Machine learning and deep learning can be used to train computers to learn by example – or receiving, processing, and filtering complex information with all five senses to produce a final output (similar to a human brain). It achieves incredible accuracy rates, ensuring products can be implemented in the real world safely.
From the virtual assistants in our phones to disease diagnostics, we can see deep learning networks everywhere. Here, we will cover the three most popular and progressive applications of deep learning as well as how they can be used together. Each of these applications poses valuable use cases for businesses across industries. While different, as technology progresses, these applications can also be combined and used in unison to solve everyday issues.
- Computer Vision (CV)
- Natural Language Processing (NLP)
- Audio Signal Processing (ASP)
- What’s next?
About us: Viso.ai provides the leading end-to-end Computer Vision Platform Viso Suite. Global organizations use our solution to develop, deploy, and scale their computer vision applications in one place, with automated infrastructure. Get a personal demo.
Application 1: Computer Vision
One exemplary application of deep learning in computer vision. Computer vision is a field under deep and machine learning that allows computers to gain a high-level understanding of digital images or videos. It is now the fastest-growing sub-field and is applied to a wide range of use cases.
Computer Vision applications are rapidly gaining in importance across industries, prominently in manufacturing, automotive, oil and gas, retail, logistics, smart city, medical image analysis, and agriculture. Such intelligent AI vision systems combine Artificial Intelligence (AI) with the Internet of Things (AIoT) and are built to collect video data from distributed camera sensors to interpret images with machine learning.
Most commercial AI vision systems are highly specialized and developed to automate visual inspection, remote monitoring, quality control, surveillance and security, organizational health, and safety, as well as to increase operational efficiency. Deep learning models can autonomously analyze the video stream of basically any camera sensor.
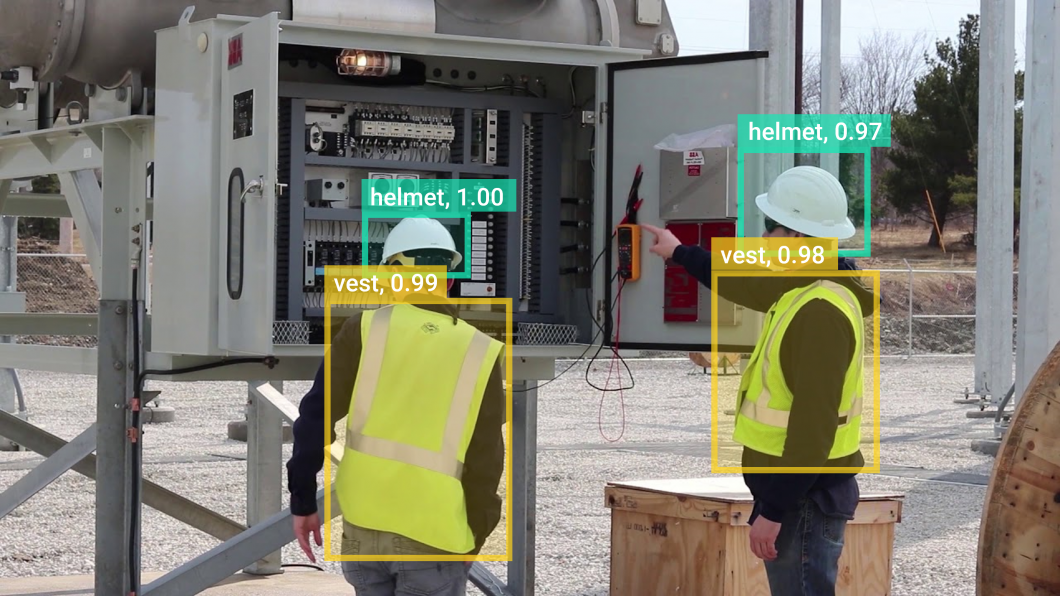
A recent trend named Edge AI allows deploying and running Deep Learning on physical Edge Devices, computers, or edge servers. The implementation of such distributed AI systems allows privacy-preserving, high scalability, and cost efficiency achieved through on-device computer vision inference.
Instead of sending the videos to the cloud, all videos are analyzed in near real-time, and only valuable metadata is collected in the cloud. A well-known example of the need for real-time data processing is that of self-driving cars and pedestrian, vehicle, and obstacle detection on the road. Read about the advantages of Edge AI for Computer Vision.
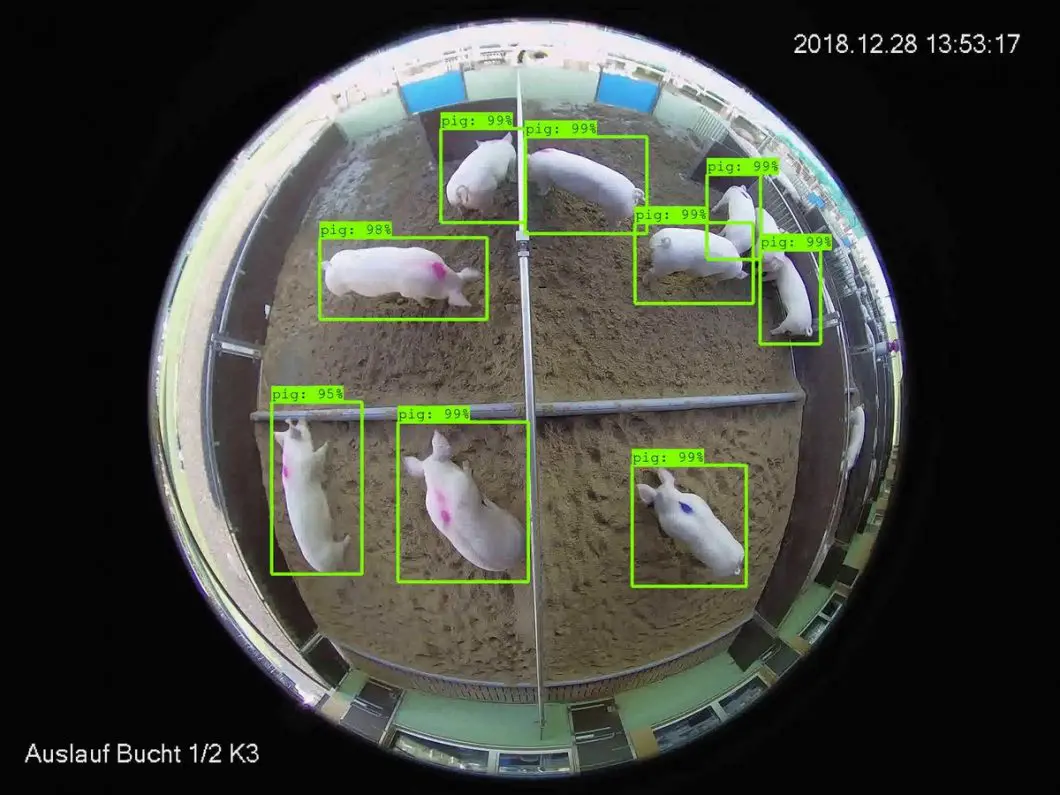
Popular Computer Vision Platforms and Tools
- Viso Suite: Viso Suite infrastructure allows enterprises to easily implement computer vision into their business applications. By consolidating the entire machine learning lifecycle into a single interface, Viso Suite allows ML teams to gain full application management. Thus, eliminating the need for point solutions.
- OpenCV: Built as an open-source library, OpenCV contains over 2500 algorithms, documentation, source code, and sample code for real-time computer vision. It is easy for developers to get started with computer vision by using the OpenCV Python package, making the tool extremely popular.
- OpenVINO: “Open Visual Inference and Neural Network Optimization” is the cross-platform, open-source learning toolkit from Intel. OpenVINO simplifies the model deployment process and optimizes neural network inference for several deep learning tasks.
- YOLO: the “You Only Look Once” model series is a popular set of open-source algorithms for various computer vision tasks. Originally developed by Joseph Redmon in 2015, there have been numerous official and unofficial versions later released by the community, including YOLOX, YOLOv3, YOLOv5, YOLOv7, YOLOv8, and YOLOv9.
To learn more about computer vision tools, check out our article on the topic.
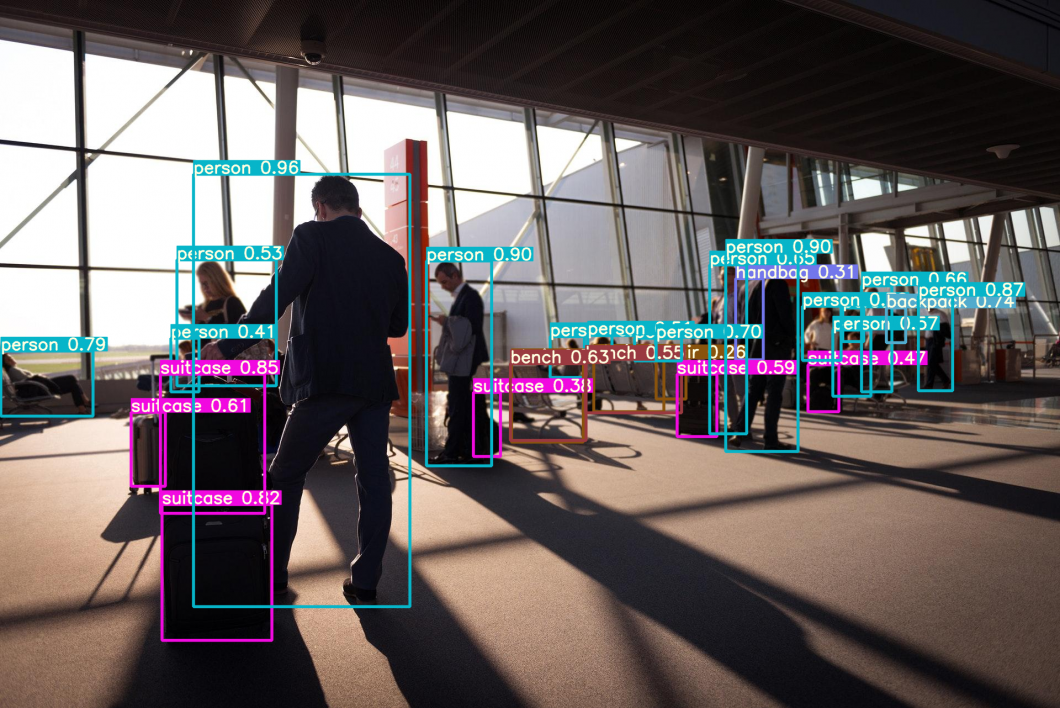
Application 2: Natural Language Processing (NLP)
Natural Language Processing, otherwise known as NLP, is another popular segment of deep learning. NLP merges artificial intelligence with human language allowing for use cases like language translation, conversational chatbots, and more. Because of the nuances and intricacies of language, NLP is seen as one of the most complex and difficult deep learning algorithms types to create.
For example, one word can take on several meanings in some languages, and NLP needs to be designed to recognize the surrounding context of that word and associate it with the correct meaning.
NLP is implemented using three main tactics: Part of Speech (PoS), parse trees, and semantics. In short, PoS defines the functions of individual words. Meanwhile, parse trees are used to determine the syntax of sentences (differentiating between verbs, nouns, adjectives, etc.).
With semantics, the computer learns to read through and understand the context (previous sentences) to deduce the appropriate meaning for a word.
The following image shows a great example application of NLP used to analyze notes of medical records to detect and remove or obfuscate sensitive personal identifying information (PII) and protected health information (PHI):
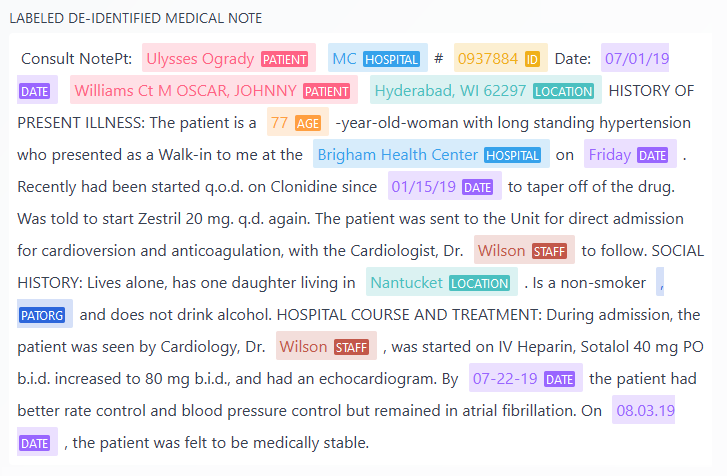
NLP has been used to create YouTube’s auto-captioning system or Apple’s Siri. Still, it is much less common when compared to other fields, such as computer vision. However, NLP remains a valuable application of deep learning.
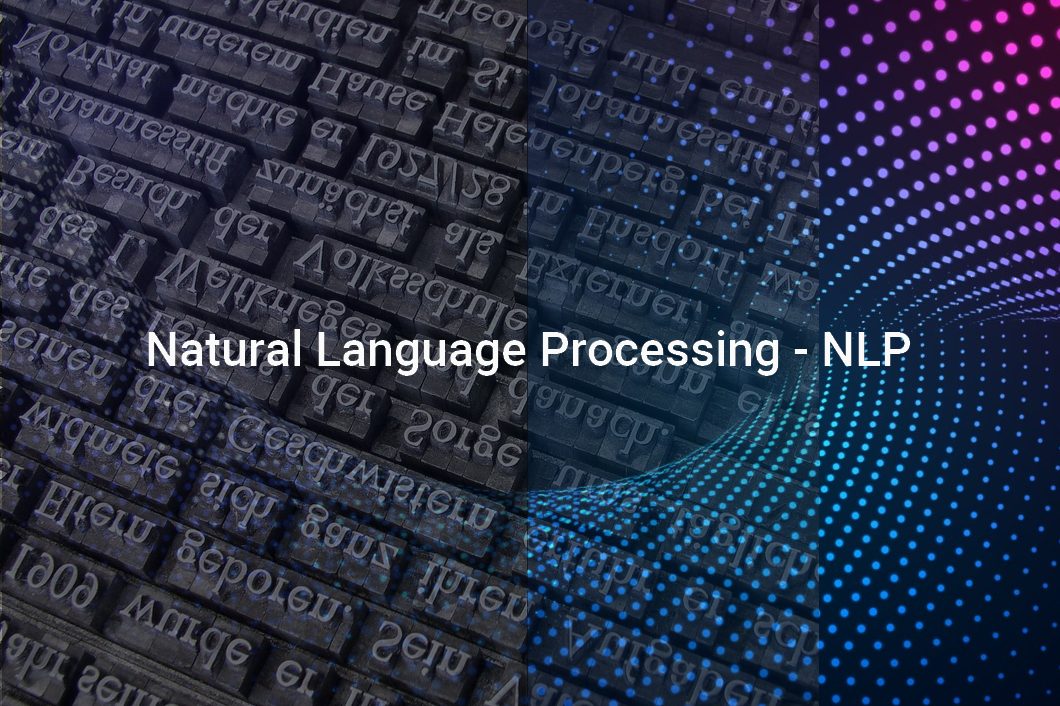
Popular Natural Language Processing (NLP) Platforms and Tools
- ChatGPT: ChatGPT is a massively popular tool developed by OpenAI. Developed as arguably the most advanced chatbot, users provide ChatGPT with a textual prompt, which the bot uses to create a human-like conversational interaction. It is based on the GPT foundation models, namely GPT-3.5 and GPT-4.0, which make conversational usage possible with fine-tuning of the large language models (LLMs).
- Transformers (Hugging Face): Hugging Face’s Transformers is a popular library for working with pre-trained language models such as BERT, GPT, and RoBERTa. Transformers make fine-tuning and deploying state-of-the-art language models easy for various NLP tasks like text classification, question answering, and language generation.
- spaCy: spaCy is a modern NLP library for Python production use. It offers efficient tokenization (entity recognition (NER)), dependency parsing, and other tasks. spaCy is fast, accurate, and easy to use, making it popular for building NLP pipelines in real-world applications.
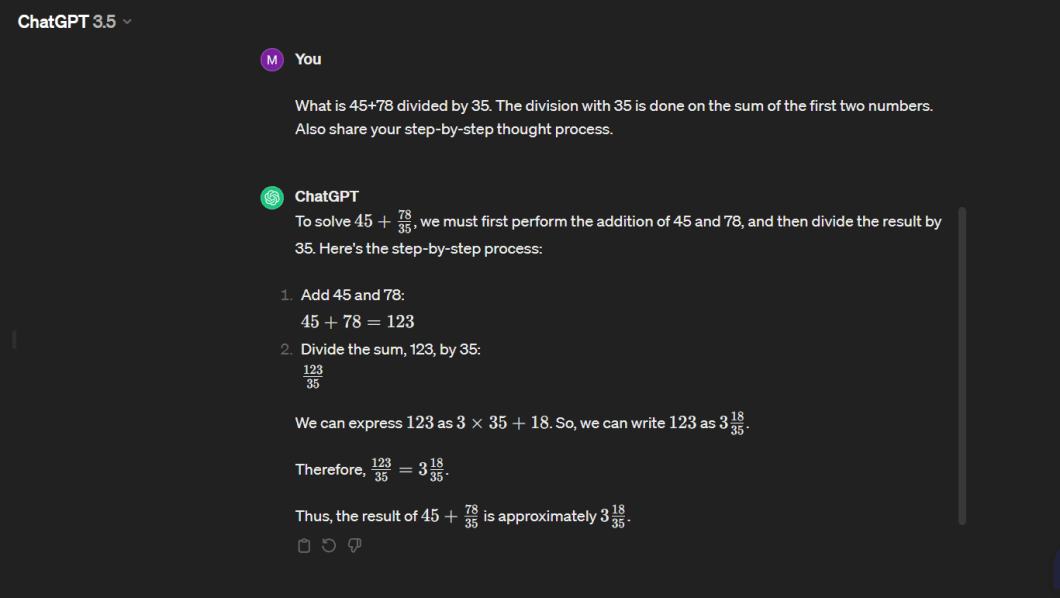
Application 3: Audio Signal Processing (ASP)
Audio Signal Processing (ASP) in artificial intelligence is the process of applying algorithms and techniques to extract meaningful information from audio signals. ASP also involves using AI-based methods such as deep learning, reinforcement learning, and machine learning to process audio signals.
With the advances in technology, audio signal processing is increasingly used to build voice search and voice-activated programs. ASP often works in combination with NLP systems. Audio recognition is a large aspect of ASP, as it uses many of the same programming techniques to create. We encounter Audio Signal Processing around in automatic speech recognition when we get a voice message that gets automatically transcribed into a script by our phones.
This technology can be applied in a variety of applications, such as sound engineering and music production. In addition, ASP techniques are becoming increasingly popular in consumer electronics, where they are used to improve the sound quality of speakers, headphones, and other devices. Furthermore, it is being applied in healthcare and medical fields to detect abnormalities in speech and language, as well as in biometric applications to recognize voice patterns.
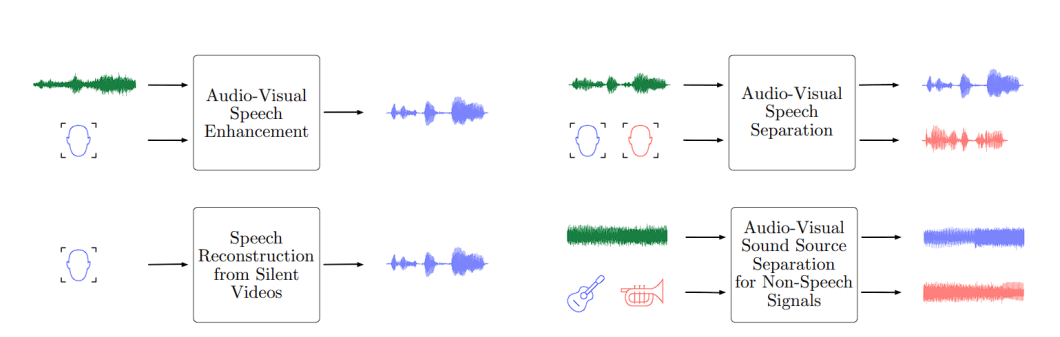
Popular Audio Signal Processing (ASP) Platforms and Tools
- MATLAB: MATLAB provides a rich set of built-in functions and toolboxes for tasks such as audio file read/write, signal filtering, spectral analysis, time-frequency analysis, and audio synthesis. MATLAB is popular for prototyping, algorithm development, and data analysis in audio processing applications.
- Audacity: Audacity is an open-source audio editing and recording software. It offers a user-friendly interface and features for editing, recording, and processing audio files, including multi-track editing, audio effects (such as equalization, noise reduction, and reverb), and audio analysis tools (such as spectrograms and waveforms).
- Adobe Audition: Adobe Audition is a professional digital audio workstation (DAW) software developed by Adobe. It offers tools for audio editing, mixing, mastering, and restoration. Adobe Audition provides advanced non-destructive editing, multi-track recording, real-time effects processing, spectral editing, and audio restoration features, useful for music creation.
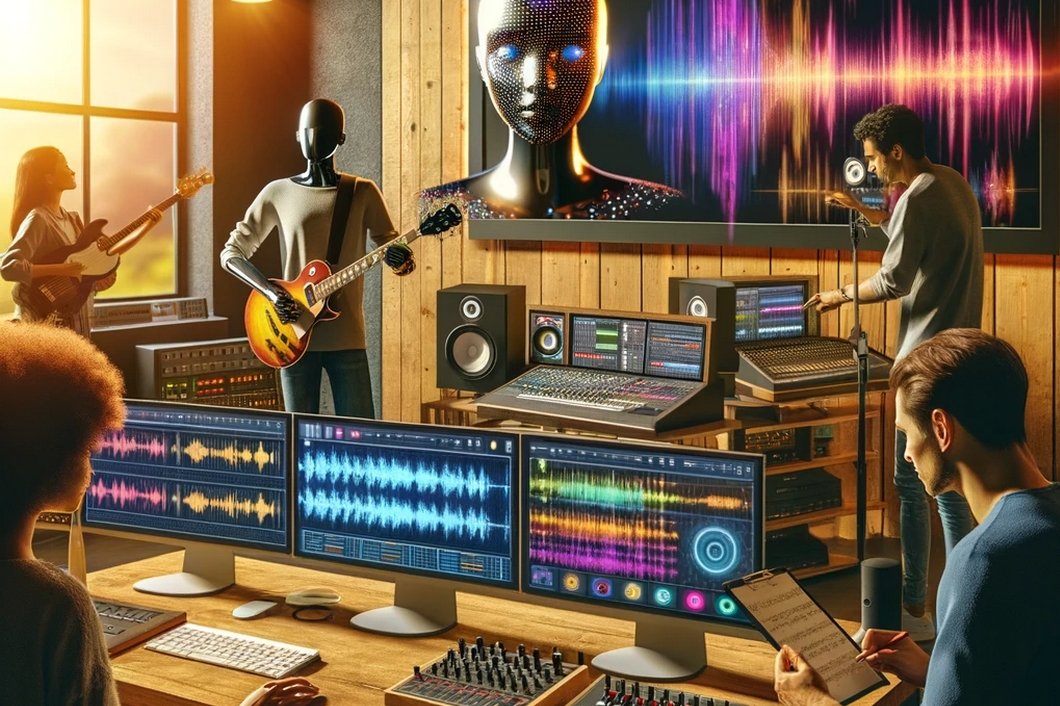
Combining Computer Vision and Natural Language Processing (NLP)
A prime example of using both computer vision and NLP is promptable object detection, where computer vision algorithms analyze visual content to glean pertinent details from images. On the other side of this NLP models act to explain what is going on in those images. This makes it possible for systems to carry out tasks such as tagging photos, suggesting content, and describing visuals to assist visually impaired people.
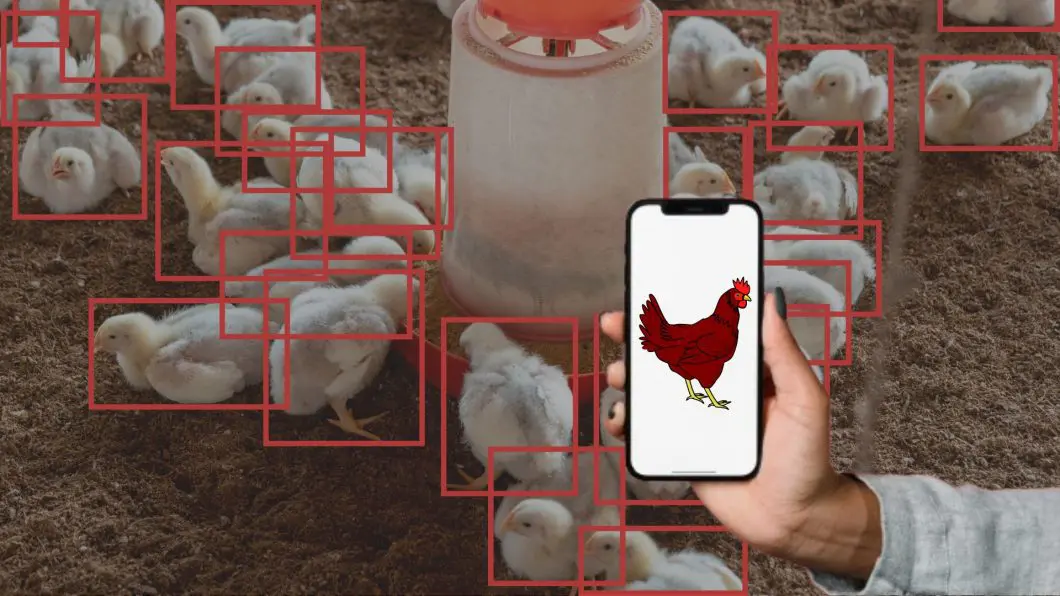
Additionally, computer vision paired with NLP can facilitate sentiment analysis for visual content. The deep learning system can extract the underlying sentiment expressed in text, i.e. social media comments or product reviews, and compare this with emotional cues and visual context in accompanying images. This approach can lead to improved brand monitoring, market research, and content moderation on social media platforms.
Combining Computer Vision and Audio Signal Processing (ASP)
Computer vision and ASP can be combined in use cases like live stream monitoring and video content analysis. While the computer vision algorithm identifies objects, actions, or events, the ASP system can extract audio and sound features, giving context to the video content. This can aid in use cases such as content recommendation, video summarization, and event detection in surveillance systems.
Human-computer interaction with robotics is another use case combining audio and visual aspects of AI. When dealing with smart home devices, cameras and microphones can recognize user gestures, facial expressions, and commands, enabling multimodal interactions with smart devices and appliances. This will help enhance the usability of home robots and appliances.
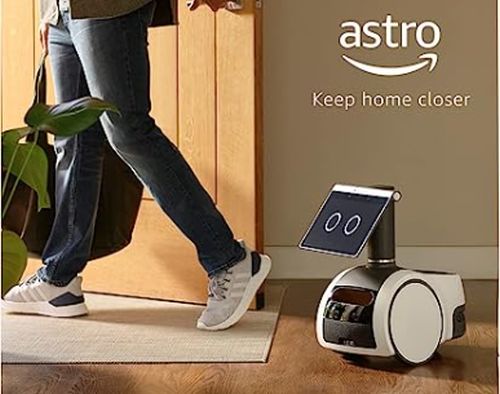
Combining Natural Language Processing (NLP) and Audio Signal Processing (ASP)
For speech recognition and transcription, ASP techniques can convert spoken words into digital signals, while NLP algorithms analyze these signals to transcribe and understand the spoken language. This use case is particularly valuable in improving the quality of voice-controlled assistants, dictation software, and automatic transcription services.
Another example would be sentiment analysis of audio content. Here, NLP can be applied to give context to the emotional tone of language expressed in audio snippets. The ASP system would analyze the pitch, intonation, and speech rate, while the NLP system would assign sentiment polarity and emotional cues to those acoustic details. This process can improve customer sentiment analysis, voice-based emotion recognition, and personalized feedback systems.
What’s Next with Deep Learning Applications?
ASP, NLP, and Computer Vision are extremely powerful and fast-growing applications of deep learning. Read more about computer vision news and technologies:
- The Ultimate List of Computer Vision Applications
- Deep Neural Network: The 3 Popular Types (MLP, CNN, and RNN)
- An Introduction to Artificial Neural Networks (ANNs)
- Image Segmentation with Deep Learning
- Fraud Detection with Computer Vision
- Not Enough Training Data? Synthetic Data Provides a Model Training Solution
Viso Suite infrastructure allows enterprise teams to build full-scale solutions with computer vision. Viso Suite consolidates the entire machine learning lifecycle into a single interface, thus eliminating the need for point solutions. Additionally, Viso Suite integrates seamlessly into existing tech stacks, making it possible for ML teams to start realizing value from computer vision in just three days. Find out how Viso Suite can help automate your business processes with computer vision by booking a demo.
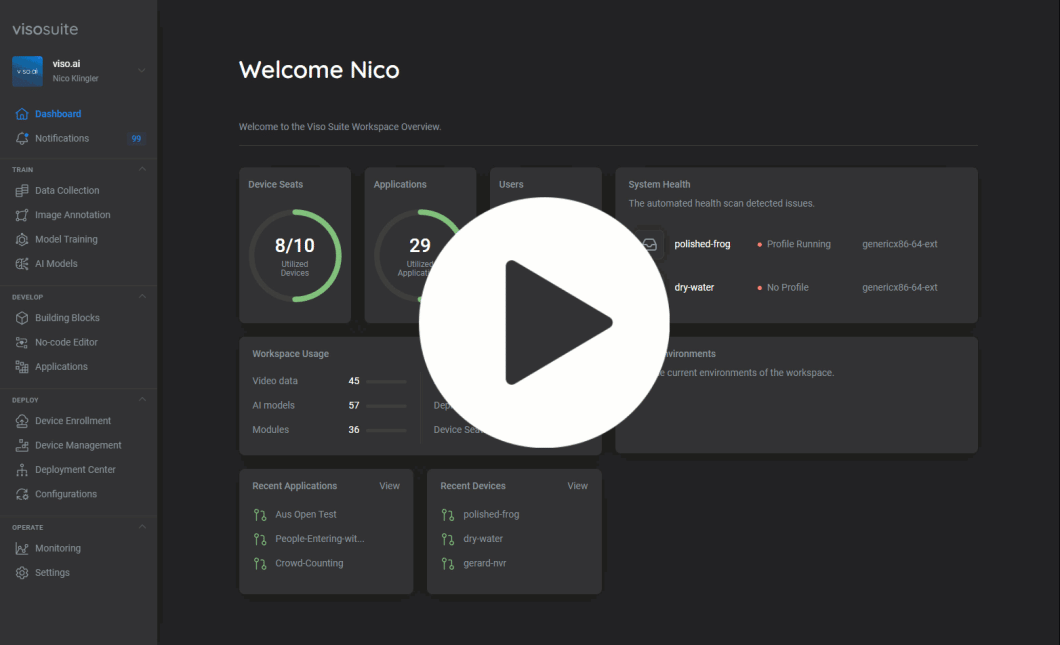