Promptable Object Detection (POD) allows users to interact with object detection systems using natural language prompts. Thus, these systems are grounded in traditional object detection and natural language processing frameworks.
Object detection systems typically use frameworks like Convolutional Neural Networks (CNNs) and Region-based CNNs (R-CNNs). In most conventional applications, the detection tasks it must perform are predefined and static.
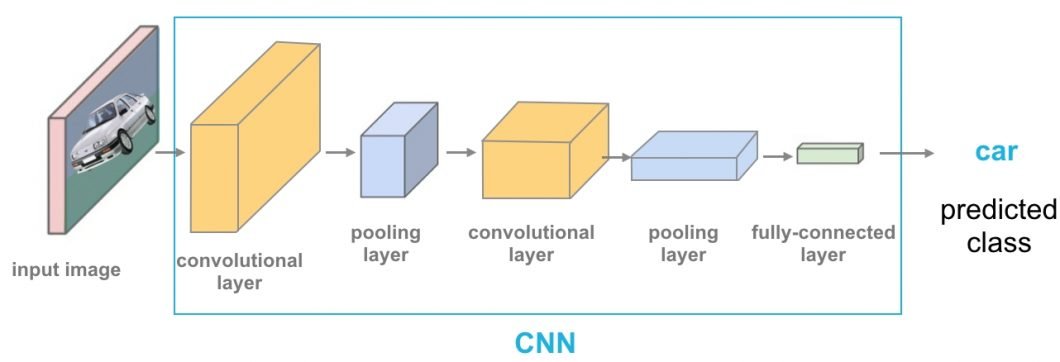
However, in prompt object detection systems, users dynamically direct the model with many tasks it may not have encountered before. Therefore, these models must have greater degrees of adaptability and generalization to perform these tasks without needing re-training.
Hence, the challenge POD systems must overcome is the inherent rigidity built into many current object detection systems. These systems are not always designed to adapt to new or unusual objects or prompts. In some cases, this may require time-consuming and resource-intensive re-training.
Detecting specific objects (object detectors) in cluttered, overlapping, or complex scenes is still a major challenge. And, in models where it’s possible, it may be too computationally expensive to be useful in everyday applications. Plus, improving these models often requires large and diverse datasets.
In this article, we’ll look at how POD systems aim to address these issues. Advancements are being made to enable more precise and contextually relevant detections with higher efficiency.
Theoretical Foundation of POD Systems
Many of the foundational deep learning models in the field of computer vision also play a key role in the development of POD:
- Convolutional Neural Networks: CNNs often serve as the primary architecture for many computer vision systems due to their efficacy in detecting patterns and features in visual imagery.
- Region-Based CNNs: As the name implies, these models excel at identifying regions where objects are likely to occur. CNNs then detect and classify the individual objects.
- You Only Look Once: YOLO can be easily installed with a pip install and processes images in a single pass. Unlike R-CNNs, it divides an image into a grid of bounding boxes with calculated probabilities. The YOLO architecture is fast and efficient, making it suitable for real-time applications like video monitoring.
- Single Shot Multibox Detector: SSD is similar to YOLO but uses multiple feature maps at different scales to detect objects. It can typically detect objects on hugely different scales with a high degree of accuracy and efficiency.
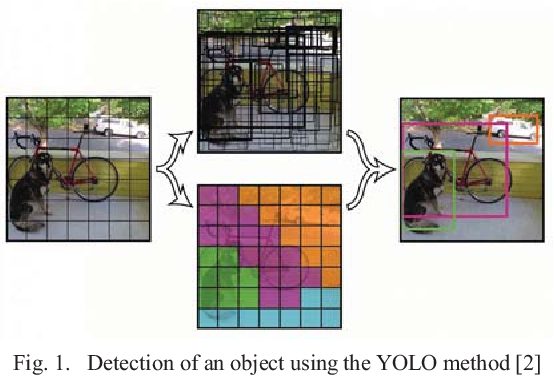
Another important concept in POD is that of transfer learning. This is the process of repurposing a model designed for a specific task to do another. Successful transfer learning helps overcome the challenge of requiring massive data sets or extensive retraining times.
In the context of POD, it allows fine-tuning pre-trained models to work on smaller, specialized detection datasets. For example, models trained on comprehensive datasets like the ImageNet database.
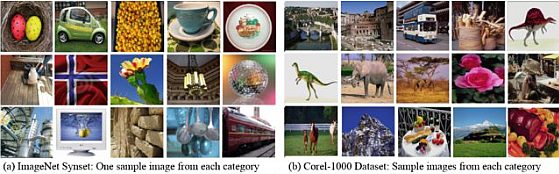
Another benefit is improving the model’s accuracy and adaptability when encountering new tasks. In particular, it improves models’ ability to recognize never-before-seen object classes and perform well under novel conditions.
Integration of Object Detection and Natural Language Processing
As mentioned, POD is a marriage of traditional object detection and Natural Language Processing (NLP). This allows for the execution of object detection tasks by human actors naturally interacting with the system.
Thanks to the outbreak of tools like ChatGPT, the general public is intimately familiar with this type of prompting. Typically, transformer-based architectures like BERT (Bidirectional Encoder Representations from Transformers) and GPT (Generative Pre-trained Transformer) serve as the foundations for these systems.
These models can interpret human prompts by analyzing both the context and content. This gives them the ability to respond in highly naturalistic ways and execute complex instructions. With impressive generalization, they are also adept at completing novel instructions on a grand scale.
In particular, BERT’s bidirectional training gives it an even more accurate and nuanced understanding of context. On the other hand, GPT has more advanced generative capabilities, with the ability to produce relevant follow-up prompts. PODs can use the latter to produce an even more interactive experience.
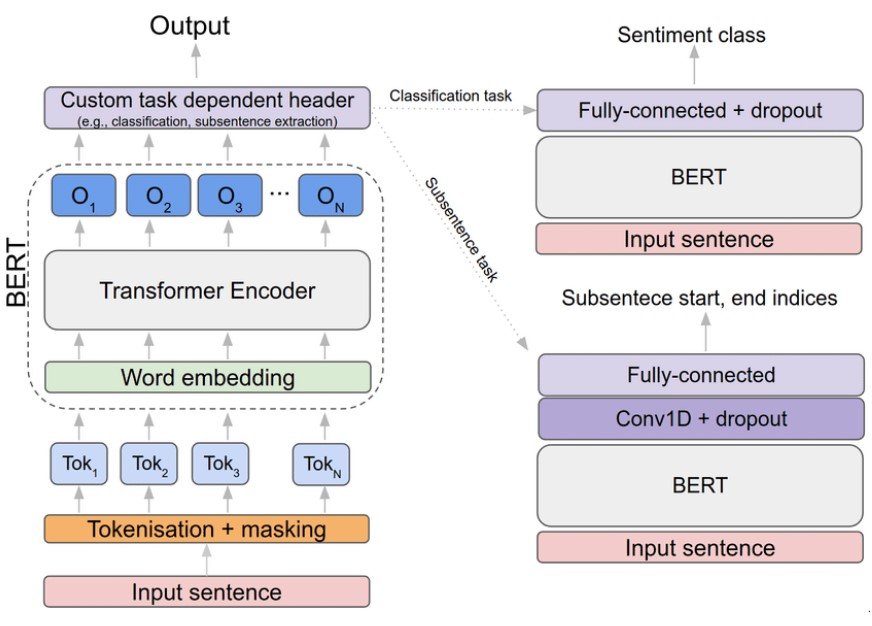
The root of what we’re trying to get here is the semantic understanding of prompts. Sometimes, it’s not enough to execute prompts based on a direct interpretation of the words. Models must also be capable of discerning the underlying meaning and intent of queries.
For example, a user may issue a command like “Identify all red vehicles moving faster than the speed limit in the last hour.” First, the system needs to break it up into its key components. In this case, it may be “identify all,” “red vehicle,” “moving faster than the speed limit,” and “in the last hour.”
The color “red” is tagged as an attribute of interest, “vehicles” as the object class to be detected, “moving faster than” as the action, and “speed limit” as a contextual parameter. “In the last” hour is another filterable variable, placing a temporal constraint on the entire search.
Individually, these parameters may seem simple to deal with. However, collectively, there is an interplay of ideas and concepts that the system needs to orchestrate to generate the correct output.
Frameworks and Tools for Promptable Object Detection
Today, developers have access to a large stack of ready-made AI software and libraries to develop POD systems. For most applications, TensorFlow and PyTorch are still the gold standard in deep learning. Both are backed by a comprehensive ecosystem of technologies and are designed for rapid prototyping and testing.
TensorFlow even features an object detection API. It has a depth of pre-trained models and tools that one can easily adapt for POD applications to create interactive experiences.
PyTorch’s value stems from its dynamic computation graphs, or “define-by-run” graphs. This enables on-the-fly readjustment of the model’s architecture in response to prompts. For example, when a user submits a prompt that requires a novel detection feature, the model can adapt in real-time. It alters its neural network pathways to accurately interpret and execute the prompt.
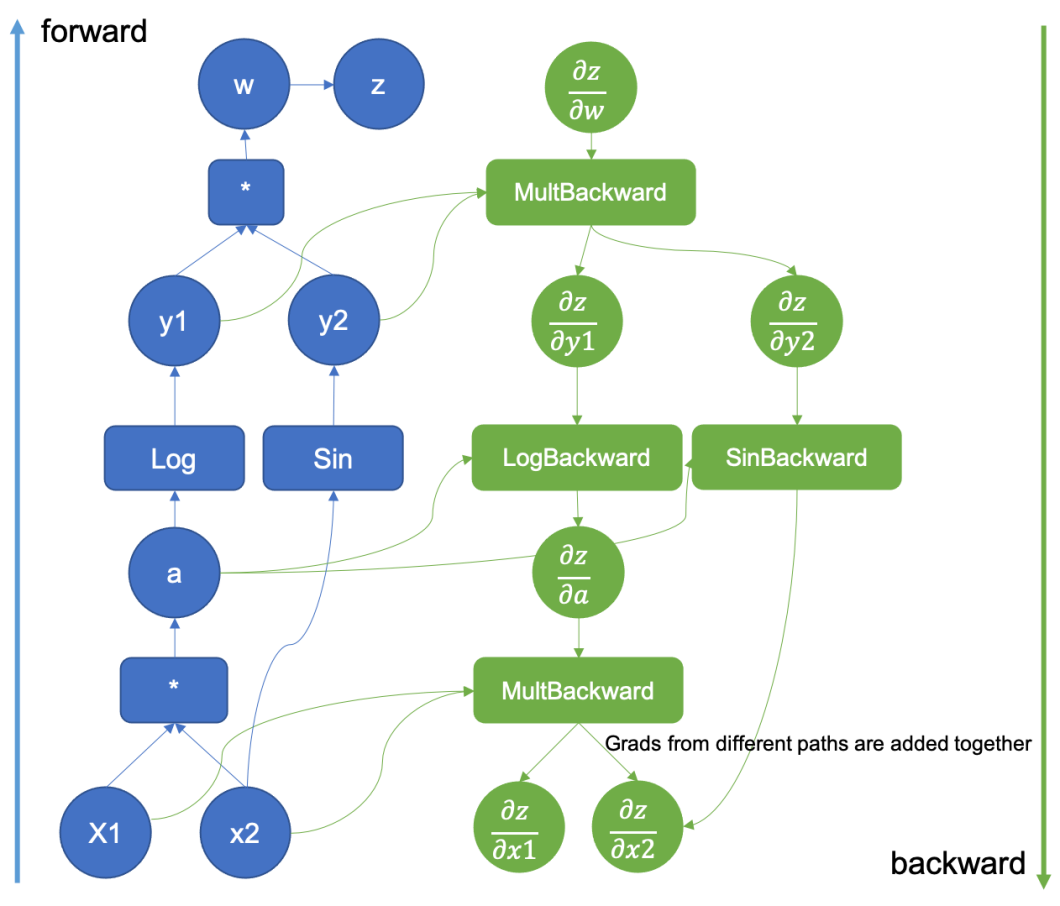
Both these features make these models attractive for real-world applications. TensorFlow, for its ease of deployment and development. PyTorch, for its ability to respond to a vast spectrum of human-language queries.
OpenCV, on the other hand, offers a comprehensive computer vision toolkit for expanding a system’s scope. Specifically for POD applications, it offers easy integration for functions like: