in smart sensing, machine vision, or predictive maintenance. In the following, we will describe some of the most popular AIoT use cases.
Automated Vehicles
Autonomous vehicles are capable of driving safely from one point to another without any human intervention. This is made possible by a range of advanced technologies, at the core of which lies AIoT. Cars by Tesla are a good example of how a combination of AI and IoT can drastically transform the automobile industry. These use sensors, gadgets, cameras, and a host of advanced technologies that work together to ensure safe automated driving.
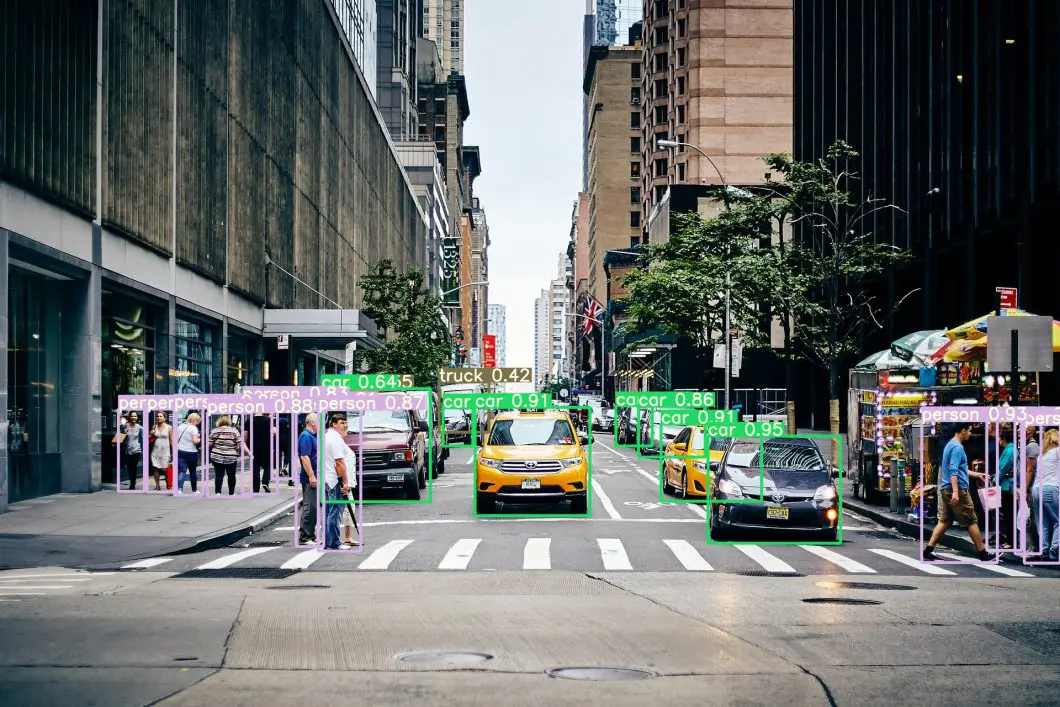
The computers of autonomous vehicles can collect and analyze information from several sources in real-time to make the most accurate driving decisions. Accordingly, autonomous vehicles are a prime example of Edge AI; distributed computers are connected over the Cloud and process the visuals without data offloading (on-device).
Video Surveillance
The incorporation of AI and IoT makes video surveillance and security smarter. With traditional video management systems (VMS), human operators are required to watch multiple video feeds. As a result, manually operated security video systems depend on subjective judgment, limited attentiveness, and inconsistent reaction times.
AIoT combines machine learning algorithms with the video feed of cameras to analyze data in real-time, detect objects, recognize people, and identify events automatically. Smart Video Surveillance is commonly applied in retail. For example, the US supermarket giant Walmart uses image recognition cameras at checkouts to detect theft. Other typical deep learning security applications include weapon detection or intrusion event detection with virtual fences.
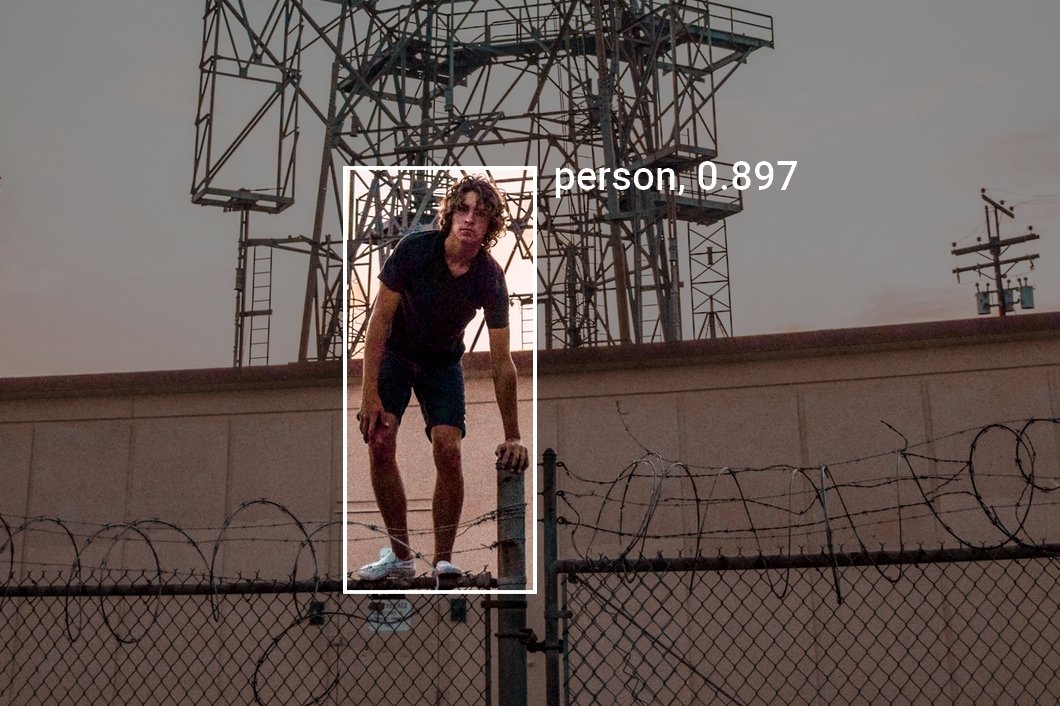
Monitoring Traffic
AIoT-based sensors and devices are widely popular for real-time traffic analysis in Smart Cities. Such AI systems include smart drones to assess congestion levels and perform crowd analysis. In transportation, computer vision-based AIoT systems automatically identify accidents, traffic violations, stopped vehicles, and more.
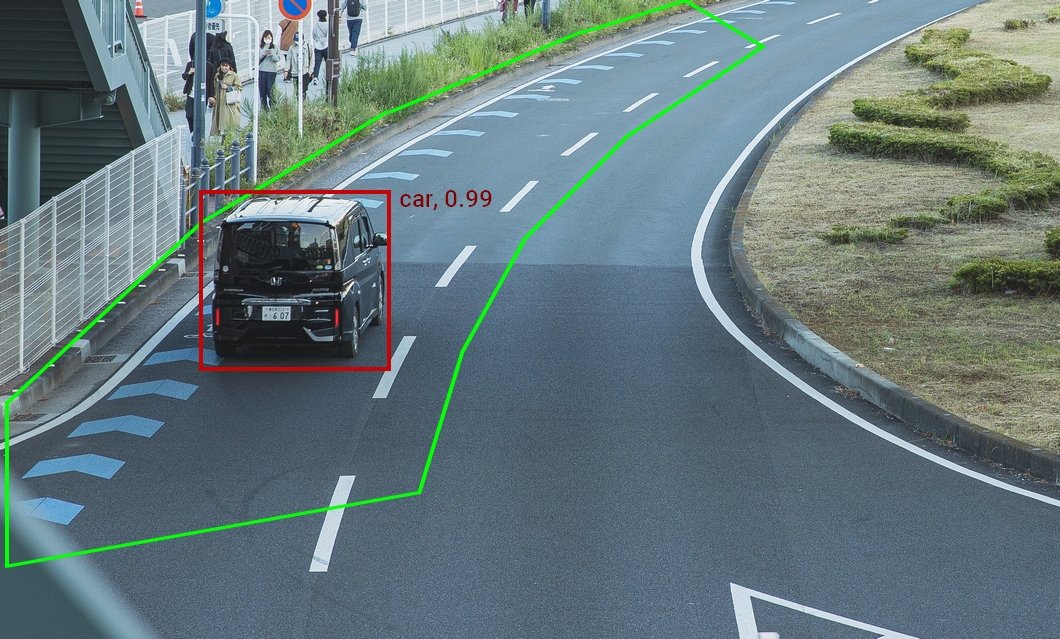
Manufacturing And Production
AIoT systems are very popular in IIoT, the Industrial Internet of Things. In manufacturing, such intelligent systems are used for real-time monitoring of machinery and to detect defective parts. Conventional machine vision systems for quality control are increasingly being replaced by modern deep learning applications. Deep neural networks are trained to detect defective parts in real time by analyzing the real-time video feed of inexpensive cameras with AI models.
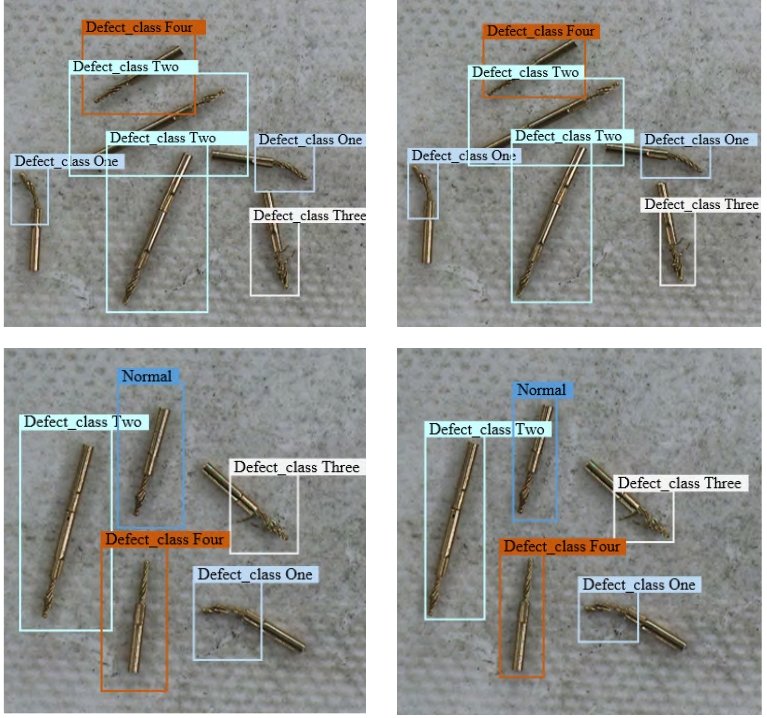
Smart Buildings
IoT sensors and cameras are commonly used to power smart building applications, for example, to detect room occupancy in real-time. Such systems are used for employee safety and security.
What’s Next
AIoT combines the power and efficiency of both AI and IoT, making it suitable for solving specific problems with distributed, intelligent systems. From retail to manufacturing, healthcare, security, oil and gas, banking, and insurance, the number of industries turning to AIoT-powered solutions is growing rapidly. In all possibilities, the technologies are all set for further advancement soon.
Get Started With AIoT Applications
At viso.ai, we enable companies across industries to build AIoT for computer vision. Viso Suite is the leading end-to-end computer vision infrastructure to build, deploy and scale their AIoT applications. It provides programming with automated, enterprise-grade infrastructure and is used by leading businesses worldwide.
Read the Viso Suite Whitepaper and get a personalized demo of our AIoT solution for computer vision.